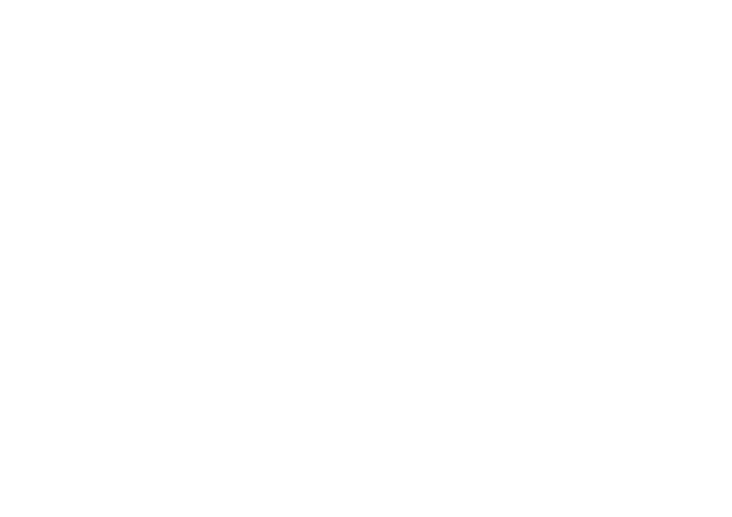
Our mission
Steering transformative technology towards benefitting life and away from extreme large-scale risks.
We believe that the way powerful technology is developed and used will be the most important factor in determining the prospects for the future of life. This is why we have made it our mission to ensure that technology continues to improve those prospects.
Just launched
The
Autonomous Weapons Newsletter
Stay up-to-date on the autonomous weapons space with a monthly newsletter covering policymaking efforts, weapons systems technology, and more.
Sign up to the newsletter
Our mission
Ensuring that our technology remains beneficial for life
Our mission is to steer transformative technologies away from extreme, large-scale risks and towards benefiting life.
Read more
How certain technologies are developed and used has far-reaching consequences for all life on earth.
If properly managed, these technologies could change the world in a way that makes life substantially better, both for those alive today and those who will one day be born. They could be used to treat and eradicate diseases, strengthen democratic processes, mitigate - or even halt - climate change and restore biodiversity.
If irresponsibly managed, they could inflict serious harms on humanity and other animal species. In the most extreme cases, they could bring about the fragmentation or collapse of societies, and even push us to the brink of extinction.
The Future of Life Institute works to reduce the likelihood of these worst-case outcomes, and to help ensure that transformative technologies are used to the benefit of life.
Our missionIf properly managed, these technologies could change the world in a way that makes life substantially better, both for those alive today and those who will one day be born. They could be used to treat and eradicate diseases, strengthen democratic processes, mitigate - or even halt - climate change and restore biodiversity.
If irresponsibly managed, they could inflict serious harms on humanity and other animal species. In the most extreme cases, they could bring about the fragmentation or collapse of societies, and even push us to the brink of extinction.
The Future of Life Institute works to reduce the likelihood of these worst-case outcomes, and to help ensure that transformative technologies are used to the benefit of life.
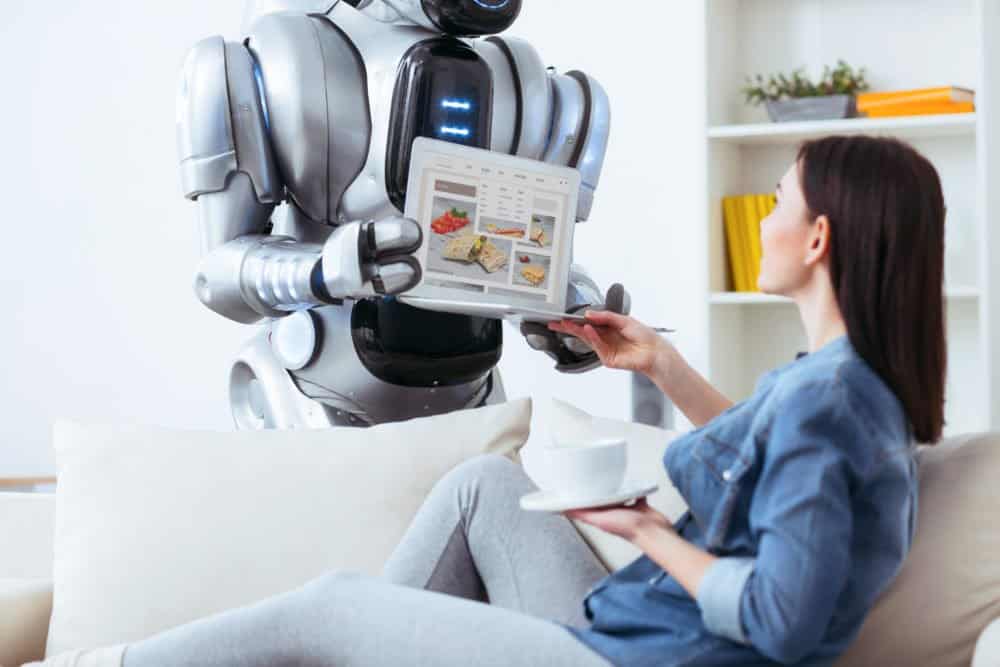
Cause areas
The risks we focus on
We are currently concerned by three major risks. They all hinge on the development, use and governance of transformative technologies. We focus our efforts on guiding the impacts of these technologies.
Artificial Intelligence
From recommender algorithms to chatbots to self-driving cars, AI is changing our lives. As the impact of this technology grows, so will the risks.
Artificial Intelligence
Biotechnology
From the accidental release of engineered pathogens to the backfiring of a gene-editing experiment, the dangers from biotechnology are too great for us to proceed blindly.
Biotechnology
Nuclear Weapons
Almost eighty years after their introduction, the risks posed by nuclear weapons are as high as ever - and new research reveals that the impacts are even worse than previously reckoned.
Nuclear Weapons
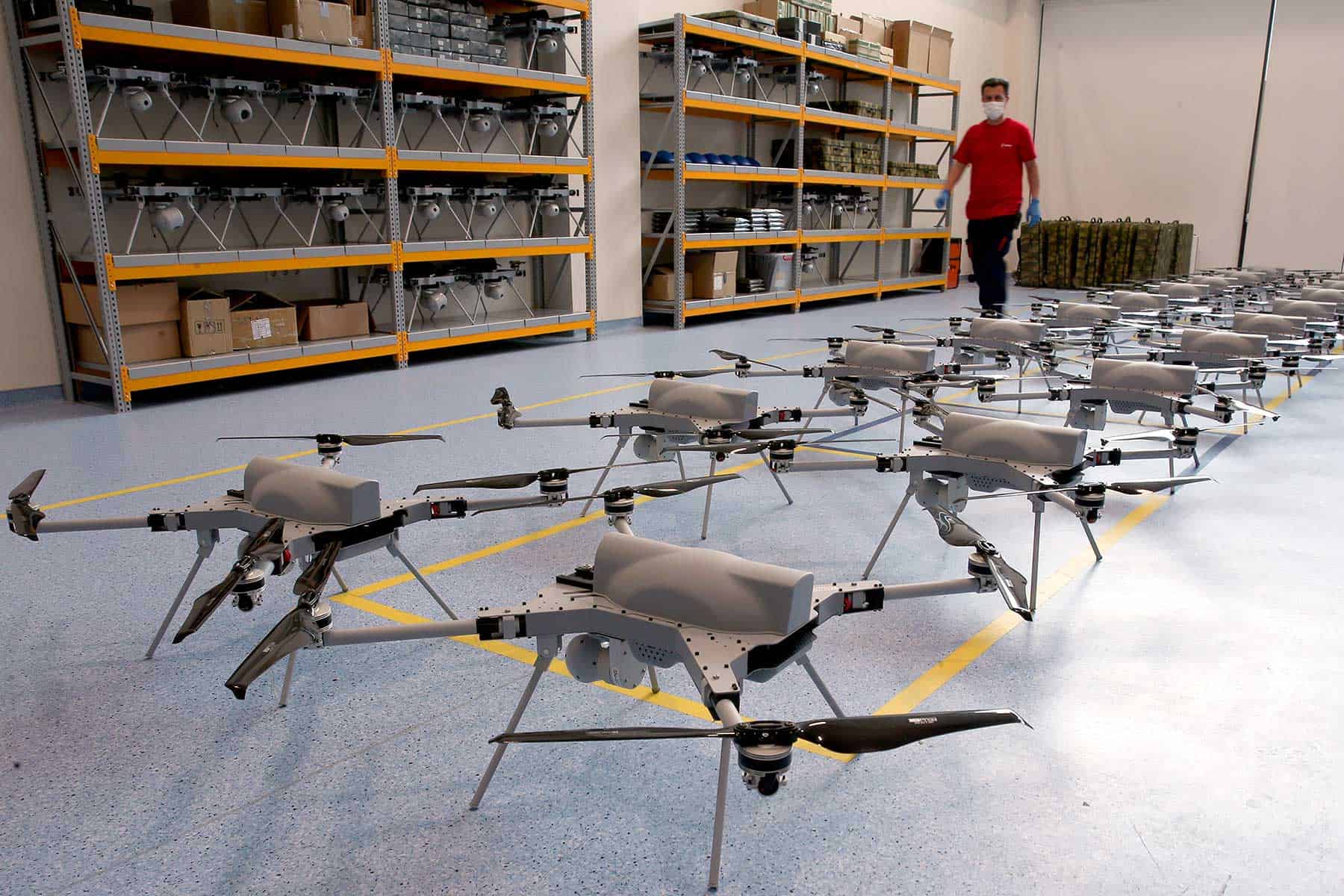
UAV Kargu autonomous drones at the campus of OSTIM Technopark in Ankara, Turkey - June 2020.
