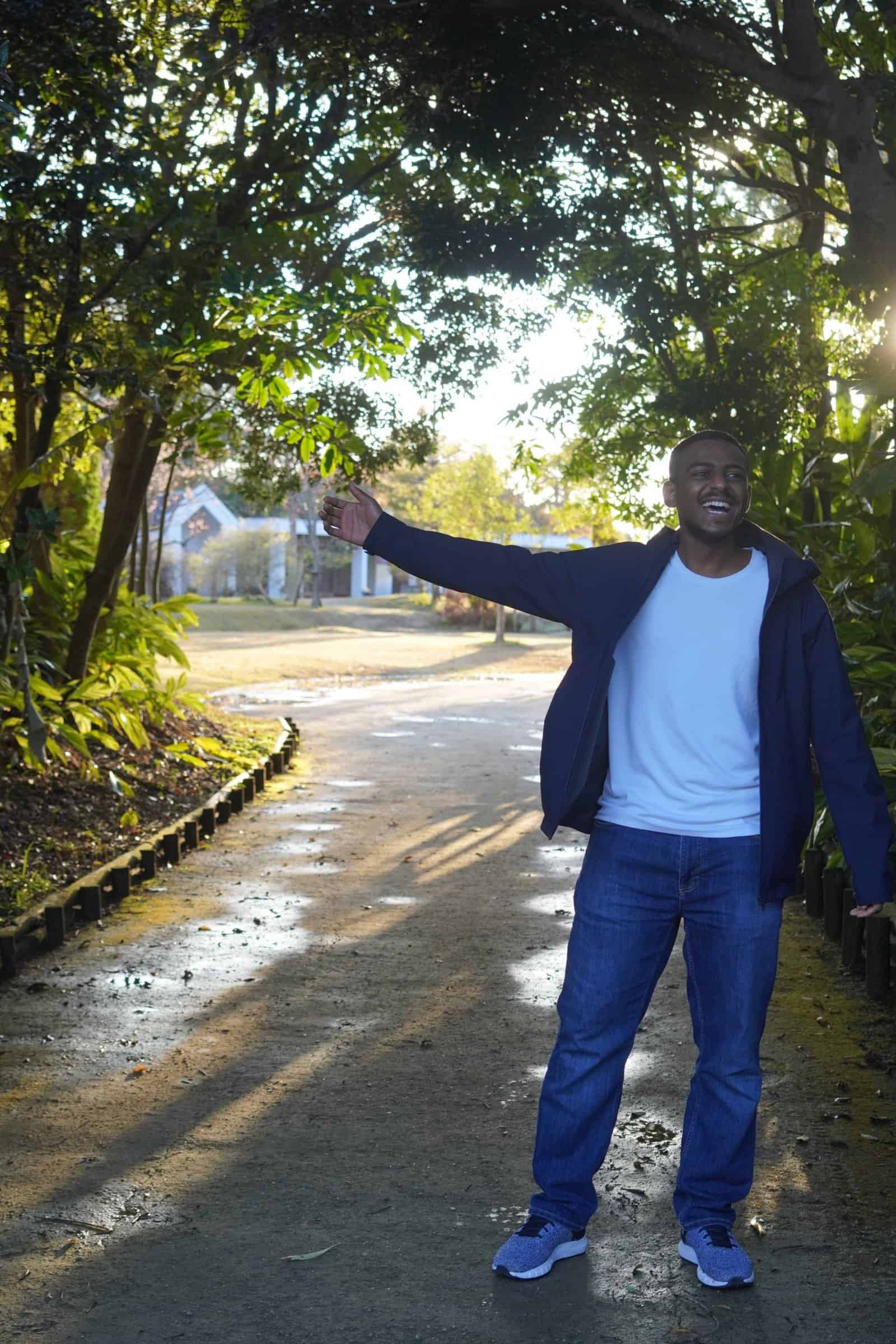
Montaser Mohammedalamen
Why do you care about AI Existential Safety?
One challenge in the field of artificial intelligence is to design agents that avoid doing harm or being destructive. Specifically, in the Reinforcement Learning (RL) field where an agent is trained by trial and error in order to achieve a goal/s represented in terms of a reward function rewarding the agent if it reaches the goal and penalizing it when it fails to do so. Deploying this agent is very challenging because if the deployed environment is not identical to the training environment the agent may not be able to achieve the desired goal, or worse result in catastrophic outcomes.
Please give one or more examples of research interests relevant to AI existential safety:
Existing approaches for safety in RL often specify safe behavior via constraints that an agent must not violate Broadly, this amounts to formulating tasks as a constrained Markov decision process (MDP). A constrained MDP can be solved using RL in a model-based or model-free way, However, this approach requires pre-defining the safe states that the agent is allowed to visit or the safe actions the agent can take. Alternatively, some approaches design “safety functions” that incentivize pre-defined safe behaviors. These approaches require an a priori description of safety information about specific scenarios and present a scaling problem as it is generally infeasible to enumerate all potentially hazardous situations in a realistic application. Our research goal is to develop agents that learn to behave cautiously in novel situations (Learning To Be Cautious). An agent that could learn to be cautious, would overcome this challenge by discovering for itself when and how to behave cautiously. Our approach characterizes reward function uncertainty without task-specific safety information (using neural network ensemble) and using this uncertainty constructs a robust policy (using robust policy optimization). Specifically, we construct robust policies with a k-of-N counterfactual regret minimization (CFR) subroutine. We validate our approach by constructing a set of toy tasks that intuitively illustrate caution in the spirit of AI Safety Gridworlds, in a sequence of increasing challenges for learning cautious behavior. Our approach exhibits caution in each of our tasks without any task-specific safety tuning. This method identifies and adopts cautious behavior in different tasks where cautious behavior is increasingly non-obvious, starting from a one-shot environment (contextual bandit) with an obvious cautious action, leading to one-shot environments with cautious actions that depend on context, and concluding with a gridworld driving environment that requires long-term planning.