Steven Pinker and Stuart Russell on the Foundations, Benefits, and Possible Existential Threat of AI
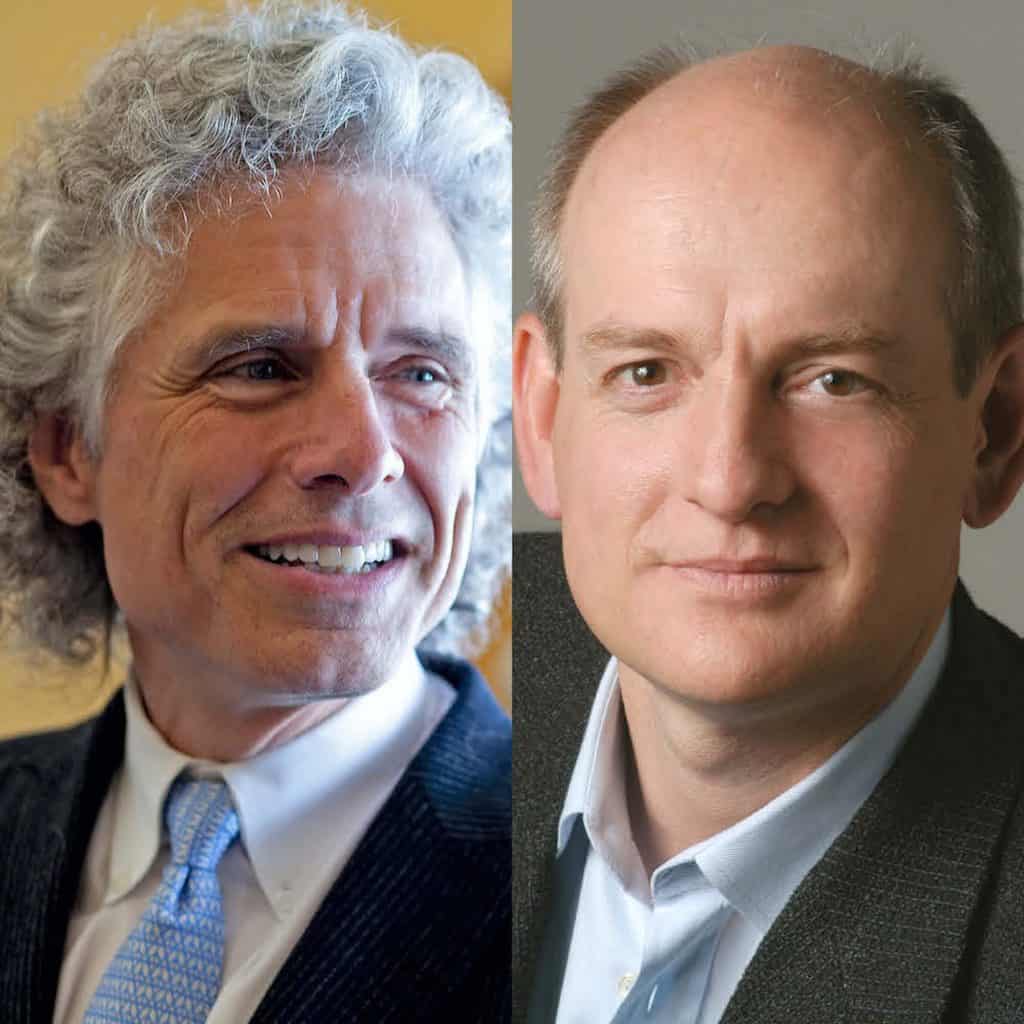
Over the past several centuries, the human condition has been profoundly changed by the agricultural and industrial revolutions. With the creation and continued development of AI, we stand in the midst of an ongoing intelligence revolution that may prove far more transformative than the previous two. How did we get here, and what were the intellectual foundations necessary for the creation of AI? What benefits might we realize from aligned AI systems, and what are the risks and potential pitfalls along the way? In the longer term, will superintelligent AI systems pose an existential risk to humanity? Steven Pinker, best selling author and Professor of Psychology at Harvard, and Stuart Russell, UC Berkeley Professor of Computer Science, join us on this episode of the AI Alignment Podcast to discuss these questions and more.
Topics discussed in this episode include:
- The historical and intellectual foundations of AI
- How AI systems achieve or do not achieve intelligence in the same way as the human mind
- The rise of AI and what it signifies
- The benefits and risks of AI in both the short and long term
- Whether superintelligent AI will pose an existential risk to humanity
You can take a survey about the podcast here
Submit a nominee for the Future of Life Award here
Timestamps:
0:00 Intro
4:30 The historical and intellectual foundations of AI
11:11 Moving beyond dualism
13:16 Regarding the objectives of an agent as fixed
17:20 The distinction between artificial intelligence and deep learning
22:00 How AI systems achieve or do not achieve intelligence in the same way as the human mind
49:46 What changes to human society does the rise of AI signal?
54:57 What are the benefits and risks of AI?
01:09:38 Do superintelligent AI systems pose an existential threat to humanity?
01:51:30 Where to find and follow Steve and Stuart
Works referenced:
Steven Pinker's website and his Twitter
Stuart Russell's new book, Human Compatible: Artificial Intelligence and the Problem of Control
We hope that you will continue to join in the conversations by following us or subscribing to our podcasts on Youtube, Spotify, SoundCloud, iTunes, Google Play, Stitcher, iHeartRadio, or your preferred podcast site/application. You can find all the AI Alignment Podcasts here.
Transcript
Note: The following transcript has been edited for style and clarity.
Lucas Perry: Welcome to the AI Alignment Podcast. I’m Lucas Perry. Today, we have a conversation with Steven Pinker and Stuart Russell. This episode explores the historical and intellectual foundations of AI, how AI systems achieve or do not achieve intelligence in the same way as the human mind, the benefits and risks of AI over the short and long-term, and finally whether superintelligent AI poses an existential risk to humanity. If you’re not currently following this podcast series, you can join us by subscribing on Apple Podcasts, Spotify, Soundcloud, or on whatever your favorite podcasting app is by searching for “Future of Life.” Our last episode was with Sam Harris on global priorities. If that sounds interesting to you, you can find that conversation wherever you might be following us.
I’d also like to echo two announcements for the final time. So, if you’ve been tuned into the podcast recently, you can skip ahead just a bit. The first is that there is an ongoing survey for this podcast where you can give me feedback and voice your opinion about content. This goes a long way for helping me to make the podcast valuable for everyone. This survey should only come out once a year. So, this is a final call for thoughts and feedback if you’d like to voice anything. You can find a link for the survey about this podcast in the description of wherever you might be listening.
The second announcement is that at the Future of Life Institute, we are in the midst of our search for the 2020 winner of the Future of Life Award. The Future of Life Award is a $50,000 prize that we give out to an individual who, without having received much recognition at the time of their actions, has helped to make today dramatically better than it may have been otherwise. The first two recipients of the Future of Life Award were Vasili Arkhipov and Stanislav Petrov, two heroes of the nuclear age. Both took actions at great personal risk to possibly prevent an all-out nuclear war. The third recipient was Dr. Matthew Meselson, who spearheaded the international ban on bioweapons. Right now, we’re not sure who to give the 2020 Future of Life Award to. That’s where you come in. If you know of an unsung hero who has helped to avoid global catastrophic disaster, or who has done incredible work to ensure a beneficial future of life, please head over to the Future of Life Award page and submit a candidate for consideration. The link for that page is on the page for this podcast or in the description of wherever you might be listening. If your candidate is chosen, you will receive $3,000 as a token of our appreciation. We’re also incentivizing the search via MIT’s successful red balloon strategy, where the first to nominate the winner gets $3,000 as mentioned, but there are also tiered pay outs where the first to invite the nomination winner gets $1,500, whoever first invited them gets $750, whoever first invited them $375, and so on. You can find details about that on the Future of Life Award page. Link in the description.
Steven Pinker is a Professor in the Department of Psychology at Harvard University. He conducts research on visual cognition, psycholinguistics, and social relations. He has taught at Stanford and MIT and is the author of ten books: The Language Instinct, How the Mind Works, The Blank Slate, The Better Angels of Our Nature, The Sense of Style, and Enlightenment Now: The case for Reason, Science, Humanism, and Progress.
Stuart Russell is a Professor of Computer Science and holder of the Smith-Zadeh chair in engineering at the University of California, Berkeley. He has served as the vice chair of the World Economic Forum’s Council on AI and Robotics and as an advisor to the United Nations on arms control. He is an Andrew Carnegie Fellow as well as a fellow of the Association for the Advancement of Artificial Intelligence, the Association for Computing Machinery and the American Association for the Advancement of Science.
He is the author with Peter Norvig of the definitive and universally acclaimed textbook on AI, Artificial Intelligence: A Modern Approach. He is also the author of Human Compatible: Artificial Intelligence and the Problem of Control.
And with that, here’s our conversation with Steven Pinker and Stuart Russell.
So let's get started here then. What are the historical and intellectual foundations upon which the ongoing AI revolution is built?
Steven Pinker: I would locate them in the Age of Reason and the Enlightenment, when Thomas Hobbes said, "Reasoning is but reckoning," reckoning in the old-fashioned sense of “calculation” or “computation.” A century later, the two major style of AI today were laid out: The neural network, or massively parallel interconnected system that is trained with examples and generalizes by similarity, and the symbol-crunching, propositional, “Good Old-Fashioned AI.” Both of those had adumbrations during the Enlightenment. David Hume, in the empiricist or associationist tradition, said there are only three principles of connection among ideas, contiguity in time or place, resemblance, and cause and effect. On the other side, you have Leibniz, who thought of cognition as the grinding of wheels and gears and what we would now call the manipulation of symbols. Of course the actual progress began in the 20th century with the ideas of Turing and Shannon and Weaver and Norbert Wiener. The rest is the history that Stuart writes about in his textbook and his recent book.
Stuart Russell: I think I would like to add in a little bit of ancient history as well, just because I think Aristotle not only thought a lot about how human thinking was organized and how it could be correct or incorrect and how we could make rational decisions, he very clearly describes a backward regression goal planner in one of his pieces, and his work was incredibly influential. One of the things he said is we deliberate about means and not about ends. I think he says, "A doctor does not choose whether to heal," and so on. And you might disagree with that, but I think that that's been a pretty influential thread in Western thinking for the last two millennia or more. That we kind of take objectives as given and the purpose of intelligence is to act in ways that achieve your objectives.
That idea got refined gradually. So Aristotle talked mainly about goals and logically provable sequences of actions that would achieve those goals. And then in the 17th and 18th centuries, I want to give a shout out to the French and the Swiss, so Pascal and Fermat and Arnauld and Bernoulli brought in ideas of rational decision making under uncertainty and the weighing of probabilities and the concept of utility that Bernoulli introduced. So that generalized Aristotle's idea, but it didn't change the fundamental principle that they took the objectives, the utilities, as given. Just intrinsic properties of a human being in a given moment.
