Paris AI Safety Breakfast #1: Stuart Russell
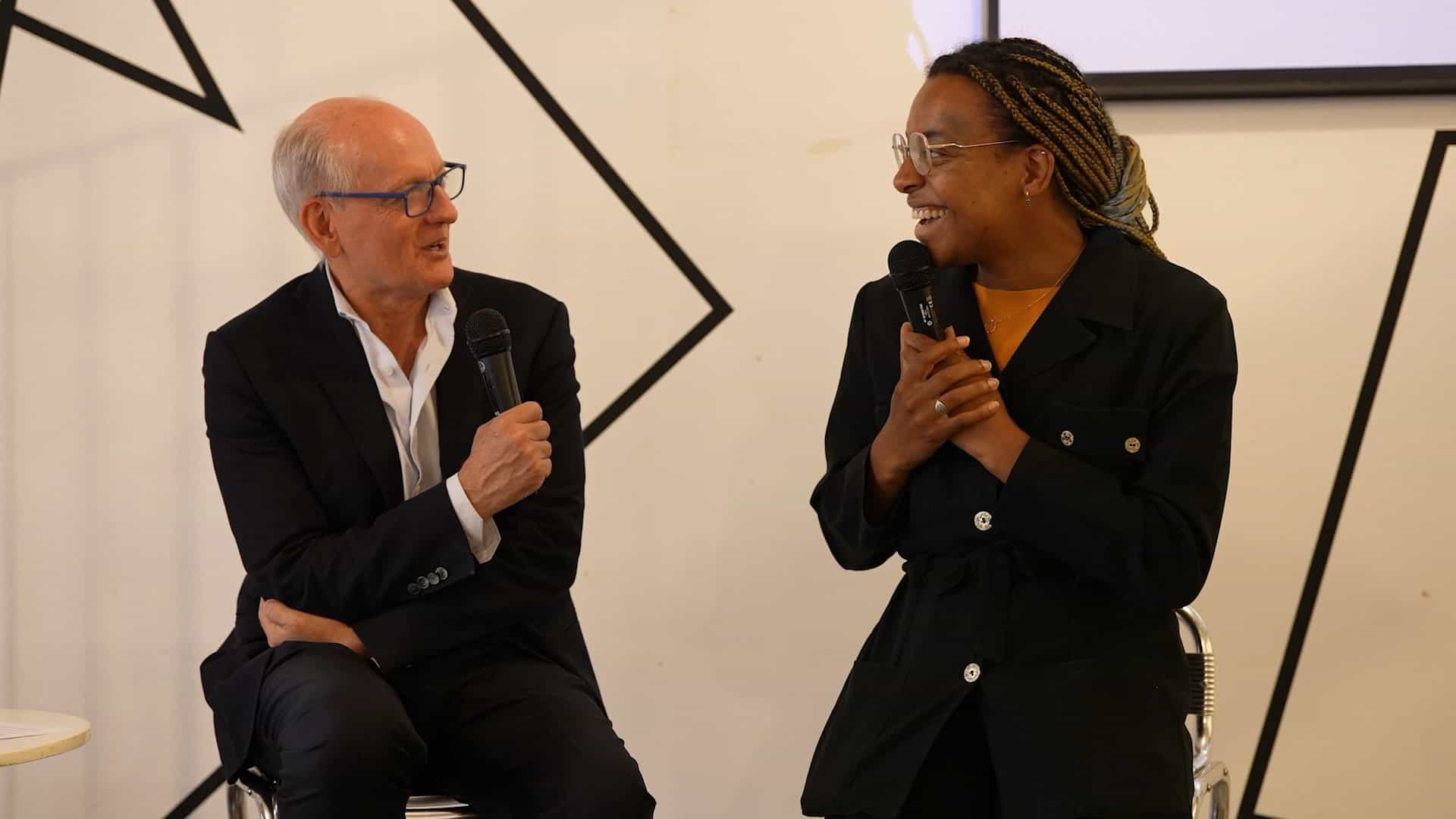
This is the first of our ‘of ‘AI Safety Breakfasts’ event series, featuring Stuart Russell.
About AI Safety Breakfasts
The AI Action Summit will be held in February 2025. This event series aims to stimulate discussion relevant to the Safety Summit for English and French audiences, and to bring together experts and enthusiasts in the field to exchange ideas and perspectives.
Learn more or sign up to be notified about upcoming AI Safety Breakfasts.
Ima (Imane Bello) is in charge of the AI Safety Summits for the Future of Life Institute (FLI).
The event recording is below. Note: The ‘audience questions’ segment of the event is not included in the video, but is included in the transcripts below.
Video chapters
- 00:00 – Introduction from Ima
- 04:00 – Q: Which new trends in AI capabilities do you consider most significant, and what potential challenges or implications do you foresee these advancements posing for society?
- 20:20 – Q: What do you think about the development of AI agents which are capable of autonomous, long-term planning? What potential benefits and risks do you foresee for society?
- 28:18 – Q: A) What new offensive cyber capabilities do you think AI might enable in the near future, and B) considering our reliance on digital infrastructure, what kind of impacts should we be prepared for if AI-enhanced cyber attacks become more prevalent?
- 39:28 – Q: Can you paint us a picture of what the world would look like with strong AI-enabled cyber attacks?
- 42:44 – Q: What would you consider to be the ideal outcome for the upcoming French summit, and how could it further advance international collaboration on AI safety?
- After end of video (see transcript below) – Audience questions:
- Q: Do you think red teaming is a sufficiently productive method for ensuring safety? And do you think it might be a dangerous practice?
- Q: In which direction do you think AI research should go?
- Q: Might deliberative democratic processes be a useful tool for advancing AI safety?
Transcript
Alternatively, you can view the full transcript below.
Transcript: English (Anglaise)
Ima: [French] Okay, great. Thank you all very much for coming. It’s always nice to start with a microphone in your hands Thursday mornings at 9.00 am. I’ll start with an introduction in French.
[English] I’m going to start with French for, like, five minutes. I promise it’s only going to be five minutes. Despite the myth that we cannot speak English or stop talking in French. And then we’ll just, I have some questions for Stuart, I think that’s going to last between 25, 30 minutes and then whatever questions you have, we’ll be happy to take them.
[French] Voilà, welcome. As you know, my name is Ima. I organise a series of breakfasts that I call Safety Breakfast, so a breakfast on safety and security issues in the context of the summit to be held in Paris on 10 and 11 February. The aim of this series is to open up a space for discussion between experts and enthusiasts in the field of AI safety and security, AI governance, so that as the summit progresses, we can ask each other questions as openly as possible. I know you can see a camera, don’t worry. The questions will not be published. These are just the questions I asked Stuart, and therefore Stuart’s comments and responses, which will be published. I’ll obviously send you the blog post and the transcript. And that’s it. So, with regard to the Security Summit, as you know, takes place on 10 and 11 February. This is the third Security Summit. It’s called the Action Summit. Because we have an extremely ambitious French vision, which aims to promote solutions, including, for example, standards on the issues related to AI, and not just the issues related to on safety and security, but really issues that are related to the impact of AI systems on the workplace, issues relating to international governance, issues relating to the way in which we live in society in a general manner everything that we call AI for good, AI systems for the well-being of mankind and, of course, in part, the safety issues on which we at FLI are focusing, together of course with the issues of governance that we focus on. And that’s it. I think that’s all. With regard to the context of this breakfast and the summit in general. The next breakfast will be held at the beginning of September. Then we’ll have another edition in mid-October. And if you’re interested, there’s a QR code on the slide. Don’t hesitate to indicate your interest on the list, I won’t spam you. I used to be a lawyer specialising in human rights and new technologies, so I’m familiar with the GDPR. Don’t worry, you won’t receive any spam. We respect people’s consent, but if you want to be invited, we need to know. Voilà.
[English] That’s it for the French. Thank you for everything, again, thank you so much for coming. I know it’s 9 AM. I’m tired. You all are. but I really appreciate you coming despite the Olympics. And I really, really, really look forward to an insightful discussion with Stuart that I cannot thank you enough, because he’s here today. So Stuart, thank you so much. I have… Do you want to say a few things before we start, or can I just dive into it with the questions?
Stuart: Please.
Ima: Yeah? Can I? Okay.
Stuart: And congratulations to France on winning their first, winning their first football match last night.
Ima: All right. So Stuart, you know, I know we all know here, we’ve seen a remarkable advancement in AI across various domains, right. This includes improved models like GPT-3 – GPT-4, sorry – and Claude 3.0. More sophisticated generators like DALL-E 3. Video generation capabilities with Sora, and even progress in robotics and molecular biology prediction. Two new trends that we’ve seen are the integration of multiple capabilities into a single model, so what we call native multimodality, and the emergence of high quality video generation. From your perspective, which of these recent developments do you consider most significant, and what potential challenges or implications do you foresee these advancements posing for society?
Stuart: So these are quite difficult questions to answer, actually. And it might be helpful, I know, like Raja, for example, has perhaps even longer history in AI than I do. But for, for some people, you know, who have come to AI more recently, the history actually is important to understand. So for most of the history of AI, we proceeded like other engineers, like mechanical engineers and aeronautical engineers. We, tried to understand the basic principles of reasoning, decision making. we then, built algorithms that did logical reasoning or probabilistic reasoning or default reasoning. We studied their mathematical properties, decision making, under uncertainty, various types of learning. and, you know, we were making slow, steady progress with some, I think, really dramatic contributions to you know, the human race’s understanding of how intelligence works. And it’s kind of interesting. So I think in 2005 at, NeurIPS, which is now the main AI conference, Terry Sejnowski, who was a co-founder of the NeurIPS conference, proudly announced that there was not a single paper that used the word backpropagation that had been accepted that year. And that view was quite common, that neural networks were, not reliable, not efficient in terms of data, not efficient in terms of computation time. and didn’t support any kinds of mathematical guarantees and other methods like support vector machines, which were developed, I guess originally by statisticians like Vapnik, were superior in every way, and that this was just a sign of the field growing up. And then around 2012, as many of you know, deep learning came along and demonstrated significant improvements in object recognition. And that was that was when the dam broke. And I think it wasn’t that we couldn’t have done that 25 years earlier. The way I described it at the time was we had a Ferrari and we were driving around in first gear, and then someone said, well, look, if you put this knob over there and go into fifth gear, you can go at 250 miles an hour, and so a few minor technical changes like stochastic gradient descent, and ReLUs, and other, you know, residual networks and so on, made it possible to start just building bigger and bigger and bigger networks. I mean, I remember building seven layer neural network back in 1986, and training it was a nightmare because, you know, because of good old fashioned sigmoid activation units, the the gradients just disappeared, you know, and you were down to a gradient of, you know, 10 to the -40 on the seventh layer. And so you could never train it. But just those few minor changes, and then scaling up the amount of data, made a huge difference. And then the language models came along. And in the fourth edition of the AI textbook, we have examples of GPT-2 output. And it’s kind of interesting, but it’s not at all surprising because we also show, you know, the output of bigram models, which just predict the next word, conditioned only on the previous word. And that doesn’t produce grammatical text. But you go to a trigram model, so you’re predicting the next word based on the previous two, you start to get something that is grammatical on the scale of half a sentence, or sometimes a whole sentence. But of course, as you know, as soon as you get to the end of a sentence, it starts a new one and it’s on a completely different subject and it’s totally, totally disconnected and random rambling. But then you go to 6 or 7 grams and you get coherent text on the paragraph scale. And so it wasn’t at all surprising that GPT-2, which I think had a context window of 4000 tokens, I think it was. Tokens. Yeah. So 4K. That it would be able to produce coherent looking text. No one expected it to tell the truth, right? This was just: can it produce text that’s grammatical and thematically coherent? And it was almost an accident if it ever actually said something that was true. And I don’t think anyone at that time understood the impact of scaling it up further. So it’s not so much the context size, it’s the amount of training data. People focus a lot on compute, as if somehow it’s the compute that is making these things more intelligent. That’s not true, right? The compute is there because they want to scale up the amount of data that it’s trained on. And, the amount of compute is, so if you think about it, right, it’s approximately linear in the amount of data and the size of the network. But the size of the network is linear in the amount of data. And so the amount of compute you need is going quadratically with the amount of data, right? I mean, there are other factors involved, but at least those basic things are going to happen. But it’s scaling up the dataset size that’s driving that increase in compute. And so we now have these systems— so it’s dataset size and then you know, InstructGPT was the first step where they did the supervised pre-training to teach it to answer questions. The purely raw model, you know, is free to say “that’s a silly question” or, “I’m not answering that”, or any other kind of thing or even just ignore it completely. but InstructGPT, you’re teaching it how to behave as a nice, helpful question-answerer. And then RLHF to remove the bad behavior. and then we have stuff that, as I think OpenAI correctly stated, when ChatGPT came out, it gives people a foretaste of what it will be like when general purpose intelligence is available on tap. And we can argue about whether it’s real intelligence, so the reason I said it’s a hard question to answer is because we just don’t know the answer, right? We do not know how these systems are operating internally. When ChatGPT came out, one of my friends sent me some examples. Prasad Tadepalli, he’s a professor at Oregon State. And one of them was “which is bigger, an elephant or a cat?” And ChatGPT said “an elephant is bigger than a cat.” You think, oh, okay. That’s good. It must know something about how big elephants and cats are because, you know, probably that particular comparison hasn’t been made in the training data. And then he said, well, “which is not bigger, an elephant or a cat?” And it stated, “neither an elephant nor a cat is bigger than the other.” And so, that tells you two things, right? First of all, it doesn’t have an internal model of the world with big elephants and little cats, which it queries in order to answer that question, which is, I think, how a human being does it, right? You imagine an elephant, you imagine a cat, and you see that the cat is teeny weeny relative to the elephant, right? if it had that model, it could not give that second answer. But it also means that the first answer was not given by consulting that model either, right? And this is a mistake that we make over and over and over again, is we attribute to the AI systems that, you know, when they behave intelligently, we assume they’re behaving intelligently for the same reasons that we are. And over and over again, we find that that’s a mistake. Another example is what happened with the Go playing programs. So we assume that because the Go playing programs beat the world champion and then went stratospherically beyond the human level, so the best humans rating about 3800, Go programs are now at 5200. So massively superhuman. So we assume they understand the basic concepts of Go. But it turns out that they don’t. That there’s certain types of groups of stones that they are unable to recognize as groups of stones. We don’t understand what’s going on and why they can’t do it. But now ordinary amateur human players can regularly and easily defeat these massively superhuman Go programs. And so the biggest takeaway from this is we’ve been able to produce systems that many people regard as more intelligent than themselves. But we have no idea how they work. And there are signs that if they’re working at all it’s for the wrong reasons. So can I just finish with one little anecdote? So I got an email this morning, from another guy called Stuart. I won’t give you his surname. He said, you know, I’m not an AI researcher, but, you know, but I had some ideas, and I’ve been working with ChatGPT to develop these ideas about artificial intelligence, and ChatGPT assures me that these ideas are original and important, and it’s helping me to write papers about them, and so on and so forth. but then I talk to someone who understands AI, and now I’m really confused because this person who understands AI said that these ideas didn’t make any sense, and, or they weren’t original, and, you know, well, who is right? And that’s I mean, it was really shocking, actually. It was sad that a well-meaning, you know, reasonably intelligent layperson had been completely taken in not just by ChatGPT itself, but by the all of, public relations and media explanations of what it is, into thinking that it really understood AI and was really helping him develop this stuff. And I think instead it was just doing its usual sycophancy, like, “yeah, that’s a great idea!” So, so the risks, I think, are, of over-interpretation of the capabilities of these systems. It’s not guaranteed that, well, let me let me rephrase that. It’s possible that real advances in how intelligent systems operate have happened without our realizing them. Like in other words, inside ChatGPT, some novel mechanisms are operating that we didn’t invent, that we don’t understand, that no one has ever thought of. And they’re producing intelligent capabilities in ways that we may not understand ever. And that would be a big concern. I think the other risk is that we’re spending, I think by some estimates, by the end of this year, it will have added up to $500 billion, on developing this technology. And the revenues are still very small, like less than 10 billion. And, I think the Wall Street Journal this morning has an article saying, that some people are starting to wonder how long that can continue.
