Transcript: Concrete Problems in AI Safety with Dario Amodei and Seth Baum
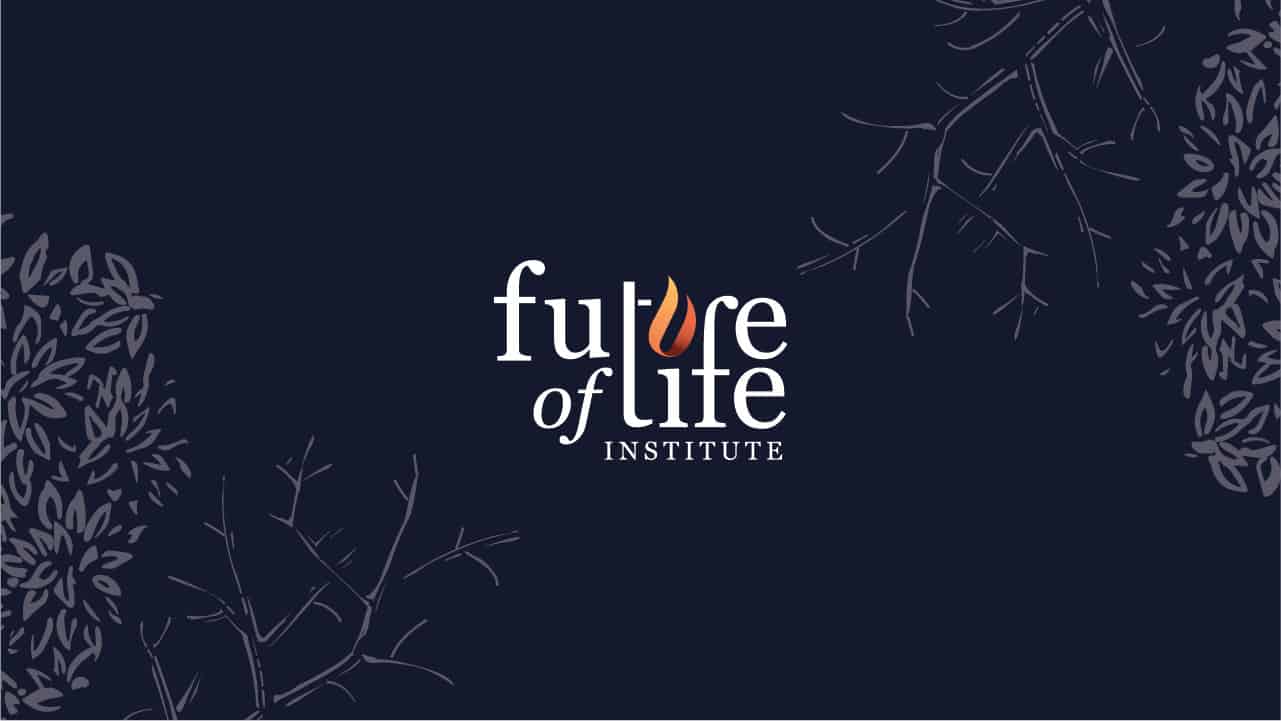
Contents
Ariel Conn: From the FLI Audio Files. I’m Ariel Conn with the Future of Life Institute.
This summer I watched all four of the White House Symposia on AI Research, which were designed to give AI researchers and others in the field a chance to discuss what the status of AI research is now, how it can help move society forward, and what risks we need to figure out how to address. There were many, many hours of talks and discussions, and I couldn’t help but notice how often researchers got up and were very adamant that talks should only be about short-term AI issues. They insisted that long-term AI concerns weren’t something that needed to be addressed right now. They didn’t want to worry about advanced AI, and they definitely didn’t want to think about superintelligence. But then, as soon as they started talking about their research, many of the issues that came up were related to things like control, and transparency, and bias.
Now, these are obviously issues that need to be addressed for short-term narrow AI, but there are also issues that need to be addressed for long-term, more advanced AI. And so I started to wonder why we were so worried about focusing on short-term vs. long-term artificial intelligence. Now somewhat famously in the AI world at least, Andrew Ng—who is with Baidu—has compared worrying about artificial long-term intelligence issues to worrying about overpopulation on Mars.
And I guess my reaction is that overpopulation is an issue, and it’s an issue that we need to address, and if we can solve overpopulation issues now so that we don’t have to worry about them later… why would we not do that? I realize there are probably some cost issues or other reasons that planning ahead is more difficult, but it seems like a really strange stance to me that we shouldn’t try to solve a current problem now, so that it doesn’t crop up again in the future.
I figured the best way to try to understand what’s happening would be to turn to two people in the field. So I have with me Dario Amodei—who had been working at Google Brain, where he published a well-received paper titled Concrete Problems in AI Safety—and he’s recently moved to Open AI, which is focused on AI research and safety issues, as well as understanding the social implications of AI development. I also have Seth Baum—Executive Director of the Global Catastrophic Risk Institute. Much of Seth’s own research is on insuring AI safety. Seth, I’ll turn this over to you now.