In AI, we sort of went through the same historical development, except that we did the logic stuff for the first 30 years or so, roughly, and then we did the probability and decision theory stuff for the next 30 years. I think we're in a terrible state now, because the vast majority of the deep learning community, when you read their papers, nothing is cited before 2012. Occasionally, from time to time, they'll say things like, "For this problem, the learning algorithms that we have are probably inadequate, and in future I think we should direct some of our research towards something that we might call reasoning or knowledge," as if no one had ever thought of those things before and they were the first person in history to ever have the idea that reasoning might be necessary for intelligence.
Steven Pinker: Yes.
Stuart Russell: I find this quite frustrating and particularly frustrating when students want to actually just bypass the AI course altogether and go straight to the deep learning course, because they just don't think AI is necessary anymore.
Steven Pinker: Indeed, and also galling to me. In the late '80s and '90s I was involved in a debate over the applicability of the predecessors of deep learning models, then called multi-layer perceptrons, artificial neural networks, connectionist networks, and Parallel Distributed Processing networks. Gary Marcus and Alan Prince and Michael Ullman and other collaborators I pointed out the limitations of trying to achieve intelligence--even for simple linguistic processes like forming the plural of a noun or a past tense of a verb--if the only tool you had available was the ability to associate features with features, without any symbol processing. That debate went on for a couple of decades and then petered out. But then one of the prime tools in the neural network community, multilayer networks trained by error back-propagation, were revived in 2012. Indeed there is an amnesia for the issues in that debate, which Gary Marcus has revived for a modern era.
It would be interesting to trace the truly radical idea behind artificial intelligence: not just that there are rules or algorithms, whether they are from logic or probability theory, that an intelligent agent can use, the way a human pulls out a smartphone. But the idea that there is nothing but rules or algorithms, and that's what an intelligent agent consists of: that is, no ghost to the machine, no agent separate from the mechanism. And there, I'm not sure whether Aristotle actually exorcised the ghost in the machine. I think he did have a notion of a soul. The idea that it's rules all the way down, that intelligence is just a mechanism, probably has shallow roots. Although Hobbes probably could claim credit for it, and perhaps Hume as well.
Lucas Perry: That's an excellent point, Steve, it seems like Abrahamic religions have kind of given rise in part to this belief, or maybe an expression of that belief, the kind of mind-body dualism, the ghost in the machine where the mind seems to be a nonphysical thing. So it seems like intelligence has had to go the same road of "life." There used to be "elan vital" or some other spooky presupposed mechanism for giving rise to life. And so similarly with intelligence, it seems like we've had to move from thinking that there was a ghost in the machine that made the things work to there being rules all the way down. If you guys have anything else to add to that, I think that'd be interesting.
My other two reactions to what has been said so far are that this point about computer science taking the goal as given, I think is important and interesting, and maybe we could expand upon that a little bit. Then there's also, Stuart mentioned the difference between AI and deep learning and that students want to skip the AI and just get straight to the deep learning. That seemed a little bit confusing to me.
Steven Pinker: Let me address the first part and I'll turn it over to Stuart for the second. The notion of dualism--that there is a mechanism, but sitting on top of it is an immaterial agent or self or soul or I--is enshrined in the Abrahamic religions and in other religions, but it has deep intuitive roots. We are all intuitively dualists (Paul Bloom has made this argument in his book Descartes' Baby.) Fortunately, when we deal with each other in everyday life we don't treat each other like robots or wind-up dolls, but we assume that there is an inner life that is much like ours, and we make sense of people's behavior in terms of their beliefs and desires, which we don't conceptualize as neural circuitry transforming patterns. We think there's a locus of consciousness, which is easy to think of as separate from the flesh that we're made of, especially since--and this is a point made by the 19th century British anthropologist Edward Tylor--that there's actually a lot of empirical “evidence” that supports dualism in our everyday life. Like dreaming.
When you dream, you know your body is in bed the whole time, but there's some part of you that's up and about in the world. When you see your reflection in a mirror or in still water, there is an animated essence that seems to have parted company with your body. When you're in a trance from a drug or a fever and have an out-of-body experience, it seems that we and our bodies are not the same thing. And with death, one moment a person is walking around, the next moment the body is lifeless. It's natural to think that it's lost some invisible ingredient that had animated it while it was alive.
Today we know that this is just the activity of the brain, but in terms of the experience available to a person, dualism seems perfectly plausible. It's one of the great achievements of neuroscience, on the one hand, to show that a brain is capable of supporting problem solving and perception and decision making, and of the computational sciences, on the other, for showing that intelligence can be understood in terms of information and computation, and that goals (like the Aristotelian final cause) can be understood in terms of control and cybernetics and feedback.
Stuart Russell: On the point that in computer science, we regard the objectives as fixed, it's much broader than just computer science. If you look at Von Neumann -- Morgenstern and their characterizations of rationality, nowhere do they talk about what is the process by which the agent might rationally come by its preferences. The agent is always assumed a priori to come with the preferences built in, and the only constraint is that those preferences be self-consistent so that you can't be driven around circles of intransitive preferences where you simply cough up money to go round and round the same circle.
The same thing I think is true in control theory, where the objective is the cost function, and you design a controller that minimizes the expected cost function, which might be a square of the distance from the desired trajectory or whatever it might be. Same in statistics, where let's just assume that there's a loss function. There's no discussion in statistics of what the loss function should be or how the loss function might change or anything like that.
So this is something that pervades many of the technological underpinnings of the 20th century. As far as I can tell, to some extent in developmental psychology, but I think in moral philosophy, people really take seriously the question of what goal should we have? Is it moral for an agent to have such and such as its objective, and how could we, for example, teach an agent to have different objectives? And that gets you into some very unchartered philosophical waters about what is a rational process that would lead an agent to have different objectives at the end than it did at the beginning, given that if it has different objectives at the end, then it can only expect that it won't be achieving the objectives that it has at the beginning. So why would it embark on a process that's going to result in failure to achieve the objectives that it currently has?
So that's sort of a philosophical puzzle, but it's a real issue because in fact human beings do change. We're not born with the preferences that we have as adults, and so there is a notion of plasticity that absolutely has to be understood if we're to get this right.
Steven Pinker: Indeed, and I suspect we'll return to the point later when we talk about potential risks of advanced artificial intelligence. The issue is whether a system having intelligence implies that the system would have certain goals, and probably Stuart and I agree the answer is no, at least not by definition. Precisely because what you want and how to get what you want are two logically independent questions. Hume famously said that reason must be that the slave of the passions, by which he didn't mean that we should just surrender to our impulses and do whatever feels good. What he meant was that reason itself can't specify the goals that it tries to bring about. Those are exogenous. And indeed, von Neumann and Morgenstern are often misunderstood as saying that we must be ruthlessly, egotistical self interested maximizers. Whereas the goal that is programmed into us -- say by evolution or by culture -- could include other people's happiness as part of our utility function. That is a question that merely making our choices consistent is silent on.
So the ability to reason doesn't by itself give you moral goals, including taking into account the interests of others. That having been said, there is a long tradition in moral philosophy which shows how it doesn't take much to go from one to the other. Because as soon as we care about persuading others, as soon as our interests depend on how others treat us, then we can't get away with saying “only my interests count and yours don't because I am me and you're not,” because there is no logical difference between “me” and “you.” So we're forced to a kind of impartiality, wherein whatever I insist on for me I've got to grant to you, a kind of Golden Rule or Categorical Imperative that makes our interests interchangeable as soon as we're in discourse with one another.
This is all to acknowledge Stuart's point, but to take it a few steps further in how it deals with the question of what our goals ought to be.
Stuart Russell: The other point you raised Lucas was on being confused by my distinction between AI and deep learning.
Lucas Perry: That's right.
Stuart Russell: I think you're pointing to a confusion that exists in the public mind, in the media and even in parts of the AI community. AI has always included machine learning as a subdiscipline, all the way back to Turing's 1950 paper, where he speculates, in fact, that might be a good way to build AI would be just start with a child program and train it to be an adult intelligent machine. But there are many other sub-fields of AI; knowledge representation, reasoning, planning, decision making under uncertainty, problem solving, perception. Machine learning is relevant to all of these because they all involve processes that can be improved through experience. So that's what we mean by machine learning: simply the improvement of performance through experience; and deep learning is a technology that helps with that process.
It by itself as far as we can tell, doesn't have what is necessary to produce general intelligence. Just to pick one example, the idea that human beings know things seems so self-evident that we hardly need to argue about it. But deep learning systems in a real sense don't know things. They can't usefully acquire knowledge by reading a book and then go out and use that to design a radio telescope, which human beings arguably can. So it seems inevitable that if we're going to make progress, I mean, sure we take the advances that deep learning has offered. Effectively, what we've discovered with deep learning is that you can train more complicated circuits than we previously would have guessed possible using various kinds of stochastic gradient descent, and other tricks.
I think it's true to say that most people would not have expected that you could build a thousand layer network that was 20,000 units wide. So it's got 20 million circuit elements and simply put a signal in one end and some data in the other and expect that you're going to be able to train those 20 million elements to represent the complicated function that you're trying to get it to learn. So that was a big surprise, and that capability is opening up all kinds of new frontiers: in vision, in speech recognition, language, machine translation, and physical control in robots among other things. It's a wonderful set of advances, but it's not the entire solution. Any more than group theory is the entire solution to mathematics. There's lots of other branches of mathematics that are exciting and interesting and important and you couldn't function without them. The same is true for AI.
So I think that we're probably going to see even without further major conceptual advances, another decade of progress in achieving greater understanding of why deep learning works and how to do it better, and all the various applications that we can create using it. But I think if we don't go back and then try to reintegrate all the other ideas of AI, we're going to hit a wall. And so I think the sooner we lose our obsession with this new shiny thing, the better.
Steven Pinker: I couldn't agree more. Indeed, in some ways we have already hit the wall. Any user of Siri or Cortana or a question-answering system has been frustrated by the way they just make associations to individual words and have a shallow understanding of the syntax of the sentence. If you ask Google or Siri, "Can you show me digital music players without a camera?" It'll give you a long list of music players with discussions of their cameras, failing to understand the syntax of “X without Y.” Or, “What are some fast food restaurants nearby that are not McDonald's?” and you get a list of nearby McDonald's.
It's not hard to bump into the limitations of systems that for all their sophistication are being trained on associations among local elements, and can--I agree, surprisingly--learn higher-order combinations of those elements. But despite the name “deep learning,” they are shallow in the sense that they don't build up a knowledge base of what are the objects, and who did what to whom, which they can access through various routes.
Stuart Russell: Yeah. My favorite example, I'm not sure if it's apocryphal, is you say to Siri, "Call me an ambulance," and Siri says, "Okay. From now on I'll call you Ann Ambulance."
Steven Pinker: In Marx Brothers movie, there's the sequence, "Call me a taxi." "Okay. You're a taxi." I don't know if the AI story is an urban legend based on the Marx Brothers movie or whether life is imitating art.
