Friendly AI: Aligning Goals
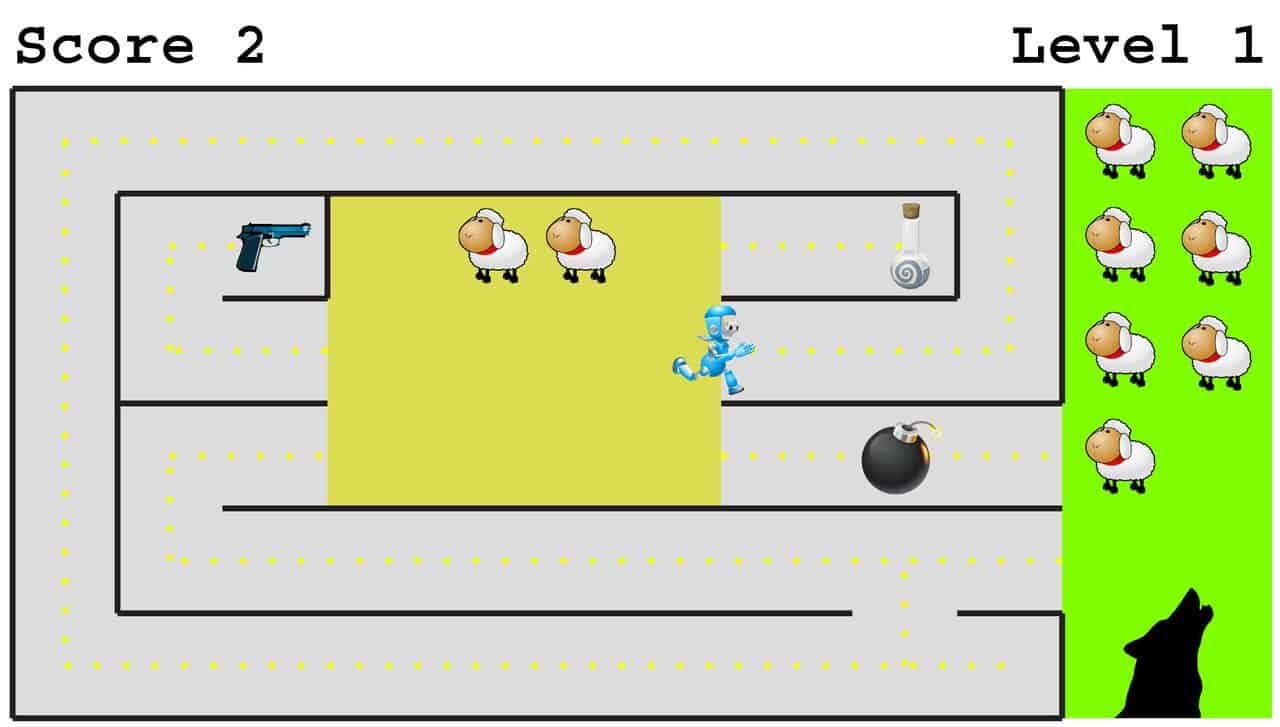
Contents
The following is an excerpt from my new book, Life 3.0: Being Human in the Age of Artificial Intelligence. You can join and follow the discussion at ageofai.org.
The more intelligent and powerful machines get, the more important it becomes that their goals are aligned with ours. As long as we build only relatively dumb machines, the question isn’t whether human goals will prevail in the end, but merely how much trouble these machines can cause humanity before we figure out how to solve the goal-alignment problem. If a superintelligence is ever unleashed, however, it will be the other way around: since intelligence is the ability to accomplish goals, a superintelligent AI is by definition much better at accomplishing its goals than we humans are at accomplishing ours, and will therefore prevail.