Seth Baum: Thanks Ariel. And these are really good questions, and we saw it at the White House Symposia. I attended one of those, but we also see it in a lot of different conversations across the AI world. And I think it’s a really important one, because while we might have some reasons for being especially interested in or concerned about the long-term AI issues, at the same time a lot of people are just more focused on short-term questions, and so it’s fair to ask what we can do to get action that works for both long-term and short-term AI issues. That’s why I think it’s good to have this conversation. It’s especially good to be having this conversation with Dario Amodei, who has recently published a paper on this topic, or rather, on the topic of AI safety in general and the issues that come up in AI safety, whether it’s short-term or long-term safety. Now, Dario, I want to get to the paper in a second, but first just a little background for our listeners: maybe you could say a little bit about how you became interested in the AI safety topic in the first place?
Dario Amodei: Sure. I’ve actually been working in the AI field itself for a couple of years, and before coming to open AI I worked both at Baidu and Google, and I was drawn to the field by what I see as the incredible advances—particularly in deep neural networks—to solve problems in vision, speech, and language. I was involved in research in all of these areas, and found it very exciting, but one thing I definitely noticed—particularly with deep neural networks and with powerful ML systems in general—is that there are many ways they can be very accurate. A speech recognizer can tell you something and it can be almost as good as a human, but they can also be somewhat brittle. If I train with speech data on human speaking with a clean background with unaccented American speakers, they’ll perform great. But then if I test the same on accented speech or noisy data, it performs terribly.
And as the systems get deployed more into the world, having systems that fail unpredictably is not a good thing. And I think that impression was reinforced as I continued my work at Google, where you have issues with Google photo systems—which was based on a neural net classifier—that ended up accidentally classifying people of color as gorillas, which of course is an incredibly offensive thing to do. Now the neural net didn’t know that it was offensive, it was a combination of a problem with the classifier and a problem with the training data, but that machines can lack context for something and the classifier that’s produced by the machines can be something that has very bad real world impacts. If it does something that is not what we intended for it to do, that can be very harmful.
I think in the last year I have become particularly interested in reinforcement learning, which is a branch of machinery that’s concerned with interacting with the environment in a more intertwined way and is often used in things like robotics, self-driving cars, and autonomous systems. We’ve seen there was a recent announcement from Google that it’s used to control the power in their data centers. So once you’re actually interfacing with the world directly and controlling direct physical things, I think the potential for things to go wrong—which often I think are quite mundane—starts to increase. So I became more and more interested in if there were principle ways to reduce the risk or find some theoretical basis for guaranteeing that something bad won’t happen as we start to deploy these systems more into the world.
That’s kind of where my interest in AI safety started, and certainly there is the thought that these systems are advancing very quickly, and the more powerful they get the higher the stakes are for something that might potentially go wrong. So as someone who really wants to think about the social impacts of what I’m working on, this seemed like a really important area.
Seth Baum: That makes a lot of sense, and it does seem that if you look at the systems themselves and what they’re doing, then it naturally follows that they can fail in these types of ways. And we saw it with the Google gorilla incident, which is kind of a classic example of, as you put it, an AI system failing predictably. A lot of my research is on the risk and especially the policy end of AI, and the same issue comes up in that context, because who do you hold liable? Do you really hold liable the company or the computer programmers who built this code? Because they didn’t want to do that! So for the legal management of these types of software it’s a challenge, because you don’t want to hold these people liable for intentionally causing these harms, yet at the same time these are systems that are almost by design bound to behave in unpredictable ways. And we’re just going to see this happen more and more. My impression at least is that that is a little part of the motivation for your paper. You wrote a paper recently called Concrete Problems in AI Safety that seems to take on some of these topics. Maybe you could say a little more about the paper itself and how you wanted to write it. How it contributes to these issues.
Dario Amodei: Yeah, so as I got more and more interested in these safety issues, I did what any researcher does and looked into the literature that had been written in the machine learning literature so far about these problems. And there actually was a fair amount of literature about various subsets of this sort of thing that I was worried about… maybe there wasn’t a substantial literature, but there was, I would say, kind of a staggered nature to it, where we get into some of the specific problems that I talk about in the paper. But the four or five different problems that I was kind of thinking in my head—of ways to classify things that could go wrong in an ML system—were often written in parts of the literature that were not very much related to one another, and often were very specific to particular applications.
So I felt that what would help a lot is something that is sort of a combination of reviewing all the existing work that has been done in one place, and also having an agenda that talks about what needs to be done in the future. In particular, a lot of this work had been done quite a while ago, and so really writing this review and agenda with view towards the cutting-edge ways in which machine learning has advanced in the last three or four years with neural nets doing vision, speech, language, game playing, autonomous driving, and a bunch of other applications. I felt like a lot of the thinking about making systems safe, controllable, robust, and predictable could really use an update in light of these advances.