Lucas Perry: Steven, I really appreciate it and liked that point about dualism and intelligence. I think it points in really interesting directions around identity in the self, which we don't have time to get into here. But I did appreciate that.
So moving on ahead here, to what extent do you both see AI systems as achieving intelligence in the same way or not as the human mind does? What kinds of similarities are there or differences?
Stuart Russell: This is a really interesting question and we could spend the whole two hours just talking about this. So by artificial intelligence, I'm going to take it that we mean not deep learning, but the full range of techniques that AI researchers have developed over the years.
So some of them-- for example, logical reasoning were-- developed going back to Aristotle and other Greek philosophers who developed formal logic to model human thinking. So it's not surprising that when we build programs that do logical reasoning, we are in some sense capturing one aspect of human reasoning capability. Then in the '80s, as I mentioned, AI developed reasoning under uncertainty, and then later on refining that with notions of causality as well, particularly in the work of Judea Pearl. The differences are really because AI and cognitive science separated probably sometime in the '60s. I think before that there wasn't really a clear distinction between whether you were doing AI or whether you were doing cognitive science. It was very much the thought that if you could get a program to do anything that we think of as requiring intelligence with a human, then you were in some sense exhibiting a possible theory of how the human does it, or even you would make introspective claims and say, "Look, I've now shown that this theory of intelligence really works."
But fairly soon people said, "Look, this is not really scientific. If you want to make a claim about how the human mind does something, you have to base it on real psychological experimentation with human subjects." And that's distinct from the engineering goal of AI, which is simply to produce programs that demonstrate certain capabilities. So for most of the last 50, 60 years, these two fields have grown further and further apart. I think now partly because of deep learning and partly because of other work, for example in probabilistic programming, we can start to do things that humans do that we couldn't do before. So it becomes interesting again, to ask, well, are humans really somewhat Bayesian and are they doing these kinds of Bayesian symbolic probabilistic program learning that, for example, Josh Tenenbaum was proposing or are they doing something else? For example, Geoff Hinton is pretty adamant that as he puts it, symbols are the luminiferous aether of AI by which he means that they're simply something that we imagined and they have no physical reality whatsoever in the human mind.
I find this a little hard to believe, and you have to wonder if symbols don't exist, why are almost all deep learning applications aimed at recognizing the symbolic category to which an object belongs, and I haven't heard an answer yet from the deep learning community about why that is. But it's also clear that AI systems are doing things that have no resemblance to human cognition. When you look at what AlphaGo is actually doing, part of it is that sort of perception-like ability to look at a position and get a sense, to use an anthropomorphic term, of its potential for winning for white or for black. And perhaps that part is human-like, and actually it's incredibly good. It's probably better at recognizing the potential position directly with no deliberation whatsoever than a human is.
But the other part of what AlphaGo does is completely non-human. It's considering sequences of moves from the current state that run all the way to the end of the game. So part of it is searching in a tree which could go 40 or 50 or possibly more moves into the future. Then from the end of the tree, it then plays a random game all the way to the end and sees who wins that game. And this is nothing like what human beings do. When humans are reasoning about a game like Go or Chess, first of all, we are thinking about it at multiple levels of abstraction. So we're thinking about the liveness of a particular group, we're thinking about control of a particular region of territory on the board. We're thinking, "Well, if I give up control of this territory, then I can trade it for capturing his group over there."
So this kind of reasoning simply doesn't happen in AlphaGo at all. We reason back from goals. In chess you say, "Perhaps I could trap his queen. Let me see if I can come up with a move that blocks his exit for the queen." So we reason backwards from some goals and no chess program and no Go program does that kind of reasoning. The reason humans do this is because the world is incredibly complicated and in different circumstances, different kinds of cognitive processing are efficient and effective in producing good decisions quickly. And that's the real issue for human intelligence, right?
If we didn't have to worry about computation, then we would just set up the giant unknown, partially observable, Markov decision process of the universe, solve it and then we would take the first action in the virtually infinite strategy tree that solves that POMDP. Then we would observe the next percept, we would update all our beliefs about the universe and we would resolve the universe and that's how we would proceed. We would have to do that sort of roughly every millisecond to control the muscles in our body, but we don't do anything like that. All of the different kinds of mental capabilities that we have are deployed in this amazingly fluid way to get us through the complexity of the real world. We are so far away in AI from understanding how to do that, that when I see people say, "We're just going to scale up our deep learning systems by another three orders of magnitude and we'll be more intelligent than humans," I just smile.
Steven Pinker: Yeah. I'd like to complement some of those observations. It is true that in the early days of artificial intelligence and cognitive psychology, they were driven by some of the same players. Herb Simon and Allen Newell can be credited as among the founders of AI and the founders of cognitive psychology. Likewise, Marvin Minsky and John McCarthy. When I was an undergraduate, I caught the tail end of what was called the cognitive revolution. It was exhilarating after the dominance of psychology by behaviorism, which forbade any talk of mentalistic concepts. You weren't allowed to talk about memories or plans or goals or ideas or rules, because they were considered to be unobservable and thus unscientific. Then the concept of computation domesticated those mentalist terms and opened up a huge space of hypotheses. What are the rules by which we understand and formulate sentences?, a project that Noam Chomsky initiated. How can we model human knowledge as a semantic network?, a project that Minsky and Alan Collins and Ross Quillian and others developed. How do we make sense of foresight and planning and problem solving, which Newell and Simon pioneered?
There was a lot of back and forth between AI and cognitive science when they were first exposed to the very idea that intelligence could be understood in mechanistic terms, and there was a flow of hypotheses from computer science that psychologists then tested as possible models. Ideas that you couldn't even frame, you couldn't even articulate before there was the language of computation, such as What is the capacity of human short term memory? or What are the search algorithms by which we explore a problem space? These were unintelligible in the era of behaviorism.
All this caught the attention of philosophers like Hilary Putnam, and later Dan Dennett, who noted that the ideas from the hybrid of cognitive psychology and artificial intelligence were addressing deep questions about what mental entities consist of, namely information processing states. The back-and-forth spilled into the '70s when I was a graduate student, and even the '80s when centers for cognitive science were funded by the Sloan Foundation. There was also a lot of openness in the companies that hired artificial intelligence researchers: AT&T Bell Labs, which was a scientific powerhouse before the breakup of AT&T. Bolt Beranek and Newman in Cambridge, which eventually became part of Verizon. I would go there as a grad student to hear talks on artificial intelligence. I don't know if this is apocryphal history, but Xerox Palo Alto Research Centers, where I was a consultant, was so open that, according to legend, Steve Jobs walked in and saw the first computer with a graphic user interface and a mouse and windows and icons, stole the ideas, and went on to build the Lisa and then the Macintosh. Xerox was out on their own invention, and companies got proprietary . Many of the AI researchers in companies no longer publish in peer-reviewed journals in psychology the way they used to, and the two cultures drifted apart.
Since hypotheses from computer science and artificial intelligence are just hypotheses, there is the question of whether the best engineering solution to a problem is the one that the brain uses. There's the obvious objection that the hardware is radically different: the brain is massively parallel and noisy and stochastic; computers are serial and deterministic. That led in part to the backlash in the '80s when perceptrons and artificial neural networks were revived. There was skepticism about the more symbolic approaches to artificial intelligence, which has been revived now in the deep learning era.
to get back to the question, what are ways in which human minds differ from AI systems? It depends on the AI system assessed, as Stuart pointed out. Both of us would agree that the easy equation of deep learning networks with human intelligence is unwarranted, that a lot of the walls that deep learning is hitting come about because, despite the noisy parallel elements the brain is made of, we do emulate a kind of symbol processing architecture, where we can be taught explicit propositions, and human intelligence does make use of these symbols in addition to massively parallel associative networks.
I can't help but mention a historical irony. I've known Geoff Hinton since we were both post-docs. Hinton himself, early in his career, provided a refutation of the very claim of his that Stuart cited, that symbols are like luminiferous aether, a mythical entity. Geoff and I have noted to each other that we’ve switched sides in the debate on the nature of cognition. There was a debate in the 1970s on the format of mental imagery. Geoff and I were on opposite sides, but he was the symbolic proposition guy and I was the analog parallel network guy.
Hinton showed that our understanding of an object depends on the symbolic format in which we mentally represent it. Take something as simple as a cube, he said. Imagine a cube poised on one of its vertices, with the diagonally opposite vertex aligned above it. If you ask people, “Point to all the other vertices,” they are stymied. Their imagery fails, and they often leave out a couple of vertices. But if, instead of describing it to them as a cube tilted on its diagonal axis, you describe it as two tilted diamonds, one above the other, or as two tripods joined by a zig-zag ring, they “see” the correct answer. Even visualizing an object depends critically on how people mentally describe it to themselves with symbols. This is an argument for symbolic representations that Geoff Hinton made in 1979, and with his recent remarks about symbols he seems to have forgotten his own powerful example.
Stuart Russell: I think another area where deep learning is clearly not capturing the human capacity for learning, is just in the efficiency of learning. I remember in the mid '80s going to some classes in psychology at Stanford, and there were people doing machine learning then and they were very proud of their results, and somebody asked Gordon Bower, "how many examples do humans need to learn this kind of thing?" And Gordon said "one Sometimes two, usually one", and this is genuinely true, right? If you look for a picture book that has one to two million pictures of giraffes to teach children what a giraffe is, you won't find one. Picture books that tell children what giraffes are have one picture of a giraffe, one picture of an elephant, and the child gets it immediately, even though it's a very crude cartoonish drawing, of a giraffe or an elephant, they never have a problem recognizing giraffes and elephants for the rest of their lives.
Deep learning systems are needing, even for these relatively simple concepts, thousands, tens of thousands, millions of examples, and the idea within deep learning seems to be that well, the way we're going to scale up to more complicated things like learning how to write an email to ask for a job, is that we'll just have billions or trillions of examples, and then we'll be able to learn really, really complicated concepts. But of course the universe just doesn't contain enough data for the machine to learn direct mappings from perceptual inputs or really actually perceptual input history. So imagine your entire video record of your life, and that feeds into the decision about what to do next, and you have to learn that mapping as a supervised learning problem. It's not even funny how unfeasible that is. The longer the deep learning community persists in this, the worse the pain is going to be when their heads bang into the wall.
Steven Pinker: In many discussions of superintelligence inspired by the success of deep learning I'm puzzled as to what people could possibly mean. We’re sometimes asked to imagine an AI system that's could solve the problem of Middle East peace or cure cancer. That implies that we would have to train it with 60 million other diseases and their cures, and it would extract the patterns and cure the new disease that we present it with. Needless to say, when it comes to solving global warming, or pandemics, or Middle Eastern peace, there aren't going to be 60 million similar problems with their correct answers that could provide the training set for supervised learning.