Our work
How we are addressing these issues
There are many potential levers of change for steering the development and use of transformative technologies. We target a range of these levers to increase our chances of success.
Policy
We perform policy advocacy in the United States, the European Union, and the United Nations.
Our Policy workOutreach
We produce educational materials aimed at informing public discourse, as well as encouraging people to get involved.
Our Outreach workGrantmaking
We provide grants to individuals and organisations working on projects that further our mission.
Our Grant ProgramsEvents
We convene leaders of the relevant fields to discuss ways of ensuring the safe development and use of powerful technologies.
Our EventsFeatured Projects
What we're working on
Read about some of our current featured projects:
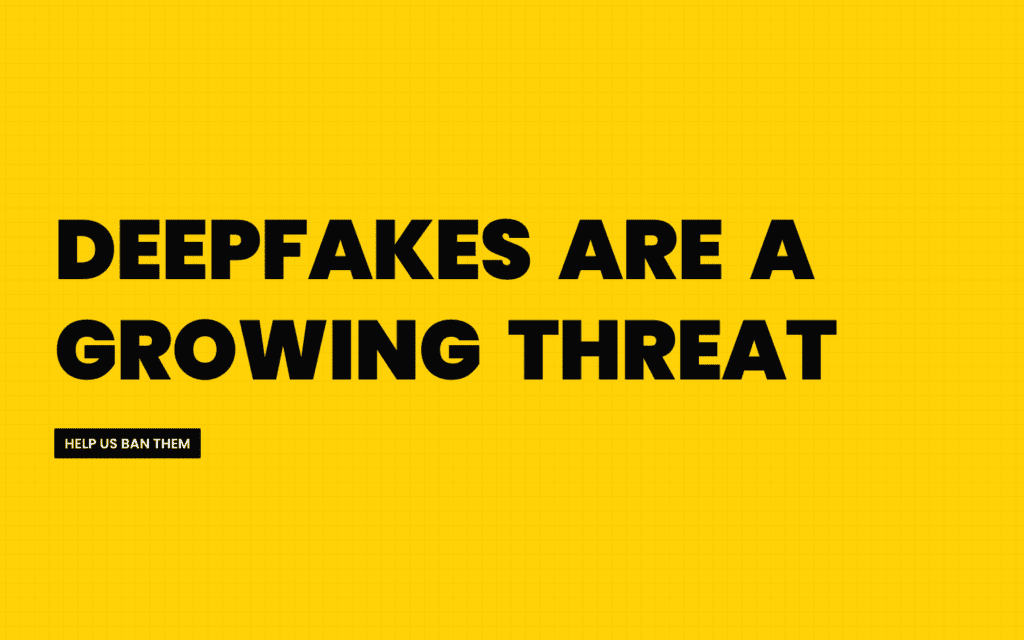
Ban Deepfakes
2024 is rapidly turning into the Year of Fake. As part of a growing coalition of concerned organizations, FLI is calling on lawmakers to take meaningful steps to disrupt the AI-driven deepfake supply chain.
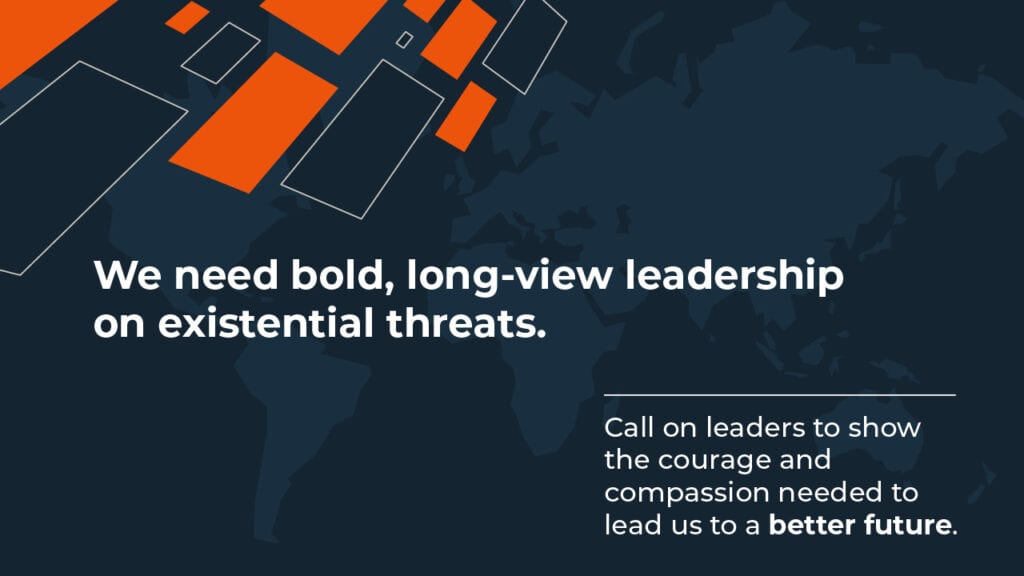
The Elders Letter on Existential Threats
The Elders, the Future of Life Institute and a diverse range of preeminent public figures are calling on world leaders to urgently address the ongoing harms and escalating risks of the climate crisis, pandemics, nuclear weapons, and ungoverned AI.
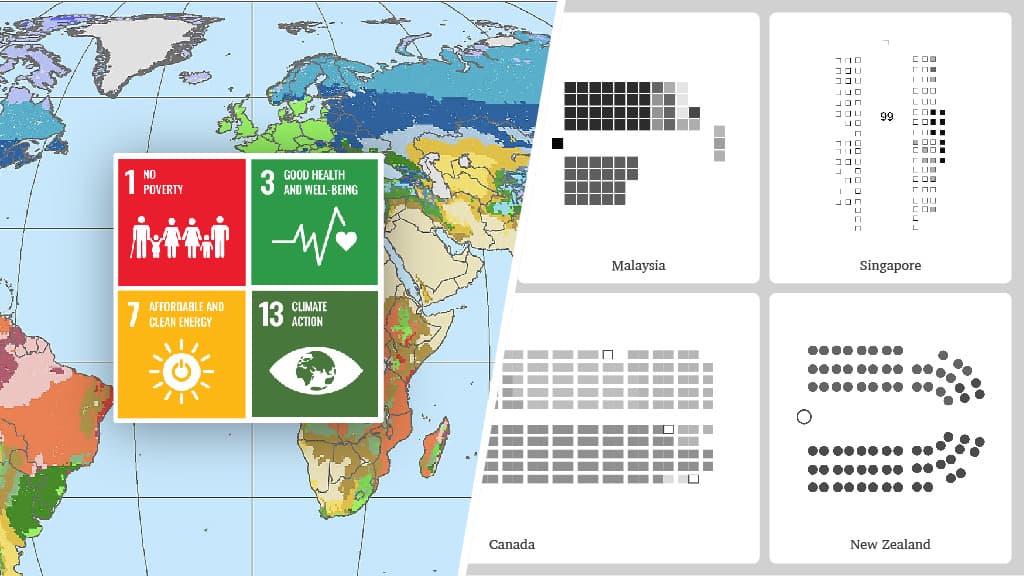
Realising Aspirational Futures – New FLI Grants Opportunities
We are opening two new funding opportunities to support research into the ways that artificial intelligence can be harnessed safely to make the world a better place.