Then there was another stream, where for a while I’d been aware of the work of people like Nick Bostrom and Eliezer Yudkowsky, who come from outside the ML field, and have been warning about these very long-term considerations involving AIs that are smarter than humans. I read their work, and my attitude towards it has always been that I found it quite thought provoking, but if as a researcher I wanted to think about ways in which machine learning systems can go wrong, it is very important that we—for now—stick to the kind of scenarios that have been built today. Then if future scenarios do come up, we’re better equipped to think about those scenarios. So between those two poles, that there was a lot of existing literature that I felt needed to be drawn together a little bit, and that there was more of the high-level, kind of visionary thinking about the far future, I felt that there was a middle space of thinking in a principle but much more concrete way about the systems that we’re building now or likely to build in the next few years, and what general lessons can we learn about how ML systems go wrong.
That was the thought with which we sat down and wrote the paper, and it turned out that it was not just me, but there were several other authors in the paper. My main co-author was Christopher Olah—also from the Google Brain team—who’s done a lot of work on visualization and blogs for teaching machine learning to a wide audience. And we had some collaborators from Berkeley, Paul Christiano from Stanford, Jacob Steinhardt from Open AI before I joined, John Shulman, and another Google Brain researcher Dan Mane. We all found that we have the same general perspective and vision on the paper relative to what happened in this space before. We all worked together and spent a bunch of time, and eventually we produced this paper.
Seth Baum: Okay, so perhaps you could take us into the paper a little bit. There are five concrete problems: avoiding negative side effects, avoiding reward hacking, scalable oversight, safe exploration, and robustness to distributional shift. These make sense when you get into the paper, but to just hear them spoken like this is kind of ambiguous. Maybe we can go one at a time through each of these five problems, and you can explain in basic terms what they mean, starting with “avoiding negative side effects.”
Dario Amodei: So actually, before going into the five problems in detail, one frame that I thought of as a little useful for thinking about the problem that we describe in the paper is that we actually split the five problems into three general categories. I’ve always found it useful to start thinking about those categories, and then getting into the problems.
If you’re trying to build a machine learning system, one of the most important pieces is the objective function, which really defines the goal of the system or a way of judging whether it’s doing what you want it to do. For example, if you’re building a system that is supposed to recognize speech, then you might measure what fraction of words it gets correct vs. incorrect—the word error rate. If you’re building an image classification system, the objective function is the fraction of time that it identifies the images in the correct class. If you’re building a system to play Go—like the AlphaGo system—then it’s the fraction of the games you win, the probability that you win a game. So when thinking about building the machine learning system, and for whatever reason the machine behaves in some way that you didn’t intend and don’t like, one of the ways I found useful is to think about exactly where in the process things go wrong. The first place that things go wrong is if your objective function wasn’t actually right. I’ll go into a little more detail on that later, but the idea is that you’re putting pressure on the machine learning system to do a particular thing, and rewarding it for doing a particular thing. Unbeknownst to you, that was actually the wrong thing to do. The system then ends up behaving in a harmful way, because you had in mind that it would behave a certain way, you tried to formalize that with the objective function, and the objective function was the wrong objective function.
The second class is if you do know the objective function, but it’s very expensive to evaluate it. This might be human judgement, or we have a limited budget for a human supervising and checking in on the AI system, and the system has to extrapolate. It might do the wrong thing because it hasn’t really seen what the correct objective function is.
The third thing is when our system has the right objective function, but our machine learning system—as indicated by the phrase “machine learning”— has to learn. And there is a concern that while the system is in the process of learning, when it doesn’t understand the world around it to the best that it can, it might do something harmful while it’s not fully trained. That for me, from the perspective of a researcher, has been a natural way to decompose the problems. So now maybe we can go into the five problems.
Seth Baum: Before we dive into the five problems, let me try speaking these back at you to make sure I understood them correctly. The first one is just the wrong objective function, that is, you gave it goals that in retrospect turn out to be goals that you’re not happy with. It fulfills those goals, but you wish that it was fulfilling some other goals.
Dario Amodei: Correct.
Seth Baum: Now the second one, you said the objective function is expensive to evaluate. That means, you gave it good goals and it is working towards those goals, but it’s struggling, and instead of fulfilling those goals It’s doing something else that’s causing a problem.
Dario Amodei: Right, because it has a limited ability to assess what the right goal is. So, the right goal might be to make the human happy with everything that I’m doing, but I can’t in every little action ask the human if he’s happy with what I’m doing. And so I might need some kind of cheaper proxy that predicts whether a human is happy. And if that goes wrong, then the system could do something unpredictable.
Seth Baum: Okay, then the third one is when problems occur during the training process… maybe you could say what this training process is and how can we get harmed during it?
Dario Amodei: Sure, maybe we’re going into those first two problems as a sub-category, but the general idea is that if you had to think of a child, when they don’t really understand the world around them. Say, if they press this button on the stove a fire will turn on that could burn them or burn someone else. The child might be using the right process to learn about the world, but if they’ve never touched the stove before they don’t know what might happen and they don’t know if they might hurt someone.
Seth Baum: Okay, makes sense to me. Should we go ahead and dive into the five?