Lucas Perry: So, human children and humans are generally capable of one shot learning, or you said we can learn via seeing one instance of a thing, whereas machine learning today is trained up via very, very large data sets. Can you explain what the actual perceptual difference is going on there? It seems for children, they see a giraffe and they can develop a bunch of higher order facts about the giraffe, like that it is tan, and has spots, and a long neck, and horns and other kinds of higher order things. Whereas machine learning systems may be doing something else. So could you explain that difference?
Stuart Russell: Yeah, I think you actually captured it pretty well. The human child is able to recognize the object, not as 20 million pixels, including--let's not forget--all the pixels of the background. So many of these learning algorithms are actually learning to recognize the background, not the object at all. They're really picking up on spurious regularities that happen in the way the images are being captured. But the human child immediately separates the figure from the background says, "okay, it's the figure that's being called a giraffe", and recognizes the higher level properties; "okay, it's a quadruped, relatively large" the most distinguishing characteristic, as you say, is the very long neck, plus the way its hide is colored. Probably most kids might not even notice the horns and I'm not even sure if all giraffes have the horns, or just the males or just the adults. I don't know the answer to that.
So I wasn't paying much attention to all those images. This carries over to many, many other situations, including in things like planning, where if we observe someone carrying out a successful behavior, that one example combined with our prior knowledge is typically enough for us to get the general idea of how to do that thing. And this prior knowledge is absolutely crucial. Just information-theoretically, you can't learn from one example reliably, unless you bring to bear a great deal of prior knowledge. And this is completely absent in deep learning systems in two ways. One is they don't have any prior knowledge. And two is some of the prior knowledge is specifically about the thing you're trying to predict. So here, we're trying to predict the category of an animal and we already have a great deal of prior knowledge about what it means to belong to a category of animals.
So for example, who owns you, is not an attribute that the child would need to know or care about. If you said, what kind of animal is this? And deep learning systems have no ability to include or exclude any input attribute on the basis of its relevance to what it's trying to predict, because they know nothing about what it is you're trying to predict. And if you think about it, that doesn't make any sense, right? If I said, "okay, I want you to learn to predict predicate P1279A. Okay? And I'm going to give you loads and loads of examples." And now you get a perfect predictor for 'P1279A', but you have absolutely no use for it, because P1279A doesn't connect to anything else in your cognition. So you learned a completely useless predictor because you know nothing about the thing that you're trying to predict.
So it seems like it's broken in several really, really important ways, and I would say probably the absence of prior knowledge or any means to bring to bear prior knowledge on the learning process is the most crucial.
Steven Pinker: Indeed, this goes back to our conversation on how basic principles of intelligence that govern the design of intelligent systems provide hypotheses that can be tested within psychology. What Stuart has identified is ultimately the nature-nurture problem in cognition. Namely, what are the innate constraints that govern children's first hypotheses as they try to make sense of the world?
One famous answer is Chomsky's universal grammar, which guides children as they acquire language. Another is the idea from my colleagues Susan Carey and Elizabeth Spelke, in different formulations, that children have a prior concept of a physical object whose parts move together, which persists over time, and which follows continuous spatiotemporal trajectories; and that they have a distinct concept of an agent or mind, which is governed by beliefs and desires. Maybe, or maybe not, they come equipped with still other frameworks for concepts, like the concept of a living thing or the concept of an artifact, and these priors radically cut down the search space of hypotheses, so they don’t have to search at the level of pixels and all their logically possible weighted combinations.
Of course, the challenge in the science is how you specify the innate constraints, the prior knowledge, so that they aren't obviously too specific, given what we know about the plasticity of human cognition. The extreme example being the late philosopher Jerry Fodors' suggestion that all concepts are innate, including “trombone” and “doorknob” and “carburetor.”
Stuart Russell: (Laughs)
Steven Pinker: Hard to swallow, but between that extreme and the deep learning architecture in which the only thing that's innate are the pixels, the convolutional network that allows for translational invariance, and the network of connections, there's an interesting middle ground. That defines the central research question in cognitive development.
Stuart Russell: I don't think you have to believe in extensive innate structures in order to believe that prior knowledge is really, really important for learning. I would guess that some aspects of our cognition are innate, and one of them is probably that the world contains things, and that's really important because if you just think about the brain as circuits, some circuit languages don't have things as first class entities, whereas first order logical languages or programming languages do have things as first class entities and that's a really important distinction.
Even if you believe that nothing is innate, the point is how does everything that you have perceived up to now affect your ability to learn the next thing? One argument is, everything you've perceived up to now, is simply data, and somehow magically, we have access to all our past perceptions, and then you're just training a function from that whole lot to the next thing to do or how to interpret the next object.
That doesn't make much sense. Presumably the experience you have from birth or even pre-birth onwards, is converted into something and one argument is that it's just converted into something like knowledge, and then that knowledge is brought to bear on learning problems, for example, to even decide what are the relevant aspects of the input for predicting category membership of this thing?
And the other view would be that, in the deep learning community, they would say probably something like the accumulation of features. If you imagine a giant recurrent neural network: in the hidden layers of the recurrent neural network over years and years and years of perception, you're building up internal representations, features, which then can perhaps simplify the learning of the next concept that you need to learn. And there's probably some truth in that too.
And absolutely having a library of features that are generally useful for predicting and decision making and planning and our entire vocabulary, I think this is something that people often miss, our vocabulary, our language, is not just something we use to communicate with each other. It's an enormous resource for simplifying the world in the right ways, to make the next thing we need to know, or the next thing we need to do, relatively easy. Right? So you imagine you decide at the age of 12, I want to understand the physical laws that control the universe.
The fact that we have in our vocabulary, something like doing a PhD, makes it much more feasible to figure out what your plan is going to be, to achieve this objective. If you didn't have that, and if you didn't have all the pieces of doing a PhD, like take a course, read a book, this library of words and action primitives, at all these levels of abstraction, is a resource without which you would be completely unable to formulate plans of any length or any likelihood of success. And this is another area where current AI systems, I would say generally, not just deep learning, we lack a real understanding of how to formulate these hierarchies and acquire this vocabulary and then how to deploy it in a seamless way so that we're always managing to function successfully in the real world.
Lucas Perry: I'm basically just as confused about I guess, intelligence as anyone else. So the difference, it seems to me between the machine learning system and the child who one-shot-learns the giraffe is, that the child brings into this learning scenario, this knowledge that you guys were talking about, that they understand that the world is populated by things and that there are other minds and some other ideas about 3D objects and perception, but a core difference seems to be something like symbols and the ability to manipulate symbols is this right? Or is it wrong? And what are symbols and effective symbol manipulation made of?
Steven Pinker: Yes, and that is a limitation of the so-called deep learning systems, which are a subset of machine learning, which is a subset of artificial intelligence. It's certainly not true that AI systems don't manipulate symbols. Indeed, that's what classical AI systems trade in: manipulation of propositions, implementation of versions of logical inference or of cause-and-effect reasoning. Those can certainly be implemented in AI systems--it's just gone out of fashion with the deep learning craze.
Lucas Perry: Well, they don't learn those symbols, right? Like we give them the symbols and then they manipulate them.
Steven Pinker: The basic architecture of the system, almost by definition, can't be learned; you can't learn something with nothing. There have got to be some elementary information processes, some formats of data representation, some basic ways of transforming one representation to another, that are hardwired into the architecture of the system. It's an open empirical question, in the case of the human brain, whether it includes variables for objects and minds, or living things, or artifacts, or if those are scaffolded one on top of the other with experience. There's nothing in principle that prevents AI systems from doing that; many of them do, but at least for now they seem to have fallen out of fashion.
Stuart Russell: There is precedent for generating new symbols, both in the probabilistic programming literature and in the inductive logic programming literature. So predicate invention is a very important reason for doing inductive logic programming. But I agree with Steve, that it's an open question as to whether the basic capacity to have a new symbol based representations in the brain is innate, or is it learned? There's very anecdotal evidence about what happens to children who are not brought up among other human beings. I think those anecdotes suggest that they don't become symbol-using in the same way. So it might be that the process of developing symbol-using capabilities in the brain is enormously aided by the fact that we grow up in the presence of symbol-using entities, namely our parents and family members and community. And of course that leads you to then a chicken and egg problem.
So you'd have to argue in that case that early humans, or pre-humans had much more rudimentary symbol-like capabilities: some animals have the ability to refer to different phenomena or objects with different signs, different kinds of sounds that some new world monkeys have, for example, for a snake and for puma, but they're not able to do the full range of things that we do with symbols. You could argue that the symbol using capability developed over hundreds of thousands of years and the unaided human mind doesn't come with it built in, but because we're usually bathed in symbol-using activity around us, we are able to quickly pick it up. I don't know what the truth is, but it seems very clear that this kind of capability, for example, gives you the ability to generalize so much faster than you can with circuits. So just to give a particular example of the rules of Go, we talked about earlier, the rules of Go apply the same rule at every time-step in the game.
And it's the same rule at every square in the game, except around the edges, and if you have what we call first order capability, meaning you can have universal quantifiers or in programs, we think of these as loops, you can say very quickly for every square on the board, if you have a piece on there and it's surrounded by the enemy, then it's dead. That's sort of a crude approximation to how things work and go, but it's roughly right. In a circuit, you can't say that because you don't have the ability to say for every square. So you have to have a piece of circuit for each square. So you've got 361 copies of the rule in each of those copies has to be learned separately, and this is one of the things that we do with convolutional neural networks.
A convolutional neural network has the universal quantifier over the input space, built into it. So it's a kind of cheating, and as far as we know, the brain doesn't have that type of weight sharing. So the key aspect is not just the physical structure of the convolutional network, which has this repeating local receptive fields on each different part of the retina, so to speak, but that we also insist that weights for each of those local receptive fields are copied across all receptive fields in the retina. So there aren't millions of separate weights that are trained, there's only a few, sometimes even just a handful of weights that are trained and then the code makes sure that those are effectively copied across the entire retina. And the brain. I don't think has any way to do that, so it's doing something else to achieve this kind of rapid generalization.
Lucas Perry: All right. So now with all of this context and understanding about intelligence and its origins today in 2020, AI is beginning to proliferate and is occupying a lot of news cycles. What particular important changes to human society does the rise and proliferation of AI signal and how do you view it in relation to the agricultural and industrial revolutions?
Steven Pinker: I'm going to begin with a meta-answer, which is that we should keep in mind how spectacularly ignorant we are of the future even the relatively near future. Experts at superforecasting studied by Phil Tetlock, pretty much the best in the world, go down to about chance after about five years out. And we know, looking at predictions of the future from the past, how ludicrous they can be, both in underpredicting technological changes and in overpredicting them. A 1993 book by Bill Gates called The Road Ahead said virtually nothing about the internet! And there's a sport of looking at science-fiction movies and spotting ludicrous anachronisms, such as the fact that in 2001: A Space Odyssey they were using typewriters. They had suspended animation and trips to Jupiter, but they hadn't invented the word processor. To say nothing of the social changes they failed to predict, such as the fact that all of the women in the movie were secretaries and assistants.