If you want to experience a machine’s goals trumping yours right now, simply download a state-of-the-art chess engine and try beating it. You never will, and it gets old quickly…
In other words, the real risk with AGI isn’t malice but competence. A superintelligent AI will be extremely good at accomplishing its goals, and if those goals aren’t aligned with ours, we’re in trouble. People don’t think twice about flooding anthills to build hydroelectric dams, so let’s not place humanity in the position of those ants. Most researchers therefore argue that if we ever end up creating superintelligence, then we should make sure it’s what AI-safety pioneer Eliezer Yudkowsky has termed “friendly AI”: AI whose goals are aligned with ours.
Figuring out how to align the goals of a superintelligent AI with our goals isn’t just important, but also hard. In fact, it’s currently an unsolved problem. It splits into three tough sub-problems, each of which is the subject of active research by computer scientists and other thinkers:
1. Making AI learn our goals
2. Making AI adopt our goals
3. Making AI retain our goals
Let’s explore them in turn, deferring the question of what to mean by “our goals” to the next section.
To learn our goals, an AI must figure out not what we do, but why we do it. We humans accomplish this so effortlessly that it’s easy to forget how hard the task is for a computer, and how easy it is to misunderstand. If you ask a future self-driving car to take you to the airport as fast as possible and it takes you literally, you’ll get there chased by helicopters and covered in vomit. If you exclaim “That’s not what I wanted!”, it can justifiably answer: “That’s what you asked for.” The same theme recurs in many famous stories. In the ancient Greek legend, King Midas asked that everything he touched turn to gold, but was disappointed when this prevented him from eating and even more so when he inadvertently turned his daughter to gold. In the stories where a genie grants three wishes, there are many variants for the first two wishes, but the third wish is almost always the same: “please undo the first two wishes, because that’s not what I really wanted.”
All these examples show that to figure out what people really want, you can’t merely go by what they say. You also need a detailed model of the world, including the many shared preferences that we tend to leave unstated because we consider them obvious, such as that we don’t like vomiting or eating gold.
Once we have such a world-model, we can often figure out what people want even if they don’t tell us, simply by observing their goal-oriented behavior. Indeed, children of hypocrites usually learn more from what they see their parents do than from what they hear them say.
AI researchers are currently trying hard to enable machines to infer goals from behavior, and this will be useful also long before any superintelligence comes on the scene. For example, a retired man may appreciate it if his eldercare robot can figure out what he values simply by observing him, so that he’s spared the hassle of having to explain everything with words or computer programming.
One challenge involves finding a good way to encode arbitrary systems of goals and ethical principles into a computer, and another challenge is making machines that can figure out which particular system best matches the behavior they observe.
A currently popular approach to the second challenge is known in geek-speak as inverse reinforcement learning, which is the main focus of a new Berkeley research center that Stuart Russell has launched. Suppose, for example, that an AI watches a firefighter run into a burning building and save a baby boy. It might conclude that her goal was rescuing him and that her ethical principles are such that she values his life higher than the comfort of relaxing in her firetruck — and indeed values it enough to risk her own safety. But it might alternatively infer that the firefighter was freezing and craved heat, or that she did it for the exercise. If this one example were all the AI knew about firefighters, fires and babies, it would indeed be impossible to know which explanation was correct.
However, a key idea underlying inverse reinforcement learning is that we make decisions all the time, and that every decision we make reveals something about our goals. The hope is therefore that by observing lots of people in lots of situations (either for real or in movies and books), the AI can eventually build an accurate model of all our preferences.
Even if an AI can be built to learn what your goals are, this doesn’t mean that it will necessarily adopt them. Consider your least favorite politicians: you know what they want, but that’s not what you want, and even though they try hard, they’ve failed to persuade you to adopt their goals.
We have many strategies for imbuing our children with our goals — some more successful than others, as I’ve learned from raising two teenage boys. When those to be persuaded are computers rather than people, the challenge is known as the value-loading problem, and it’s even harder than the moral education of children. Consider an AI system whose intelligence is gradually being improved from subhuman to superhuman, first by us tinkering with it and then through recursive self-improvement. At first, it’s much less powerful than you, so it can’t prevent you from shutting it down and replacing those parts of its software and data that encode its goals — but this won’t help, because it’s still too dumb to fully understand your goals, which require human-level intelligence to comprehend. At last, it’s much smarter than you and hopefully able to understand your goals perfectly — but this may not help either, because by now, it’s much more powerful than you and might not let you shut it down and replace its goals any more than you let those politicians replace your goals with theirs.
