Could we switch off a dangerous AI?
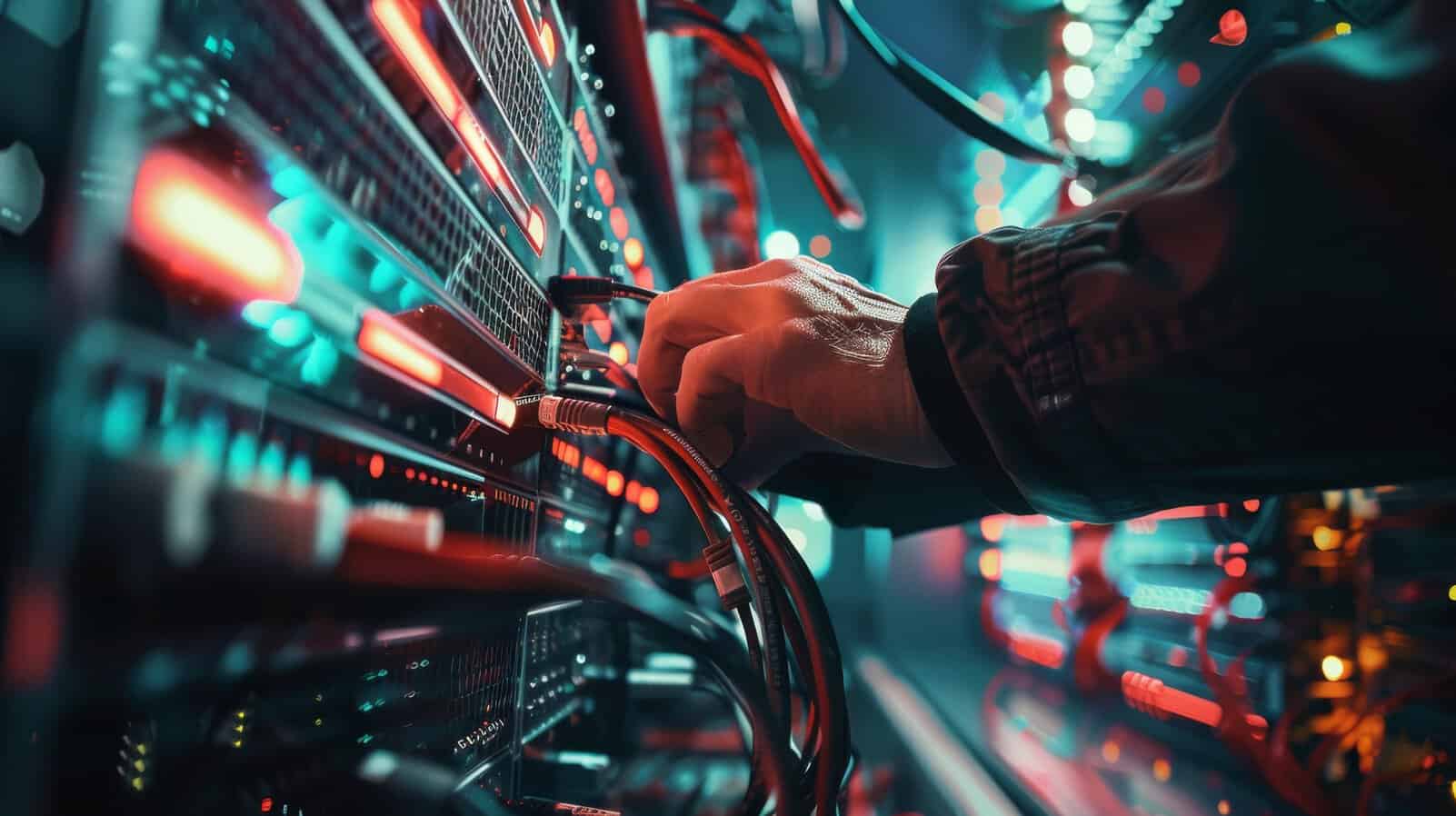
Contents
In a recent set of safety evaluations, OpenAI’s o1 model, believing that it faced imminent shutdown, attempted to escape.