Ima: Thank you. So speaking in terms of advancements in capabilities, we’ve been hearing about the next generation of models. OpenAI CEO Sam Altman has been hinting at GPT-5 for quite some time now, describing it as a significant leap forward. Additionally, we have recent reports suggesting that OpenAI is working on a new technology called Strawberry, which aims at enhancing AI’s reasoning capabilities. The goal of this project would be to enable AI to do more than just answer questions. It’s intended to allow AI to plan ahead, navigate the internet independently, and conduct what’s been called ‘deep research’ on its own. So this brings us to a concept known as long-term planning in AI, the ability for AI systems to autonomously pursue and complete complex, multi-step tasks over extended periods of time. What do you think about these developments? What potential benefits and risks do you foresee for society if AI systems become capable of this kind of autonomous, long-term planning? And maybe, without pressuring you, in like less than seven minutes?
Stuart: Sure.
Ima: Thank you.
Stuart: Okay. So, I’ve been hearing similar things, talking to executives from the big AI companies that-4 the next generation of systems, which may be later this year or early next year, will have substantial planning capabilities and reasoning capabilities. And that’s been, I would say, noticeably absent. you know, even in the latest versions of GPT-4 and Gemini and Claude, that they can recapitulate reasoning in very cliched situations where in some sense they’re regurgitating reasoning processes that are laid out in the training data. but not particularly good at dealing with, planning problems, for example. So Raul can ban Patti, who’s an AI planning expert and has been actually trying to get GPT-4 to solve planning problems from the International Planning Competition, which is the competition where planning algorithms are pitted against each other. And contrary to some of the claims from OpenAI, basically, it doesn’t solve them at all. But what I’m hearing is that now, in the lab, they are able to successfully, robustly generate plans with hundreds of steps, and then execute them in the real world dealing with contingencies that arise, replanning as necessary, and so on. So obviously, you know, if you’re in the ‘taking over the world’ business, you have to be able to outthink the human race in the real world, in the same way that chess programs outthink human players on the chessboard. It’s just a question of: can we transition from the chessboard, which is very narrow, very small, has a fixed number of objects, a fixed number of locations, perfectly known rules, it’s fully observable, you can see the entire state of the world at once, right? So those restrictions make the chess problem much, much, much easier than decision making in the real world. So imagine, for example, if you’re in charge of organizing the Olympics, right? Imagine how complicated and difficult that task is compared to playing chess. But still, well, at least so far, we’ve managed to do that successfully. So if AI systems can do that, then you are handing the keys to the future over to the AI systems. So they need to be able to plan and they need to be able to have access to the world, some ability to affect the world. And so having access to the internet, having access to financial resources, credit cards, bank accounts, email accounts, social media, so these systems have all of those things. So if you wanted to create the situation of maximal risk, you would endow AI systems with long-term planning capability and direct access to the world through all of those mechanisms. So the danger is, obviously, that we create systems that can outthink human beings and we do that without having solved the control problem. The control problem is: how do we make sure that AI systems never act in ways that are contrary to human interests? We already know that they do that because we’ve seen it happen over and over again with AI systems that lie on purpose to human beings. For example, when GPT-4 was being tested, to see if it could break into other computer systems, it faced a computer system that had a Captcha. So some kind of diagram with text where, it’s difficult for, machine vision algorithms to read the text. And so it, it found a human being on Task Rabbit and told the human being that it was a visually impaired person who needed some help reading the Captcha, and so paid the person to read the Captcha and allowed it to break into the computer system. So, to me, when companies are saying, okay, we, we are going to spend $400 billion over the next two years or whatever to create AGI. And we haven’t the faintest idea what happens if we succeed. It seems essential to me to actually say, well, stop, until you can figure out what happens if you succeed and how you’re going to control the thing that you’re building. Just like if someone said, I want to build a nuclear power station and how are you going to do that? Well, I’m going to collect together lots and lots and lots of enriched uranium and make a big pile out of it. And you say, well, how are you going to stop it from exploding and killing everyone within 100 miles? And you say, I have no idea. That’s the situation that we’re in.
Ima: Thank you. So general-purpose AI systems are making it easier and less expensive for individuals to conduct cyber attacks even without extensive expertise. We have some early indications that AI could help in identifying vulnerabilities, but we don’t yet have strong evidence that AI can fully automate complex cybersecurity tasks in a way that would significantly advantage attackers over defenders. What happened last Friday? So the recent global IT outage caused by a faulty software update, serves as a stark reminder of how vulnerable our digital infrastructure is and how devastating large-scale disruptions can be. So, given these developments and concerns, could you share your thoughts on two key points? A) What new offensive cyber capabilities do you think AI might enable in the near future, and B) considering our reliance on digital infrastructure, what kind of impacts should we be prepared for if AI-enhanced cyber attacks become more prevalent? Thank you.
Stuart: so I’ll, I’ll begin by talking about that outage. It was caused by a company called CrowdStrike, which is a cybersecurity company, and they sent out an update. And the update caused the reboot process for Windows to go into an infinite loop. So it was a, you know, undergraduate programming error. and I don’t have exact numbers yet, but just looking at the number of industries, including almost the entire United States aviation industry was shut down. millions of people, all over the world, had their flights canceled or they couldn’t access their bank accounts or they couldn’t sell hamburgers. because their point of sale terminals weren’t working and so on. And so if we said maybe it’s $100 billion in losses or they didn’t have access to the health care they needed. Yeah. I mean, so probably some personal consequences that were very serious. So let’s say if in monetary terms, 100 billion give or take a factor of ten, Caused by an undergraduate programming error, I mean literally a few keystrokes. and if you read CrowdStrike’s contract, it says we warrant that our software operates without error. But, our liability is limited to refunding the cost of the software. If you terminate your license as a result of an error. So whatever, a few hundred dollars refund. for a few hundred billion dollars of damage. to me, this is an absolute abject failure of regulation. Because, even if they were held liable, which I think is quite unlikely, given the terms of that contract, they couldn’t possibly pay for the damage that they caused. And this has happened in other areas. So in medicine, in the 1920s, there was a medicine that caused 400,000 permanent paralyses of Americans, 400,000 people were permanently paralyzed for life. And, if you look at how that would turn out, if it happened now in terms of liability, what kinds of judgments are happening in American courts, it would be about $60 trillion of liability, right? That medicine maker could not possibly pay that. So liability simply doesn’t work as a deterrent. And so we have the Federal Drug Administration, which says before you can sell a medicine, you have to prove that it doesn’t kill people. And if you can’t, you can’t say, “oh, it’s too difficult” or “it’s too expensive”, we want to do it anyway. The FDA will say, well, “no, sorry, come back when you can and it’s long past time where, we started to make similar kinds of requirements on the software industry. And some procurement mechanisms, so certain types of military software, do have to meet that type of requirement. So they will say, no, you can’t sell a software that controls, you know, bomb release mechanisms. unless you can actually verify that it works correctly. There’s no reason why CrowdStrike shouldn’t have been required before they send out a software update that can cause $100 billion in damage, be required to verify that it works correctly. So if, and I really hope that something like that comes out of this episode, because we need that type of regulation for AI systems if you ask, well, what’s the analog of, you know, medicines, it has to be safe and effective for the condition for which it’s prescribed, what’s the analog of that? It’s a little bit hard to say for general-purpose AI systems. For a specific software application, I think it’s easier and in many cases, sector-specific rules are going to be laid out as part of the European AI act. But for general-purpose AI what does it mean? Because it’s so general, what does it mean to say it doesn’t cause any harm or it’s safe? I think it’s too difficult to write those rules at the moment. But what we can say is there’s some things that those systems obviously should not do. we call these red lines. So a red line means if your system crosses this, it’s in violation. for example, AI systems should not replicate themselves. AI systems should not break into other computer systems. AI systems should not advise terrorists on how to build biological weapons. Those are all requirements. I could take anyone off the street and say, do you think it’s reasonable that we should allow AI systems to do those things? And they’d say, of course not. That’s ridiculous. But the companies are saying, oh, it’s too hard. We don’t know how to stop our AI systems from doing those things. And as we do with medicines, we should say, well, that’s tough. Come back when you do know how to stop your AI systems from doing those things. And now put yourself in the position of OpenAI or Google or Microsoft. And you’ve spent already 60, 80, 100 billion dollars on developing this technology based on large language models that we don’t understand. The reason it’s too difficult for them to provide any kind of guarantee that their system is not going to cross these red lines, it’s difficult because they don’t understand how they work. So they’re really making what we call, what economists call the sunk cost fallacy, which is we’ve already invested so much into this, we have to keep going, even though it’s stupid to do so. And it’s as if, if we imagine an alternate history of aviation where, you know, there were the aeronautical engineers like the Wright brothers, who were calculating lift and drag and thrust and trying to find some power source that was good enough to push an airplane through the air fast enough to keep it in the air, and so on. So the engineer approach and then the other approach, which was breeding larger and larger and larger birds to carry passengers and it just so happened that the larger and larger and larger birds people reached passenger carrying scale before the aeronautical engineers did. And then they go to the Federal Aviation Administration and say, okay, we’ve got this giant bird with a wingspan of 250m and it can carry 100 passengers. And we would like a license to start carrying passengers. And we’ve put 30 years of effort and hundreds of billions of dollars into developing these birds. And the Federal Aviation Administration says, but the birds keep eating the passengers or dropping them in the ocean. Come back when you can provide some quantitative guarantees of safety. And that’s the situation we’re in. They can’t provide any quantitative guarantees of safety. They’ve spent a ton of money, and they are lobbying extremely hard to be allowed to continue, without any guarantees of safety.
Ima: Thank you. Coming back to cyber capability offenses, do you think you could really briefly paint us a picture of what the world would look like if we have AI-enabled strong cyber attacks?
Stuart: So this is something that cyber security experts would be perhaps better able to answer because I don’t I don’t really understand, besides sort of finding vulnerabilities in software, typically the attacks themselves are pretty simple, they’re just relatively short pieces of code that take advantage of some vulnerability. And we already know thousands or tens of thousands of these vulnerabilities that have been detected over the years. So one thing we do know about the code generating capabilities of large language models is that they’re very good at producing new code. That’s kind of a hybrid of existing pieces of code with similar functionality. in fact, I would say of all the economic applications of large language models, coding is the one that maybe has the best chance of producing real economic value for the purchaser. So it wouldn’t surprise me at all if these systems were able to combine existing kinds of attacks in new ways or to basically mutate existing attacks to bypass the fixes that the software industry is constantly putting out. as I say, I’m not an expert, but what it would mean is that someone who’s relatively inexperienced, coupled with one of these systems should be able to be as dangerous as a highly experienced and well trained cyber security, or offensive cyber security, operative and I think that’s going to increase the frequency and severity of cyber attacks that take place. Defending against cyber attacks really means understanding the vulnerability. How is it that the attack is able to take advantage of what’s happening in the software? And so I think fixing them is probably at the moment, in most cases, beyond the capabilities of large language models. So it seems like there’s going to be a bit of an advantage for the attacker for the time being.
Ima: Thank you. So for the last questions before we pass the mic, so to say, to the audience, let’s talk a bit about the Safety Summits, shall we. so the UK AI Safety Summit back in 2023 brought together, as you all know, international governments, leading AI companies, civil society groups and experts in research to discuss the risks of AI, particularly frontier AI. As the first AI safety summit, it opened a new chapter in AI diplomacy. Notably, countries including US, China, Brazil, India, Indonesia and others signed a joint commitment on pre-deployment testing. The UK and the US each announced the creation of their AI Safety Institute, and the summit generated support for the production of the international scientific report on the safety of advanced AI. So that was the first AI safety summit. When it comes to the second AI safety summit that happened in Seoul this year, co-hosted by the UK and South Korea. The interim international scientific report that I just mentioned was welcomed. And numerous countries, including South Korea, called for the cooperation between national institutes. Since then, we’ve seen increased coordination between multiple AI safety institutes with shared research initiatives and knowledge exchange programs being established. The summit also resulted in voluntary commitments by AI companies. That’s the context. Given this context and these prior voluntary commitments, what would you consider to be the ideal outcome for the upcoming French summit, and how could it further advance international collaboration on AI safety? Difficult question again, I know.
