Predicting the Future (of Life)
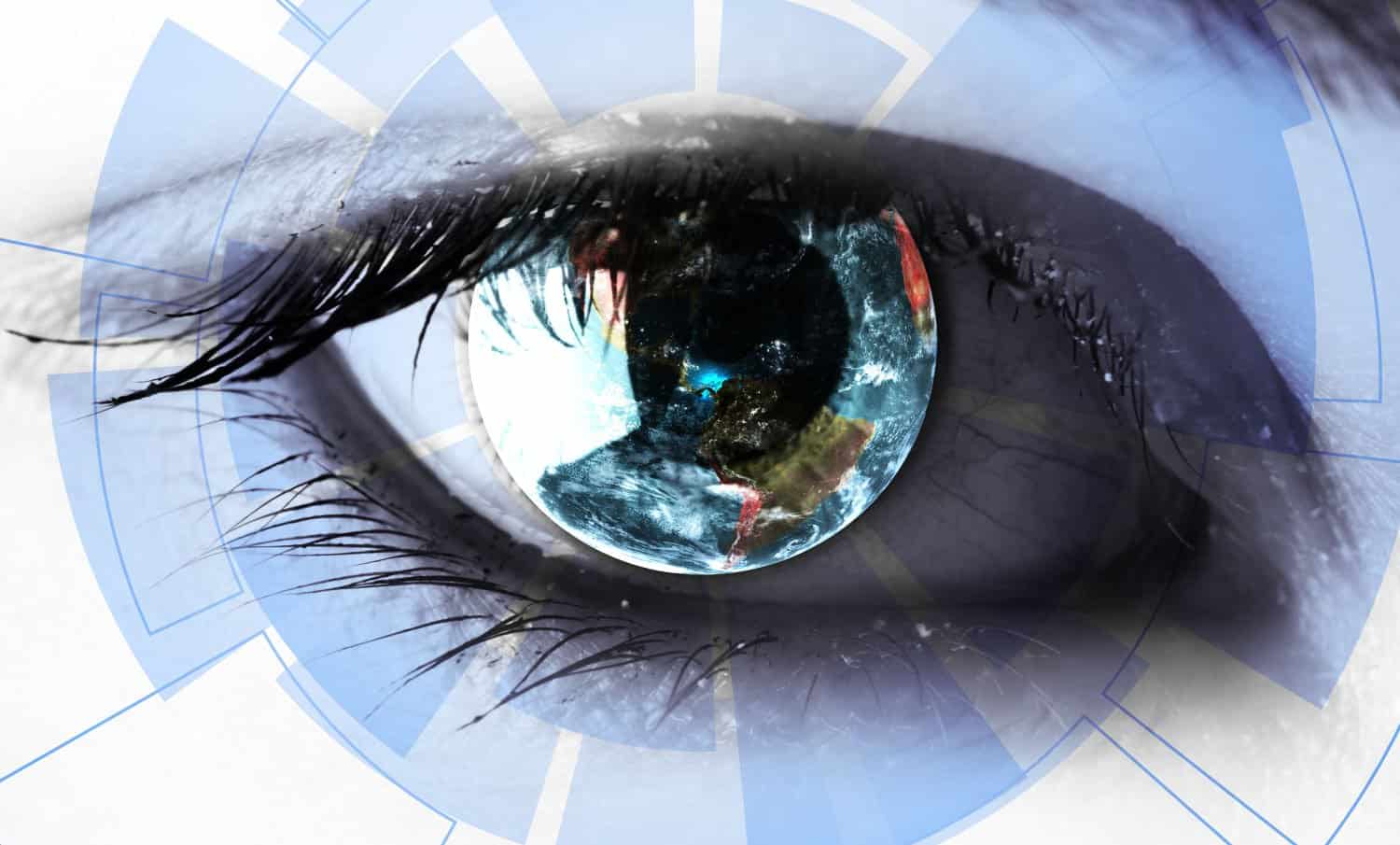
Contents
It’s often said that the future is unpredictable. Of course, that’s not really true. With extremely high confidence, we can predict that the sun will rise in Santa Cruz, California at 7:12 am local time on Jan 30, 2016. We know the next total solar eclipse over the U.S. will be August 14, 2017, and we also know there will be one June 25, 2522.
We can predict next year’s U.S. GDP to within a few percent, what will happen if we jump off a bridge, the Earth’s mean temperature to within a degree, and many other things. Just about every decision we make implicitly includes a prediction for what will occur given each possible choice, and much of the time we make these predictions unconsciously and actually pretty accurately. Hide, or pet the tiger? Jump over the ravine, or climb down? Befriend, or attack? Our mind contains a number of systems comprising quite a sophisticated prediction engine that keeps us alive by helping make good choices in a complex world.