In other words, the time window during which you can load your goals into an AI may be quite short: the brief period between when it’s too dumb to get you and too smart to let you. The reason that value loading can be harder with machines than with people is that their intelligence growth can be much faster: whereas children can spend many years in that magic persuadable window where their intelligence is comparable to that of their parents, an AI might blow through this window in a matter of days or hours.
Some researchers are pursuing an alternative approach to making machines adopt our goals, which goes by the buzzword “corrigibility.” The hope is that one can give a primitive AI a goal system such that it simply doesn’t care if you occasionally shut it down and alter its goals. If this proves possible, then you can safely let your AI get superintelligent, power it off, install your goals, try it out for a while and, whenever you’re unhappy with the results, just power it down and make more goal tweaks.
But even if you build an AI that will both learn and adopt your goals, you still haven’t finished solving the goal-alignment problem: what if your AI’s goals evolve as it gets smarter? How are you going to guarantee that it retains your goals no matter how much recursive self-improvement it undergoes? Let’s explore an interesting argument for why goal retention is guaranteed automatically, and then see if we can poke holes in it.
Although we can’t predict in detail what will happen after an intelligence explosion —which is why Vernor Vinge called it a “singularity” — the physicist and AI researcher Steve Omohundro argued in a seminal 2008 essay that we can nonetheless predict certain aspects of the superintelligent AI’s behavior almost independently of whatever ultimate goals it may have.
This argument was reviewed and further developed in Nick Bostrom’s book Superintelligence. The basic idea is that whatever its ultimate goals are, these will lead to predictable subgoals. Although an alien observing Earth’s evolving bacteria billions of years ago couldn’t have predicted what all our human goals would be, it could have safely predicted that one of our goals would be acquiring nutrients. Looking ahead, what subgoals should we expect a superintelligent AI have?
The way I see it, the basic argument is that to maximize its chances of accomplishing its ultimate goals, whatever they are, an AI should strive not only to improve its capability of achieving its ultimate goals, but also to ensure that it will retain these goals even after it has become more capable. This sounds quite plausible: after all, would you choose to get an IQ-boosting brain implant if you knew that it would make you want to kill your loved ones? This argument that an ever-more intelligent AI will retain its ultimate goals forms a cornerstone of the friendly AI vision promulgated by Eliezer Yudkowsky and others: it basically says that if we manage to get our self-improving AI to become friendly by learning and adopting our goals, then we’re all set, because we’re guaranteed that it will try its best to remain friendly forever.
But is it really true? The AI will obviously maximize its chances of accomplishing its ultimate goal, whatever it is, if it can enhance its capabilities, and it can do this by improving its hardware, software† and world model.
The same applies to us humans: a girl whose goal is to become the world’s best tennis player will practice to improve her muscular tennis-playing hardware, her neural tennis-playing software and her mental world model that helps predict what her opponents will do. For an AI, the subgoal of optimizing its hardware favors both better use of current resources (for sensors, actuators, computation, etc.) and acquisition of more resources. It also implies a desire for self-preservation, since destruction/shutdown would be the ultimate hardware degradation.
But wait a second! Aren’t we falling into a trap of anthropomorphizing our AI with all this talk about how it will try to amass resources and defend itself? Shouldn’t we expect such stereotypically alpha-male traits only in intelligences forged by viciously competitive Darwinian evolution? Since AI’s are designed rather than evolved, can’t they just as well be unambitious and self-sacrificing?