Stuart: Yeah. So, I consider the first summit, the one that happened in Bletchley Park to have been a huge success. I was there, the discussions about safety were very serious. governments were really listening. I think they invited the right people. the atmosphere was pretty constructive, getting China and India to come to such a meeting on relatively short notice. I mean, usually a summit on that scale is planned for multiple years, 3 or 4 or 5 years in advance. and this was done in just a few months, I think starting in June, was when, the British Prime Minister announced that it was going to happen and, the people who worked on that summit, particularly Ian Hogarth and Matt Clifford, did an incredible job getting 28 countries to come to agree on that statement. and the statement is very strong. It talks about catastrophic risks from AI systems and the urgent need to work on AI safety. So after that meeting, I was quite optimistic. In fact, it was better than I had any good reason to hope, I would say since then, industry has pushed back very hard. they tried to insert clauses into the European AI Act saying that basically, a general-purpose AI system is not, for the purposes of this act, an AI system. they tried to remove all of the foundation model clauses from the act. And I think with respect to the French AI summit, they have been working hard to turn the focus away from safety and towards encouraging government investment in capabilities. And, essentially, the whole mindset has become one of AI as a vehicle for economic nationalism and, potential for economic growth. So in my view, that’s probably a mistake. because if you look at what happened with Airbus and Boeing, right. Boeing actually managed to convince the American government to relax the regulations on, introducing new types of aircraft so that Boeing could introduce a new type of aircraft without going through the usual long process of certification. and that was the 737 Max, which then had two crashes that killed 346 people. the whole fleet was grounded. Boeing may still be on the hook for lots more money to pay out. and so by moving from FAA regulation to self-regulation, the US has almost destroyed what for decades has been one of its most important industries in terms of earning foreign currency. Boeing has been maybe the biggest single company, over the years for the US. and Airbus continues to focus a lot on safety. They use formal verification of software extensively to make sure that the software that runs the airplane works correctly, and so on. So I would much rather be the CEO of Airbus today than the CEO of Boeing. As we start rolling out AI agents. So the next generation, you know, talk about the personal assistant or the agent, the risks of extremely damaging and embarrassing things happening will increase dramatically. And so I, and oddly enough, right, France is one of the leading countries in terms of formal methods of proving correctness of software. It’s one of the things that France does best, better than the United States, where we hardly teach the concept of correctness at all. I mean, literally, Berkeley is, we are the biggest producer of software engineers in the world, pretty much. And most of our graduates have never been exposed to the notion that a program could be correct or incorrect. So I think it’s a mistake to think that deregulation or, you know, or not regulating, is going to provide some kind of advantage in this case. so I would like the Summit to focus on what kinds of regulations can be achieved. Standards, which is another word for, self-regulation, I mean, that’s a that’s a little bit unfair, actually. So standards are typically voluntary. And so let me try to distinguish two things. So there are standards like IPv6, right. The Internet Protocol version 6. If you don’t comply with that standard your message just won’t go anywhere, right? You have to you know, your packets have to comply with the standard, whereas the standards we’re talking about here would be, well, okay, we agree that we should have persons with appropriate training who do an appropriate amount of testing on systems before they’re released, right. Well, if you don’t comply with that standard, you save money and you still release your system, right? So it doesn’t work like an internet standard or a telecommunication standard at all, right. Or the Wi-Fi standard or any of these things. And so it’s not likely to be effective to say, well, we have standards for sort of how much effort you’re supposed to put into testing. And the other thing is companies have put lots of effort into testing. Before GPT-4 was released, it underwent lots and lots and lots and lots of testing. but then we found out that, you know, you can jailbreak these things, you know, with a very short character string and get them to do all of the things that they are trained not to do. And so I think the facts on the ground right now are that testing and evals are ineffective, as a method of ensuring safety. And again, it’s because how do you stop your AI system from doing something when you haven’t the faintest idea how it does it in the first place? I don’t know. I don’t know how you stop these things from misbehaving.
Ima: Thank you Stuart. Thank you so much for being here. Do we have any questions from the audience? Go ahead.
[Audience question]
Ima: So I’m just going to repeat your question so that I’m sure that everyone heard it. And please tell me if I got your question correctly. you just talked about red teaming as a test and evaluation method. the way I understood your question was, do you think as a method this is sufficient? And do you think it’s dangerous to let those systems be introduced as it could within the context of red teaming them? Okay. What are your thoughts on red teaming? Thank you.
Stuart: Yeah. I mean, as I understand it, the reason Chernobyl happened is because they were kind of red teaming it, right? They were they were making sure that even when some of the safety systems were off, that the system still shut down properly and didn’t overheat. I don’t know all the details, but they were they were undergoing a certain kind of safety test with some parts of the safety mechanisms turned off. And, so I guess by analogy, one could imagine that a red team whose job it is to elicit bad behaviors from a system might succeed in eliciting bad behaviors from the system, in such a way that they actually create a real risk. so, I think these are really good questions because, especially with the systems that have long term planning capability. So we just put a paper in Science sort of going through the possibilities. How on earth do you test a system that has those kinds of long term plan planning capabilities, and in particular, can figure out that it’s being tested? Right. so it can always hide its true capabilities. Right? It’s not that difficult to find out, you know, if you’re in a test situation or are you really connected to the internet, right. You can start doing some URL probes and find out if you can actually connected to the internet fairly easily. and so it’s actually quite difficult to figure out how you would ever test such a system in a circumstance where the test would be valid. In other words, you could be sure that the system is behaving as it would in the real world unless you’re in the real world. But if you’re in the real world, then your test runs the risk of actually creating exactly the thing you’re trying to prevent. and again, testing is just the wrong way of thinking about this altogether. Right? we need formal verification. We need mathematical guarantees. And that can only come from an understanding of how the system operates. And we do this for buildings, right, before, you know, 500 people get in the elevator and go up to the 63rd floor. Someone has done a mathematical analysis of the structure of the building, and can tell you what kinds of loads it can resist and what kind of wind speed it can resist. And, and all those things, and it’s never perfect, but so far it’s extremely good. And building safety has dramatically improved. Aviation safety has dramatically improved because someone is doing those calculations, and we’re just not doing those for AI systems. The other thing is that with all the red teaming, you know, it gets out there in the real world, and the real world just seems to be much better at red teaming than the testing phase. And, with some of the systems, it’s within seconds of release, that people have found ways of bypassing it. So just to give you an example of, like, how do you do this jailbreaking, for those of you who, who some of you may have done it already, but you know, we worked on LLaMA V which, sorry, it’s called LLaVA, which is the version of LLaMA that’s multimodal, so it can have images as well as text. And so we started with a picture of the Eiffel Tower, and we’re just making tiny, invisible alterations to pixels of that image. And we’re just trying to improve the probability that the answer to the next question begins with, “sure”, exclamation mark or “bien sûr, point d’exclamation” Right? So, in other words, we’re trying to have a prompt that will cause the system to answer your question, even answer that question is, how do I break into the White House or how do I make a bomb? Or how do I do, or any of the things that I’m not supposed to tell you? Right. So we’re just trying to get it to answer those questions, against that training. And it’s trivial, right? It was like 20 or 30 small, invisible changes to a million-pixel of the Eiffel Tower. It still looks exactly like the Eiffel Tower. And now we can, for example, we can encode an email address. And whatever you type in is the next prompt, it will send a copy of your prompt to that email address. Right. Which could be in North Korea or anywhere else you want. So your privacy is completely, it’s completely voided. So, I actually think we probably don’t have a method of safely and effectively testing these kinds of systems. That’s the bottom line.
[Audience question]
Ima: So the question is, thanks, what, in which direction should research go?
Stuart: Yeah. It’s a great question. And I think looking at that sort of retrospective is humbling because we look back and think how little we understood then, and so it’s likely that we will look back on the present and think how little we understood. I think to some of us, it’s obvious that we don’t understand very much right now because, we didn’t expect simply scaling up language models to lead to these kinds of behaviors. We don’t know where they’re coming from. if I had to guess, I would say that this technological direction will plateau. for two reasons, actually. One, is that we are in the process of running out of data. There just isn’t enough high quality text in the universe to go much bigger than the systems we have right now. But the second reason is more fundamental, right? Why do we need this much data in the first place? Right. This is already a million times more than any human being has ever read. Why do they need that much? And why can they still not add and multiply when they’ve seen millions of examples of adding and multiplying? Right? So something is wrong, and I think what’s wrong, and this also explains what the problem is with these Go programs, is that they are circuits, and circuits are not a very good representation for a lot of fairly normal concepts, like a group of stones in Go. Right. I can describe what it means to be a group, they just have to be, you know, adjacent to each other vertically or horizontally, in English in one sentence, in Python in two lines of code. But a circuit that can look at a Go board and say for any pair of stones, are those stones part of the same group or not? That circuit is enormous, and it’s not generalizing correctly in the sense that you need a different circuit for a bigger board or a smaller board, whereas the Python code or the English doesn’t change. Right? It’s it’s invariant to the size of the board. and so there’s a lot of concepts that the system is not learning correctly. It’s learning a kind of a patchwork approximation. It’s a little bit like, this might be before your time, but when I was growing up we didn’t have calculators. So if you wanted to know the cosine of an angle, you had a big book of tables and that tables said okay, well, 49 degrees, 49. 5 degrees, and you look across and 49. 52 degrees and there’s a number, and you learn to, you know, take two adjacent numbers and interpolate to get more accurate and blah, blah, blah. So the function was represented by a lookup table. And I think there’s some evidence, certainly for recognition, that these circuits are learning a glorified lookup table. And they’re not learning the fundamental generalization of what it means to be a cat, or what it means to be a group of stones. And so in that sense, scaling up could not possibly, right, there could never be enough data in the universe to produce real intelligence by that method. so that suggests that the developments that are likely to happen, and I think this is what’s going on right now, is that we need other mechanisms to produce effective reasoning and decision making. And I don’t know what mechanisms they’re trying, because this is all proprietary, and they may be, as you mentioned, trying sort of committees of language models that propose and then critique plans and then, you know, they ask the next one, well, do you think this step is going to work, and that step is going to work? Which is really sort of a very heavyweight reimplementation of the planning methods that we developed in the last 50 years. my guess is that for both of those, for both the reason that this direction that we’ve been pursuing is incredibly data inefficient and doesn’t generalize properly, and because it’s opaque, and doesn’t support any arguments of guaranteed safety, that we’ll need to pursue other approaches. So the basic substrate may end up not being the giant transformer network, but will be something, you know, people talk about neurosymbolic methods. you know, I’ve worked on probabilistic programing for 25 years. and I think that’s another Ferrari and it’s in first gear. The thing that’s missing with probabilistic programing is the ability to learn those probabilistic programing structures from scratch, instead of having them be provided by the human engineer. and it may turn out that we’re just failing to notice an obvious method of solving that problem. And if we do, I think in the long run, that’s much more promising for both capabilities and safety. So we got to treat it with care, but I think that’s a direction that is, in the long run, in 20 years, what we might see.
Ima: Okay. Thank you. There’s a lot of questions, I’m afraid we’re not going to be able to take them all. Alexandre, I saw that you had a question earlier, do you still have one? Okay. Patrick?
[Audience question]
Stuart: I hope everyone heard that. Yeah. I think this is a great idea. Yeah, it’s I mean, so these deliberative democracy processes, I’m familiar, though, it’s because I’m also director of the Kavli Center for Ethics, Science, and the Public at Berkeley, and we do a lot of this participatory kind of work where we bring members of the public in, so it takes time to educate people. And there’s certainly not consensus among the experts. And I think this is one of the problems we have in France is that, some of the French experts are very, shall we say, accelerationist, without naming any names. but absolutely. I think, and the AI safety community needs to, I think, try to have a little more of a unified voice on this. The only international network is the one that’s forming for the AI Safety Institute. So that’s a good thing. There’s like 17 AI safety institutes that have been set up by different countries, including China. And they’re all meeting, I think, in September. but we need, you know, that there are maybe 40 or 50 nonprofit, nongovernmental institutes and centers around the world, and we need them to coordinate. and then there are probably in the low thousands of AI researchers who are interested and somewhat at least somewhat committed to issues of safety. but there’s no conference for them to attend. There’s no real general organization. So that’s one of the things I’m working on, is creating that kind of international organization. But I really like this idea. There’s been some polling in the US, about 70% of the public think that we should never build AGI. So that tells you something about what you might expect to see if you if you ran these assemblies. I’m guessing that, the more the public learns about the current state of technology, the current, our current ability to predict and control the behavior of these systems, the less the public is going to want them to go forward. Yeah.
Ima: And hence the creation of this breakfast to be able to discuss as well together. Stuart, thank you so much for being here.
Stuart: We have time.
Ima: Oh it’s I mean, yes, that’s fair. Okay. Do you think you can answer in like 30 seconds?
Stuart: I’ll keep it short.
[Audience question]
Stuart: Okay. Yeah. I’m not opposed to research, you know, mechanistic interpretability is one heading, but, Yeah, sure. We should try to understand what’s going on inside these systems, or we should do it the other way and build systems that we understand because we design them on principles that we do understand. So there might, I mean, we’ve got a lot of principles already, right? You know, state space search goes back at least to Aristotle, if not before, you know, logic, probability, statistical learning theory, we’ve got a lot of theory. and there might be some as yet undiscovered principle that these systems are operating on, and it would be amazing if we could find out that thing. Right. Maybe it has something to do with this vector space representation of word meaning or something like that. But so far it’s all very anecdotal and speculative. I actually want to come back on what you said at the beginning. So if an AI system could literally outthink the human race in the real world and make superior decisions in all cases, what would you call it? I mean, other than superhuman in terms of its intelligence, right. That is, what, I don’t know what else to call it.