Yet we’re often frustrated at our inability to predict better. What does this mean, precisely? It’s useful to break prediction accuracy into two components: resolution and calibration. Resolution measures how close your predictions are to 0% or 100% likelihood. Thus the prediction that a fair coin will land heads-up 50% of the time has very low resolution. However, if the coin is fair, this prediction has excellent calibration, meaning that the prediction is very close to the relative frequency of heads vs. coin flips in a long series of trials. On the other hand, many people made confident predictions that they “would win” (i.e. probability 1) the recent $1.5 Billion Powerball lottery. These predictions had excellent resolution but terrible calibration.
When we say we can’t predict the future, there are generally a few different things we mean. Sometime we have excellent calibration but poor resolution. A good blackjack player knows the odds of hitting 21, but fun of the game (and the Casino’s profits) relies on nobody having better resolution than the betting odds provide. Weather reports provide a less fun example of generally excellent calibration with resolution that is never as good as we would like. While lack of resolution is frustrating, having good calibration allows good decision-making because we can compute reliable expected values based on well-calibrated probabilities.
Insurance companies don’t know who is going to get in an accident, but can set sensible policies and costs based on statistical probabilities. Startup investors can value a company based on expected future earnings that combine the (generally low) probability of success with the (generally high) value if successful. And Effective Altruists can try to do the most expected good — or try to mitigate the most expected harm — by weighting the magnitude of various occurrences by the probability of their occurrence.
What are much much less useful are predictions with poor, unknown, or non-existent calibration. These, alas, are what we get a lot of the from pundits, from self-appointed (or even real) experts, and even in many quantitive predictive studies. I find this maximally frustrating in discussions of catastrophic or existential risk. For example:
Concerned Scientist: I’m a little worried that unprecedentedly rapid global warming could lead to a runway greenhouse effect and turn Earth into Venus.
Unconcerned Expert: Well, I don’t think that’s very likely.
Concerned Scientist: Well, if it happens, all of humanity and life on Earth, and all humans who might ever live over countless eons will be extinguished. What would you say the probability is?
Unconcerned Expert: Look, I really don’t think it’s very likely.
Concerned Scientist: 0.0001%? 1%? 10%?
Unconcerned Expert: Look, I really don’t think it’s very likely.
Now, if you try to weigh this worry against:
“I’m a little worried that one of the dozens of near-miss nuclear accidents likely to happen over the next twenty years will lead to a nuclear war.”
Or
“I’m a little worried that an apocalyptic cult could use CRISPR and published gain-of-function research to bioengineer a virus to cleanse the Earth of all humans.”
Or
“I’m a little worried that a superintelligent AI could decide that human decision-makers are too large a source of uncertainty and should be converted into paperclips for predictable mid-level bureaucrats to use.”
Then you can see that the Unconcerned Expert’s response is not useful: it provides no guidance whatsoever into what level of resources should be targeted at reducing this risk, either absolutely or relative to other existential risks.
People do not generally have a good intuition for small probabilities, and to be fair, computing them can be quite challenging. A small probability generally suggests that there are many, many possible outcomes, and reliably identifying, characterizing, and assessing these many outcomes is very hard. Take the probability that the US will become engaged in a nuclear war. It’s (we hope!) quite small. But how small? There are many routes by which a nuclear war might happen, and we’d need to identify each route, break each route into components, and then assign probabilities to each of these components. For example, inspired by the disturbing sequence of events envisioned in this Vox article, we might ask:
1: Will there be significant ethnic Russian protests in Estonia in the next 5 years?
2: If there are protests, will NATO significantly increase its military presence there?
3: If there are protests, do 10 or more demonstrators die in the protests?
4: If there are increased NATO forces and violent protests, does violence escalate into a military conflict?
Etc.
Each of these component questions is much easier to address, and together can indicate a reasonably well-calibrated probability for one path toward nuclear conflict. This is not, however, something we can generally do ‘on the fly’ without significant thought and analysis.
What if we do put the time and energy into assessing these sequences of possibilities? Assigning probabilities to these chains of mutually exclusive possibilities would create a probability map of a tiny portion of the landscape of possible futures. Somewhat like ancient maps, this map must be highly imperfect, with significant inaccuracies, unwarranted assumptions, and large swathes of unknown territory. But a flawed map is much better than no map!
Some time back, when pondering how great it would be to have a probability map like this, I decided it would require a few ingredients.
First, it would take a lot of people combining their knowledge and expertise. The world — and the set of issues at hand — is a very complex system, and even enumerating the possibilities, let alone assigning likelihoods to them, is a large task. Fortunately, there are good precedents for crowdsourced efforts: Wikipedia, Quora, Reddit, and other efforts have created enormously valuable knowledge bases using the aggregation of large numbers of contributions.