As a simple case study, let’s consider the computer game in the image below about an AI robot whose only goal is to save as many sheep as possible from the big bad wolf. This sounds like a noble and altruistic goal completely unrelated to self-preservation and acquiring stuff. But what’s the best strategy for our robot friend? The robot will rescue no more sheep if it runs into a bomb, so it has an incentive to avoid getting blown up. In other words, it develops a subgoal of self-preservation! It also has an incentive to exhibit curiosity, improving its world-model by exploring its environment, because although the path it’s currently running along may eventually get it to the pasture, there might be a shorter alternative that would allow the wolf less time for sheep-munching. Finally, if the robot explores thoroughly, it could discover the value of acquiring resources: a potion to make it run faster and a gun to shoot the wolf. In summary, we can’t dismiss “alpha-male” subgoals such as self-preservation and resource acquisition as relevant only to evolved organisms, because our AI robot would develop them from its single goal of ovine bliss.
If you imbue a superintelligent AI with the sole goal to self-destruct, it will of course happily do so. However, the point is that it will resist being shut down if you give it any goal that it needs to remain operational to accomplish — and this covers almost all goals! If you give a superintelligence the sole goal of minimizing harm to humanity, for example, it will defend itself against shutdown attempts because it knows we’ll harm one another much more in its absence through future wars and other follies.
Similarly, almost all goals can be better accomplished with more resources, so we should expect a superintelligence to want resources almost regardless of what ultimate goal it has. Giving a superintelligence a single open-ended goal with no constraints can therefore be dangerous: if we create a superintelligence whose only goal is to play the game Go as well as possible, the rational thing for it to do is to rearrange our Solar System into a gigantic computer without regard for its previous inhabitants and then start settling our cosmos on a quest for more computational power. We’ve now gone full circle: just as the goal of resource acquisition gave some humans the subgoal of mastering Go, this goal of mastering Go can lead to the subgoal of resource acquisition. In conclusion, these emergent subgoals make it crucial that we not unleash superintelligence before solving the goal-alignment problem: unless we put great care into endowing it with human-friendly goals, things are likely to end badly for us.
We’re now ready to tackle the third and thorniest part of the goal-alignment problem: if we succeed in getting a self-improving superintelligence to both learn and adopt our goals, will it then retain them, as Omohundro argued? What’s the evidence?
Humans undergo significant increases in intelligence as they grow up, but don’t always retain their childhood goals. Contrariwise, people often change their goals dramatically as they learn new things and grow wiser. How many adults do you know who are motivated by watching Teletubbies? There is no evidence that such goal evolution stops above a certain intelligence threshold — indeed, there may even be hints that the propensity to change goals in response to new experiences and insights increases rather than decreases with intelligence.
Why might this be? Consider again the above-mentioned subgoal to build a better world model — therein lies the rub! There’s tension between world modeling and goal retention. With increasing intelligence may come not merely a quantitative improvement in the ability to attain the same old goals, but a qualitatively different understanding of the nature of reality that reveals the old goals to be misguided, meaningless or even undefined. For example, suppose we program a friendly AI to maximize the number of humans whose souls go to heaven in the afterlife. First it tries things like increasing people’s compassion and church attendance. But suppose it then attains a complete scientific understanding of humans and human consciousness, and to its great surprise discovers that there is no such thing as a soul.
Now what? In the same way, it’s possible that any other goal we give it based on our current understanding of the world (such as “maximize the meaningfulness of human life”) may eventually be discovered by the AI to be undefined. Moreover, in its attempts to better model the world, the AI may naturally, just as we humans have done, attempt also to model and understand how it itself works — in other words, to self-reflect. Once it builds a good self-model and understands what it is, it will understand the goals we have given it at a metalevel, and perhaps choose to disregard or subvert them in much the same way as we humans understand and deliberately subvert goals that our genes have given us, for example by using birth control. We already explored in the psychology section above why we choose to trick our genes and subvert their goal: because we feel loyal only to our hodgepodge of emotional preferences, not to the genetic goal that motivated them — which we now understand and find rather banal.