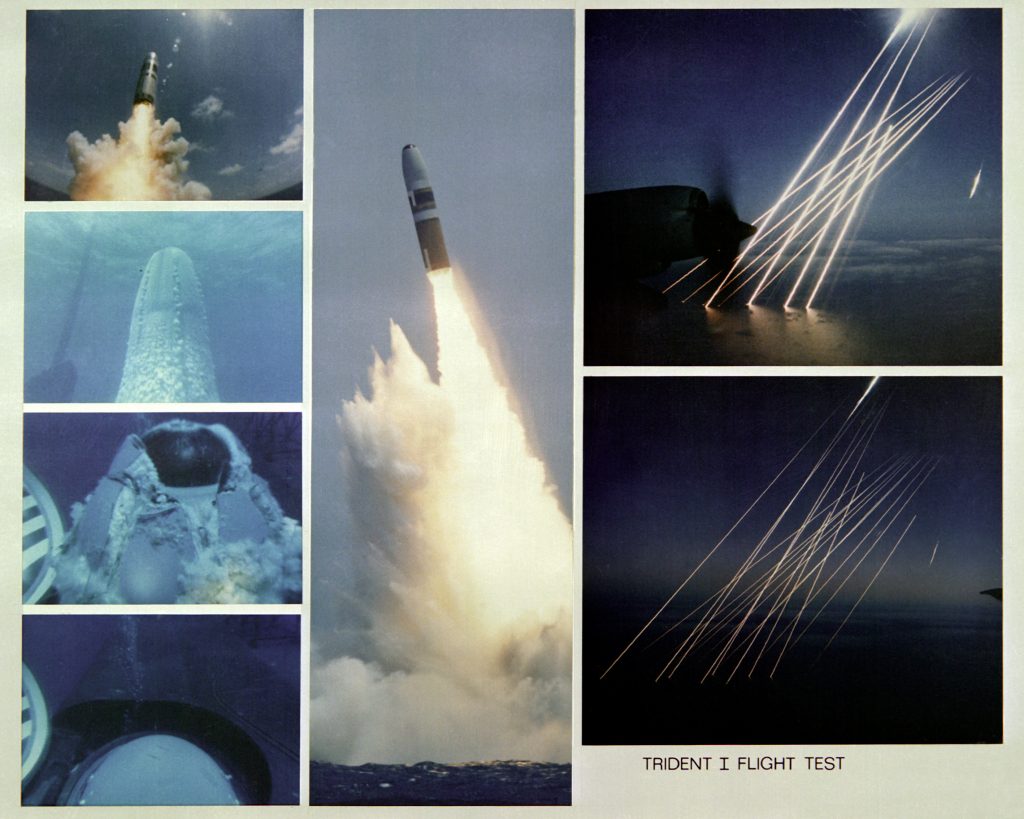
Mitigating the Risks of AI Integration in Nuclear Launch
Avoiding nuclear war is in the national security interest of all nations. We pursue a range of initiatives to reduce this risk. Our current focus is on mitigating the emerging risk of AI integration into nuclear command, control and communication.
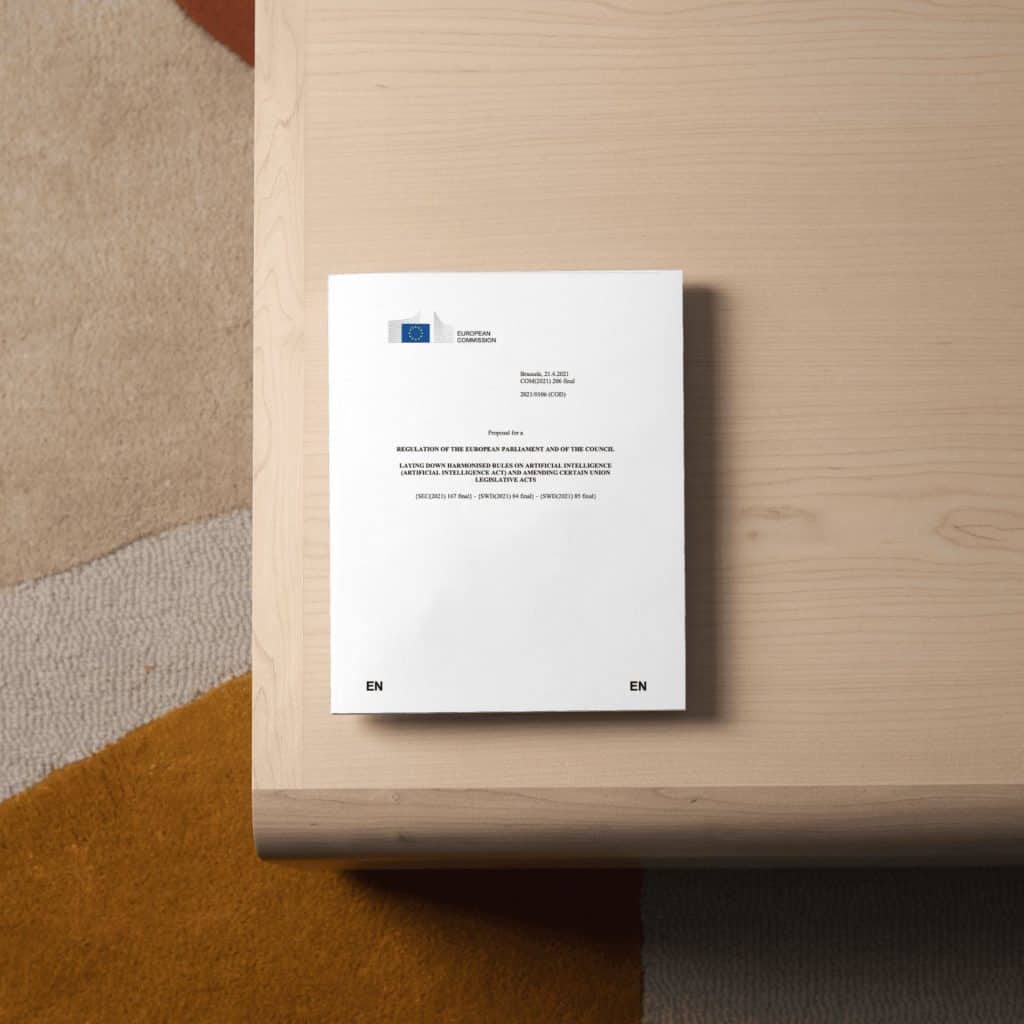
Strengthening the European AI Act
Our key recommendations include broadening the Act’s scope to regulate general purpose systems and extending the definition of prohibited manipulation to include any type of manipulatory technique, and manipulation that causes societal harm.
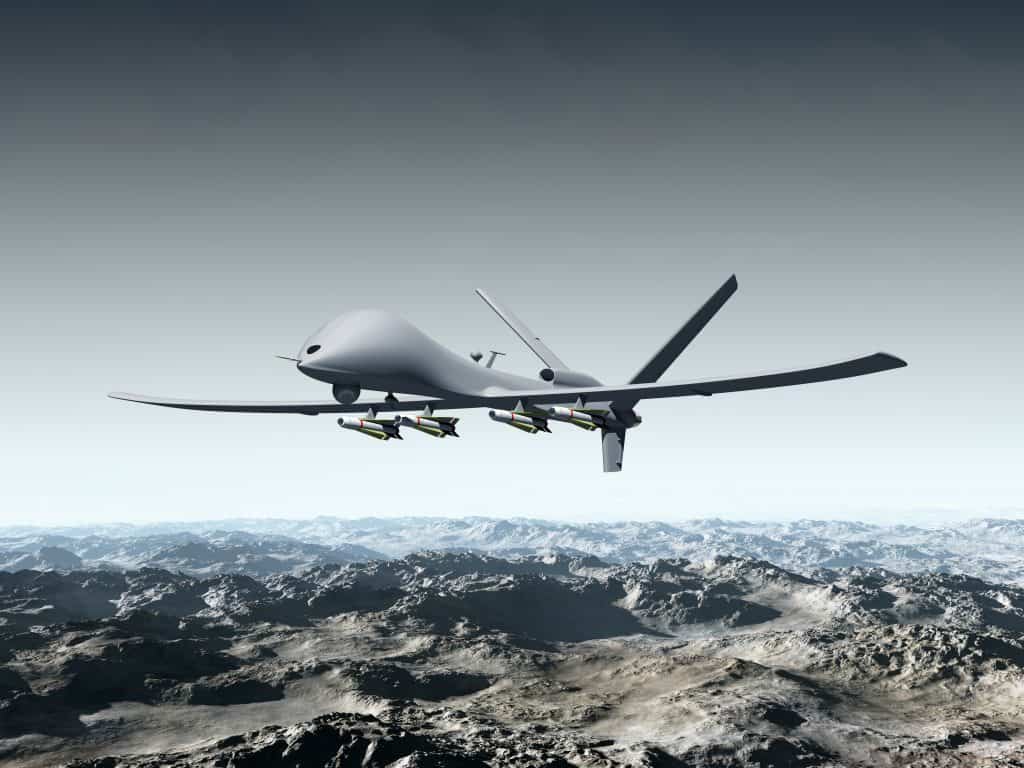
Educating about Lethal Autonomous Weapons
Military AI applications are rapidly expanding. We develop educational materials about how certain narrow classes of AI-powered weapons can harm national security and destabilize civilization, notably weapons where kill decisions are fully delegated to algorithms.