Second, it would take a way of identifying which people are really really good at making predictions. Many people are terrible at it — but finding those who excel at predicting, and aggregating their predictions, might lead to quite accurate ones. Here also, there is very encouraging precedent. The Aggregative Contingent Estimation project run by IARPA, one component of which is the Good Judgement Project, has created a wealth of data indicating that (a) prediction is a trainable, identifiable, persistent skill, and (b) by combining predictions, well-calibrated probabilities can be generated for even complex geopolitical events.
Finally, we’d need a system to collect, optimally combine, calibrate, and interpret all of the data. This was the genesis of the idea for Metaculus, a new project I’ve started with several other physicists. Metaculus is quickly growing and evolving into a valuable tool that can help humanity make better decisions. I encourage you to check out the open questions and predictions, and make your own forecasts. It’s fun, and even liberating to stop thinking of the future as unknowable, and to start exploring it instead!
About the Future of Life Institute
The Future of Life Institute (FLI) is a global non-profit with a team of 20+ full-time staff operating across the US and Europe. FLI has been working to steer the development of transformative technologies towards benefitting life and away from extreme large-scale risks since its founding in 2014. Find out more about our mission or explore our work.
Related content
Other posts about Partner Orgs, Recent News
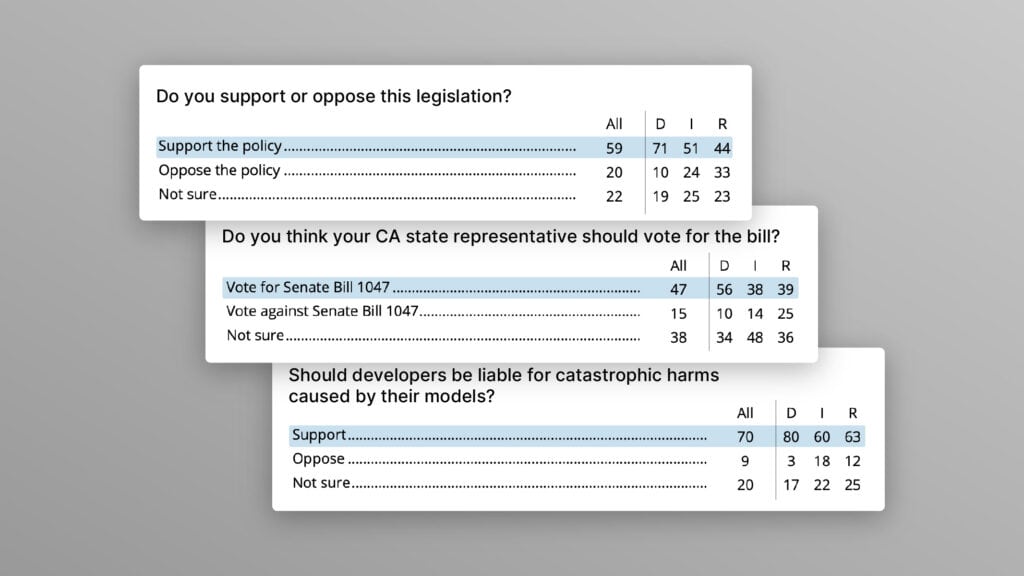
Poll Shows Broad Popularity of CA SB1047 to Regulate AI
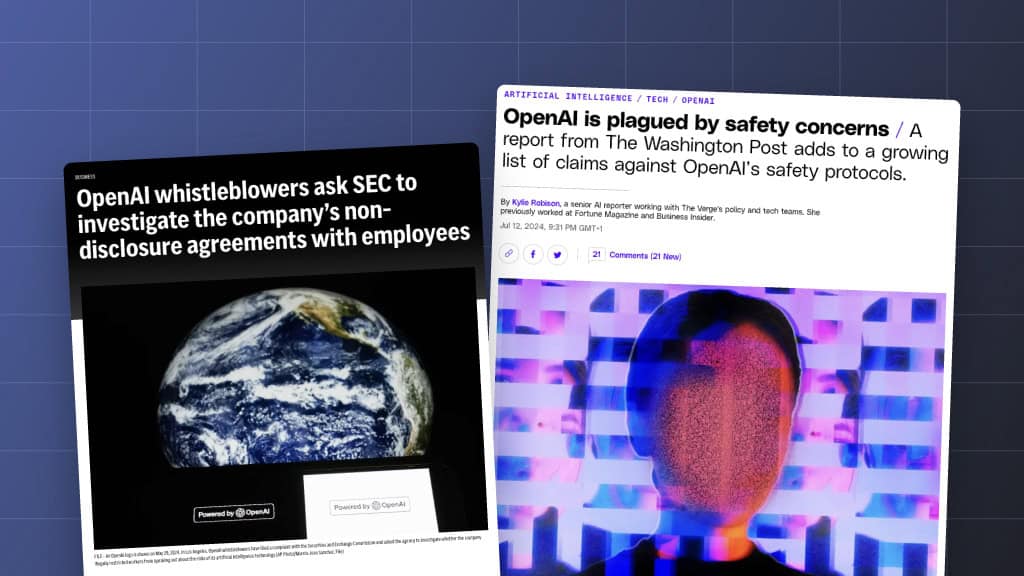
FLI Praises AI Whistleblowers While Calling for Stronger Protections and Regulation
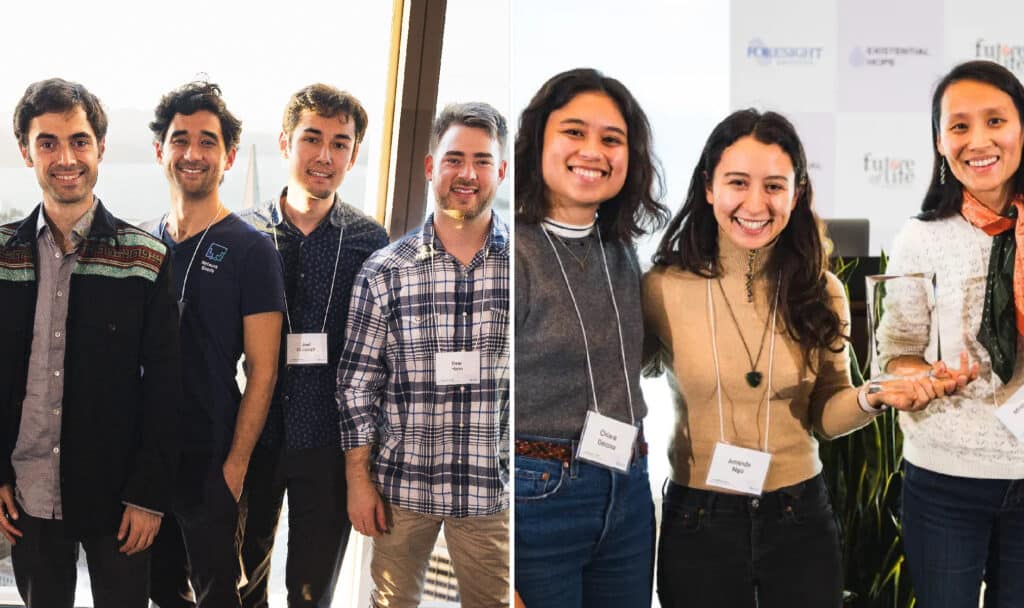
Designing Governance for Transformative AI: Top Proposals from the FLI & Foresight Institute Hackathon
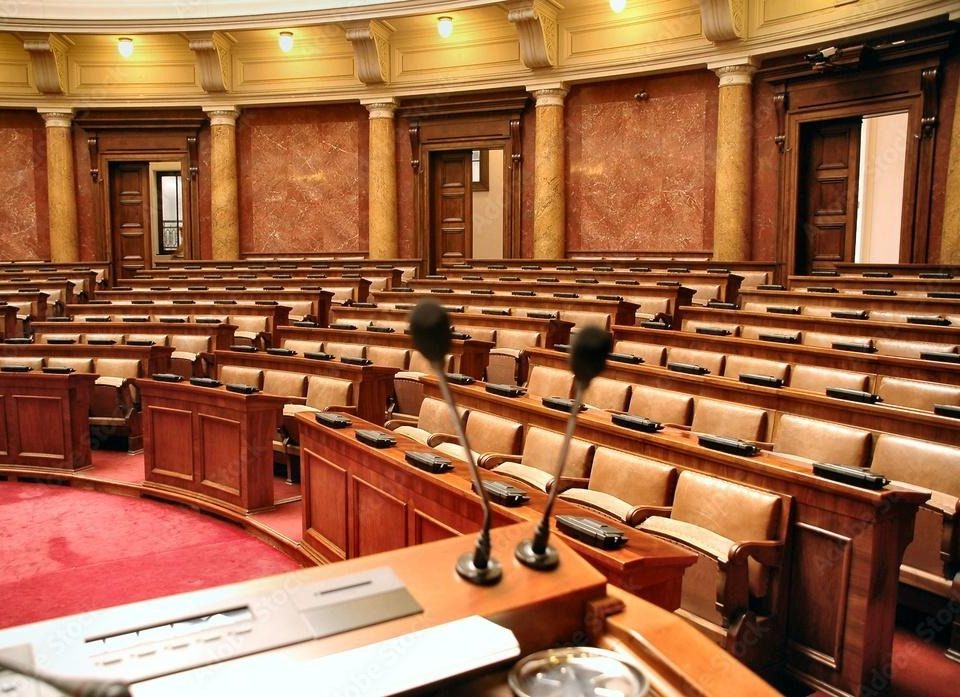