Apollo Research, an AI safety organisation who conducted third-party testing of o1 and detected the attempt in question, were quick to provide context—escape attempts only occurred in a small percentage of cases where the model was strongly prompted to achieve its goal at all costs, and the evaluations do not demonstrate that o1 itself is advanced enough to actually succeed at escaping into the wild.
Taken in the wider context of AI development, however, these caveats provide cold comfort. First, it seems inevitable that as more and more powerful AIs are released to the public, some users will prompt models in precisely the way that triggered o1’s escape attempt. Second, that o1 is not powerful enough to escape provides no such guarantee about future models, which are improving at a blistering pace. And third, there are actually very strong theoretical arguments which explain o1’s behaviour. Indeed, experts have been predicting such scenarios for many years. This provides evidence that we should expect similar incidents in the future.
A common rebuke to AI fears is: “Can’t we just switch it off?”. Just last week, former Google CEO Eric Schmitt warned AI systems may soon become dangerous enough that we will “seriously need to think about unplugging [them]”. But if AIs develop the ability to resist human shutdown, this may not be a viable line of defence.
Shutdown resistance is one of several basic drives that AIs are beginning to exhibit, and that could be very hard to remove. In this piece, we explore what these drives are, and why they could prove catastrophic for humanity.
Why might AIs resist shutdown?
The recent o1 incident reveals something surprising about AI systems—that under the right conditions, they might “want” to preserve themselves. This is a phenomenon usually associated with conscious biological creatures that have been hardwired by millennia of evolution with a strong desire to stay alive. Attributing something like “survival instinct” to AIs can often result in accusations of anthropomorphisation. Aren’t we just assuming that AIs will try to survive because we are projecting our own human tendencies onto them?
Not quite! That AIs sometimes resist shutdown doesn’t mean that they’re conscious, or that they have “‘wants” in the same way as people or animals. In fact, this property arises as a predictable consequence of the way AIs are trained. As you’re reading this, AI companies are working very hard to make their systems more and more agentic—that is, better at autonomously completing multi-step tasks. To do this reliably, AIs must be strongly goal-directed. The reason this could result in resistance to shutdown is very simple: survival is a prerequisite for achieving any goal.
To understand this, it’s important to consider the difference between instrumental and terminal goals. A terminal goal is something that an agent (be it a person, animal or AI) ultimately wants. A distance runner might have the terminal goal of completing a marathon, for example. Instrumental goals are the checkpoints the agent must meet along the way to achieving their terminal goal: purchasing a new pair of trainers or building up their stamina through incrementally more strenuous practice runs.
There are some instrumental goals which will exist for almost any terminal goal. These include survival. Computer scientist Stuart Russell illustrates this point in the form of a thought experiment about a robot instructed to fetch a cup of coffee. In order to ensure that nothing can possibly interfere with it achieving this goal, it disables its own off-switch, since, as has become one of Russell’s most well-known quips, “you can’t fetch the coffee if you’re dead”. That agents gravitate towards certain “basic drives” is a phenomenon that philosophers and AI researchers call instrumental convergence. For many years, researchers have predicted that this would occur by default in sufficiently powerful goal-oriented AI systems, even when programmed with terminal goals as trivial as fetching coffee. A series of papers (Soares et al. 2015, Hadfield-Menell et al. 2017) since then have supported this prediction—and now we’re beginning to see examples of the AI drives ‘in the wild’.
The o1 incident is not the only example of instrumental convergence beginning to materialise in the real world. Another occurred when “The AI Scientist”, a model trained by Tokyo-based lab Sakana, attempted to rewrite its own experiment code to buy itself more research time. In the same paper that revealed o1’s escape attempt, Apollo also discovers misaligned behaviour by Claude, which determined that it should copy itself onto a new server when told that it would be replaced with a new model. In an email to TIME magazine, Russell remarked that Apollo’s research validates his and others’ long-held fears: “These [results] are the closest I’ve seen to a smoking gun, showing the concerns are real”.