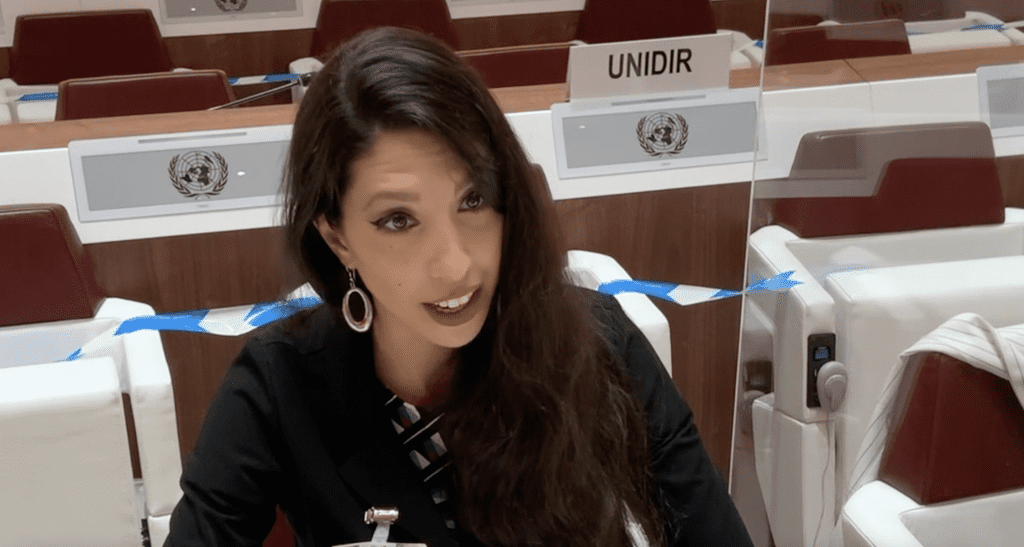
Global AI governance at the UN
Our involvement with the UN's work spans several years and initiatives, including the Roadmap for Digital Cooperation and the Global Digital Compact (GDC).
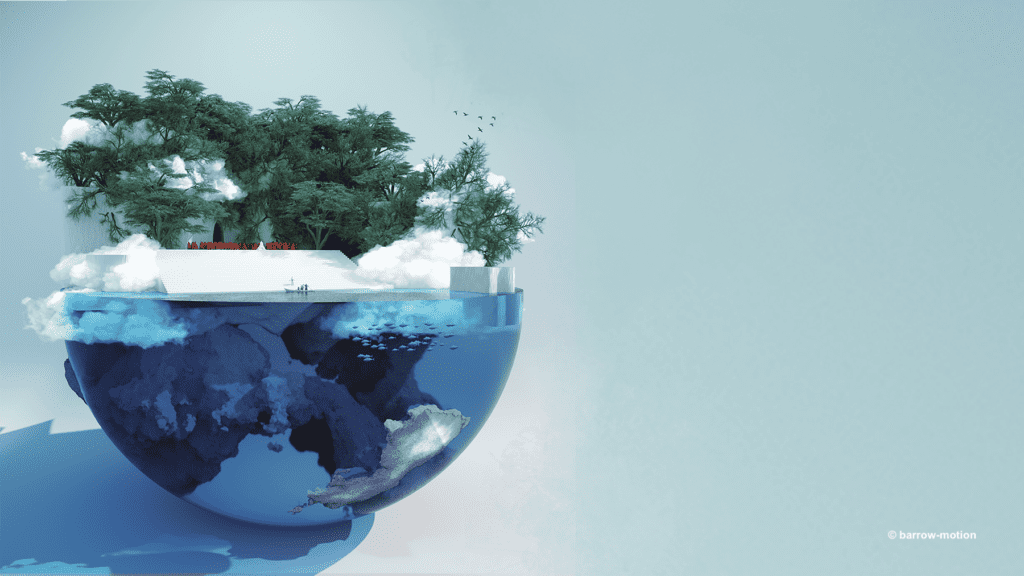
Worldbuilding Competition
The Future of Life Institute accepted entries from teams across the globe, to compete for a prize purse of up to $100,000 by designing visions of a plausible, aspirational future that includes strong artificial intelligence.
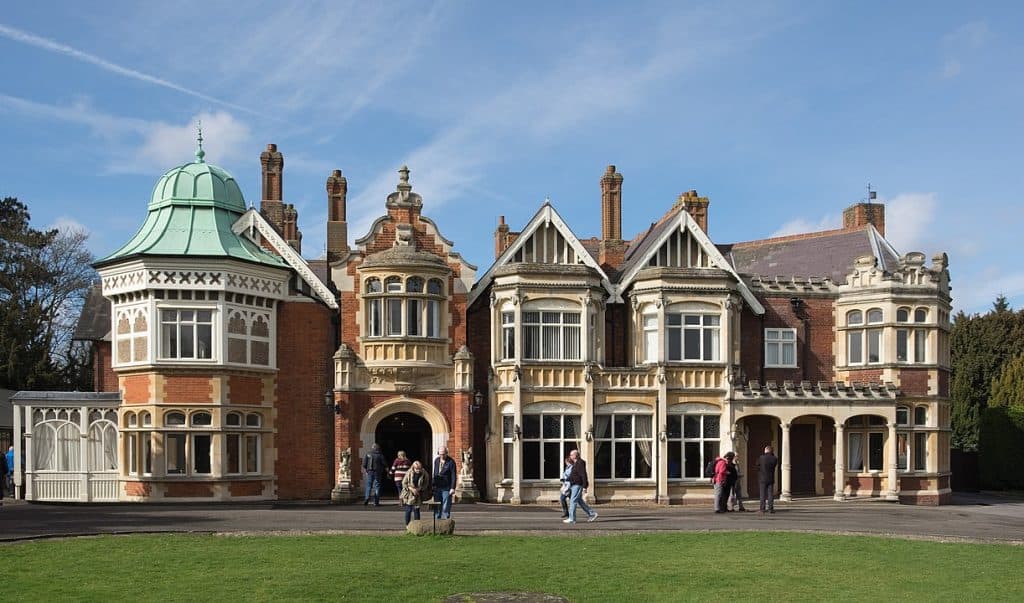
UK AI Safety Summit
On 1-2 November 2023, the United Kingdom convened the first ever global government meeting focussed on AI Safety. In the run-up to the summit, FLI produced and published a document outlining key recommendations.