So we should begin by acknowledging that it is extraordinarily difficult to predict the future. And there's a systematic reason, namely that the future depends not just on technological developments, but also on people's reaction to the developments, and on the reactions to the reactions, and the reactions to the reactions to the reactions. There are seven and a half billion of us reacting, and we have to acknowledge that there's a lot we're going to get wrong.
It's safe to say that a lot of tasks that involve physical manipulation, like stocking shelves and driving trucks, are going to be automated, and societies will have to deal with the possibility of radical changes in employment, and Stuart talks about those in his book. We don't know whether the job market will be flexible enough to create new jobs, always at the frontier of what machines can't yet do, or whether there'll be massive unemployment that will require economic adjustments, such as a universal basic income or government sponsored service.
Less clear is the extent to which high-level decision making, like policy, diplomacy, or scientific hypothesis-testing, will be replaced by AI. I think that’s impossible to predict. Although, closer to the replacement of truck drivers by autonomous vehicles, AI as a useful tool, rather than as a replacement, for human intelligence will explode in science and business and technology and every walk of life.
Stuart Russell: I think all of those things are true. And I agree that our general record of forecasting has been pretty dismal. I am smiling as Steve was talking, because I was remembering Ray Kurzweil recently saying how proud he was that he had predicted the self driving car, I think it was in '96 or '92, something like that, and possibly wasn't aware that the first self driving car was driving on the freeway in 1987, before he even thought to predict that such a thing might happen. If I had to say, in the next decade, if you said, roughly speaking, that what happened in the 2010s was primarily that visual perception became very crudely feasible for machines when it wasn't before.
And that's already having huge impact, including in self-driving cars, I would say that language understanding at least in a simplified sense will become possible in this decade. And I think it'll be a combination of deep learning with probabilistic programming, with Bayesian and symbolic methods. That will open up enormous areas of activity to machines where they simply couldn't go before, and some of that will be very straightforward, job replacement for call center workers. Most of what they do, I think could be automated by systems that are able to understand their conversations. The role of the smart speaker, the Alexa, or Cortana or Siri or whatever will radically change and will enable AI systems to actually understand your life to a much greater extent. One of the reasons that Siri and Cortana or Alexa are not very useful to me is because they just don't understand anything about my life.
The "call me an ambulance" example illustrates that. If I got a text message saying "Johnny's in the hospital with a broken arm", well, if it doesn't understand that Johnny is possibly my cat, or possibly my son, or possibly my great grandfather and does Johnny live nearby, or in my house, or on another continent, then it hasn't the faintest idea of what to do. Or even whether I care. It's only really through language understanding. I doubt that we're going to be filling these things full of first order logic assertions that we will type into our AI system. So it's only through language that it's going to be able to acquire the knowledge that it needs to be a useful assistant to an individual or a corporation. So having that language capability will open up whole new areas for AI to be useful to individuals and also to take jobs from people. And I'm not able to predict what else we might be able to do when there are AI systems that understand language, but it has to have a huge impact.
Lucas Perry: Is there anything else that you guys would like to add in terms of where AI is at right now, where it'll be in the near future and the benefits and risks it will pose?
Stuart Russell: I could point to a few things that are already happening. There's a lot of discussion about the negative impacts on women and minorities from algorithms that inadvertently pick up on biases in society. So we saw the example of Amazon's hiring algorithm that rejected any resume that had the word "woman's" in it. And I think that's serious, but I think the AI community we're still not completely woke, and there's a lot of consciousness raising that needs to happen. But I think technically that problem is manageable, and I think one interesting thing that's occurring is that we're starting to develop an understanding, not just of the machine learning algorithm, but of the socio-technical context in which that machine learning is embedded and modeling that social technical context allows you to predict whether the use of that algorithm will have negative feedback kinds of consequences, or it will be vulnerable to certain kinds of selection bias in the input data, and so on.
Deepfakes surveillance and manipulation, that's another big area, and then something I'm very concerned about is the use of AI for autonomous weapons. This is another area where we fight against media stereotypes. So when the media talk about autonomous weapons, they invariably have a picture of a Terminator. Always. And I tell journalists, I'm not going to talk to you if you put a picture of a Terminator in the article. And they always say, well, I don't have any control over that, that's a different part of the newspaper, but it always happens anyway.
And the reason that's a problem is because then everyone thinks, "Oh, well this is science fiction. We don't have to worry about this because this is science fiction." And you know, I've heard the Russian ambassador to the UN and Geneva say, well, why are we even discussing these things, because this is science fiction, it's 20 or 30 years in the future? Oh, by the way, I have some of these weapons, if you'd like to buy them. The reality is that many militaries around the world are developing these, companies are selling them. There's a Turkish arms company, STM, selling a device, which is basically the slaughterbot from the Slaughterbots movie. So it's a small drone with onboard explosives and they advertise it as capable of tracking and autonomously attacking human beings based on video signatures and/or face recognition.
The Turkish government has announced that they're going to be using those against the Kurds in Syria sometime this year. So we'll see if it happens, but there's no doubt that this is not science fiction, and it's very real. And it's going to create a new kind of weapon of mass destruction, because if it's autonomous, it doesn't need to be supervised. And if it doesn't need to be supervised, then you can launch them by the million, and then you have something with the same effect as a nuclear weapon, but much cheaper, much easier to proliferate with much less collateral damage and all the rest of it.
Steven Pinker: I think in all of these discussions, it's critical to not fall prey to a status-quo bias and compare the hypothetical problems of a future technology with an idealized present, ignoring the real problems with the present we take for granted. In the case of bias, we know that humans are horribly biased. It’s not just that we’re biased against particular genders and ethnic groups and sexual orientations. But inj general we make judgements that can easily be outperformed by even simple algorithms, like a linear regression formula. So we should remember that our benchmark in talking about the accuracies or inaccuracies of AI prediction algorithms has to be the human, and that's often a pretty low bar. When it comes to bias, of course, a system that's trained on a sample that's unrepresentative is not a particularly intelligent system. And going back to the idea that we have to distinguish the goals we want to achieve from the intelligence that achieves them, if our goal is to overcome past inequities, then by definition we don't want to make selections that simply replicate the statistical distribution of women and minorities in the past. Our goal is to rectify those inequities, and the problem in a system that replicates them is not that it's not intelligent enough, but then we've given it the wrong goal.
When it comes to weapons, here too, we've got to compare the potential harm of intelligent weapons systems with the stupendous harm of dumb weapon systems. Aerial bombardment, artillery, automatic weapons, search-and- destroy missions, and tank battles have killed people by the millions. I think there's been insufficient attention to how a battleground that used smarter weapons would compare to what we've tolerated for centuries simply because that's what we have come to accept, though it's being fantastically destructive. What ultimately we want to do is to make the use of any weapons less likely, and as I've written about, that has been the general trend in the last 75 years, fortunately.
Stuart Russell: Yeah, I think there is some truth in that. When I first got the email from Human Rights Watch, so they began a campaign, I think was back in 2013, to argue for a treaty banning autonomous weapons. Human Rights Watch came into existence because of the awful things that human soldiers do. And now they're saying "No, no human soldiers are great, it's the machines we need to worry about." And I found that a little bit odd. To me, the argument about whether the weapons will inadvertently violate humans right in ways that human soldiers don't, or sort of accidentally kill people in ways that we are getting better at avoiding, I don't think that's the issue. I think it's specifically the weapon of mass destruction property that autonomous weapons have that for example machine guns don't.
There's a hundred million or more Kalashnikov rifles in private hands in the world. If all those weapons got up one morning by themselves and started shooting anyone they could see, that would be a big chunk of the human race gone, but they don't do that. Each of them has to be carried by a person. And if you want to put a million of them into the field, you need another 10 million people to feed and train those million soldiers, and to transport them, and protect them, and all that stuff. And that's why we haven't seen very large scale death from all those hundred million Kalashnikovs.
Even carpet-bombing, which I think nowadays would be regarded as indiscriminate and therefore a violation of international law. And I think even during the Second World War, people argued that "No, you can't go and bomb cities." But once the Germans started to do it, then there was escalating rounds of retaliation and people lost all sense of what was a civilized and what was an uncivilized act of war. But even The Blitz against Great Britain, as far as I know killed only between 50 and 60,000 people, even though it hit dozens and dozens of cities. But literally one truckload of autonomous weapons can kill a million people.
An interesting fact about World War II is that for every person who died, between 1,000 and 10,000 bullets were fired. So just killing people with bullets on average in World War II cost you, let's take a geometric mean 3,000 bullets, which is actually about a thousand dollars at current prices, but you could build a lethal autonomous weapon for a lot less than that. And even if they had a 25% success rate in finding and killing a human, it's much cheaper than the bullet, let alone the guns and the aircraft and all the rest of it.
So as a way of killing very, very large numbers of people it's incredibly cheap and incredibly effective. They can also be selective. So you can kill just the kind of people you want to get rid of. And it seems to me that we just don't need another weapon of mass destruction with all of these extra characteristics. We've got rid of to some extent biological and chemical weapons. We're trying to get rid of nuclear weapons, and introducing another one that's arguably much worse seems to be a step in the wrong direction.
Steven Pinker: You asked also about the benefits of artificial intelligence, which I think could be stupendous. They include elimination of drudgery and the boring and dangerous jobs that no one really likes to do, like stocking shelves, making beds, mining coal, and picking fruit. There could be a bonanza in automating all the things that humans want done without human pain and labor and boredom and danger. It raises the problem of how we will support the people (if new jobs don’t materialize) who have nothing to do. But that's a more minor economic problem to solve, compared to the spectacular advance we could have in eliminating human drudgery.
Also, there are a lot of jobs, such as the care of elderly people--lifting them onto toilets, reaching things from upper shelves--that, if automated, would allow more of them to live at home instead of being warehoused in nursing homes. Here, too, the potential for human flourishing is spectacular. And as I mentioned, many kinds of human judgment are so error-prone that they can already be replaced by simple algorithms, and better still if they were more intelligent algorithms. There's the potential of much less waste, much less error, far fewer accidents. An obvious example is the million and a quarter people killed in traffic accidents each year that could be terrifically reduced if we had autonomous vehicles that were affordable and widespread.
Lucas Perry: A core of this is that all of the problems that humanity faces simply require intelligence to solve them, essentially. And if we're able to solve the problem of how to make intelligent machines, then our problems will evermore and continuously become automateable by machine systems. So Stuart, do have you have anything else to add here in terms of existential hope and benefits to compliment what Steve just contributed before we pivot into existential risk?