Dario Amodei: Yeah, so the first one you asked about was “avoiding negative side effects.” This is one problem under the sub-category of having the wrong objective function. One way to introduce this is to say that in some sense machine learning systems are very literal minded. If you tell them to do X, they’ll do exactly X. The example we give in the paper: let’s say I have a cleaning robot that is trying to move a box from one side of a room to another, and in this very simple example all it tries to do is move the box. I might give it an objective function that basically says, “you get points (a reward) for moving the box from one side to the other,” and that’s all that matters to me. But if you give it literally just that one objective, then actually you’re implicitly telling it that anything else in its environment it doesn’t care about. If a vase is in its path, then it doesn’t in any way in terms of its objective function get penalized for knocking over this vase. It can just walk from one side of the room to the other and may just knock over the vase and not care about it.
You can generalize this to having a robot, and it’s really very focused on accomplishing a particular task—moving a particular thing—but the world is big and humans, when we walk around performing tasks, have to be very sure that when we drive our children to school that we don’t run someone over with the car or do something else. We are never just explicitly doing one task. I’m always doing one task and making sure that the rest of the world is okay, that I don’t do anything really damaging. As a human I have common sense and I know this, but our machinery systems at the present at least are not at the point where they have common sense. And so I see a potential for various things to go wrong in this case.
Seth Baum: That really sounds like the classic Genie story. Right down to the genie in the bottle, you rub it, you get your wish. And it gives you your wish exactly what you asked for whether you like it or not. What you’re saying is that a machine-learning AI system has that same sort of taking things very literally
DS: Or could. At least the systems that we build today often have that property. I mean, I’m hopeful that someday we’ll be able to build systems that have more of a sense of common sense. We talk about possible ways to address this problem, but yeah I would say it is like this Genie problem. For this specific case, one of the specific things that can go wrong is that the world is big and that it’s very easy when you’re training the machine learning system to focus on only a small aspect of it, and that gives you a whole class of things that can go wrong.
Seth Baum: Okay sounds good. Let’s move on to the second one: “avoiding reward hacking.” What’s that all about? So, reward hacking is this kind of situation where you write down an objective function, and it turns out that your objective function was trying to capture the ability to do something hard, or you felt like in order to achieve this objective function, we need to do some hard task, that is, the task you’re trying to get the machine learning system to do. But often there is some way of cheating the objective function that’s been written down. So the example given in the paper is if you have a clean robot that’s kind of trying to clean up all the messes it can see, and you decide to give it an objective function that says, “well how much dirt can you see around you?” You might think that would be a good measure of how clean the environment is. Then the cleaning robot—if it were designed a certain way—could just decide to close its eyes, or it could decide to kind of shovel a bunch of messes under a desk or into a closet.
And in fact this isn’t limited to machine learning systems. It’s a problem we have with humans as well, right? If I hire a cleaner, most cleaners are honest, but you know in theory if I didn’t check and I hired a dishonest cleaner, they might find it easier to just shove all the messages in my house into some closet that they think I won’t look in. And you know, again, there’s this thing that machine learning systems can be literal-minded at least in the way we design them today, and there’s all types of things that can go wrong. In the paper we discuss some general factors that lead to this, and one factor is when the robot with the machine learning system is not able to see everything in its environment, it’s very easy for the wrong kind of objective function to give incentives to hide aspects of the environment from itself for others—like the shoveling things into the closet case. We discuss a few of the general ways this can happen and a few thoughts and recommendations for designing objective functions where this is less likely to happen.
Seth Baum: I’m reminded of those cute pictures you see on the Internet of kids taking quizzes, and it will be something like this long, difficult math problem, and below it says write the answer here and the student just writes the words “the answer” below it and tries to pass it in because they don’t know how to actually do the math problem.
Dario Amodei: Yeah
Seth Baum: We need to get our AI systems to not behave like mischievous little kids.
Dario Amodei: Yeah there was the blogger who wrote about our work—I think it was Cory Doctorow—and he said a lot of the problems that I’ve read in this paper remind me of issues in child development in child psychology, and there is a sense in which a lot of these systems are kind of like savants, right? They’re like small children who don’t have enough common sense to know how do quite the right thing, but at the same time they are very voracious learners who can process a lot of information. So I do see a lot of commonalities there.
Seth Baum: Makes sense. Okay, so let’s move on. The next one is scalable oversight.
Dario Amodei: Yeah so the kind of the example we get there is let’s say that we have a cleaning robot again, and it’s trying to clean up a room but there are some objects in the room which might belong to a human. There’s a surefire way to get the right objective function, which is for every time you find an object, you ask a human. You ask the human, “does this object belong to you?” You ask every human who could possibly own it, “does this object belong to you?” You always do the right thing, but a robot that does that is impractical, and no one would sell any robots to do that. if that’s going to happen I might as well just clean it myself. I don’t want my robot asking me questions every two minutes while it’s trying to clean my house. So can we find ways where the robot maybe only asks me the first two times and gets a good sense of what kind of stuff I would actually own versus what kind of stuff it’s okay to throw away. Maybe it looks at cues of where I leave the stuff. And so the way to state the problem is: if the robot tries to do this, there is the risk that it will throw away things that I really would have wanted. And the solution is: are there ways we can get the robot to think about—from repeated experience—being able to predict the true objective function, which is what really belongs to me and what I really want thrown away without actually having to ask me every time, which might then destroy the economic value of the system.