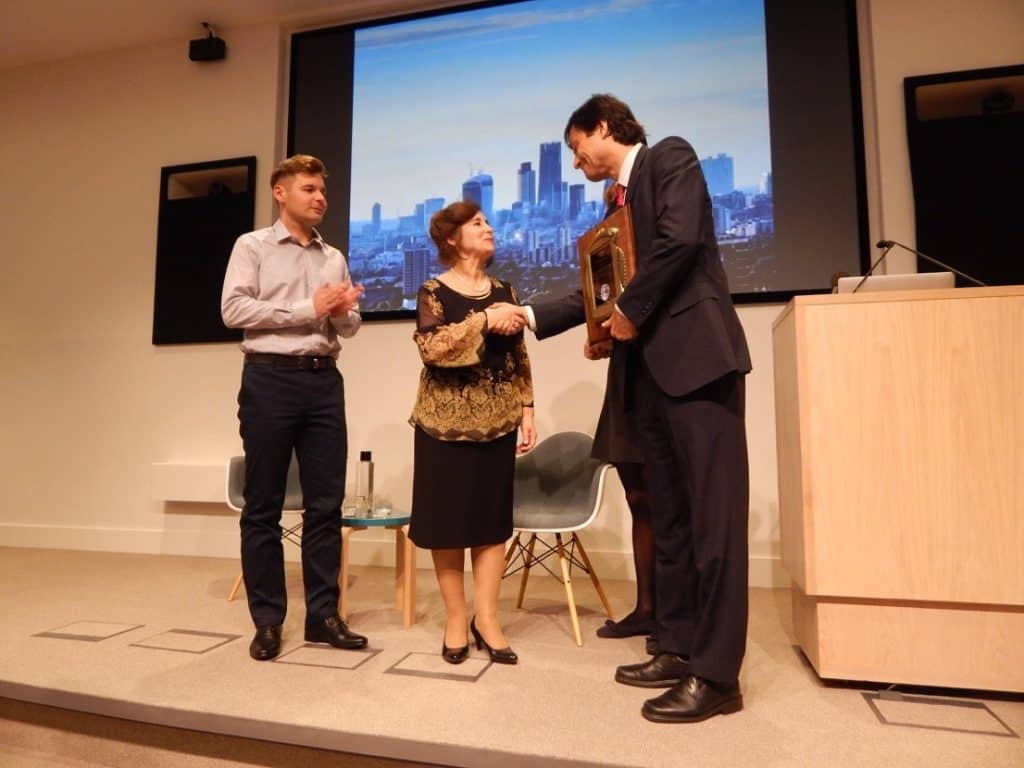
Future of Life Award
Every year, the Future of Life Award is given to one or more unsung heroes who have made a significant contribution to preserving the future of life.
View all projects
newsletter
Regular updates about the technologies shaping our world
Every month, we bring 41,000+ subscribers the latest news on how emerging technologies are transforming our world. It includes a summary of major developments in our cause areas, and key updates on the work we do. Subscribe to our newsletter to receive these highlights at the end of each month.
Future of Life Institute Newsletter: A pause didn’t happen. So what did?
Reflections on the one-year Pause Letter anniversary, the EU AI Act passes in EU Parliament, updates from our policy team, and more.
Maggie Munro
April 2, 2024
Future of Life Institute Newsletter: FLI x The Elders, and #BanDeepfakes
Former world leaders call for action on pressing global threats, launching the campaign to #BanDeepfakes, new funding opportunities from our Futures program, and more.
Maggie Munro
March 4, 2024
Future of Life Institute Newsletter: The Year of Fake
Deepfakes are dominating headlines - with much more disruption expected, the Doomsday Clock has been set for 2024, AI governance updates, and more.
Maggie Munro
February 2, 2024
Read previous editions
Our content
Latest posts
The most recent posts we have published:
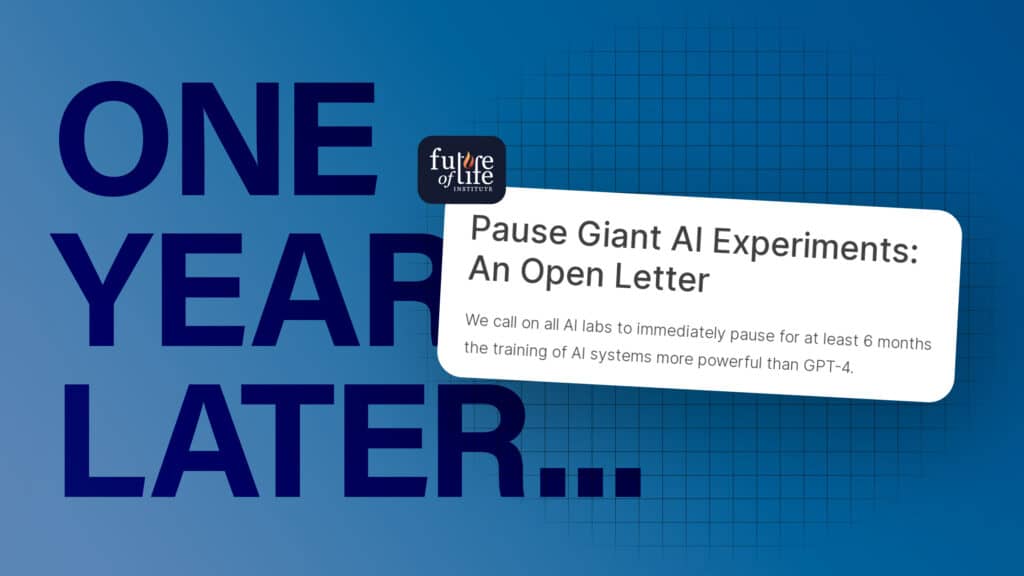
The Pause Letter: One year later
It has been one year since our 'Pause AI' open letter sparked a global debate on whether we should temporarily halt giant AI experiments.
March 22, 2024
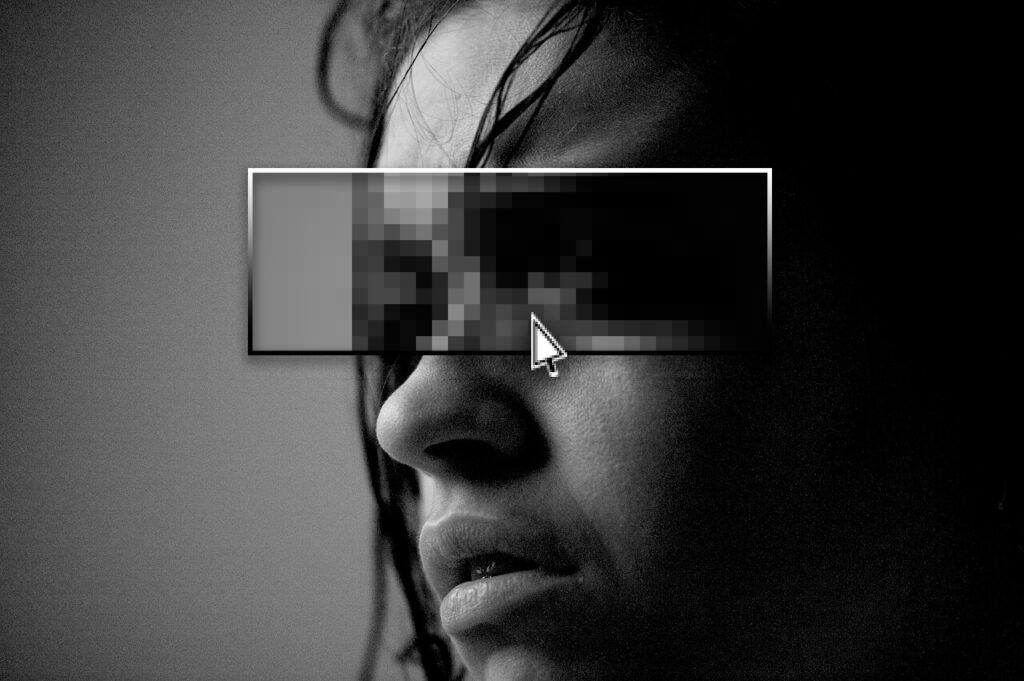
Disrupting the Deepfake Pipeline in Europe
Leveraging corporate criminal liability under the Violence Against Women Directive to safeguard against pornographic deepfake exploitation.
