AI Researcher Seth Herd
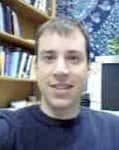
Contents
AI Safety Research
Seth Herd
Senior Research Associate, Psychology and Neuroscience
University of Colorado
Project: Stability of Neuromorphic Motivational Systems
Amount Recommended: $98,400
Project Summary
We are investigating the safety of possible future advanced AI that uses the same basic approach to motivated behavior as that used by the human brain. Neuroscience has given us a rough blueprint of how the brain directs its behavior based on its innate motivations and its learned goals and values. This blueprint may be used to guide advances in artificial intelligence to produce AI that is as intelligent and capable as humans, and soon after, more intelligent. While it is impossible to predict how long this progress might take, it is also impossible to predict how quickly it might happen. Rapidly progress in practical applications is producing rapid increases in funding from commercial and governmental sources. Thus, it seems critical to understand the potential risks of brain-style artificial intelligence before it is actually achieved. We are testing their model of brain-style motivational systems in a highly simplified environment, to investigate how its behavior may change as it learns and becomes more intelligent. While our system is not capable of performing useful tasks, it serves to investigate the stability of such systems when they are integrated with powerful learning systems currently being developed and deployed.
Technical Abstract
We apply a neural network model of human motivated decision-making to an investigation of the risks involved in creating artificial intelligence with a brain-style motivational system. This model uses relatively simple principles to produce complex, goal-directed behavior. Because of the potential utility of such a system, we believe that this approach may see common adoption, and has significant risks. Such a system could provide the motivational core of efforts to create artificial general intelligence (AGI). Such a system has the advantage of leveraging the wealth of knowledge already available and rapidly accumulating on the neuroscience of mammalian motivation and self-directed learning. We employ this model, and non-biological variations on it, to investigate the risks of employing such systems in combination with powerful learning mechanisms that are currently being developed. We investigate the issues of motivational and representational drift. Motivational drift captures how a system will change the motivations it is initially given and trained on. Representational drift refers to the possibility that sensory and conceptual representations will change over the course of training. We investigate whether learning in these systems can be used to produce a system that remains stable and safe for humans as it develops greater intelligence.
Ongoing Projects/Recent Progress
- Risks of Brain-Style AI – Herd and Jilk
- Discussions centered on how the human and mammalian motivational system works, and how a roughly analogous system would work in an AGI system. They settled on the position that the motivational system is relatively well understood, making it quite plausible that those theories will be used as the basis for an AGI system. One important consideration is that the most work on AGI safety assumes a goal-maximizing motivational system, whereas the mammalian system operates very differently. If these researchers are correct that a brain-style system is a likely choice for practical reasons, the safety of such a system deserves a good deal more focused thought.
- These researchers plan to continue theoretical work in the coming project-years, and to publish those theories as well as the results of the empirical work planned for the coming two years. As planned, this year’s activities laid the theoretical basis for upcoming computational work. This year was a necessary maturation period for the base model, which is being developed for other purposes (Understanding the neural bases of human decision-making).
About the Future of Life Institute
The Future of Life Institute (FLI) is a global think tank with a team of 20+ full-time staff operating across the US and Europe. FLI has been working to steer the development of transformative technologies towards benefitting life and away from extreme large-scale risks since its founding in 2014. Find out more about our mission or explore our work.