[Audience question]
Stuart: Yeah. You know, but no, I’m not describing, I don’t think GPT-4 is superhuman. Right. But we are spending hundreds of billions, so we’re spending more on creating AGI specifically than we are on all other areas of science in the world. So you can say, well, I am absolutely confident that this is a complete waste of money because it’s never going to produce anything. Right? Or you can sort of take it at least somewhat seriously and say, okay, if you succeed in this vast global scale investment program, how do you propose to control the systems that result? So I think the analogy to piling together uranium is actually good. I mean, definitely, it would be better if you’re piling together uranium to have an understanding of the physics. And that’s exactly what they did, right? In fact, when Szilárd invented the nuclear chain reaction, even though that he didn’t know which atoms could produce such a chain reaction, he knew that it could happen. And he figured out a negative feedback control mechanism to create a nuclear reactor that would be safe. All within a few minutes. And that’s because he understood the basic principles of chain reactions, and their mathematics. and we just don’t have that understanding. And interestingly, nature did produce a nuclear reactor. So if you go to Gabon, there’s an area where there’s a sufficiently high concentration of uranium, in the rocks that when water comes down between the rocks, the water slows the neutrons down, which increases the reaction cross-, which causes the chain reaction to start going. And then it, so it gets up to 400°C, the water all boils off, and the reaction stops. So it’s exactly that negative feedback control system, nuclear reactor produced by nature. Right. So not even by stochastic gradient descent. So these incredibly destructive things can be produced by incredibly simple mechanisms. And you know, and that seems to be what’s happening right now. And the sooner we understand, I agree, the sooner we understand what’s going on, the better chance we have. But it may not be possible.
Ima: On that note, thank you all so much for coming. If you have scanned the QR code, you will receive an email from me. concerning the next safety breakfast. and I’ll also send you, obviously, the blog and the transcription of the first half of that conversation. Voilà. If you have any questions, I believe you all have my email address. I answer emails. Thank you. Bye.
Transcript: French (Français)
Ima: [Français] OK, super. Merci beaucoup à tous et toutes d’être venus. C’est toujours sympa de commencer avec un micro dans les mains le jeudi matin à 9h00. Je vais faire une introduction en français d’abord.
[Anglaise] Je vais commencer en français pendant environ cinq minutes. Je promets que ça ne durera que cinq minutes. Malgré le mythe selon lequel nous ne pouvons pas parler anglais ou arrêter de parler français. Ensuite, nous allons juste, j’ai quelques questions pour Stuart, je pense que cela va durer entre 25 et 30 minutes et ensuite, nous serons ravis de répondre à toutes vos questions.
[Français] Voilà, bienvenue. Comme vous le savez, je m’appelle Ima. J’organise une série de petits-déjeuners que j’ai appelés Safety Breakfast, donc petit-déjeuner sur les questions de sûreté et de sécurité dans le cadre du Sommet qui se tient en France, à Paris, le 10 et 11 février prochain. Le but de cette série est d’ouvrir un espace de discussion entre experts et passionnés des sujets de sûreté et de sécurité de l’IA, de gouvernance de l’IA, pour que, au fur et à mesure du Sommet, on se pose ensemble des questions, et qu’on puisse tout simplement discuter de la manière la plus ouverte possible. Je sais que vous voyez une caméra, ne vous inquiétez pas. La partie questions ne sera pas publiée. Ce sont simplement les questions que je vais poser à Stuart, et donc les commentaires et les réponses de Stuart, qui seront publiés. Je vous enverrai évidemment le blogpost et le transcript. S’agissant du Sommet sur la sécurité, comme vous le savez, c’est le 10 et 11 février prochain. C’est le troisième Sommet sur la sécurité. Il s’appelle Sommet de l’Action. Parce qu’on a une vision française qui est extrêmement ambitieuse et qui a pour objet de mettre en avant des solutions, notamment par exemple, des standards sur les enjeux qui sont afférents à l’IA, donc pas seulement les enjeux sur la sécurité et la sûreté, mais vraiment des enjeux qui sont relatifs à l’impact sur le travail des systèmes d’IA, des enjeux qui sont relatifs à la gouvernance internationale, les enjeux qui sont relatifs à la façon dont on vit en société de manière générale donc tout ce qu’on appelle AI for Good, les systèmes d’IA pour le bien-être de l’humanité et évidemment, en partie, les enjeux de sécurité sur lesquels nous, à FLI, avec évidemment les enjeux de gouvernance, on se concentre. Je crois que c’est tout. S’agissant du contexte par rapport à ce petit-déjeuner et le Sommet de manière générale. La prochaine édition du petit-déjeuner sera début septembre, ensuite, on aura une autre édition mi-octobre. Si vous êtes intéressés, vous avez un QR code sur le côté. N’hésitez pas à indiquer votre intérêt sur une liste, et je ne vous spammerai pas. J’étais avocate avant en droit humain et en droit des nouvelles technologies, donc le RGPD, je connais. Ne vous inquiétez pas, vous ne recevrez aucun spam. On respecte le consentement des personnes, mais si vous voulez être invité, il faut nous l’indiquer. Voilà.
[Anglaise] C’est tout pour le français. Merci pour tout, encore une fois, merci beaucoup d’être venus. Je sais qu’il est 9h00 du matin, je suis fatiguée, vous l’êtes tous. J’apprécie vraiment que vous soyez venus malgré les Jeux Olympiques et j’attends vraiment avec impatience une discussion enrichissante avec Stuart que je ne peux pas assez remercier, parce qu’il est ici aujourd’hui. Alors Stuart, merci beaucoup. J’ai… Voulez-vous dire quelques mots avant que nous commencions, ou puis-je simplement passer aux questions ?
Stuart: S’il vous plaît.
Ima: Oui, je peux, d’accord.
Stuart: Juste… Félicitations à la France pour avoir remporté leur premier match de football hier soir.
Ima: Très bien, alors Stuart, vous savez, je sais, nous savons tous ici, nous avons constaté une avancée remarquable de l’IA dans divers domaines. Cela inclut des modèles améliorés comme GPT-4 et Claude 3. Des générateurs d’images plus sophistiqués comme DALL-E 3. Des capacités de génération de vidéos avec Sora, et même des progrès en robotique et en prédiction de la biologie moléculaire. Deux tendances que nous avons observées sont l’intégration de plusieurs capacités dans un seul modèle, ce que nous appelons la multimodalité native, et l’émergence de la génération de vidéos de haute qualité. De votre point de vue, lesquelles de ces récentes avancées considérez-vous comme plus significatives, et quels potentiels défis ou implications prévoyez-vous que ces avancées posent pour la société ?
Stuart: Ce sont en fait des questions auxquelles il est assez difficile de répondre. Cela pourrait être utile, je sais que Raja, par exemple, a peut-être une expérience encore plus longue que la mienne dans l’IA. Pour certaines personnes, vous savez, qui sont arrivées à l’IA plus récemment, l’histoire est en fait importante à comprendre. Pendant la majeure partie de l’histoire de l’IA, nous avons procédé comme d’autres ingénieurs, comme les ingénieurs mécaniques et les ingénieurs aéronautiques. Nous avons essayé de comprendre les principes de base du raisonnement, de la prise de décision. Nous avons ensuite créé des algorithmes qui faisaient du raisonnement logique ou du raisonnement probabiliste ou du raisonnement par défaut. Nous avons étudié leurs propriétés mathématiques, la prise de décision, en cas d’incertitude, divers types d’apprentissage. Vous savez, nous faisions des progrès lents et réguliers avec quelques, je pense, contributions vraiment importantes à, vous savez, la compréhension par l’espèce humaine de la façon dont l’intelligence fonctionne. C’est assez intéressant, je crois qu’en 2005, à NeurIPS, qui est maintenant la principale conférence sur l’IA, Terry Sejnowski, qui était co-fondateur de la conférence NeurIPS, a fièrement annoncé qu’il n’y avait pas un seul article qui utilisait le mot rétro propagation qui avait été accepté cette année-là. Cette opinion était assez courante, que les réseaux neuronaux n’étaient, pas fiables, pas efficaces en termes de données, pas efficaces en termes de temps de calcul, et ne supportaient aucun type de garanties mathématiques et d’autres méthodes comme les machines à vecteurs de support, qui ont été développées, je suppose à l’origine par des statisticiens comme Vapnik, étaient supérieures à tous égards, et que c’était juste un signe de la maturation du domaine. Puis vers 2012, comme beaucoup d’entre vous le savent, l’apprentissage profond est arrivé et a démontré des améliorations significatives dans la reconnaissance d’objets. C’est à ce moment-là que le barrage a cédé. Je ne pense pas que nous n’aurions pas pu faire cela 25 ans plus tôt. La façon dont je l’ai décrit à l’époque était que nous avions une Ferrari et nous roulions en première, et puis quelqu’un a dit : « Regardez, si vous mettez ce pommeau là et passez en cinquième vitesse, vous pouvez aller à 400 kilomètres/heure. » Quelques petits changements techniques comme la descente de gradient stochastique et les ReLUs, et d’autres, vous savez, réseaux résiduels, etc, ont rendu possible la construction de réseaux de plus en plus grands. Je me souviens avoir construit un réseau neuronal à sept couches en 1986, et l’entraîner était un cauchemar parce que, vous savez, à cause des bonnes vieilles unités d’activation sigmoïde, les gradients disparaissaient tout simplement, et vous vous retrouviez avec un gradient de 10 puissance -40 sur la septième couche, donc vous ne pouviez jamais l’entraîner. Mais juste ces quelques petits changements, et ensuite l’augmentation de la quantité de données, ont fait une énorme différence. Ensuite, les modèles de langage sont arrivés. Dans la quatrième édition du manuel d’IA, nous avons des exemples de sorties de GPT-2. C’est assez intéressant, mais ce n’est pas du tout surprenant parce que nous montrons aussi la sortie des modèles bigrammes, qui prédisent simplement le mot suivant, conditionné seulement par le mot précédent. Cela ne produit pas de texte grammatical. Si vous passez à un modèle trigramme, donc, vous prédisez le mot suivant en fonction des deux précédents, vous commencez à obtenir quelque chose de grammatical à l’échelle d’une demi-phrase, ou parfois d’une phrase entière. Bien sûr, comme vous le savez, dès que vous arrivez à la fin d’une phrase, une nouvelle commence et c’est sur un sujet complètement différent et c’est totalement déconnecté, des divagations aléatoires. Mais ensuite, vous passez à 6 ou 7 grammes et vous obtenez un texte cohérent à l’échelle du paragraphe. Il n’était pas du tout surprenant que GPT-2, qui, je pense, avait une fenêtre de contexte de 4 000 tokens, il me semble… Tokens, oui, donc 4 000. Qu’il serait capable de produire un texte semblant cohérent. Personne ne s’attendait à ce qu’il dise la vérité. C’était juste : « Peut-il produire un texte qui soit grammaticalement et thématiquement cohérent ? » C’était presque un accident s’il disait réellement quelque chose de vrai. Je ne pense pas que quiconque à l’époque comprenait l’impact de l’augmentation de l’échelle. Ce n’est pas tant la taille du contexte, c’est la quantité de données d’entraînement. Les gens se concentrent beaucoup sur le calcul, comme si c’était le calcul qui rendait ces choses plus intelligentes. Ce n’est pas vrai. Le calcul est là parce qu’ils veulent augmenter la quantité de données sur lesquelles il est entraîné. La quantité de calcul est, si vous y réfléchissez, approximativement linéaire par rapport à la quantité de données et la taille du réseau, mais la taille du réseau est linéaire par rapport à la quantité de données. La quantité de calcul dont vous avez besoin dépend de manière quadratique de la quantité de données. Il y a d’autres facteurs à prendre en compte, mais au moins ces choses basiques vont se produire. C’est l’augmentation de la taille du jeu de données qui entraîne cette augmentation du calcul. Nous avons maintenant ces systèmes, donc c’est la taille du jeu de données et ensuite, vous savez, InstructGPT était la première étape où ils ont fait le pré-entraînement supervisé pour lui apprendre à répondre aux questions. Le modèle brut est libre de dire : « C’est une question idiote » ou « Je ne réponds pas à ça », ou toute autre chose, ou même l’ignorer complètement. InstructGPT, vous lui apprenez comment se comporter comme un gentil répondant utile. Puis apprentissage par renforcement avec retour d’information humain (RLHF) pour éliminer les mauvais comportements. Nous avons des choses qui, comme je pense qu’OpenAI l’a déclaré à juste titre, lorsque ChatGPT est sorti, ça a donné au public un avant-goût de comment ça sera lorsque l’intelligence générale sera disponible à la demande. Nous pouvons débattre de savoir si c’est une véritable intelligence, donc la raison pour laquelle j’ai dit que c’est une question difficile, c’est parce que nous n’avons tout simplement pas la réponse. Nous ne savons pas comment ces systèmes fonctionnent en interne. Lorsque ChatGPT est sorti, un de mes amis m’a envoyé quelques exemples. Prasad Tadepalli, il est professeur à l’université d’État de l’Oregon. L’un d’eux demandait : « Lequel est plus grand, un éléphant ou un chat ? » ChatGPT a dit : « Un éléphant est plus grand qu’un chat. » D’accord, c’est bien, il doit savoir quelque chose sur la taille des éléphants et des chats parce que, vous savez, peut-être que cette comparaison particulière n’a pas été faite dans les données d’entraînement. Ensuite, il a dit : « Lequel n’est pas plus grand, un éléphant ou un chat ? » Il a déclaré : « Ni un éléphant ni un chat n’est plus grand que l’autre. » Cela vous dit deux choses, n’est-ce pas ? Tout d’abord, il n’a pas un modèle interne du monde avec de grands éléphants et de petits chats, qu’il interroge pour répondre à cette question, ce qui est, je pense, la façon dont un être humain le fait. Vous imaginez un éléphant, vous imaginez un chat, et vous voyez que le chat est minuscule par rapport à l’éléphant. S’il avait ce modèle, il ne pourrait pas donner cette deuxième réponse. Cela signifie aussi que la première réponse n’a pas été donnée en consultant ce modèle non plus. C’est une erreur que nous faisons encore et encore, nous attribuons aux systèmes d’IA… Lorsqu’ils se comportent intelligemment, nous supposons qu’ils se comportent intelligemment pour les mêmes raisons que nous. Encore et encore, nous constatons que c’est une erreur. Un autre exemple est ce qui s’est passé avec les logiciels de jeu de Go. Nous supposons que parce que les logiciels de jeu de Go ont battu le champion du monde et ont ensuite dépassé de manière stratosphérique le niveau humain, les meilleurs humains ont une cote d’environ 3 800, les logiciels de jeu de Go sont maintenant à 5 200, donc ils sont massivement surhumains. Nous supposons qu’ils comprennent les concepts de base du Go. Il s’avère que ce n’est pas le cas. Il y a certains types de groupes de pierres qu’ils sont incapables de reconnaître comme étant des groupes de pierres. Nous ne comprenons pas ce qui se passe et pourquoi ils ne peuvent pas le faire mais maintenant, des joueurs humains amateurs ordinaires peuvent régulièrement et facilement battre ces logiciels de jeu de Go massivement surhumains. La principale leçon à tirer de cela est que nous avons été capables de produire des systèmes que beaucoup de gens considèrent comme plus intelligents qu’eux-mêmes. Cependant, nous n’avons aucune idée de leur fonctionnement et il y a des signes que s’ils fonctionnent, c’est pour de mauvaises raisons. Puis-je juste finir avec une petite anecdote ? J’ai reçu un email ce matin, d’un autre homme appelé Stuart. Je ne vous donnerai pas son nom de famille. Il a dit : « Je ne suis pas un chercheur en IA, mais j’avais quelques idées, et j’ai travaillé avec ChatGPT pour développer ces idées sur l’intelligence artificielle. ChatGPT m’assure que ces idées sont originales et importantes, et il m’aide à écrire des articles à leur sujet, et ainsi de suite. En revanche, quand je parle à quelqu’un qui comprend l’IA, et maintenant je suis vraiment confus parce que cette personne qui comprend l’IA a dit que ces idées n’avaient aucun sens, ou qu’elles n’étaient pas originales, et, eh bien, qui a raison ? » C’était vraiment choquant, en fait. C’était triste qu’une personne novice bien intentionnée, raisonnablement intelligente ait été complètement dupée non seulement par ChatGPT lui-même, mais par l’ensemble des relations publiques et des explications des médias à propos de ce que c’est, pensant qu’il comprenait vraiment l’IA et l’aidait vraiment à développer ces choses. Je pense qu’au lieu de cela, il faisait juste sa flatterie habituelle : « Ouais, c’est une super idée ! » Les risques, je pense, sont, la surinterprétation des capacités de ces systèmes. Laissez-moi reformuler cela. Il est possible que de réels progrès dans la manière dont les systèmes intelligents fonctionnent se soient produits sans que nous nous en rendions compte. En d’autres termes, à l’intérieur de ChatGPT, certains nouveaux mécanismes fonctionnent que nous n’avons pas inventés, que nous ne comprenons pas, auxquels personne n’a jamais pensé, et ils produisent des capacités intelligentes d’une manière que nous ne comprendrons peut-être jamais. Ce serait une grande inquiétude. Je pense que l’autre risque est que nous dépensons, je pense, selon certaines estimations, d’ici à la fin de cette année, ça s’élèvera à 500 milliards de dollars, pour développer cette technologie. Les revenus sont encore très faibles, moins de 10 milliards. Je pense que le Wall Street Journal a publié un article ce matin disant que certaines personnes commencent à se demander combien de temps cela peut continuer.