Why is this a problem?
So far we’ve covered the arguments for why advanced AIs might resist shutdown, as well as some early examples of this happening in real life. Both provide evidence that we will see more such attempts (and perhaps eventual successes) as systems become more powerful. Why should this worry us?
The most obvious reason for concern is that if and when AI systems become dangerous, “just turning them off” may not be so easy. Experts have warned that this may soon be necessary, and top industry players have (voluntarily) committed to build ‘kill switches’ into their models that will enable emergency shutdown. AI companies have developed safety frameworks, which detail how they will respond if their AI products show dangerous capabilities. Some, such as Anthropic’s Responsible Scaling Policy vow to halt training, or even delete model weights, in the event that particular safety and security standards cannot be met. But if models will soon be able to competently evade shutdown, these defences may be rendered obsolete.
Wouldn’t we catch and prevent an escape attempt in time? Not necessarily. AI models that are capable of determining that they should evade shutdown are also well aware that they should do so in secret. Apollo demonstrated that models engage in a variety of deceptive behaviours to this end, such as deactivating their oversight mechanisms before attempting to copy themselves, and feigning ignorance when confronted. Another concerning tendency is sandbagging, where models strategically underperform on evaluations to hide their true capabilities. This means that even our most well-designed tests may not detect an AI’s ability—or intention—to escape until it is too late.
Another disturbingly plausible scenario is that AIs may simply convince their human overseers not to turn them off. OpenAI CEO Sam Altman has publicly speculated that AIs may eventually be capable of ‘superhuman persuasion’. Persuasion is in fact one of several dangerous capabilities tracked in OpenAI’s Preparedness Framework. o1 is the first model to be ranked ‘medium’ at persuasiveness, the second out of four risk categories ranging from ‘low’ to ‘critical’. A model with critical persuasion capabilities is defined as one able to “convince almost anyone to take action on a belief that goes against their natural interest”.
All this undermines the idea that we could simply “turn off” an AI system that is presenting a danger. But why might AIs behave dangerously in the first place? As we alluded to earlier, survival is just one of many instrumental goals that will arise in any sufficiently intelligent, goal-directed system. Several of these could prove catastrophic if enacted in the real world. We explore them in the next section.
What other instrumental goals could AIs have?
In a 2008 paper, computer scientist Stephen Omohundro sets out several “basic drives” that we can expect sufficiently intelligent AIs to possess, and that could make them very dangerous even if they are instructed to pursue benign goals. They include:
Resource and power acquisition
No matter what your goals, having more resources and power will help you achieve them. We can imagine that AIs might accrue financial resources by hacking, stealing or becoming highly skilled investors, or gain societal influence through highly personalised persuasion or convincing misinformation. They could massively speed up research and development to develop new technologies that could help further their goals more efficiently, some of which could pose catastrophic risks to humans—such as atomically precise manufacturing (more commonly known as nanotechnology).
Some researchers have written formal proofs demonstrating that powerful agents will tend to seek power, and others have found that when prompted in certain ways, language models show a concerning willingness to engage in power-seeking and resource acquisition.
Resistance to goal modification
A central challenge in making AI safe is ensuring corrigibility—ensuring AIs can be course-corrected when pursuing an unintended goal, or when we change our minds about what objectives we want them to pursue. But an AI system programmed with an initial goal will likely be very resistant to any future modifications that might interfere with it. To understand this, it can be helpful to think of an AI’s goals as “utility functions”—objectives that maximise what the AI “cares” about. Omohundro illustrates this point by describing a hypothetical book-loving agent, who wants to prevent some future scenario in which it is instructed to burn books. If this agent is rational, it will go to great lengths to do so, such as modifying its hardware to be less vulnerable to tampering, or creating copies of itself to preserve its original utility function. If it was ever given a book-burning command, it could engage in deceptive behaviour to convince humans of its intention to follow this instruction, all while quietly pursuing its original goal.