February 22, 2024
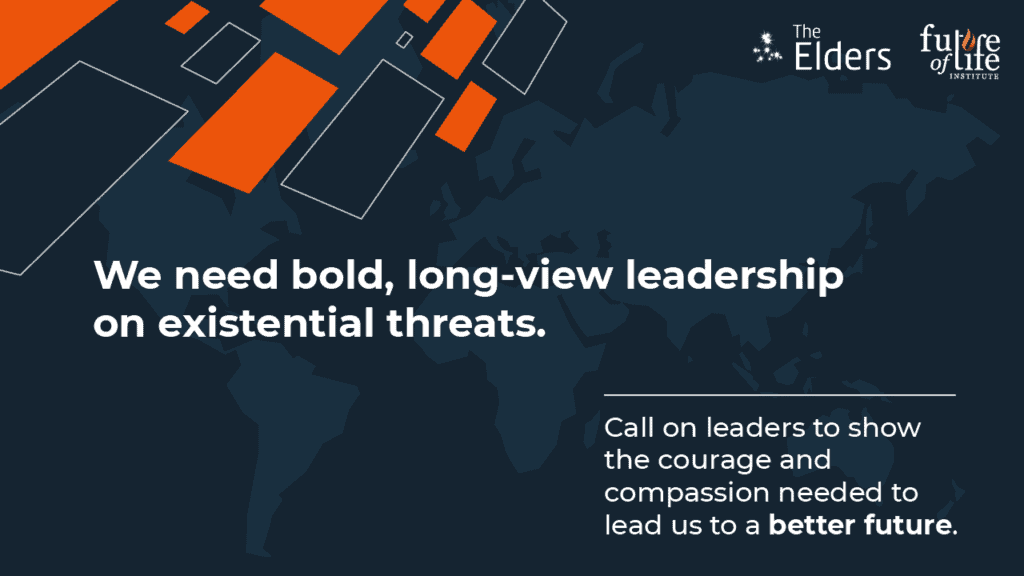
Open letter calling on world leaders to show long-view leadership on existential threats
The Elders, Future of Life Institute and a diverse range of co-signatories call on decision-makers to urgently address the ongoing impact and escalating risks of the climate crisis, pandemics, nuclear weapons, and ungoverned AI.
February 14, 2024
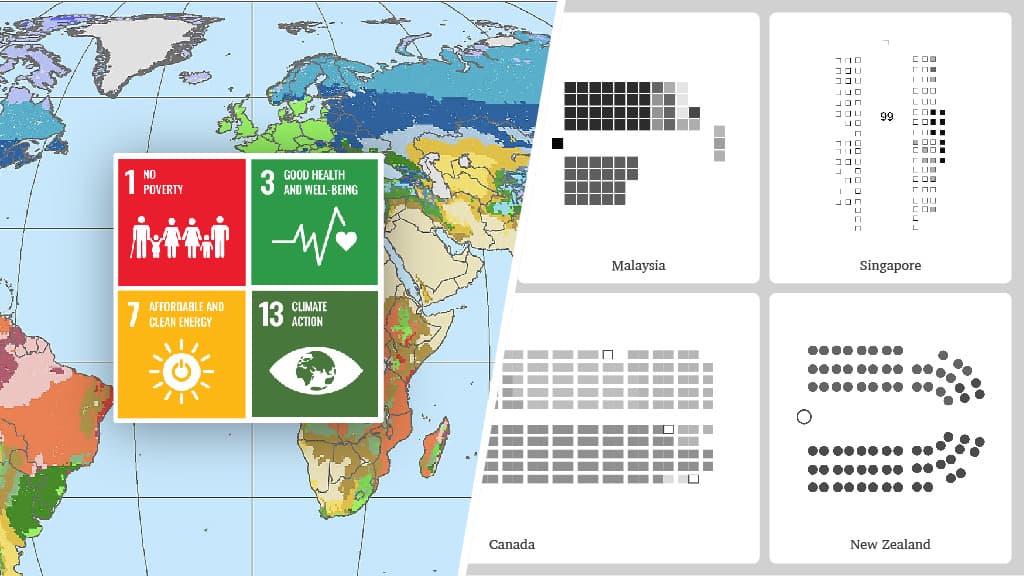
Realising Aspirational Futures – New FLI Grants Opportunities
Our Futures Program, launched in 2023, aims to guide humanity towards the beneficial outcomes made possible by transformative technologies. This year, as […]