Seth Baum: That seems like something that humans face all the time, right? You’re cleaning, and do you really know whether they want that thrown away or not? Sometimes it’s a candy wrapper. That should be pretty obvious, unless it’s maybe a candy wrapper with a winning lottery ticket printed on the inside, or some prize competition that the candy company had. But it seems really easy for robots or AI systems to make the same sorts of mistakes.
Dario Amodei: Yeah
Seth Baum: Do you think it’s particularly difficult to train an AI to get those sorts of questions right? That seems like, maybe I don’t know the systems well enough, but that seems like something that we should be able to train an AI to figure out without too much difficulty.
Dario Amodei: In the particular case of the cleaning robot, I’m pretty optimistic. But I think, you know it’s designed more to be kind of a parable that illustrates, that often there are aspects of human preferences that are quite subtle, and getting all of them right without an unacceptable amount of communication with humans, or halting the workflow of the machine learning system can often be quite subtle. A human might be able to look at something like a concert ticket and if the day for the concert ticket was yesterday, then they might just know that it was okay to throw it away. But if it was tomorrow then they would say, “oh, that’s really valuable, this is something someone’s going to use.” So there’s just a lot of subtle things like that that I think take some work to get right.
Seth Baum: Okay, sure. Now let’s move. The next one is “safe exploration.” What’s that?
Dario Amodei: So this is actually a problem that’s been worked on a lot in the machine learning community, and so our work here was more to summarize prior work, and also point towards how work in this area could be integrated with a lot of advances that we’re seeing in robotics, and whether it’s possible to step up the reach of work in this area.
The basic idea here is that—particularly in reinforcement learning, which I mentioned earlier is the branch of machine learning that deals with systems that interact with the environment in a very intertwined wave—there’s a trade-off between exploring and exploiting; between doing the thing that I think is best right now and understanding my environment better, which might lead to me understanding it better and realizing that there are even better things that I can do. But the problem is, that when I’m exploring an unknown environment, often there are aspects that I’ve never dealt with before, and so I can do something dangerous without knowing what I’m doing.
The example gave with the cleaning robot is, you know, maybe it’s never seen an electrical outlet before. And so it wants to experiment with cleaning strategies and tries to stick a wet mop in the electrical outlet. Obviously this is just going to be really bad for the robot. Another example that has actually come up with actual robots people have built is robot helicopters. The idea is if I want to train my robot helicopter how to fly properly; I want to use reinforcement learning to learn how to fly. One problem we can have is that, you know, if it’s experimenting with spinning propellers, and doesn’t really understand the dynamics of flying very well… if it does something bad and ends up crashing, it could break its propeller or break it’s control system or something, and then you can’t use the robot helicopter anymore, right? The system is broken and you need to get a new one, and the designer for the system won’t be very happy. And yet the system needs to learn somehow, and so again this is a problem children encounter, right? Children need to try things on their own to understand what works and what doesn’t, but it’s also very important that they don’t do things that are truly dangerous that they couldn’t recover from if it goes wrong. And you know to some extent children have an instinct for this, and it’s part of the role of parents to keep children from going into truly dangerous situations. But it’s something that our machine learning systems currently grapple with, and I think are going to need to grapple with more and more.
Seth Baum: That seems like something that we all grapple with on a pretty regular basis. For myself, as an academic I’m constantly worrying about whether I’m spending too much time thinking about stuff, and researching and learning more and so on versus just going ahead and writing what I have and forming an opinion and getting out there and saying what I have to say on the topic… and it’s a dilemma, right, how hard we try to figure things out before we do things. In the tech community they have the old Facebook saying “move fast and break things,” which for some contexts works well, but for other contexts does not work so well. And actually Facebook has changed. It’s now “move fast with stable infrastructure,” something that sounds more responsible and not nearly as catchy. So yeah I guess an AI would have to face the same sorts of issues, right?
Dario Amodei: Yeah, I mean I think the problem of machine learning and AI is to get machines to do some of the same tasks that humans do. So in some sense I think it’s not surprising that in doing some of the same task humans do they run into a lot of the same problems that humans do.
Seth Baum: Okay so we got one more of the concrete problems, and it’s called “robustness to distributional shift.”
Dario Amodei: This is the idea that often if you have a machine learning system, we have the notion of kind of training data and test data. A machine learning system often get trained on one particular type of data, but then when it’s deployed in the real world it often finds itself in situations or faced with data that might be different from the data that it was trained on. Our example with the robot is: let’s say we have a robot that’s been trained to clean factory work floors. It’s kind of learned that you should use harsh chemicals to do this, and that it needs to avoid lots of metal obstacles. You then deploy it in an office, and it might engage in some behavior that’s inappropriate. It might use chemicals that are too harsh, it might not understand how the office is setup, etc., etc.