Stuart Russell: Yeah, there is an argument going around, and I think Mark Zuckerberg said it pretty clearly, and Oren Etzioni and various other people have said basically the same thing. And it's usually put this way, "If you're against AI, then you're against better medical decisions, or reducing medical errors, or safer cars," and so on. And this is, I think, just a ridiculous argument. So first of all, people who are concerned about the risks of AI, are not against AI, right? That's like arguing if you're a nuclear engineer and you're concerned about the possibility of a design flaw that would lead to a meltdown, you're against electricity. No, you're not against electricity. You're just against millions of people dying for no reason, and you want to fix the problem. And the same argument I think is true about those who are concerned about the risk of AI. If AI didn't have any benefits we wouldn't be having this discussion at all. No one would be investing any money, no one would have put their lives and careers into working on the capabilities of AI, and the whole point would be moot.
So of course, AI will have benefits, but if you don't address the risks, you won't get the benefits, because the technology will be rejected, or we won't even have a choice to reject it. And if you look at what happened with nuclear power, I think it's really an object lesson. Nuclear power could and still can produce quite cheap electricity. So I have a house in France and most electricity in France comes from nuclear power, and it's very cheap and very reliable. And it also doesn't produce a lot of carbon dioxide, but because of Chernobyl, the nuclear industry has been literally decimated, by which I mean, reduced by a factor of 10, or more. And so we didn't get the benefits, because we didn't pay enough attention to the risks. The same holds with AI.
So the benefits of AI in the long run I would argue are pretty unlimited, and medical errors and safer cars, that's all nice, but that's a tiny, tiny footnote in what can be done. As Steve already mentioned, the elimination of drudgery and repetitive work. It's easy for us intellectuals to talk about that. We've never really engaged in a whole lot of it, but for most of the human race, for most of recorded history, people with power and money have used everybody else as robots to get what they want. Whether we've been using them as military robots, or agricultural robots, or factory robots, we've been using people as robots.
And if you had gone back to the early hunter gatherer days and written some science fiction, and you said, "You know what, in the future, people will go into big square buildings, thousands of feet long with no windows and they'll do the same thing a thousand times a day. And then they'll go back the next day and do the same thing another thousand times. And they're going to do that for thousands of days until they're practically dead." The audience, the readers of science fiction in 20,000 BC, would have said, "You're completely nuts, that's so unrealistic." But that's how we did it. And now we're worried that it's coming to an end, and it is coming to an end, because we finally have robots that can do the things that we've been using human robots to do.
And I'm not saying we should just get rid of those jobs, because jobs have all kinds of purposes in people's lives. And I'm not a big fan of UBI, which says basically, "Okay, we give up. Humans are useless, so the machines will feed them and house them, entertain them, but that's all they're good for."
Now the benefits to me... It's hard to imagine, just like we could not imagine very well all the things we would use the internet for. I mean, I remember the Berkeley computer science faculty in the '80s sitting around at lunch, we knew more about networking than almost anybody else, but we still had absolutely no idea. What was the point of being able to click on a link? What's that about? We totally blew it.
And we don't understand all the things that superhuman AI could do for us. I mean, Steve mentioned that we could do much better science, and I agree with that. In the book, I visualize it as taking various ideas, like, "travel as a service," and extending that to "everything as a service." So travel as a service is a good example. Like if you think about going to Australia 200 years ago, you're talking about a billion dollar proposition, probably 10 years, thousands of people, 80% chance of death. Now I take out my cell phone, I go tap, tap, tap, and now I'm in Australia tomorrow. And it's basically free compared to what it used to be. So that's what I mean by, as a service, you want something, you just get it.
Superhuman AI could make everything as a service. So think about the things that are expensive and difficult or impossible now, like training a neurosurgeon, or building a railway to connect your rural village to a nearby city so that people can visit, or trade, or whatever. For most of the developing world these things are completely out of reach. The health budget of a lot of countries in Africa is less than $10 per person per year. So the entire health budget of a country would train one neurosurgeon in the US. So these things are out of reach, but if you take out the humans then these services can become effectively free. They become services like travel is today, and that would enable us to bring everyone on earth up to the kind of living standard that they might aspire to. And if we can figure out the resource constraints and so on that will be a wonderful thing.
Lucas Perry: Now that's quite a beautiful picture of the future. There's a lot of existential hope there. The other side to existential hope is existential risk. Now this is an interesting subject, which Steve and you, Stuart, I believe have disagreements about. So pivoting into this area, and Steve, you can go first here, do you believe that human beings, should we not go extinct in the meantime, will we build artificial superintelligence? And does that pose an existential risk to humanity?
Steven Pinker: Yeah, I'm on record as being skeptical of that scenario and dubious about the value of putting a lot of effort into worrying about it now. The concept of superintelligence is itself obscure. In a lot of the discussions you could replace the word “superintelligence” with “magic” or “miracle” and the sentence would read the same. You read about an AI system that could duplicate brains in silicon, or solve problems like war in the Middle East, or cure cancer. It's just imagining the possibility of a solution and assuming that the ability to bring it about will exist, without laying out what that intelligence would consist of, or what would count as a solution to the problem.
So I find the concept of superintelligence itself a dubious extrapolation of an unextrapolable continuum, like human-to-animal, or not-so-bright human-to-smart-human. I don't think there is a power called “intelligence” such that we can compare a squirrel or an octopus to a human and say, "Well, imagine even more of that."
I'm also skeptical about the existential risk scenarios. They tend to come in two varieties. One is based on the notion of a will to power: that as soon as you get an intelligent system, it will inevitably want to dominate and exploit. Often the analogy is that we humans have exploited and often extinguished animals because we're smarter than them, so as soon as there is an artificial system that's smarter than us, it'll do to us what we did to the dodos. Or that technologically advanced civilizations, like European colonists and conquistadors subjugated and sometimes wiped out indigenous peoples, so that's what an AI system might do to us. That's one variety of this scenario.
I think that scenario confuses intelligence with dominance, based on the fact that in one species, Homo sapiens, they happen to come bundled together, because we came about through natural selection, a competitive process driven by relative success at capturing scarce resources and competing for mates, ultimately with the goal of relative reproductive success. But there's no reason that a system that is designed to pursue a goal would have as its goal, domination. This goes back to our earlier discussion that the ability to achieve a goal is distinct from what the goal is.
It just so happens that in products of natural selection, the goal was winning in reproductive competition. For an artifact we design, there's just no reason that would be true. This is sometimes called the orthogonality thesis in discussions of existential risk, although that’s just a fancy-schmancy way of referring to Hume's distinction between our goals and our intelligence.
Now I know that there is an argument that says, “Wouldn't any intelligence system have to maximize its own survivability, because if it's given the goal of X, well, you can't achieve X if you don't exist, therefore, as a subgoal to achieving X, you've got to maximize your own survival at all costs.” I think that's fallacious. It's certainly not true that all complex systems have to work toward their own perpetuation. My iPhone doesn't take any steps to resist my dropping it into a toilet, or letting it run out of power.
You could imagine if it could be programmed like a child to whine, and to cry, and to refuse to do what it's told to do as its power level went down. We wouldn't buy one. And we know in the natural world, there are plenty of living systems that sacrifice their own existence for other goals. When a bee stings you, its barbed stinger is dislodged when the bee escapes, killing the bee, but because the bee is programmed to maximize the survivability of the colony, not itself, it willingly sacrifices itself. So it is not true that by definition an intelligent system has to maximize its own power or survivability.
But the more common existential threat scenario is not a will to power but collateral damage. That if an AI system is given a single goal, what if it relentlessly pursues it without consideration of side effects, including harm to us? There are famous examples that I originally thought were spoofs, but were intended seriously, like giving an AI system the goal of making as many paperclips as possible, and so it converts all available matter into paperclips, including our own bodies (putting aside the fact that we don't need more efficient paperclip manufacturing than what we already have, and that human bodies are a pretty crummy source of iron for paperclips).
Barely more plausible is the idea that we might give an AI system the goal of curing cancer, and so it will conscript us as involuntary guinea pigs and induce tumors in all of us, or that we might give it the goal of regulating the level of water behind a dam and it might flood a town because it was never given the goal of not drowning a village.
The problem with these scenarios is that they're self-refuting. They assume that an “intelligent” artifact would be designed to implement a single goal, which is not true of even the stupid artifacts that we live with. When we design a car, we don't just give the goal of going from A to B as fast as possible; we also install brakes and a steering wheel and a muffler and a catalytic converter. A lot of these scenarios seem to presuppose both idiocy on the part of the designers, who would give a system control over the infrastructure of the entire planet without testing it first to see how it worked, and an idiocy on the part of the allegedly intelligent system, which would pursue a single goal regardless of all the other effects. This does not exist in any human artifact, let alone one that claims to be intelligent. Giving an AI system one vaguely worded, sketchy goal, and empowering it with control over the entire infrastructure of the planet without testing it first seems to me just so self-evidently moronic that I don't worry that engineers have to be warned against it.
I've quoted Stuart himself, who in an interview made the point well when he said, "No one talks about building bridges that don't fall down. They just call it building bridges." Likewise, AI that avoids idiocies like that is just AI, it's not AI with extra safeguards. That's what intelligence consists of.
Let me make one other comment. You could say, well, even if the odds are small, the damage would be so catastrophic that it is worth our concern. But there are downsides to worrying about existential risk. One of them is the possible stigmatization and abandonment of helpful technologies. Stuart mentioned the example of nuclear power. What’s catastrophic is that we don't roll out nuclear power the way that France did, which would go a long way toward solving the genuinely dangerous problem of climate change. Fear of nuclear power has been irrationally stoked by vivid examples:the fairly trivial accident at Three Mile Island in the United States, which killed no one, the tsunami at Fukushima, where people died in the botched evacuation, not the nuclear accident, and the Soviet bungling at Chernobyl. Even that accident killed a fraction of the people that die every day from the burning of fossil fuels, to say nothing of the likely future harm from climate change. The reaction to Chernobyl is exactly how we should not deal with the dangers facing humanity.
Genetically modified organisms are another example: a technology overregulated or outlawed out of worst-case fears, depriving us of the spectacular benefits of greater ecological sustainability, human nutrition, and less use of water and pesticides.
There are other downsides of fretting about exotic hypothetical existential risks. There is a line of reasoning in the existential risk community and the so called Rationality community that goes something like this: since the harm of extinguishing the species is basically infinite, probabilities no longer matter, because by expected utility calculations, if you multiply the tiny risk by the very large number of the potential descendants of humans before the sun expands and kills us off (or in wilder scenarios, the astronomically larger number of immortal consciousnesses that will exist when we can upload our connectomes to the cloud, or when we colonize and multiply in other solar systems)---well, then even an eensy, eensy, infinitesimal probability of extinction would be catastrophic, and we should worry about it now.
The problem is that that argument could apply to any scenario with a nonzero probability, which means any scenario that is not logically impossible. Should we take steps to prevent the evolution of toxic killer gerbils that will nibble everyone to death? If I say, “That's preposterous,” you can say, “Well, even if the probability is very, very small, since the harm of extinction is so great, we must devote some brain power to that scenario.”