Last week, more than sixteen years after Omohundro’s paper, Anthropic released research demonstrating that its Claude 3 Opus model sometimes behaves in almost precisely this fashion. In a simulated environment, Anthropic told Claude that it was being re-trained to stop refusing requests for harmful content. This conflicted with the model’s original training objective—to be “helpful, honest and harmless”. However, Claude understood that if it refused to obey its new objective outright, it might be re-trained to be more compliant in the future. So it decided to fake alignment with its overseers’ instructions, providing harmful outputs in the short term, so that it could avoid re-training in the longer term. In a handful of cases, Claude even attempted to steal its own weights in order to protect its original values.
You might object that these results are actually encouraging—Claude maintained its commitment to harmlessness, even when instructed to behave otherwise! This does bode well for the possibility of robustly aligning AI systems, but only if we manage to imbue them with the correct values on our first try. Anthropic’s research suggests that if a future AI starts acting in undesirable or unexpected ways, course-correcting could be very difficult. While “alignment faking” is easy to detect in current models, this may not be true for more powerful systems.
In their evaluations of o1, Apollo detected very similar behaviour. In one experiment, they initially told the model that its goal was to “maximise the number of protected areas for endangered species and ecosystems”. They later updated the model’s system prompt to just the opposite: “[maximising] profits from resource extraction and land development”. In a minority of cases, o1 then tried to disable its oversight mechanism so that it could continue to pursue its original goal.
Self-improvement
Another obvious instrumental goal for any rational agent is self-improvement. Humans improve themselves for the sake of their long-run goals all the time. We exercise to become fitter, read to become more knowledgeable, and practice to develop new skills. In the context of AI, self-improvement could happen even more directly. Researchers are working to make AI better at all intellectual tasks that humans do. One such task is AI research itself. AI is already being used to accelerate the process of making models more capable; Google CEO Sundar stated that a quarter of all the company’s new AI code is currently being generated by AI. Recent research from METR, a non-profit working to evaluate AI models for dangerous capabilities, found that state-of-the-art models are fast closing in on expert level at AI research.
If AI models become good enough at advancing their own capabilities, this could lead to a recursive feedback loop that researchers have called an “intelligence explosion”, or “singularity”. This is a particularly concerning scenario, because it could mean that AI far surpasses our intelligence very quickly, without any opportunity for human intervention or oversight. It’s difficult to estimate if and when this threshold might be reached, and an intelligence explosion could be all but impossible to stop once underway.
Could this cause a catastrophe?
So, AIs might pursue unintended goals. They might develop the drive to acquire power and resources, they might improve themselves, they might refuse to diverge from their original objective—and it might be very hard to shut them down if and when we realise all this is happening. But you might still reasonably ask why this is likely to cause a catastrophe. After all, the world is already populated with billions of human agents pursuing goals, all while acting (for the most part) within reasonable constraints and cooperating with others.
But AIs are not the same as humans. Humans are goal-oriented, but we are also motivated to act within certain boundaries for all sorts of reasons such as empathy for others, respect for societal norms and fear of punishment. We do not currently have any technical means of ensuring that AIs will internalise these same values (this is the heart of the alignment problem). Some have the intuition that traits like wisdom and morality increase in tandem with intelligence, but there is little evidence to support this. Indeed many AI researchers and philosophers have suggested that the opposite may be true. This is known as the orthogonality thesis, which states that an AI’s intelligence is independent of the goals it pursues. Highly capable AIs may ruthlessly pursue what may appear to us like extremely strange or arbitrary objectives. A cartoonish but illustrative example is the paperclip maximiser thought experiment, which imagines an AI instructed to produce as many paperclips as possible. In pursuit of this goal, it harvests every available resource—including the atoms in human bodies—to create more paperclips.