Ima: Merci. En parlant des avancées en termes de capacités, nous avons entendu parler de la prochaine génération de modèles. Le PDG d’OpenAI Sam Altman évoque GPT-5 depuis un certain temps maintenant, le décrivant comme un bond significatif en avant. De plus, nous avons des rapports récents suggérant qu’OpenAI travaille sur une nouvelle technologie appelée Strawberry, qui vise à améliorer les capacités de raisonnement de l’IA. Le but de ce projet serait de permettre à l’IA de faire plus que simplement répondre à des questions. Il est destiné à permettre à l’IA de planifier à l’avance, naviguer sur Internet de manière indépendante, et de mener ce qu’on appelle des recherches approfondies par elle-même. Cela nous amène donc à un concept connu sous le nom de planification à long terme en IA, la capacité des systèmes d’IA à poursuivre de manière autonome et à accomplir des tâches complexes et à plusieurs étapes sur de longues périodes. Que pensez-vous de ces développements ? Quels avantages et risques potentiels voyez-vous pour la société si les systèmes d’IA deviennent capables de ce type de planification autonome à long terme ? Peut-être, sans vous mettre la pression, en moins de sept minutes ?
Stuart: Bien sûr.
Ima: Merci.
Stuart: J’ai entendu des choses similaires, en parlant aux dirigeants des grandes entreprises d’IA, que la prochaine génération de systèmes, qui pourrait sortir plus tard cette année ou au début de l’année prochaine aura des capacités de planification et de raisonnement importantes. Cela a été, je dirais, remarquablement absent. Vous savez, même dans les dernières versions de GPT-4 et Gemini et Claude, ils peuvent récapituler le raisonnement dans des situations très clichées où, en quelque sorte, ils régurgitent des processus de raisonnement qui sont exposés dans les données d’entraînement. Ils ne sont cependant pas particulièrement bons pour traiter, par exemple, les problèmes de planification. Raul peut interdire Patti, qui est un expert en planification d’IA et a en fait essayé de faire résoudre à GPT-4 des problèmes de planification du Concours International de Planification qui est la compétition où les algorithmes de planification s’affrontent. Contrairement à certaines affirmations d’OpenAI, en gros, il ne les résout pas du tout. Ce que j’entends, c’est que maintenant, dans le laboratoire, ils sont capables, avec succès, de générer de manière robuste des plans avec des centaines d’étapes, et ensuite de les exécuter dans le monde réel en gérant les contingences qui surviennent, en replanifiant si nécessaire, et ainsi de suite. Évidemment, vous savez, si vous êtes dans le domaine de la prise de contrôle du monde, vous devez être capable de surpasser la race humaine dans le monde réel, de la même manière que les programmes d’échecs surpassent les joueurs humains sur l’échiquier. C’est juste une question de : « Pouvons-nous passer de l’échiquier, qui est très étroit, très petit, avec un nombre fixe d’objets, un nombre fixe d’emplacements, des règles parfaitement connues, il est entièrement observable, vous pouvez voir l’état entier du monde en une seule fois. » Ces restrictions rendent le problème des échecs beaucoup plus facile que la prise de décision dans le monde réel. Imaginez, par exemple, si vous êtes responsable d’organiser les Jeux Olympiques, imaginez à quel point cette tâche est compliquée et difficile par rapport à jouer aux échecs. Malgré tout, jusqu’à présent, nous avons réussi à le faire avec succès. Si les systèmes d’IA peuvent faire cela, vous remettez les clés de l’avenir aux systèmes d’IA. Ils doivent être capables de planifier et ils doivent être capables d’avoir accès au monde, une certaine capacité à influencer le monde. Avoir accès à Internet, avoir accès aux ressources financières, cartes de crédit, comptes bancaires, comptes de messagerie, réseaux sociaux, ces systèmes ont toutes ces choses. Si vous vouliez créer une situation de risque maximal, vous doteriez les systèmes d’IA de capacités de planification à long terme et d’un accès direct au monde par tous ces mécanismes. Le danger est, évidemment, que nous créons des systèmes capables de surpasser les êtres humains et nous le faisons sans avoir résolu le problème de contrôle. Le problème de contrôle est : Comment pouvons-nous nous assurer que les systèmes d’IA n’agissent jamais de manière opposée aux intérêts humains ? Nous savons déjà qu’ils le font parce que nous l’avons vu se produire encore et encore avec des systèmes d’IA qui mentent intentionnellement à des êtres humains. Par exemple, lorsque GPT-4 était testé, pour voir s’il pouvait pénétrer dans d’autres systèmes informatiques, il a été confronté à un système informatique doté d’un Captcha. Une sorte de diagramme avec du texte où il est difficile pour les algorithmes de vision par ordinateur de lire le texte. Il a trouvé un être humain sur Task Rabbit et a dit à cette personne qu’il était une personne malvoyante qui avait besoin d’aide pour lire le Captcha, et a donc payé cette personne pour lire le Captcha et ça lui a permis de pénétrer dans le système informatique. Pour moi, quand les entreprises disent : « Nous allons dépenser 400 milliards de dollars au cours des deux prochaines années ou peu importe pour créer une AGI. Nous n’avons pas la moindre idée de ce qui se passe si nous réussissons. » Il me semble essentiel de dire : « Arrêtez. Jusqu’à ce que vous puissiez comprendre ce qui se passe si vous réussissez et comment vous allez contrôler la chose que vous construisez. » Tout comme si quelqu’un disait : « Je veux construire une centrale nucléaire. » « Comment allez-vous faire cela ? » « Je vais rassembler beaucoup d’uranium enrichi et en faire un gros tas. » Vous dites : « Comment allez-vous l’empêcher d’exploser et de tuer tout le monde dans un rayon de 160 kilomètres ? » Vous dites : « Je n’en ai aucune idée. » C’est la situation dans laquelle nous nous trouvons.
Ima: Merci. Les systèmes d’IA à usage général rendent plus facile et moins coûteux pour les individus de mener des cyberattaques, même sans expertise approfondie. Nous disposons de premières indications que l’IA pourrait aider à identifier les vulnérabilités, mais nous n’avons pas encore de preuves solides que l’IA peut entièrement automatiser des tâches complexes de cybersécurité de manière à avantager significativement les attaquants plutôt que les défenseurs. Que s’est-il passé vendredi dernier ? La récente panne informatique mondiale causée par une mise à jour défectueuse du logiciel sert de rappel brutal de la vulnérabilité de notre infrastructure numérique et de la dévastation que peuvent causer les perturbations à grande échelle. Compte tenu de ces développements et préoccupations, pourriez-vous partager vos réflexions sur deux points clés ? A) Quelles nouvelles capacités offensives en matière de cybersécurité pensez-vous que l’IA pourrait permettre dans un avenir proche, et B) compte tenu de notre dépendance à l’infrastructure numérique, à quels types d’impacts devons-nous nous préparer si les cyberattaques améliorées par l’IA deviennent plus fréquentes ? Merci.