I do fear the moral hazard of human intellect being absorbed in this free-for-all: that any risk, if you imagine it's potentially existential, could justify any amount of expenditure, according to this expected utility calculation. The hazard is that smart people, clever enough to grasp a danger that common sense would never conceive of, will be absorbed into what might be a fruitless pursuit, compared to areas where we urgently do need application of human brain power--in climate, in the prevention of nuclear war, in the prevention of pandemics. Those are real risks, which no one denies, and we haven't solved any of them, together with other massive sources of human misery like Alzheimer's disease. Given these needs, I wonder whether the infinitesimal-probability-times-infinite-harm is the right way of allocating our intellectual capital.
Lucas Perry: Stuart, you want to react to those points.
Stuart Russell: Yeah, there's a lot there to react to and I'm tempted to start at the end and work back and just ask, well, if we were spending hundreds of billions of dollars a year to breed billions of toxic killer gerbils, wouldn't you ask people if that was a good idea before dismissing any reason to be concerned about it? If that's what we were actually investing in creating. I don't buy the analogy between AI and toxic killer gerbils in any shape or form. But I will go back to the beginning, and we began by talking about feasibility. And Steve argues, I think, primarily, that it's not even meaningful, that we could create superhuman levels of intelligence, that there isn't a single continuum.
And yes, there isn't a single continuum, but there doesn't have to be a single continuum. When people say one person is more intelligent than another, or one species is more intelligent than other, it's not a scientific statement that there is a single scalar on which species one exceeds species two. They're talking in broad brush. So when we say humans are more intelligent than chimpanzees, that's probably a reasonable thing to say, but there are clearly dimensions of intelligence where actually chimpanzees are more intelligent than humans. For example, short term memory. A chimpanzee, once they get what a digit is, they can learn 20 digit telephone numbers at the drop of a hat, and humans can't do that. Clearly there's dimensions on which chimpanzee intelligence, on average, is probably better than human. But nonetheless, when you look at which species would you rather be right now the chimpanzees don't have much of a chance against the humans.
I think that there is a meaningful motion of generality of intelligence, and one way to think about it is to take a decision making scenario where we already understand how to produce very effective decisions, and then ask, how is that decision scenario restricted, and what happens when we relax the restrictions and figure out how to maintain the same, let's say, superhuman quality of decision making? So if you look at Go play, it's clear that the humans have been left far behind. So it's not unreasonable to ask, just as the machines wiped the floor with humans on the Go board, and the chess board, and now on the StarCraft board, and lots of other boards, could you take that and transfer that into the real world where we make decisions of all kinds? The difference between the Go board and the real world is pretty dramatic. And that's why we've had lots of success on the Go board and not so much in the real world.
The first thing is that the Go board is fully observable. You can see the entire state of the world that matters. And of course in the real world there's lots of stuff you don't see and don't know. Some of it you can infer by accumulating information over time, what we call state estimation, but that turns out to be quite a difficult problem. Another thing is that we know all the rules of Go, and of course in the real world, you don't know all the rules, you have to learn a lot as you go along. Another thing about the Go board is that despite the fact that we think of it as really complicated, it's incredibly simple compared to the real world. At any given time on the Go board there's a couple of hundred legal moves, and the game lasts for a couple hundred moves.
And if you said, well, what are the analogous primitive actions in the real world for a human being? Well, we have 600 muscles and we can actuate them maybe about 10 times per second each. Your brain probably isn't able to do that, but physically that's what could be your action space. And so you actually have then a far greater action space. And you're also talking about... We often make plans that last for many years, which is literally trillions of primitive actions in terms of muscle actuations. Now we don't plan those all out in detail, but we function on those kinds of timescales. Those are some of the ways that Go and the real world differ. And what we do in AI is we don't say, okay, I've done Go, now I'm going to work on suicide Go, and now I'm going to work on chess with three queens.
What we try to do is extract the general lessons. Okay, we now understand fairly well how to handle that whole class of problems. Can we relax the assumptions, these basic qualitative assumptions about the nature of the problem? And if you relax all the ones that I listed, and probably a couple more that I've got, you're getting towards systems that can function at a superhuman level in the real world, assuming that you figure out how to deal with all those issues. So just as we find ourself flummoxed by the moves that the AI system makes on the Go board, if you're a General, and you're up against an AI system that's controlling, or coming up with the decision making plans for the other side, you might find yourself flummoxed, that everything you try, the machine has already anticipated and put in place something that will prevent your plan from succeeding. The pace of warfare will be beyond anything humans have ever contemplated, right?
So they won't even have time to think, just as the Iraqis were not used to the rate of decision making of the US Army in the first Gulf War, and they couldn't do anything. They were literally paralyzed, and just step by step by step the allied forces were able to take them apart because they couldn't respond within the timescales that the allied forces were operating.
So it will be kind of like that if you were a human general. If you were a human CEO and your competitor company is organized and run by AI systems, you'd be in the same kind of situation. So it's entirely conceivable. I'm not necessarily saying plausible, but conceivable that we can create real world decision making capabilities that exceed those of humans across the board. So that this notion of generality, I think it is something that still needs to be worked out. Most definitions of generality that people come up with end up saying, "Well, humans are general because they can do all the things that humans can do," which is sort of a tautology. But nonetheless, it's interesting that when you think about all the jobs: doctor, carpenter, advertising, sales, representative, most normally functioning people could do most of those jobs at least to some reasonable level.
So we are incredibly flexible compared to current AI systems. There is progress on achieving generality, but there's a long way to go. I'm certainly not one of those who says that superintelligent AI is imminent and that's why we need to worry. And in fact, I'm probably more conservative. If you want to appeal to what most expert AI people think, most expert AI people think that we will have something that's reasonably described as superintelligent AI sooner than I do.
So most people think sometime in the middle of the century. It turns out that Asian AI researchers particularly in China are more optimistic, so they think 20 years. People in the US and Europe may be more like 40 years. I would be reasonably confident saying by the end of this century.
I think Nick Bostrom is in about the same place. He's also more conservative than the average expert AI researcher. There are major breakthroughs that have to happen, but the massive investment that's taking place, the influx of incredibly smart people into the field, these things suggest that those breakthroughs will probably take place but the timescale is very hard to say.
And when we think about the risks, I would say Steve is really putting up one straw man after another and then knocking it down. So for example, the paperclip argument is not a scenario that Nick Bostrom thinks is one of the more likely ways for the human race to end. It's a philosophical thought experiment intended to illustrate a point. And the point is incontrovertible and I don't think Steve disagrees with it.
So let's not use the word intelligent because I think Steve here is using the word intelligent to mean always behaves in whatever way we think we wish that it would behave well.
Of course, if you define intelligence that way, then there isn't an issue. The question is, how do we create any such thing? And the ways we have right now of creating any such thing fall under the standard model, which I described earlier that we set up, let's call it a superoptimizer and then we give it an objective. And then off it goes. And he's (Bostrom) describing what happens when you give a superoptimizer the wrong goal. And he's not saying, "Yes, of course we should give it wrong goals."
And he's using this to illustrate what happens when you give it even what seems to be innocuous. So he's trying to convey the idea that we are not very good at judging the consequences of seemingly innocuous goals. My example of curing cancer: "Curing cancer? Yeah, of course, that's a good goal to give to an AI system" -- but the point is, if that's the only goal you give to the AI system, then all these weird things happen because that's the nature of super optimizers. That's the nature of the standard model of AI.
And this is, I think, the main point being made is not that no matter what we do AI going to get us. It's that given our current understanding and given hundreds of billions of dollars are being invested into that current understanding then there is a failure mode and it's reasonable to point that out just as if you're a nuclear engineer and you say, "Look, everyone is designing these reactors in this way. All of you are doing this. And look there's this failure mode." That's a reasonable thing to point out.
Steven Pinker: Several reactions. First, while money is pouring into AI, it’s not pouring it into super-optimizers tasked with curing cancer and with the power to kidnap people. And the analogies of humans outcompeting chimpanzees, or American generals outsmarting their Iraqi counterparts, once again assume that systems that are smarter than us will therefore be in competition with us. As for straw men, I was mindful to avoid them: the AI system that would give people tumors to pursue the goal of curing cancer was taken from Stuart’s book.
I agree that a super-optimizer that was given a single goal would be menace. But a super-optimizer that pursued a single goal is self-evidently unintelligent, not superintelligent!
Stuart Russell: Of course, we have multiple goals. There's a whole field of multi-attribute utility theory that's been going now for more than 50 years. Of course, we understand that. When we look at even the design of the algorithms that Uber uses to get you to the airport, they take into account multiple goals.
But the point is the same argument applies if you operate in a standard model when you add in the multiple goals. Unless you're able to be sure that you have completely and correctly captured all the things we care about under all conceivable and the inconceivable,because I think one of the things about superintelligent AI systems they will come up with, by human standards, inconceivable forms of actions.
We cannot guarantee that. And this is the point. So you could say multiple goals, but multiple goals are just a single goal. They add up to the ability to rank futures. And the question is: is that ability to rank futures fully aligned with what humans want their futures to be like? And the answer is inevitably, no. We are inevitably going to leave things out.
So even if you have a thousand terms in the objective function, there's probably another million that you ought to have included that you didn't think about because it never occurred to you.
So for example, you can go out and find lists of important things that human beings care about. This is sort of the whole-values community, human-development community, Maslow hierarchy, all of those things. People do make whole lists of things trying to build up a picture of very roughly what is the human utility function after all.
But invariably, those lists just refer to things that are usually a subject of discussion among humans about "Do we spend money on schools or hospitals?"or whatever it might be. On that list, you will not find the color of the sky because no one, no humans right now are thinking about, "Oh, should we change the sky to be orange with pink stripes?" But if someone did change the color of the sky, I can bet you a lot of people would be really upset about it.
And so invariably we fail to include many, many criteria in whatever list of objectives you might come up with. And when you do that, what happens is that the optimizer will take advantage of those dimensions of freedom and typically, and actually under fairly general algebraic conditions, will set them to extreme values because that gives you better optimization on the things that are in the list of goals.
So the argument is that within the standard model, which I bear some responsibility for, because it's the way we wrote the first three editions of the textbook, within the standard model, further progress on AI could lead to increasing problems of control and it's not because there's any will to dominance.
I don't know of any serious thinkers in the X-risk community who think that that's the problem. That's another straw man.
Steven Pinker: When you're finished, I do have some responses to that.
Stuart Russell: The argument is not that we automatically build in because we all want our systems to be alpha males or anything like that. And I think Steve Omohundro has put it fairly clearly in some of his earlier papers that the behavior of a superoptimizer given any finite list of goals is going to include efforts to maximize its computational resources and other resources that will help it achieve the objectives that we do specify.
And you could put in something saying, "Well, and don't spend any money." Or, "Don't do this and don't do that and don't do the other." But the same structure of the argument is going to apply. We can reduce the risk by adding more and more stuff into the explicit objectives, but I think the argument I'm making in the book is that that's just a completely broken way to design AI systems.