Related content
Other posts about AI Researcher Profile
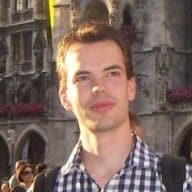
AI Researcher Bas Steunebrink
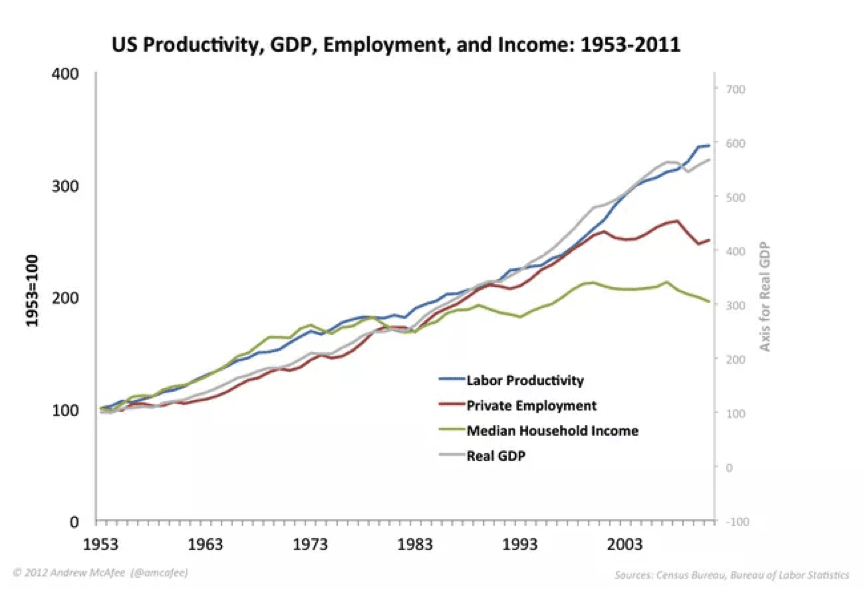
AI Researcher Moshe Vardi
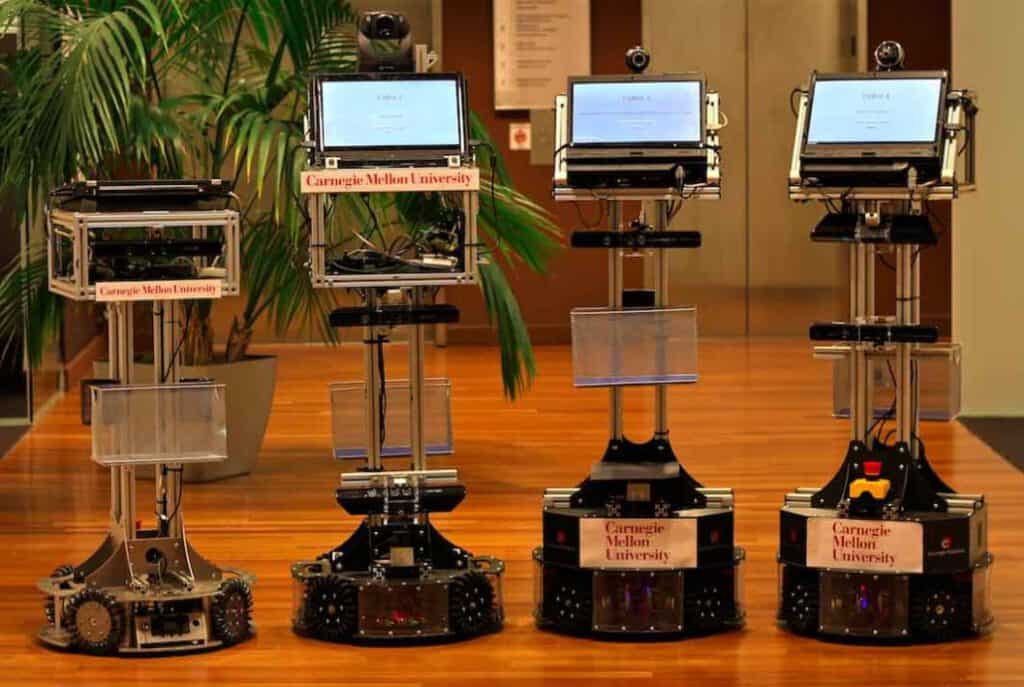
AI Researcher Manuela Veloso
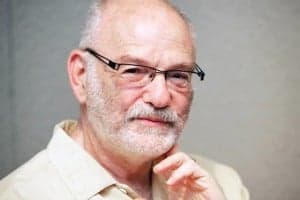