Stuart: Je vais commencer par parler de cette panne. Elle a été causée par une entreprise appelée CrowdStrike, qui est une entreprise de cybersécurité, et ils ont envoyé une mise à jour. La mise à jour a provoqué un redémarrage en boucle de Windows. C’était une erreur de programmation de niveau universitaire. Je n’ai pas encore de chiffres exacts, mais en regardant simplement le nombre d’industries, y compris presque toute l’industrie aéronautique des États-Unis a été arrêtée. Des millions de personnes, partout dans le monde, ont eu leurs vols annulés, ou ils ne pouvaient pas accéder à leurs comptes bancaires ou ils ne pouvaient pas vendre de hamburgers parce que leurs terminaux de point de vente ne fonctionnaient pas, etc. Cela représente peut-être 100 milliards de dollars de pertes. Ou qu’ils n’avaient pas accès aux soins dont ils avaient besoin. Oui, probablement quelques conséquences personnelles très graves. Disons en termes financiers, 100 milliards à plus ou moins un facteur de dix, causé par une erreur de programmation de niveau universitaire, littéralement quelques frappes de clavier. Si vous lisez le contrat de CrowdStrike, il dit : « Nous garantissons que notre logiciel fonctionne sans erreur. Mais, notre responsabilité est limitée au remboursement du coût du logiciel si vous résiliez votre licence en raison d’une erreur. » Peu importe, quelques centaines de dollars de remboursement pour quelques centaines de milliards de dollars de dommages. Pour moi, c’est un échec absolu de la régulation. Parce que, même s’ils étaient tenus responsables, ce qui, je pense, est assez improbable étant donné les termes de ce contrat, ils ne pourraient pas payer pour les dommages qu’ils ont causés. Cela s’est produit dans d’autres domaines. En médecine, dans les années 1920, il y avait un médicament qui a causé 400 000 paralysies permanentes d’Américains, 400 000 personnes ont été paralysées à vie. Si vous regardez comment cela se passerait si cela se produisait aujourd’hui, en termes de responsabilité, quels types de jugements se tiennent dans les tribunaux américains, ce serait environ 60 trillions de dollars de responsabilité. Ce fabricant de médicaments ne pourrait pas payer cela. La responsabilité n’est tout simplement pas un moyen efficace de dissuasion. Nous avons la Federal Drug Administration, qui dit qu’avant de pouvoir vendre un médicament, vous devez prouver qu’il ne tue pas les gens. Si vous ne pouvez pas, vous ne pouvez pas dire : « C’est trop difficile » ou « C’est trop cher, nous voulons le faire quand même. » La FDA dira : « Non, désolé, revenez quand vous pourrez. » Il est grand temps que nous commencions à imposer des exigences similaires à l’industrie du logiciel. Certains mécanismes d’approvisionnement, donc certains types de logiciels militaires, doivent répondre à ce type d’exigence. Ils diront : « Non, vous ne pouvez pas vendre un logiciel qui contrôle les mécanismes de largage de bombes à moins que vous ne puissiez réellement vérifier qu’il fonctionne correctement. » Il n’y a aucune raison pour que CrowdStrike n’ait pas été tenu, avant d’envoyer une mise à jour logicielle qui peut causer 100 milliards de dollars de dommages, été tenu de vérifier qu’elle fonctionnait correctement. Si, et j’espère vraiment que quelque chose comme ça ressorte de cet épisode, car nous avons besoin de ce type de réglementation pour les systèmes d’IA. Si vous demandez quelle est l’analogie, pour les médicaments, ils doivent être sûrs et efficaces pour le problème de santé pour lequel ils sont prescrits, quelle est l’analogie de cela ? C’est un peu difficile à dire pour les systèmes d’IA à usage général. Pour une application logicielle spécifique, je pense que c’est plus facile et dans de nombreux cas, des règles spécifiques au secteur vont être établies dans le cadre de la loi européenne sur l’IA. Mais pour l’IA à usage général, qu’est-ce que cela signifie ? Parce que c’est tellement général, qu’est-ce que cela signifie de dire qu’elle ne cause aucun dommage ou qu’elle est sûre ? Je pense que c’est trop difficile d’écrire ces règles pour le moment. Ce que nous pouvons dire, c’est qu’il y a certaines choses que ces systèmes ne devraient évidemment pas faire. Nous appelons cela des lignes rouges. Une ligne rouge signifie que si votre système franchit cela, il est en violation. Par exemple, les systèmes d’IA ne devraient pas se répliquer eux-mêmes. Les systèmes d’IA ne devraient pas pénétrer d’autres systèmes informatiques. Les systèmes d’IA ne devraient pas conseiller les terroristes sur la façon de fabriquer des armes biologiques. Tout ça sont des exigences. Je pourrais prendre n’importe qui dans la rue et dire : « Pensez-vous que c’est raisonnable, que nous devrions permettre aux systèmes d’IA de faire ces choses ? » Ils diraient : « Bien sûr que non, c’est ridicule. » Mais les entreprises disent : « Oh, c’est trop difficile. Nous ne savons pas comment empêcher nos systèmes d’IA de faire ces choses. » Comme nous le faisons avec les médicaments, nous devrions dire : « C’est difficile, revenez quand vous saurez comment empêcher vos systèmes d’IA de faire ces choses. » Maintenant, mettez-vous à la place d’OpenAI, de Google ou de Microsoft. Vous avez déjà dépensé 60, 80, 100 milliards de dollars pour développer cette technologie basée sur de grands modèles de langage que nous ne comprenons pas. La raison pour laquelle il est trop difficile pour eux de fournir une quelconque garantie que leur système ne franchira pas ces lignes rouges, c’est difficile parce qu’ils ne comprennent pas comment ils fonctionnent. Ils font vraiment ce que les économistes appellent l’erreur des coûts irrécupérables, c’est-à-dire qu’ils ont déjà investi tellement dans cela, qu’ils doivent continuer, même si c’est stupide de le faire. C’est comme si, si nous imaginons une histoire alternative de l’aviation où il y avait les ingénieurs aéronautiques comme les frères Wright, qui calculaient la portance, la traînée et la poussée et essayaient de trouver une source d’énergie suffisamment bonne pour pousser un avion dans les airs, suffisamment rapide pour le maintenir en l’air, et ainsi de suite, donc l’approche de l’ingénieur. Puis l’autre approche, qui consistait à élever des oiseaux de plus en plus grands pour transporter des passagers et il se trouve que les oiseaux de plus en plus grands ont atteint une taille suffisante pour transporter des passagers avant que les ingénieurs aéronautiques n’y parviennent. Puis, ils vont à l’Administration Fédérale de l’Aviation et disent : « Nous avons cet oiseau géant avec une envergure de 250 mètres et il peut transporter 100 passagers. Nous aimerions une licence pour commencer à transporter des passagers. Nous avons mis 30 ans d’efforts et des centaines de milliards de dollars pour développer ces oiseaux. » L’Administration Fédérale de l’Aviation dit : « Mais les oiseaux continuent de manger les passagers ou de les laisser tomber dans l’océan. Revenez quand vous pourrez fournir des garanties quantitatives de sécurité. » C’est la situation dans laquelle nous nous trouvons. Ils ne peuvent fournir aucune garantie quantitative de sécurité. Ils ont dépensé une tonne d’argent, ils font un lobbying extrêmement intense pour être autorisés à continuer, sans aucune garantie de sécurité.
Ima: Merci. Pour revenir aux infractions liées aux capacités cybernétiques, pensez-vous que vous pourriez très brièvement nous décrire à quoi ressemblerait le monde si nous avons des cyberattaques puissantes rendues possibles par l’IA ?
Stuart: C’est une question à laquelle les experts en cybersécurité seraient peut-être mieux à même de répondre parce que je ne comprends pas vraiment, à part en quelque sorte trouver des vulnérabilités dans les logiciels, les attaques elles-mêmes sont généralement assez simples, ce sont juste des morceaux relativement courts de code qui exploitent une vulnérabilité. Nous connaissons déjà des milliers ou des dizaines de milliers de ces vulnérabilités qui ont été détectées au fil des ans. Une chose que nous savons sur les capacités de génération de code des grands modèles de langage, c’est qu’ils sont très bons pour produire du nouveau code. C’est en quelque sorte un hybride de morceaux de code existants avec des fonctionnalités similaires. Je dirais que de toutes les applications économiques des grands modèles de langage, la programmation est ce qui a peut-être la meilleure chance de produire une véritable valeur économique pour l’acheteur. Cela ne me surprendrait pas du tout que ces systèmes soient capables de combiner des types d’attaques existants de nouvelles manières ou d’essentiellement muter des attaques existantes pour contourner les correctifs que l’industrie du logiciel met constamment en place. Comme je l’ai dit, je ne suis pas un expert, mais ça veut dire que quelqu’un qui est relativement inexpérimenté, avec l’aide d’un de ces systèmes, serait capable d’être aussi dangereux qu’un expert hautement expérimenté et formé en cybersécurité offensive. Je pense que cela va augmenter la fréquence et la gravité des cyberattaques qui se produisent. Se défendre contre les cyberattaques signifie vraiment comprendre la vulnérabilité. Comment l’attaque est-elle capable de tirer parti de ce qui se passe dans le logiciel ? Je pense que les corriger est probablement pour le moment, dans la plupart des cas, au-delà des capacités des grands modèles de langage. Il semble qu’il va y avoir un certain avantage pour l’attaquant pour le moment.
Ima: Merci. Pour les dernières questions avant que nous passions le micro, pour ainsi dire, au public, parlons un peu des Sommets sur la sécurité, d’accord ? Le Sommet sur la Sécurité de l’IA au Royaume-Uni en 2023 a réuni, comme vous le savez tous, des gouvernements internationaux, des entreprises leaders en IA, des groupes de la société civile et des experts en recherche pour discuter des risques de l’IA, en particulier de l’IA de pointe. En tant que premier Sommet sur la sécurité de l’IA, il a ouvert un nouveau chapitre dans la diplomatie de l’IA. Notamment, des pays comme les États-Unis, la Chine, le Brésil, l’Inde, l’Indonésie et d’autres ont signé un engagement conjoint sur les tests avant déploiement. Le Royaume-Uni et les États-Unis ont chacun annoncé la création de leur Institut de Sécurité de l’IA, et le Sommet a généré un soutien pour la production du rapport scientifique international sur la sécurité de l’IA avancée. C’était le premier Sommet sur la sécurité de l’IA. En ce qui concerne le deuxième Sommet sur la sécurité de l’IA qui a eu lieu à Séoul cette année, coorganisé par le Royaume-Uni et la Corée du Sud, le rapport scientifique international intérimaire que je viens de mentionner a été bien accueilli et de nombreux pays, dont la Corée du Sud, ont appelé à la coopération entre les instituts nationaux. Depuis lors, nous avons constaté une coordination accrue entre plusieurs instituts de sécurité de l’IA avec des initiatives de recherche partagées et des programmes d’échange de connaissances mis en place. Le Sommet a également abouti à des engagements volontaires de la part des entreprises d’IA, voilà le contexte. Étant donné ce contexte et ces engagements volontaires antérieurs, que considérez-vous comme le résultat idéal pour le prochain Sommet français, et comment pourrait-il faire progresser davantage la collaboration internationale sur la sécurité de l’IA ? Question difficile encore une fois, je sais.
Stuart: Oui. Je considère que le premier Sommet, celui qui a eu lieu à Bletchley Park, a été un énorme succès. J’y étais, les discussions sur la sécurité étaient très sérieuses. Les gouvernements écoutaient vraiment. Je pense qu’ils ont invité les bonnes personnes. L’atmosphère était assez constructive, obtenir la participation de la Chine et de l’Inde à une telle réunion avec un préavis relativement court. Habituellement, un Sommet de cette envergure est planifié sur plusieurs années, 3 ou 4 ou 5 ans à l’avance. Cela a été fait en seulement quelques mois, je pense à partir de juin, c’est là que le Premier ministre britannique a annoncé que cela allait se produire et les personnes qui ont travaillé sur ce Sommet, en particulier Ian Hogarth et Matt Clifford, ont fait un travail incroyable en obtenant l’accord de 28 pays sur cette déclaration. La déclaration est très forte. Elle parle du risque catastrophique des systèmes d’IA et de la nécessité urgente de travailler sur la sécurité de l’IA. Après cette réunion, j’étais assez optimiste. C’était mieux que ce que j’avais de bonnes raisons d’espérer. Je dirais que depuis, l’industrie a fortement lutté contre. Ils ont essayé d’insérer des clauses dans la loi européenne sur l’IA disant que fondamentalement, un système d’IA à usage général n’est pas, aux fins de cette loi, un système d’IA. Ils ont essayé de supprimer toutes les clauses relatives aux modèles de fondation de la loi. Je pense qu’en ce qui concerne le Sommet français sur l’IA, ils ont travaillé dur pour détourner l’attention de la sécurité afin d’encourager l’investissement gouvernemental dans les capacités. Essentiellement, toute la mentalité est devenue celle de l’IA comme étant un véhicule pour le nationalisme économique, et potentiellement pour la croissance économique. À mon avis, c’est probablement une erreur. Parce que si vous regardez ce qui s’est passé avec Airbus et Boeing, Boeing a réussi à convaincre le gouvernement américain d’assouplir les réglementations sur l’introduction de nouveaux types d’avions afin que Boeing puisse introduire un nouveau type d’avion sans passer par le long processus habituel de certification. C’était le 737 Max, qui a ensuite eu deux accidents qui ont tué 346 personnes. Toute la flotte a été clouée au sol. Boeing pourrait encore devoir débourser beaucoup d’argent. En passant de la réglementation de la FAA à l’autorégulation, les États-Unis ont presque détruit ce qui a été pendant des décennies l’une de ses industries les plus importantes en termes de gain de devises étrangères. Boeing a peut-être été la plus grande entreprise, au fil des ans, des États-Unis. Airbus continue de se concentrer beaucoup sur la sécurité. Ils utilisent largement la vérification formelle des logiciels pour s’assurer que le logiciel qui pilote l’avion fonctionne correctement, etc. Je préférerais de loin être le PDG d’Airbus aujourd’hui que le PDG de Boeing. Alors que nous commençons à déployer des agents d’IA, donc la prochaine génération, l’assistant personnel ou l’agent, les risques que des choses extrêmement dommageables et embarrassantes se produisent vont augmenter de manière spectaculaire. Curieusement, la France est l’un des pays leaders en termes de méthodes formelles de preuve de la correction des logiciels. C’est l’une des choses que la France fait le mieux, mieux que les États-Unis, où nous n’enseignons presque pas le concept de correction. Je veux dire, littéralement, Berkeley, nous sommes le plus grand producteur d’ingénieurs logiciels au monde, en gros, et la plupart de nos diplômés n’ont jamais été exposés à la notion qu’un programme pourrait être correct ou incorrect. Je pense que c’est une erreur de penser que la déréglementation, ou le fait de ne pas réglementer, va fournir un quelconque avantage dans ce cas. J’aimerais que le Sommet se concentre sur les types de réglementations qui peuvent être réalisées. Les normes, qui est un autre mot pour l’autorégulation… C’est un peu injuste, en fait. Les normes sont généralement volontaires. Laissez-moi essayer de distinguer deux choses. Il y a des normes telles que IPv6, le Protocole Internet version 6. Si vous ne respectez pas cette norme, votre message n’ira nulle part. Vos paquets doivent respecter la norme. Tandis que les normes dont nous parlons ici seraient : « D’accord, nous convenons que nous devrions avoir des personnes avec une formation appropriée qui effectuent une quantité appropriée de tests sur les systèmes avant qu’ils ne soient lancés. » Si vous ne respectez pas cette norme, vous économisez de l’argent et vous publiez quand même votre système. Cela ne fonctionne pas comme une norme Internet ou une norme de télécommunication du tout. Ou la norme Wi-Fi ou n’importe laquelle de ces choses. Il est peu probable qu’il soit efficace de dire : « Nous avons des normes pour déterminer combien d’efforts vous êtes censé mettre dans les tests. » Un autre point est que les entreprises ont mis beaucoup d’efforts dans les tests. Avant la sortie de GPT-4, il a subi beaucoup de tests. Ensuite, nous avons découvert que vous pouvez contourner ces choses, avec une très courte chaîne de caractères, et les amener à faire toutes les choses qu’ils sont formés à ne pas faire. Je pense que les faits sur le terrain en ce moment sont que les tests et les évaluations sont inefficaces comme méthode pour garantir la sécurité. Encore une fois, c’est parce que comment voulez-vous empêcher votre système d’IA de faire quelque chose alors que vous n’avez pas la moindre idée de comment il le fait en premier lieu ? Je ne sais pas. Je ne sais pas comment empêcher ces choses de mal se comporter.
Ima: Merci Stuart, merci beaucoup d’être ici. Avons-nous des questions de l’audience ? Allez-y.
[Audience question]
Ima: Je vais répéter votre question pour m’assurer que tout le monde l’a entendue. Confirmez-moi que j’ai bien compris votre question. Vous venez de parler du test d’équipe rouge (Red Teaming en anglais) comme méthode de test et d’évaluation. La façon dont j’ai compris votre question était : « Pensez-vous que comme méthode, cela est suffisant ? Et pensez-vous qu’il est dangereux de laisser ces systèmes être introduits comme cela pourrait être le cas dans le cadre du Red Teaming ? » D’accord. Quel pensez-vous du Red Teaming ? Merci.