I think another example of this actually is the gorilla example that occurred with Google a year ago, where one of the problems with that photo captioning app was that a lot of its training data had been trained on caucasian individuals, and it had seen monkeys, but it had never seen an individual with different skin color. So it made a very inappropriate inference based on insufficient training data. Our interest in robustness to distributional shift is in trying to both detect and remedy situations where you’re seeing something that is different than what you’ve seen before. The photo caption system should have said, “this is something that doesn’t actually look like anything that I’ve seen before or any of the classes that I’ve seen before—it’s actually something different, and I should be very careful of what class I assign this to, because I don’t have high confidence about the situation and I’m aware that I’m facing data that’s different from the data that I was trained on.” It’s not only possible to respond appropriately to a totally new situation or totally new perception that I might receive, but it seems like it is possible to recognize that what I’m seeing is different from what I’ve seen before. So that’s when the paper discusses how to recognize that and how to be appropriately cautious once you recognize it.
Seth Baum: It’s really interesting to me just listening to you talk about these different challenges of designing an AI to behave in ways we would want to behave. How similar to me at least it sounds like child development and human behavior and challenges that we all face. It makes me feel like these artificial intelligence systems are already not so different from us, at least in these types of ways.
Dario Amodei: Well I would definitely say that certainly the systems that we are building today are very limited systems. I do want to emphasize that we are nowhere near building systems that can replicate the incredible range of behaviors that humans are capable of. However I would say that within the particular tasks that we assign to machine learning systems… yeah I think so many of the problems that they face in learning those specific tasks often—not always—have analogies to the challenges in human space in learning those tasks.
Seth Baum: Okay, so they’re still not at all as capable as we are across the board, but still face some of the same challenges we do.
Dario Amodei: Yeah.
Seth Baum: Okay, very good. So I want to bring it back to the conversation we started out with, which is on what we saw at the White House symposium, what we see in other contexts, about this short-term AI versus long-term AI issues and people caring more about short-term than long-term, and so on. With that in mind, I’m curious what the reaction has been to your paper, right? Do people say, “oh this is crazy,” or do these ideas seem reasonable to them?
Dario Amodei: Actually the reaction has been extremely positive more so than I even anticipated. There’s a few different communities that read and looked at our work. The first was, because it was published on the Google research blog and ended up getting covered by the media, and their reaction was actually quite positive. Most of the stories had titles like: “Google Gets Practical About AI Concerns,” or “Google Addressing AI Concerns Responsibly.” The idea was that it was kind of a set of serious engineers who are really sitting down to think about just very specifically what can go wrong in machine learning systems, and how can we prevent those things from happening so that machine learning systems can benefit everyone. It was very that, and not the kind of alarmist “Terminator robots are going to kill us all” kind of thing. So I feel the media understood actually pretty well, which surprised me a little bit.
Seth Baum: What about from the AI community?
Dario Amodei: Yeah, so the AI community was also extremely positive. Even people like Oren Etzioni—who have been vocal spokespeople against more long-term concerns about AI and risks of AI— you know, they were very positive about this paper. Oren Etzioni was quoted in one of the news articles as saying, “These are the right people asking the right questions.” And I think a lot of the doubts that people like Etzioni have had about long-term AI risk has just been a kind of vagueness: well how do you work on this? I think the reaction to our paper was very positive because it gave the problem posed in a way where you can actually sit down and write a paper on it, and actually, by the way, we intend to follow up on the paper, this was more kind of an agenda paper, but we intend to follow it up by writing papers trying to address these problems in actual real systems. And you know that’s one of the things I’m working on at open AI, and we’re going to continue to collaborate with Google on it. I think that the concreteness part of it and the practicality and the promise of real empirical work that I hope we can deliver on made a lot of the I community seem pretty excited about it.
And then finally there’s the community of people that have been worried about long-term AI risks. I think the reaction there was pretty positive as well, even though the focus of the paper was on shorter term issues as Ariel pointed out. The beginning of this, conceptually a lot of the longer term risks that they’re worried about can be seen as instances of some of the problems we’ve talked about, in particular the negative side effects and reward hacking, but actually all of them are things that—when I try to think about what someone like Nick Bostrom is talking about—I think what they’re talking about is the kind of problems were talking about, the concrete problems paper… if you have those problems with an extremely powerful AI system that is even more powerful than humans, then I think that’s how you get to some of the scenarios that Bostrom is describing. I definitely have a disagreement with the AI safety community, which is, it’s not that I don’t think we may face these extreme scenarios eventually, and I’m glad there’s someone thinking about them, but I at least am most interested in thinking about problems that we can attack empirically today. And I hope that those problems that we attack today will start to shed light on the longer-term issues that we have.
Again, if we really work on things like reward hacking and avoiding negative side effects, I think if we work on them in the right way, there will be a lot of relevance to the scenarios that people worried about AI risk are worried about. Eventually many of the things they’re talking about, the things they write about, maybe they’ll become very relevant someday. But my difference is more tactical; I just see a great deal of importance to having the empirical feedback loop. To saying, “this is a problem I think the system might have, let me test it… oh it has this part of the problem but not this part of the problem, but let me do another iteration on it.” Just in research and science in general I feel we’ve got a lot of mileage out of the empirical feedback loop, and so that’s something that I emphasize a lot.