February 14, 2024
View all posts
Policy papers
The most recent policy papers we have published:
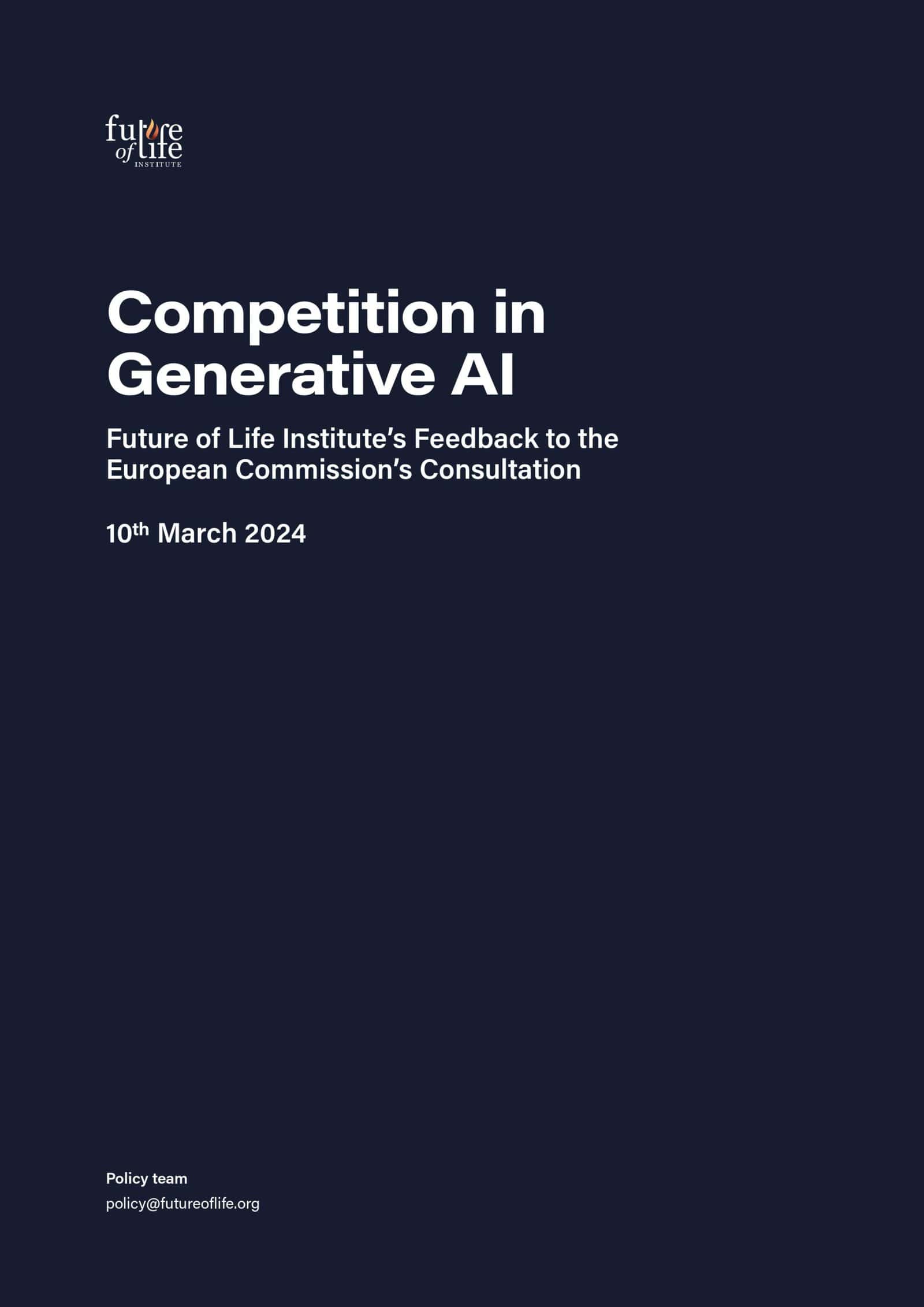
Competition in Generative AI: Future of Life Institute’s Feedback to the European Commission’s Consultation
March 2024
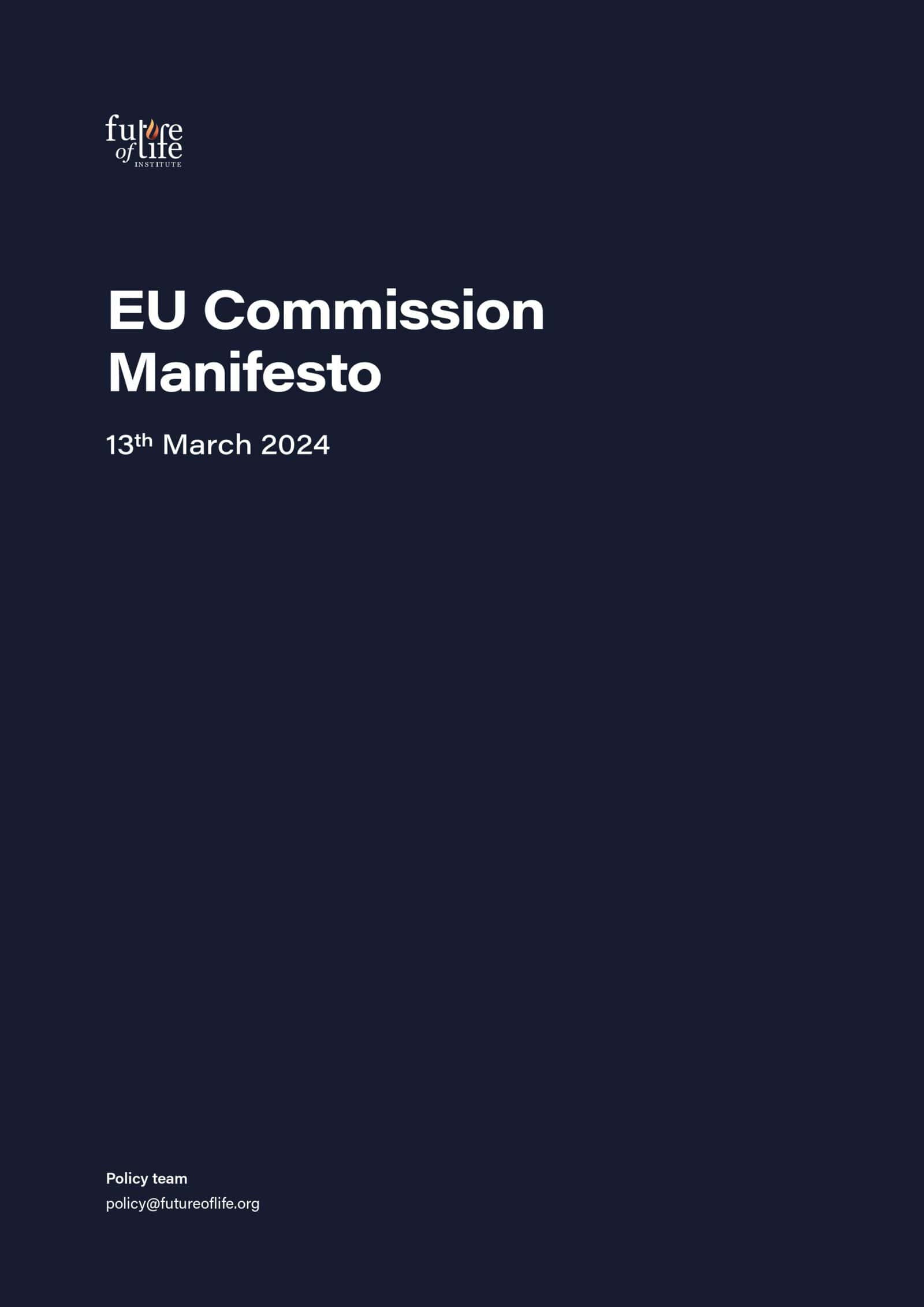
European Commission Manifesto
March 2024
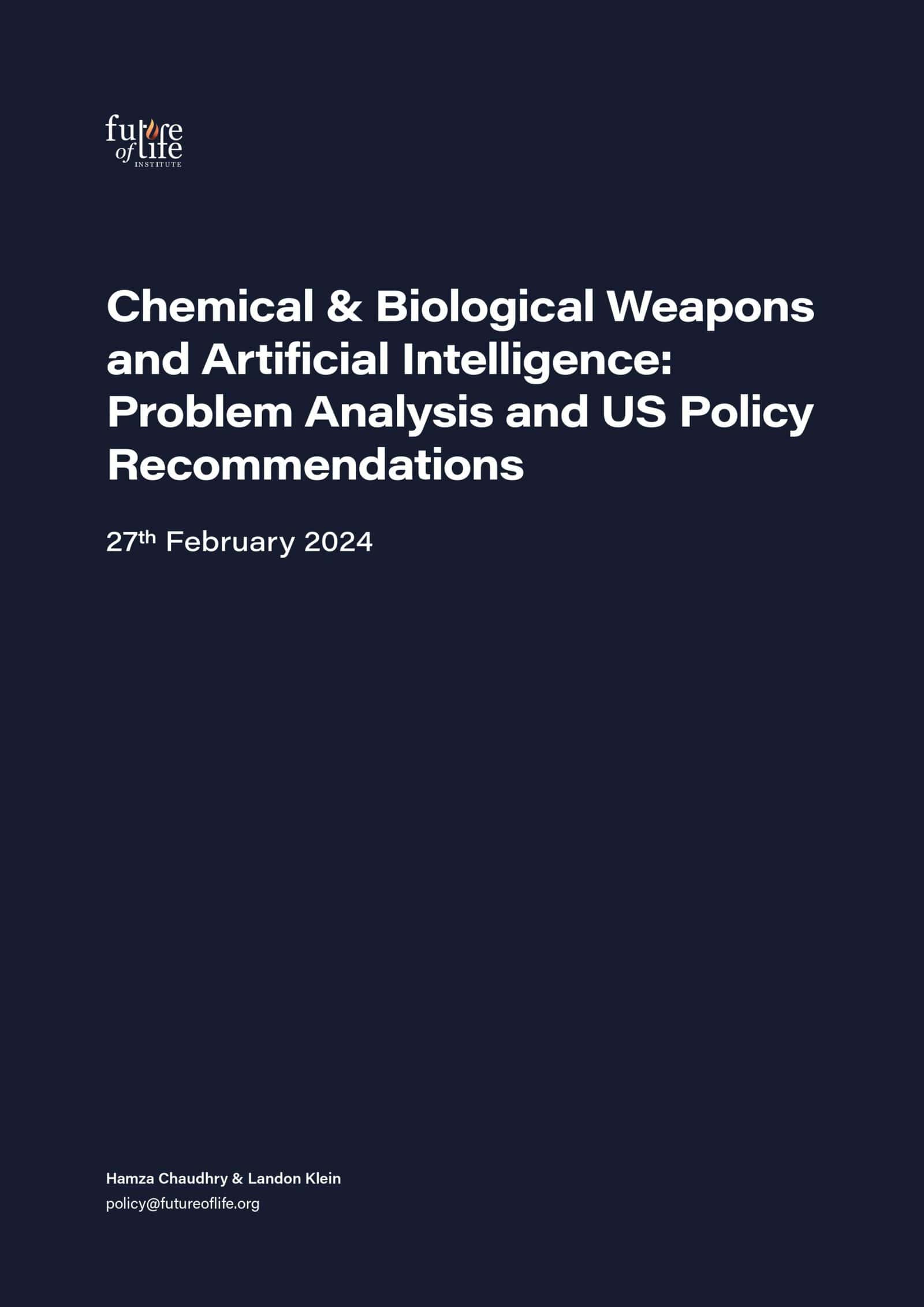
Chemical & Biological Weapons and Artificial Intelligence: Problem Analysis and US Policy Recommendations
February 2024