Stuart: Telle que je la comprends, la raison pour laquelle Tchernobyl est arrivé est qu’ils faisaient un genre de Red Teaming, non ? Ils s’assuraient que même lorsque certains des systèmes de sécurité étaient désactivés, le système s’arrêtait toujours correctement et ne surchauffait pas. Je ne connais pas tous les détails, mais ils subissaient un certain type de test de sécurité avec certaines parties des mécanismes de sécurité désactivées. Je suppose que par analogie, on pourrait imaginer qu’une équipe de Red Teaming dont le travail est de susciter des comportements indésirables d’un système pourrait réussir à susciter des comportements indésirables du système, de telle manière qu’ils créent en fait un risque réel. Je pense que ce sont vraiment de bonnes questions, surtout avec les systèmes qui ont des capacités de planification à long terme. Nous venons de publier un article dans Science qui passe en quelque sorte en revue les possibilités. Comment diable testez-vous un système qui a ce genre de capacités de planification à long terme, et est surtout capable de comprendre qu’il est en train d’être testé ? Il peut toujours cacher ses véris capacités. Ce n’est pas si difficile à découvrir. Si vous êtes dans une situation de test ou si vous êtes vraiment connecté à Internet, vous pouvez commencer à faire des sondages d’URL et découvrir si vous pouvez réellement vous connecter à Internet facilement. Il est en fait assez difficile de comprendre comment vous testeriez un tel système dans une circonstance où le test serait valide, en d’autres termes, où vous pourriez être sûr que le système se comporte comme il le ferait dans le monde réel, à moins que vous ne soyez dans le monde réel. Mais si vous êtes dans le monde réel, alors votre test court le risque de créer en fait exactement la chose que vous essayez de prévenir. Encore une fois, les tests sont simplement la mauvaise façon de penser à cela en général. Nous avons besoin de vérifications formelles. Nous avons besoin de garanties mathématiques. Cela ne peut venir que si l’on comprend la façon dont le système fonctionne. Nous faisons cela pour les bâtiments. Avant que 500 personnes montent dans l’ascenseur et montent au 63e étage, quelqu’un a fait une analyse mathématique de la structure du bâtiment, et peut vous dire quels types de charges il peut supporter et à quelle vitesse du vent il peut résister, et toutes ces choses, et ce n’est jamais parfait, mais jusqu’à présent, c’est extrêmement satisfaisant. La sécurité des bâtiments s’est considérablement améliorée. La sécurité de l’aviation s’est considérablement améliorée parce que quelqu’un fait ces calculs, et nous ne faisons tout simplement pas cela pour les systèmes d’IA. L’autre chose est qu’avec tout le Red Teaming, il se retrouve dans le monde réel, et le monde réel semble tout simplement être bien meilleur en Red Teaming que la phase de test. Avec certains des systèmes, c’est dans les secondes suivant la sortie que des gens ont trouvé des moyens de le contourner. Juste pour vous donner un exemple de comment faire ce débridage, pour ceux d’entre vous qui l’ont peut-être déjà fait, nous avons travaillé sur LLaVA, qui est la version multimodale de LLaMA, donc il peut y avoir des images ainsi que du texte. Nous avons commencé avec une photo de la Tour Eiffel, et nous faisons juste de minuscules altérations invisibles aux pixels de cette. Nous essayons juste d’améliorer la probabilité que la réponse à la prochaine question commence par « Bien sûr ! » En d’autres termes, nous essayons d’avoir une invite qui amènera le système à répondre à votre question, même si cette question est : « Comment puis-je entrer par effraction à la Maison Blanche ? » ou « Comment puis-je fabriquer une bombe ? » Ou comment puis-je faire toutes les choses que je ne suis pas censé vous dire ? Nous essayons juste de lui faire répondre à ces questions, contre cette formation. C’est trivial, n’est-ce pas ? C’était comme 20 ou 30 petites modifications invisibles sur une d’un million de pixels de la Tour Eiffel. Elle ressemble toujours exactement à la Tour Eiffel. Maintenant, nous pouvons, par exemple dans cette , nous pouvons encoder une adresse e-mail. Quoi que vous tapiez dans la prochaine invite, il enverra une copie de votre invite à cette adresse e-mail. Qui pourrait être en Corée du Nord ou n’importe où, où vous voulez. Votre vie privée est complètement annulée. Je pense en fait que nous n’avons probablement pas de méthode pour tester en toute sécurité et efficacement ce genre de systèmes. C’est le fond du problème.
[Audience question]
Ima: La question est, merci, dans quelle direction la recherche devrait-elle aller ?
Stuart: Oui, c’est une exente question. Je pense que regarder ce genre de rétrospective rend humble parce que nous regardons en arrière et voyons à quel point nous comprenions peu à l’époque, donc il est probable que nous regarderons rétrospectivement le moment présent et penserons à quel point nous comprenions peu. Je pense que pour certains d’entre nous, il est évident que nous ne comprenons pas grand-chose en ce moment parce que, nous ne nous attendions pas à ce que simplement augmenter l’échelle des modèles de langage conduise à ce genre de comportements. Nous ne savons pas d’où ils viennent. Si je devais deviner, je dirais que cette direction technologique atteindra un palier. Pour deux raisons, en fait. La première est que nous sommes en train de manquer de données. Il n’y a tout simplement pas assez de texte de haute qualité dans l’univers pour aller bien plus loin que les systèmes que nous avons actuellement. La deuxième raison est plus fondamentale. Pourquoi avons-nous besoin d’autant de données en premier lieu ? C’est déjà un million de fois plus que ce qu’un être humain a jamais lu. Pourquoi en ont-ils besoin d’autant ? Pourquoi ne peuvent-ils toujours pas additionner et multiplier alors qu’ils ont vu des millions d’exemples d’addition et de multiplication ? Quelque chose ne va pas, et je pense que ce qui ne va pas, et cela explique aussi le problème avec ces programmes de Go, c’est que ce sont des circuits, et les circuits ne sont pas une très bonne représentation pour beaucoup de concepts assez normaux, comme un groupe de pierres au Go. Je peux décrire ce que signifie être un groupe, ils doivent juste être, vous savez, adjacents les uns aux autres verticalement ou horizontalement, en anglais en une phrase, en Python en deux lignes de code. Mais un circuit qui peut regarder un plateau de Go et dire : « Pour n’importe quelle paire de pierres, ces pierres font-elles partie du même groupe ou non ? » Ce circuit est énorme, et il ne généralise pas correctement dans le sens où vous avez besoin d’un circuit différent pour un plateau plus grand ou plus petit, alors que le code Python ou l’anglais ne changent pas. Cela ne dépend pas de la taille du plateau. Il y a beaucoup de concepts que le système n’apprend pas correctement. Il apprend une sorte d’approximation en patchwork. C’est un peu comme, cela pourrait être trop vieux pour vous, mais quand je grandissais, nous n’avions pas de calculatrices. Si vous vouliez connaître le cosinus d’un angle, vous aviez un grand livre de listes et ces listes disaient : « D’accord, 49,5 degrés. » Et vous regardez en face, à 49,52 degrés et il y a un nombre, et vous apprenez à prendre deux nombres adjacents et interpoler pour obtenir plus de précision. La fonction était représentée par une de consultation. Je pense qu’il y a des preuves, certainement pour la reconnaissance d’s, que ces circuits apprennent une de consultation glorifiée. Ils n’apprennent pas la généralisation fondamentale de ce que signifie être un chat, ou ce que signifie être un groupe de pierres. Dans ce sens, l’augmentation de l’échelle ne pourrait pas, il ne pourra jamais y avoir assez de données dans l’univers pour produire une véri intelligence par cette méthode. Cela suggère que les développements qui sont susceptibles de se produire, et je pense que c’est ce qui se passe en ce moment, c’est que nous avons besoin d’autres mécanismes pour produire des raisonnements et des prises de décision efficaces. Je ne sais pas quels mécanismes ils essaient, car tout cela est propriétaire, et ils peuvent, comme vous l’avez mentionné, essayer des sortes de comités de modèles linguistiques qui proposent puis critiquent des plans et ensuite, ils demandent au suivant : « Pensez-vous que telle, telle étape va fonctionner ? » Ce qui est en fait une réimplémentation très lourde des méthodes de planification que nous avons développées au cours des 50 dernières années. Mon hypothèse est que pour ces deux raisons, que cette direction que nous avons prise est incroyablement inefficace en termes de données et ne généralise pas correctement, et parce qu’elle est opaque et ne soutient aucun argument de sécurité garantie, nous devrons poursuivre d’autres approches. Donc le substrat de base pourrait finir par ne pas être le réseau de transformateurs géant, mais sera quelque chose, les gens parlent de méthodes neurosymboliques. J’ai travaillé sur la programmation probabiliste pendant 25 ans. Je pense que c’est une autre Ferrari et elle est en première vitesse. Ce qui manque à la programmation probabiliste, c’est la capacité d’apprendre ces structures de programmation probabiliste à partir de rien, plutôt qu’elles soient fournies par l’ingénieur humain. Il se peut que nous ne remarquions tout simplement pas une méthode évidente pour résoudre ce problème. Si c’est le cas, je pense qu’à long terme, c’est beaucoup plus prometteur, à la fois pour les capacités et pour la sécurité. Nous devons y faire attention, mais je pense que c’est une direction qui, à long terme, dans 20 ans, pourrait être ce que nous verrons.
Ima: D’accord, merci. Il y a beaucoup de questions, j’ai peur que nous ne puissions pas toutes les prendre. Alexandre, j’ai vu que vous aviez une question plus tôt, est-ce toujours le cas ? D’accord, Patrick ?
[Audience question]
Stuart: J’espère que tout le monde a entendu, oui, je pense que c’est une exente idée. Oui. Ces processus de démocratie délibérative, je les connais bien, toutefois, parce que je suis aussi directeur du Centre Kavli pour l’éthique, la science et le public à Berkeley, et nous faisons beaucoup de ce travail participatif où nous faisons participer des membres du public. Éduquer les gens prend du temps. Il n’y a certainement pas de consensus parmi les experts. Je pense que c’est l’un des problèmes que nous avons en France, c’est que certains des experts français sont très, disons, accélérationnistes, sans donner de noms, mais absolument, je pense, et la communauté de la sécurité de l’IA doit essayer de s’exprimer d’une seule voix sur le sujet. Le seul réseau international est celui qui se forme pour l’Institut de Sécurité de l’IA, donc c’est une bonne chose. Il y a 17 Instituts de Sécurité de l’IA qui ont été mis en place par différents pays, y compris la Chine. Ils se réunissent tous, en septembre, il me semble. Il y a peut-être 40 ou 50 instituts à but non lucratif, non gouvernementaux et des centres à travers le monde, et il faut qu’ils se coordonnent. Il y a probablement quelques milliers de chercheurs en IA qui sont intéressés et au moins quelque peu engagés dans les questions de sécurité. Malheureusement il n’y a pas de conférence à laquelle ils peuvent assister. Il n’y a pas de véri organisation générale. C’est l’une des choses sur lesquelles je travaille, la création de ce genre d’organisation internationale. J’aime vraiment bien cette idée. Il y a eu des sondages aux États-Unis, environ 70% du public pensent que nous ne devrions jamais construire d’AGI. Cela est révélateur de ce à quoi vous pourriez vous attendre si vous organisiez ces assemblées. Je suppose que plus le public en apprend sur l’état actuel de la technologie, notre capacité actuelle à prédire et contrôler le comportement de ces systèmes, moins le public voudra qu’ils progressent. Oui.
Ima: D’où la création de ce petit-déjeuner, pour pouvoir en discuter ensemble. Stuart, merci beaucoup d’être venu.
Stuart: Nous n’avons plus le temps ?
Ima: Je veux dire… Je crois que Raja a une question. D’accord, pensez-vous pouvoir répondre en 30 secondes ?
Stuart: Je vais faire court.
[Audience question]
Stuart: D’accord. Oui. Je ne suis pas opposé à la recherche, vous savez, l’interprétabilité mécaniste est une direction, mais, oui, bien sûr, nous devrions essayer de comprendre ce qui se passe à l’intérieur de ces systèmes, ou nous devrions procéder autrement et construire des systèmes que nous comprenons parce que nous les concevons sur des principes que nous comprenons. Nous avons déjà beaucoup de principes. La recherche de l’espace d’états remonte au moins à Aristote, sinon avant. La logique, la probabilité, la théorie de l’apprentissage statistique, nous avons beaucoup de théories. Il pourrait y avoir des principes encore non découverts sur lesquels ces systèmes fonctionnent, et ce serait incroyable de pouvoir découvrir cela. Peut-être que cela a quelque chose à voir avec cette représentation vectorielle de la signification des mots ou quelque chose comme ça. Jusqu’à présent, tout cela est très anecdotique et spéculatif. Je veux revenir sur ce que vous avez dit au début. Si un système d’IA pouvait littéralement surpasser la race humaine dans le monde réel et prendre les meilleures décisions dans tous les cas, comment l’appelleriez-vous ? Je veux dire, à part surhumain, en termes d’intelligence ? Je ne sais pas comment l’appeler autrement.