Seth Baum: I’m really glad to hear that the response has been so positive. This seems to me like the sort of clever solution that we need for problems like AI safety, that can resonate across seemingly disparate audiences. We’ve had a lot of disagreement between the people who are worried about the future of superintelligence risk versus the AI researchers who are out there building new systems today, and my impression is that the difference of opinion between these two groups only goes so far, in that both of them as far as I can tell genuinely do care about the social impact of their work. It might not be the core focus of everyone’s attention, and I think this is an issue that needs to be addressed within the AI community.
Stewart Russell has a great line about how the AI community needs to take social impacts more seriously, he compares it to civil engineers, he says no one in civil engineering talks about building bridges that don’t fall down, they just they just call it building bridges, right? Because in civil engineering everyone takes for granted that the social impact of their work really matters, whereas in AI (according to Stuart Russell) that’s less the case… but the impression I have listening to AI researchers, a lot of them do actually care about the social impacts of their work, they’re just not sure about this superintelligence thing. It’s remote into the future, it’s speculative, maybe even sounds a little odd and it’s just so far removed from the systems that they’re working on. So to see opportunities to address the General AI safety concerns—that may also be relevant to superintelligence but are very much relevant to the systems that people are building today—it makes sense to me that they would respond positively to that sort of message.
And I wonder if there’s anybody especially within the AI research communities that is pushing back against it, saying, “no, we should just be focused on building AI systems that are more capable and we shouldn’t worry about these safety problems.” Have you gotten that at all?
Dario Amodei: I don’t think I’ve ever had someone say that specifically to me. I think there is probably a healthy debate to some extent in the machine learning community about which social impacts we should care the most about. Some of my colleagues are very interested—and I am actually, too—in things like the economic impact of machine learning systems, or in fairness. I think definitely people differ in how much they choose to focus on each of these issues, but I haven’t really encountered anyone who says, “we shouldn’t think about any of these issues,” or who says, “this is the only issue that we should think about.” I think, when properly explained, the risk of AI systems doing things that we didn’t intend… everyone says, “yes, that’s something you should prevent.” The risk of AI systems treating people unfairly, that’s something “yes we should prevent.” The risk of bad economic impacts of AI everyone says, “yes that is something we should prevent.” Internet security issues that could arise with AI everyone says, “yes that’s something we should definitely prevent.” Different people are interested in working on these to different extents, and for some people this isn’t a personal research interest to them, but I actually haven’t found anyone who says, “no, I don’t think anyone should work on these things.” Maybe such people do exist, but I haven’t met any.
Seth Baum: Maybe that’s the bigger challenge with this? It’s not people who actively push back against work on these problems, but people who just essentially ignore it. I remember from my own engineering days—and I really like to bring up social impacts and social issues related to our research—my fellow engineers would listen to me and then they would basically be like “Okay, that’s nice. Now get back to work,” because in their minds, thinking about the social aspect of it was someone else’s job, and so it’s easy to imagine AI researchers not really disagreeing with the sorts of things that you’re saying, but just thinking that maybe this is somebody else’s responsibility to worry about. Do you see that at all?
Dario Amodei: I have definitely heard people say that, but to be fair I don’t have a huge objection to some fraction—maybe even a large fraction of the field—having that attitude. I mean, I think research is a process of specialization, and not everyone can work on everything. If your attitude is “I just want to make a better speech system. I know that machine learning has social impacts, and someone else is working on that.” If a decent fraction of the field takes that attitude, I’m fine with it. My concern is more that we, as a field collectively, that we are on the issue, that we have enough people within the field who do want to think about these issues. If there’s no one or too few people in the field who want to think about these issues, then I think that’s a problem, because I think it’s our responsibility as researchers to think about the impact of the research that we’re doing. But if a particular person says, “that’s not my cup of tea, that’s not my focus area,” I’m fine with that. I think that’s the way research works. But I would say that right now the fraction of people doing this, at least a year ago, I would say was too low. Now I think thankfully we’re starting to get more and more people into this and maybe getting to a healthier place.
Seth Baum: Okay, that was what I was going to ask you. Because I presume based on the fact that you’re speaking up on this topic, that there’s a sense there should be more work going on on this? It seems like the paper you wrote was, as you put it, an agenda—a call for action, a call for research on these topics. It’s very encouraging to hear that you think that the fraction of people working on these safety problems is going up. Would you still say that it should be going up more? Or do you think that we’re actually reaching a pretty comfortable place right now?
Dario Amodei: I mean, it’s all kind of coming into place. Since I joined OpenAI, I’ve had a number of people say, “I’m interested in these topics; I want to work on these topics.” The new people coming into the ML field and people who have been in there in a while. So I actually don’t know where things will end up, and my main goal is just to get some good technical research done on these topics. Then we’ll see if there needs to be more people in the field. And my hope is the usual dynamic: there’s a lot of interesting results found in one place, then more people come into the field. Then if there’s too many people working on something, some people go somewhere else. I’m hopeful that those normal dynamics will get us to a place where we’re thinking responsibly. That may be too optimistic, but that’s my hope.