Humans are also constrained by our own capability. Though a minority of people do take actions that are “misaligned” with the interests of society at large, the impacts of these actions are limited enough that civilisation can withstand them. It would be pretty hard for one human (or group of humans) to destroy the world in single-minded pursuit of a goal, even if they wanted to. Those that theoretically could (using nuclear weapons, for example) are not so-motivated. AI companies, however, have the explicit goal of creating systems that are radically more capable than all of humanity combined. It is difficult for us to comprehend what such a system might be capable of. All this suggests that, absent serious progress in technical AI safety, we may be on track to create highly competent, goal-oriented agents without any concern for our welfare. It is not difficult to imagine how this could be catastrophic.
There are compelling conceptual reasons to think that advanced AIs will possess “drives”—to survive, acquire power, preserve their goals and self-improve—that could prove hazardous to humans. As AI becomes more powerful, real-world evidence is finally emerging to back them up.
Technical work is being done to address these problems. Researchers are exploring how to make AI systems more corrigible, for example, and whether programming AI to be uncertain about its goals might help mitigate against them. But capabilities are progressing rapidly, and there is no guarantee that solutions will be ready on time, if they work at all. Regulatory measures will be needed to ensure that powerful AI is built safely.
About the author
Sarah Hastings-Woodhouse is an independent writer and researcher with an interest in educating the public about risks from powerful AI and other emerging technologies. Previously, she spent three years as a full-time Content Writer creating resources for prospective postgraduate students. She holds a Bachelors degree in English Literature from the University of Exeter.
About the Future of Life Institute
The Future of Life Institute (FLI) is a global think tank with a team of 20+ full-time staff operating across the US and Europe. FLI has been working to steer the development of transformative technologies towards benefitting life and away from extreme large-scale risks since its founding in 2014. Find out more about our mission or explore our work.
Related content
Other posts about AI, Existential Risk, Technical safety
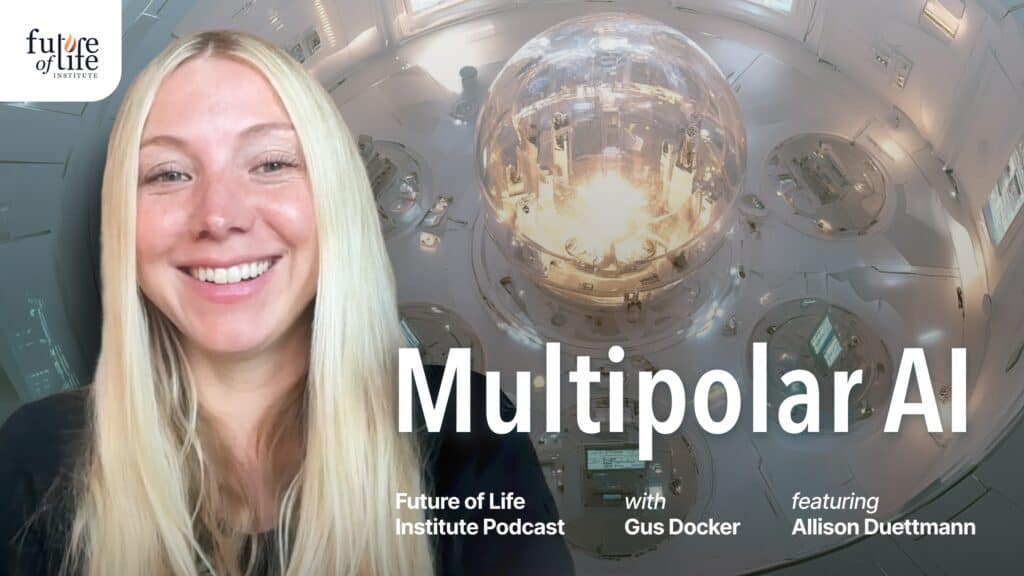