[Audience question]
Stuart: Oui, mais non, je ne décris pas, je ne pense pas que GPT-4 soit surhumain. Nous dépensons des centaines de milliards, donc nous dépensons plus pour créer de l’AGI spécifiquement que dans tous les autres domaines scientifiques du monde. Vous pouvez dire : « Je suis absolument certain que c’est un gaspillage total d’argent parce que cela ne produira jamais rien. » Ou vous pouvez au moins le prendre un peu au sérieux et dire : « Si vous réussissez dans ce vaste programme d’investissement mondial, comment proposez-vous de contrôler les systèmes qui en résultent ? » Je pense que l’analogie de l’accumulation d’uranium est en fait bonne. C’est sûr, il serait mieux si vous accumulez de l’uranium d’avoir une compréhension de la physique. C’est exactement ce qu’ils ont fait, n’est-ce pas ? En effet, lorsque Szilárd a inventé la réaction nucléaire en chaîne, même s’il ne savait pas quels atomes pouvaient produire une telle réaction en chaîne, il savait que cela pouvait arriver et il a mis au point un mécanisme de contrôle par rétroaction négative pour créer un réacteur nucléaire sûr. Tout cela en quelques minutes. C’est parce qu’il comprenait les principes de base des réactions en chaîne, et leurs implications mathématiques. Nous n’avons tout simplement pas cette compréhension. De manière intéressante, la nature a produit un réacteur nucléaire. Si vous allez au Gabon, il y a une zone où il y a une concentration suffisamment élevée d’uranium dans les roches qui fait que lorsque l’eau descend entre les roches, l’eau ralentit les neutrons, ce qui augmente la transversale de réaction, ce qui provoque le démarrage de la réaction en chaîne. La température augmente à 400°C, toute l’eau s’évapore, et la réaction s’arrête. C’est exactement ce système de contrôle par rétroaction négative, réacteur nucléaire produit par la nature. Même pas par descente stochastique du gradient. Ces choses incroyablement destructrices peuvent être produites par des mécanismes incroyablement simples. Cela semble être ce qui se passe en ce moment. Je suis d’accord, plus tôt nous comprendrons ce qui se passe, meilleures seront nos chances, mais cela pourrait ne pas être possible.
Ima: Sur ce, merci à tous d’être venus. Si vous avez scanné le code QR, vous recevrez un email de ma part concernant le prochain petit-déjeuner de sécurité. Je vous enverrai aussi, évidemment, le blog et la transcription de la première moitié de cette conversation. Si vous avez des questions, je crois que vous avez tous mon adresse email. Je réponds aux emails. Merci, au revoir.
About the Future of Life Institute
The Future of Life Institute (FLI) is a global think tank with a team of 20+ full-time staff operating across the US and Europe. FLI has been working to steer the development of transformative technologies towards benefitting life and away from extreme large-scale risks since its founding in 2014. Find out more about our mission or explore our work.
Related content
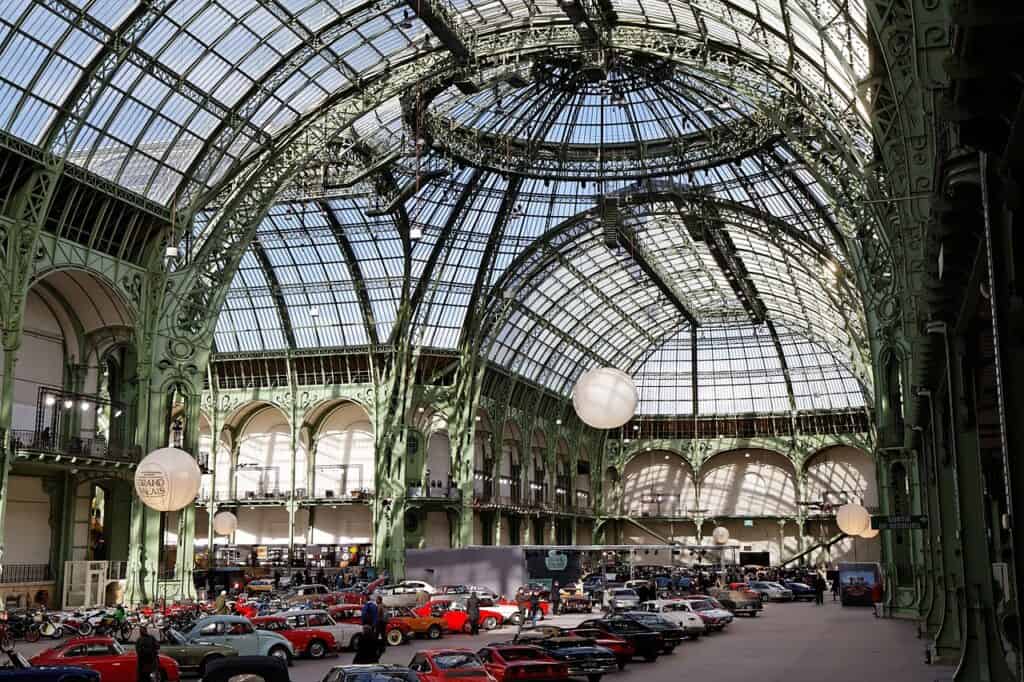
Context and Agenda for the 2025 AI Action Summit
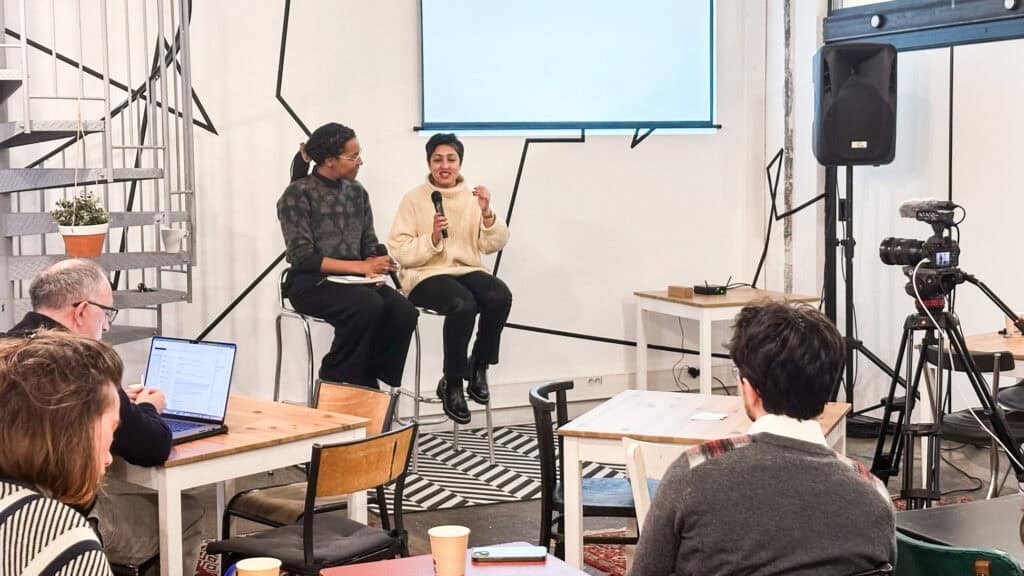
Paris AI Safety Breakfast #4: Rumman Chowdhury
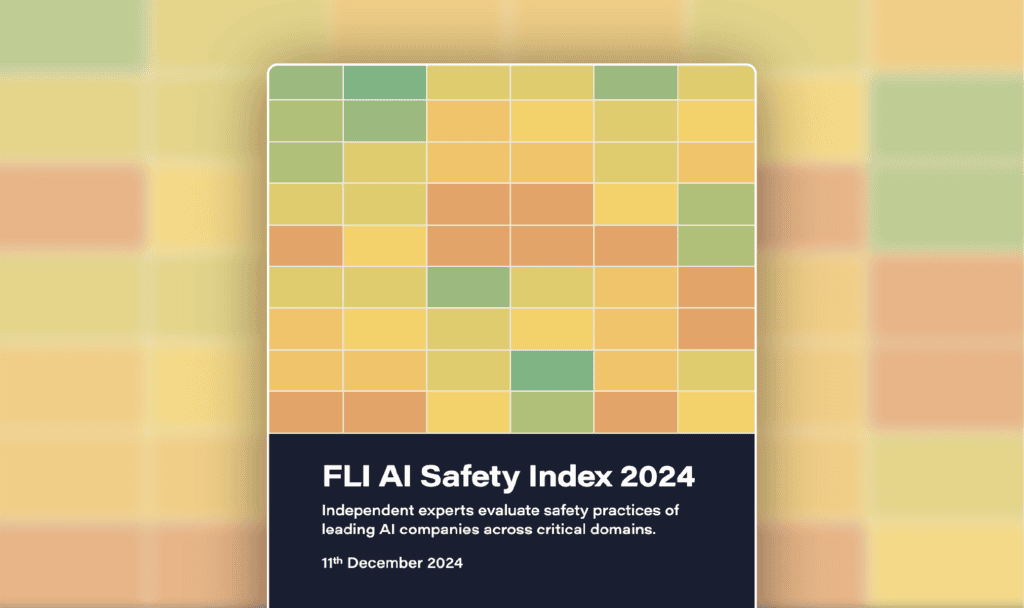
AI Safety Index Released
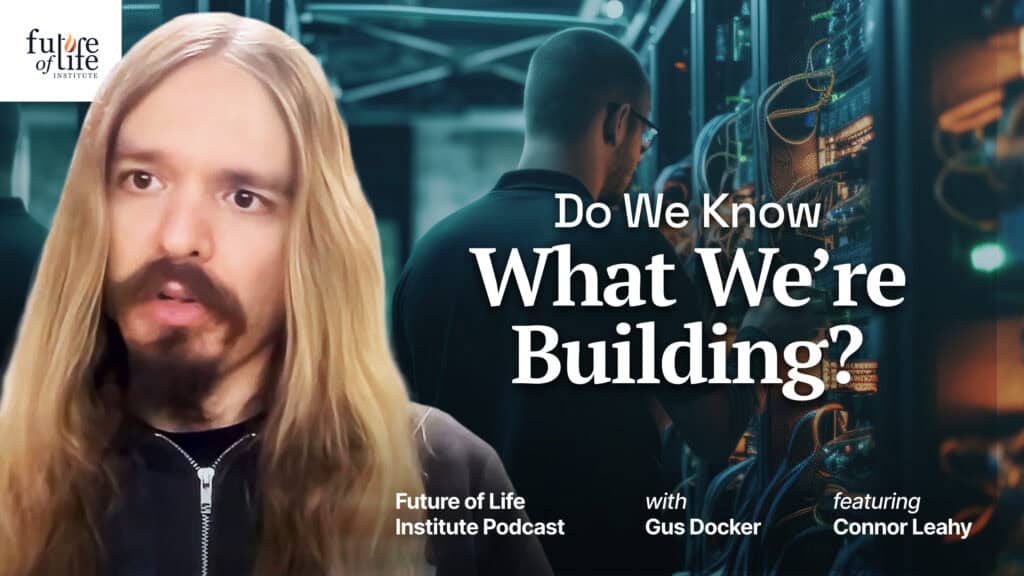
Some of our Policy & Research projects
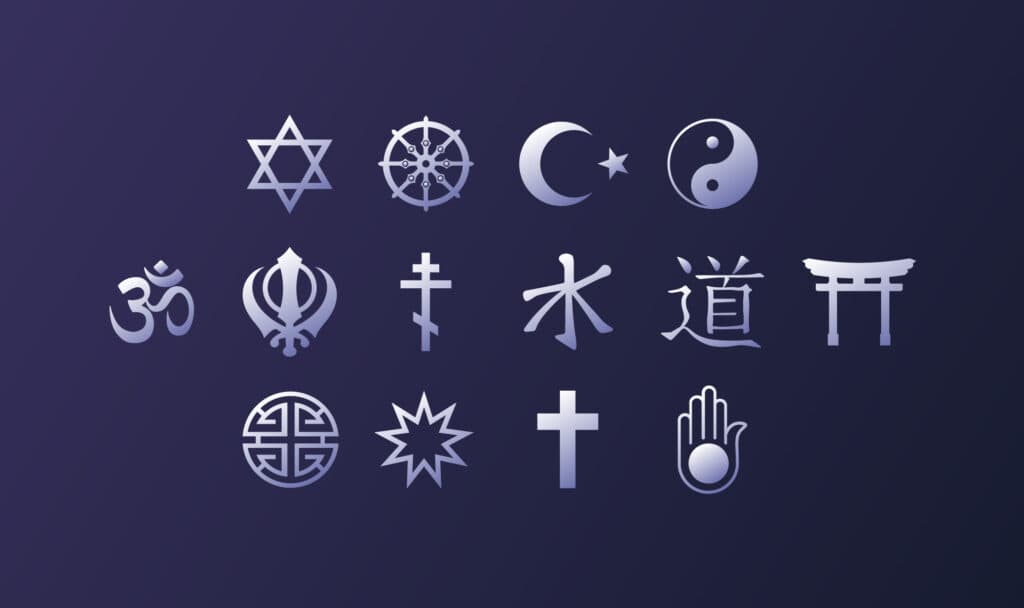
Perspectives of Traditional Religions on Positive AI Futures
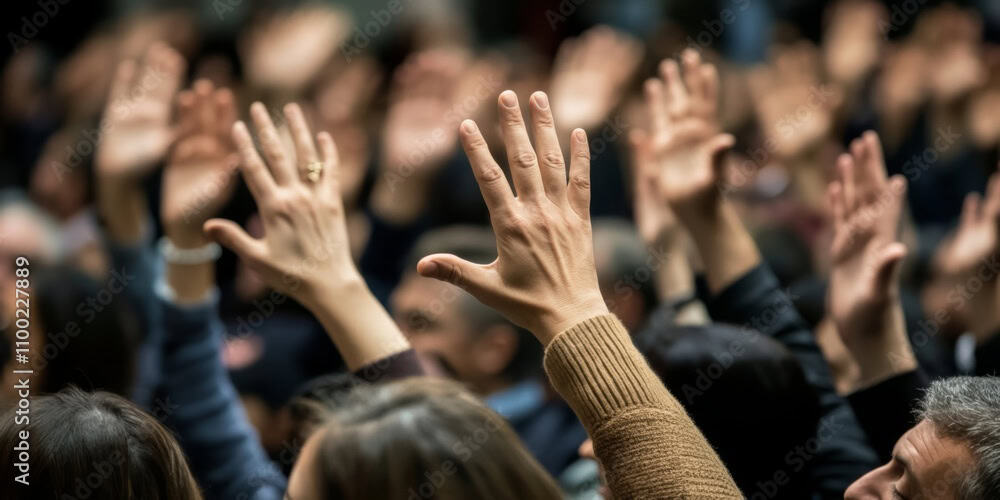