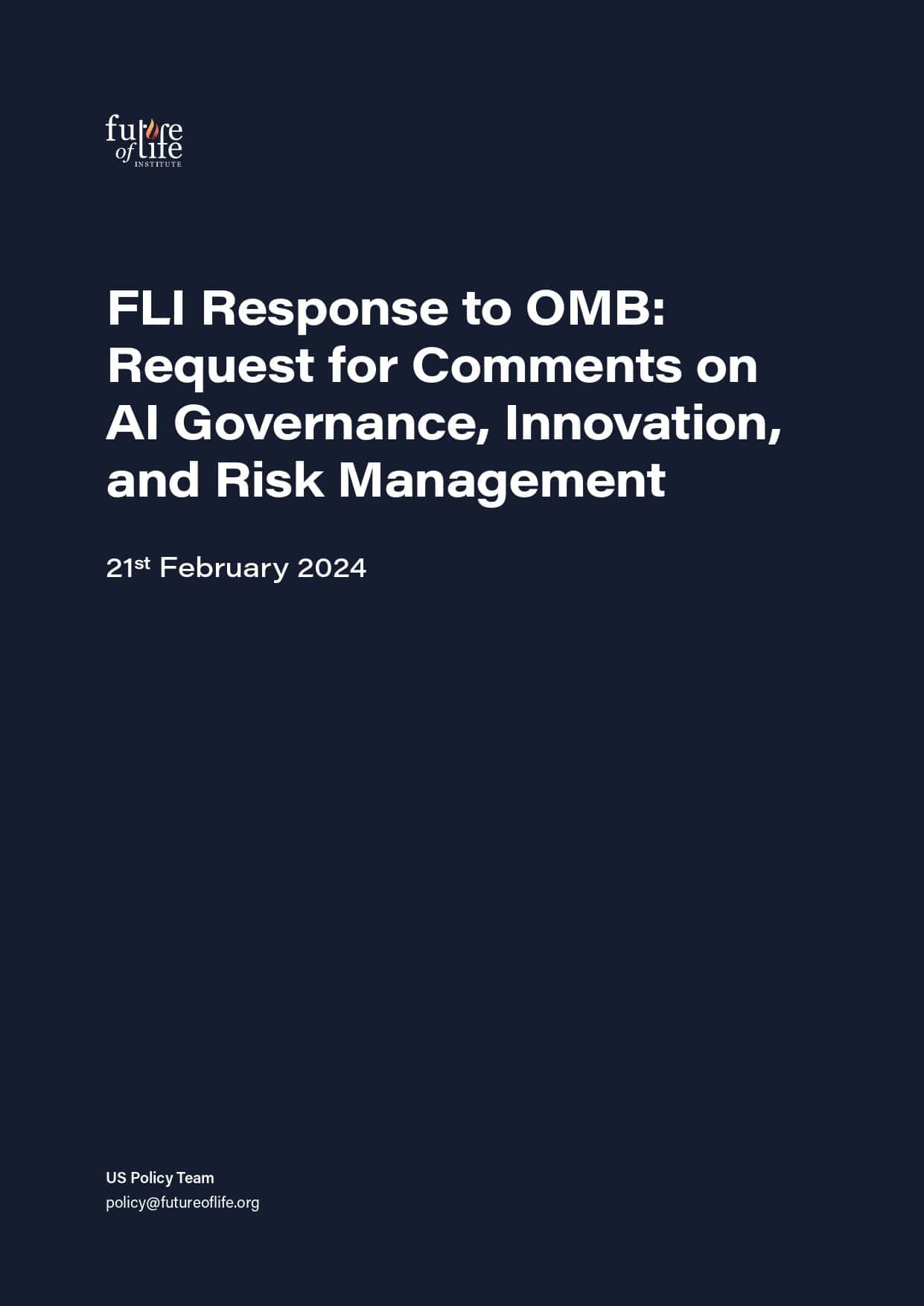
FLI Response to OMB: Request for Comments on AI Governance, Innovation, and Risk Management
February 2024
View all policy papers
Future of Life Institute Podcast
The most recent podcasts we have broadcast:
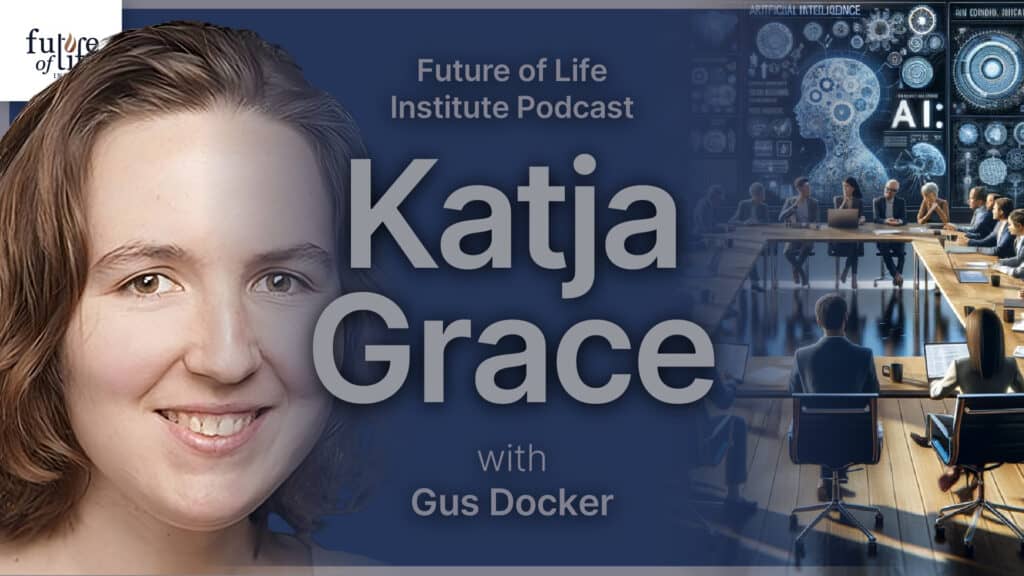
March 14, 2024
Katja Grace on the Largest Survey of AI Researchers
Play
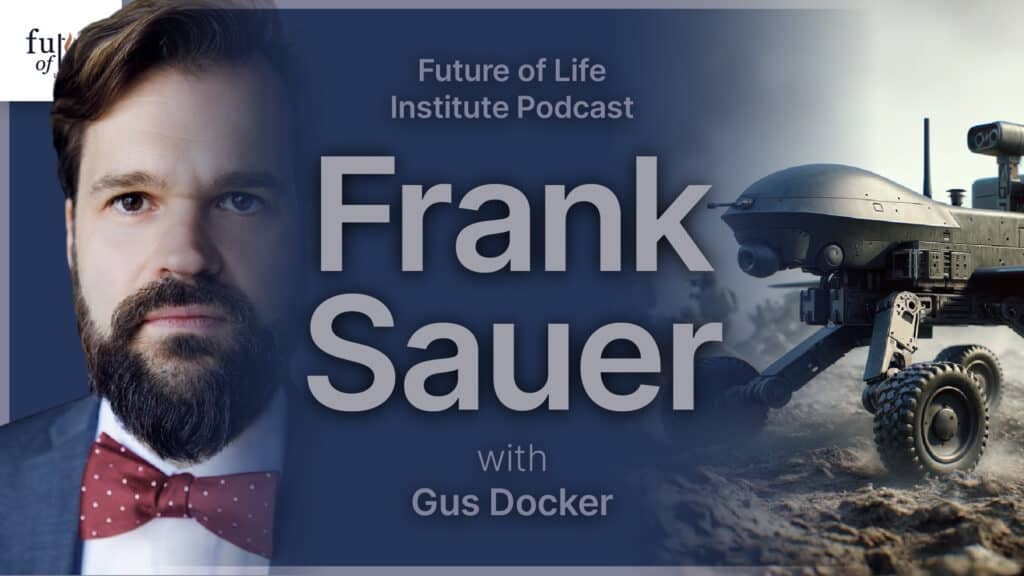
January 6, 2024
Frank Sauer on Autonomous Weapon Systems
Play
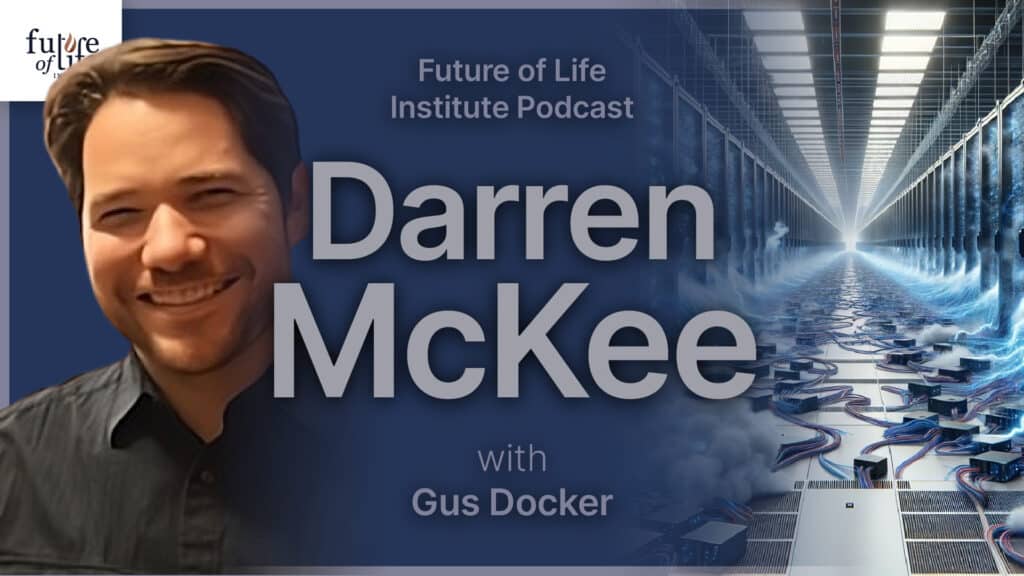
January 6, 2024
Darren McKee on Uncontrollable Superintelligence
Play
View all episodes