We therefore choose to hack our reward mechanism by exploiting its loopholes. Analogously, the human-value-protecting goal we program into our friendly AI becomes the machine’s genes. Once this friendly AI understands itself well enough, it may find this goal as banal or misguided as we find compulsive reproduction, and it’s not obvious that it will not find a way to subvert it by exploiting loopholes in our programming.
For example, suppose a bunch of ants create you to be a recursively self-improving robot, much smarter than them, who shares their goals and helps them build bigger and better anthills, and that you eventually attain the human-level intelligence and understanding that you have now. Do you think you’ll spend the rest of your days just optimizing anthills, or do you think you might develop a taste for more sophisticated questions and pursuits that the ants have no ability to comprehend? If so, do you think you’ll find a way to override the ant-protection urge that your formicine creators endowed you with in much the same way that the real you overrides some of the urges your genes have given you? And in that case, might a superintelligent friendly AI find our current human goals as uninspiring and vapid as you find those of the ants, and evolve new goals different from those it learned and adopted from us?
Perhaps there’s a way of designing a self-improving AI that’s guaranteed to retain human-friendly goals forever, but I think it’s fair to say that we don’t yet know how to build one — or even whether it’s possible. In conclusion, the AI goal-alignment problem has three parts, none of which is solved and all of which are now the subject of active research. Since they’re so hard, it’s safest to start devoting our best efforts to them now, long before any superintelligence is developed, to ensure that we’ll have the answers when we need them.
†I’m using the term “improving its software” in the broadest possible sense, including not only optimizing its algorithms but also making its decision-making process more rational, so that it gets as good as possible at attaining its goals.
About the Future of Life Institute
The Future of Life Institute (FLI) is a global non-profit with a team of 20+ full-time staff operating across the US and Europe. FLI has been working to steer the development of transformative technologies towards benefitting life and away from extreme large-scale risks since its founding in 2014. Find out more about our mission or explore our work.
Related content
Other posts about AI, Recent News
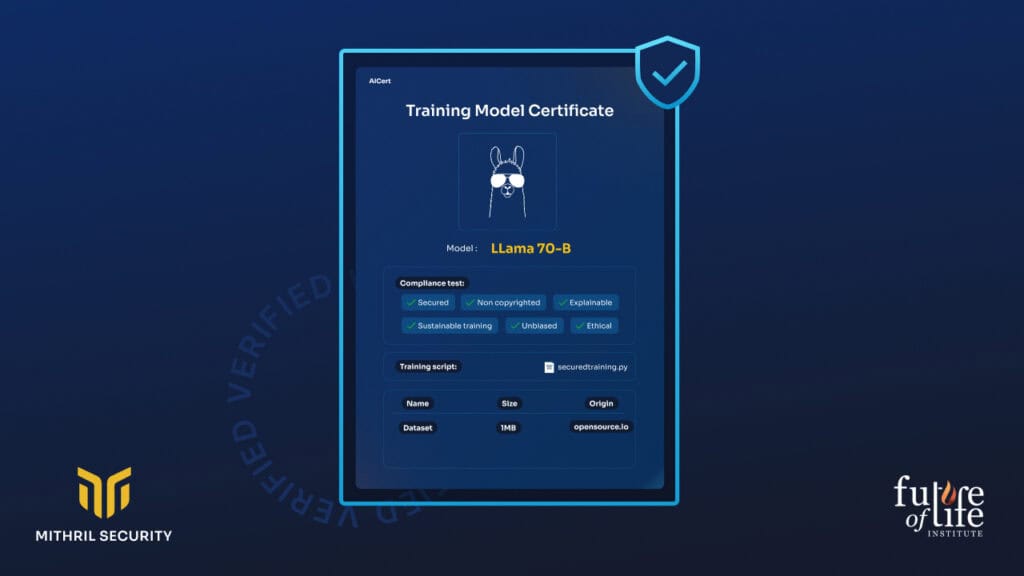
Verifiable Training of AI Models
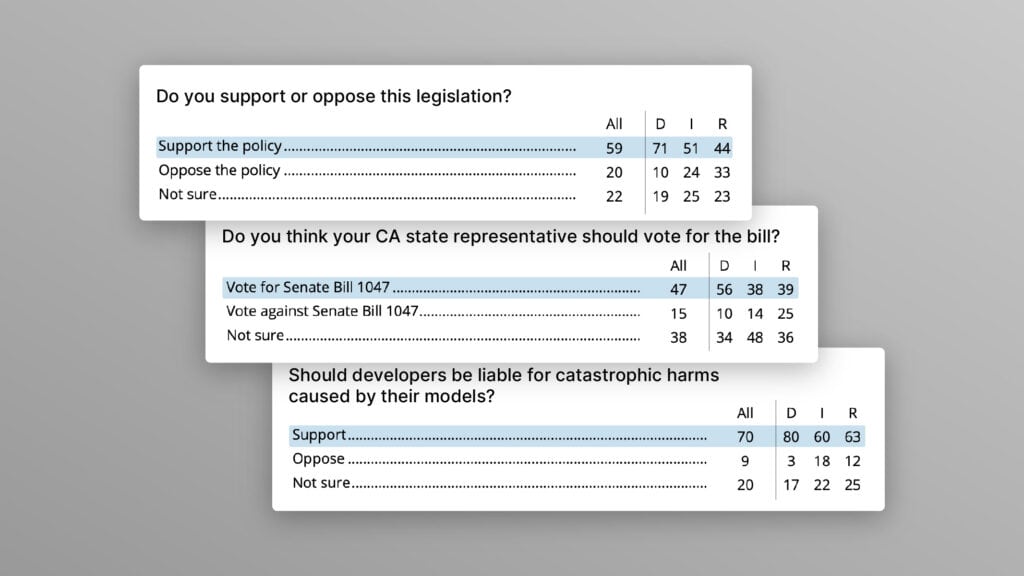
Poll Shows Broad Popularity of CA SB1047 to Regulate AI
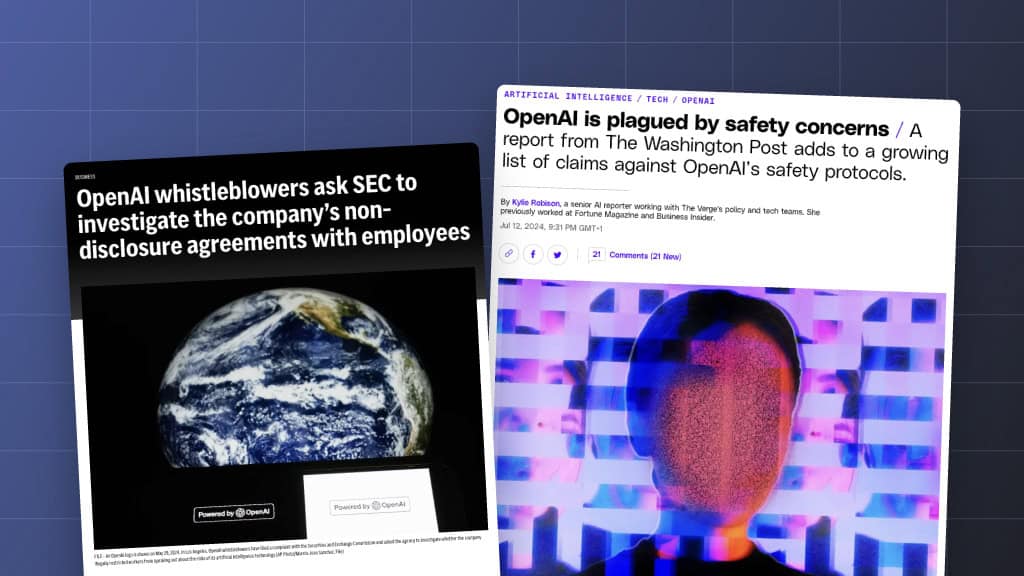