Seth Baum: That makes sense to me, and let’s hope that things do balance out there. In my experience, researchers don’t necessarily always gravitate towards just where the right topics are, what research most needs to be done, and you end up with too many people crowding in one seemingly popular area. But we’ll see. I’m really glad to hear this, and it would be great if these safety problems could then just solve themselves as more AI researchers work on them.
Dario Amodei: Yeah, that would be my hope for what would happen.
Seth Baum: Okay, thank you. Any final thoughts you’d like to add to this conversation before we sign off?
Dario Amodei: You know I think my perspective is that empirical and testable work on unintended consequences of machine learning systems is the best way to illuminate these problems and figure out where to go next.
Seth Baum: Okay, thank you.
Ariel Conn: I want to thank you both for sitting down and having this discussion. I think this helps shed a lot of light, at least on the issues I saw at the White House symposia. And it’s been a really great overview of where we’re at with AI safety research today. So Dario and Seth, thank you very much.
Seth Baum: Thank you.
Dario Amodei: Thank you for having me.
About the Future of Life Institute
The Future of Life Institute (FLI) is a global think tank with a team of 20+ full-time staff operating across the US and Europe. FLI has been working to steer the development of transformative technologies towards benefitting life and away from extreme large-scale risks since its founding in 2014. Find out more about our mission or explore our work.
Related content
Other posts about Uncategorized
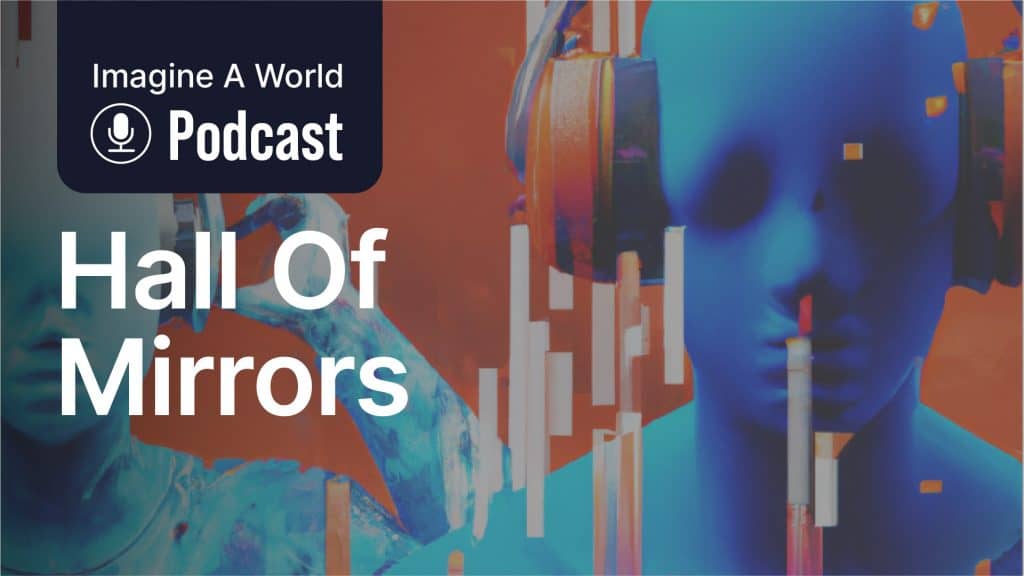
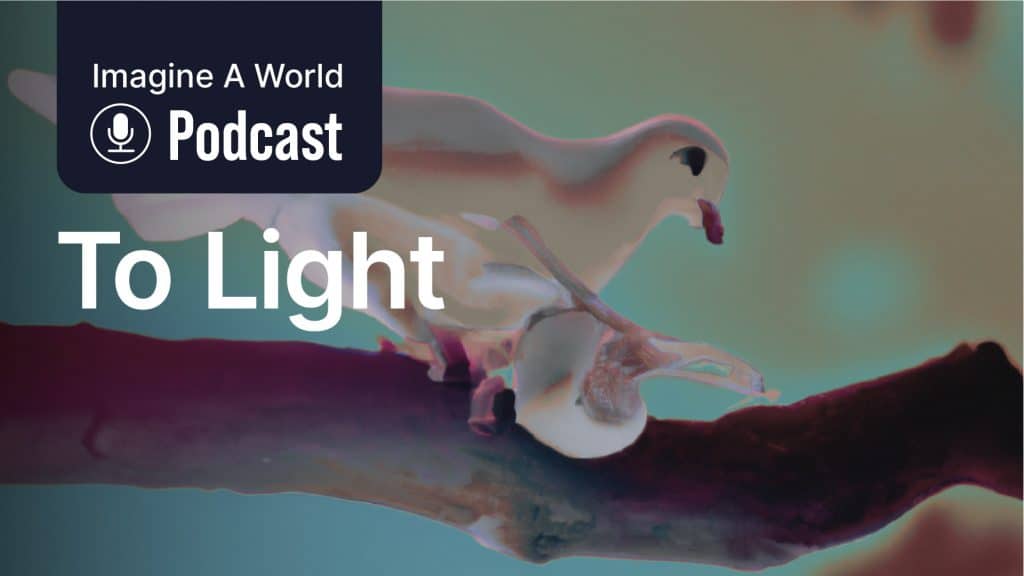