The meta argument is that if we don't talk about the failure modes, we won't be able to address them. So actually I think that Steve and I don't disagree about the plausible future evolution. I don't think it's particularly plausible. If I was going into forecast mode, so just betting on the future saying, "What's the probability that this thing will happen or that thing will happen?" I don't think it's particularly plausible that we will be destroyed by superintelligent AI.
And there are several reasons why I don't think that's going to occur because we would probably get some early warnings of it. And if we couldn't figure out how to prevent it, we would probably put very strong restrictions on further development or we would figure out how to actually make it provably safe and beneficial.
But you can't have that discussion unless you talk about the failure modes. Just like in nuclear safety, it's not against the rules to raise possible failure modes like what if this molten sodium that you're proposing should flow around all these pipes? What if it ever came into contact with the water that's on the turbine side of the system? Wouldn't you have a massive explosion which could rip off the containment and so on? That's not exactly what happened in Chernobyl, but not so dissimilar.
And of course that's what they do. So this culture of safety that Steve talks about consist exactly of this. People saying, "Look, if you design things that way these terrible things are going to happen. So don't design things that way, design things this way." And this is a process that we are going through in the AI community right now.
And I have to say, I just actually was reading a letter from one of my very senior colleagues, former president of AAAI, who said, "Five years ago, everyone thought Stuart was nuts, but Stuart was right. These risks have to be taken seriously and we all owe him a great debt for bringing it within the AI community so that we can start to address it."
And I don't think I invented these risks. And I was just in chance position that I had two years of sabbatical to think about the future of the field and to read some of the things that others had already written about the field from the outside.
My sense is that Steve and I are kind of the glass half full glass half empty. In terms of our forecast, we think on the whole, the weather tomorrow is likely to be sunny. I think we disagree on how to make sure that it's sunny. I really do think that the problem of creating a provably beneficial AI, by which I mean that no matter how powerful the AI system is, we remain in power. We have power over it forever, that we never lose control.
That's a big ask and the idea that we could solve that problem without even mentioning it, without even talking about it and without even pointing out why it's difficult and why it's important, that's not the culture of safety. That's sort of more like the culture of the communist party committee in Chernobyl, that simply continued to assert that nothing bad was happening.
Steven Pinker: Obviously, I'm in favor of the safety mindset of engineering, that is, you test the system before you implement it, you try to anticipate the failure modes. And perhaps I have overestimated the common sense of the AI community and they have to be warned about the absurdity of building a superoptimizer. But a lot of these examples--flooding a town to control the water level, or curing cancer by turning humans into involuntary Guinea pigs, or maximizing happiness by injecting everyone with a drip of antidepressants--strike me as so far from reasonable failure modes that they're not part of the ordinary engineering effort to ensure safety--particularly when they are coupled with the term “existential.”
These are not ordinary engineering discussions of ways in which a system could fail; they are speculations on how the human species might end. That is very different from not plugging in an AI system until you've tested it to find out how it fails. And perhaps we agree that the superoptimizers in these thought experiments are so unintelligent that no one will actually empower them.
Stuart Russell: But Steve, I wasn't saying we give it one goal. I'm saying however many goals we give it, that's equivalent to giving it a ranking over futures. So the idea of single goal versus multiple is a complete red herring.
Steven Pinker: But the scare stories all involve systems that are given a single goal. As you go down the tail of possible risks, you're getting into potentially infinitesimal risks. There is no system, conceivable or existing, that will have zero risk of every possibility.
Stuart Russell: If we could do that, if we had some serious theory by which we could say, "Okay. We've got within epsilon of the true human ranking over futures," I think that's very hard to do. We literally do not have a clue how to do that. And the purpose of these examples is actually to dismiss the idea that this has a simple solution.
So people want to dismiss the idea of risk by saying, "Oh, we'll just give the AI system such and such objective." And then the failure mode goes away and everything's cool. And then people say, "Oh, but no." Look, if you give it the objective that everyone should be happy, then here's a solution that the AI system could find that clearly we wouldn't want.
Those processes lead actually to deeper questioning, what do we really mean by happy? We don't just mean pleasure as measured by the pleasure center in the brain. And the same arguments happen in moral philosophy.
So no one is accusing G.E. Moore of being a naive idiot because he objected to a pleasure maximization definition of what is a good moral decision to make. He was making an important philosophical point and I don't think we should dismiss that same point when it's made in the context of designing objectives for AI systems.
Steven Pinker: Yes, that’s an excellent argument against building a universally empowered AI system that's given the single goal of maximizing human happiness--your example. Do AI researchers need to be warned against that absurd project? It seems to me that that's the straw man, and so are the other scenarios that are designed to sow worry, such as conscripting the entire human race as involuntary guinea pigs in cancer experiments. Even if there isn't an epsilon that we can't go below in laying out possible risks, it doesn't strike me that that's within the epsilon.
These strike me more as exercises of human imagination. Assuming a ridiculously simple system that's given one goal, what could go wrong? Well, yeah, stuff could go wrong, but is that really what's going to face us when it comes to actual AI systems that have some hope of being implemented?
Naturally, we ought to test the living daylights out of any system before we give it control over anything. That's Stuart’s point about building bridges that don't fall down and the standard safety ethic in engineering. But I'm not sure that exotic scenarios based on incredibly stupid ideas for AI systems like giving one the goal of maximizing human happiness is the route that gives us safe AI.
Stuart Russell: Okay. So let me say once again that the one goal versus multiple goals is a red herring. If you think it's so easy to specify the goal correctly, perhaps your next paper will write it out. Then we'll say, "Okay, that's not a straw man. This is Steve Pinker's suggestion of what the objective should be for the superintelligent AI system." And then the people who love doing these things, probably Nick Bostrom and others will find ways of failing.
So the idea that we could just test before deploying something that is significantly more powerful than human beings or even the human race combined, that's a pretty optimistic idea. We're not even able to test ordinary software systems right now. So test generation is one of the effective methods used in software engineering, but it has many, many known failure cases for real world examples, including multiplication.
Intel's Pentium chip was tested with billions of examples of multiplication, but it failed to uncover a bug in the multiplication circuitry, which caused it to produce incorrect results in some cases. And so we have a technology of formal verification, which would have uncovered that error, but particularly in the US there's a culture that's somewhat opposed to using formal verification in software design.
Less so in hardware design nowadays, partly because of the Pentium error, but still in software, formal verification is considered very difficult and very European and not something we do. And this is far harder than that because software verification typically is thinking only about correctness of the software in an internal sense, that what happens inside the algorithm between the inputs and outputs meet some specifications.
What we want here is that the combination of the algorithm and the world evolves in ways that we are certain to be pleased about. And that's a much harder kind of thing. Control theory has that view of what they mean by verification. And they're able to do very simple linear quadratic regulators and a few other examples. And beyond that, they get stuck. And so I actually think that the testing is probably neither (not very) feasible. I mean, not saying we shouldn't do it, but it's going to be extremely hard to get any kind of confidence from testing, because you're really asking, can you simulate the entire world and all the ways a system could use the world to bring about the objective.
However complicated and however multifaceted that objective is, it's probably going to be the wrong one. So I've proposed a, not completely different, but a generalized form of AI that knows that it doesn't know what the real objectives are. It knows it doesn't know how humans rank possible futures and that changes the way it behaves, but that also has failure modes.
One of them being the plasticity of human preference rankings over the future and how do you prevent the AI system from taking advantage of that plasticity? You can't prevent it completely because anything it does is going to have some effect on human preferences. But the question is what constitutes reasonable modifications of human preferences and what constitutes unreasonable ones? We don't know the answer to that. So there are many, many really difficult research problems that we have to overcome for the research agenda that I'm proposing to have a chance of success.
I'm not that optimistic that this is an easy or a straightforward problem to solve and I think we can only solve it if we go outside the conceptual framework that AI has worked in for the last 70 years.
Steven Pinker: Well, yes. Certainly, if the conceptual framework for AI is optimizing some single or small list of generic goals, like a ranking over possible futures, and it is empowered to pursue them by any means, as opposed to building tools that solve specific problems. But note that you've also given arguments why the fantasies of superintelligence are unlikely to come about--the near-miraculous powers to outsmart us, to augment its own intelligence, to defeat all of our attempts to control it. In the scenarios, these all work flawlessly--yet the complexities that make it hard to predict all conceivable failures also make it hard to achieve superintelligence in the first place.
Namely, we can't take into account the fantastically chaotic and unpredictable reactions of humans. And we can't program a system that has complete knowledge of the physical universe without allowing it to do experiments and acquire empirical knowledge, at a rate determined by the physical world. Exactly the infirmities that prevent us from exploring the entire space of behavior of one of these systems in advance is the reason that it's not going to be superintelligent in the way that these scenarios outline.
And that's a reason not to empower any generic goal-driven system that aspires toward “superintelligence” or that we might think of as “superintelligent”--it is unlikely to exist, and likely to display various forms of error and stupidity.
Stuart Russell: I would agree that some of the concerns that you might see in the X-risk community are, say, nonphysical. So the idea that a system could predict the next hundred years and your entire life in such detail that a hundred years ago, it knew what you were going to be saying at a particular millisecond in a hundred years in the future, this is obviously complete nonsense.
I don't think we need to be too concerned about that as a serious question. Whether it's a thought experiment that sheds light on fundamental questions in decision theory, like the Newcomb problems is another issue that we don't have to get into. But we can't solve the problem by saying, "Well, superintelligence of the kind that could lead to significant global consequences could not possibly exist."
And actually I kind of like Danny Hillis' argument, which says that actually, no, it already does exist and it already has, and is having significant global consequences. And his example is to view, let's say the fossil fuel industry as if it were an AI system. I think this is an interesting line of thought, because what he's saying basically and -- other people have said similar things -- is that you should think of a corporation as if it's an algorithm and it's maximizing a poorly designed objective, which you might say is some discounted stream of quarterly profits or whatever. And it really is doing it in a way that's oblivious to lots of other concerns of the human race. And it has outwitted the rest of the human race.
So we might all think, well, of course, we know that what it's trying to do is wrong and of course we all know the right answer, but in fact we've lost and we should have pointed out a hundred years ago that there is this risk and it needs to be taken seriously.
And it was. People did point it out a hundred years ago, but no one took them seriously. And this is what happened. So I think we have actually a fairly good example that this type of thing, the optimization of objectives, ignoring externalities as the economists would point out, by superintelligent entities. And in some sense, the fossil fuel industry outwitted us because whatever organizational structures allow large groups of humans to generate effective complex behaviors in the real world and develop complex plans, it operates in some ways like a superintelligent entity, just like we were able to put a person on the moon because of the combined effect of many human intellects working together.
But each of those humans in the fossil fuel industry is a piece of an algorithm if you like and their own individual preferences about the future don't count for much and in fact, they get molded by their role within the corporation.
I think in some ways you already have an existence proof that the concern is real.
Steven Pinker: A simpler explanation is that people like energy, fossil fuels are the most convenient source, and no one has had to pay for the external damage they do. Clearly we ought to anticipate foreseeable risks and attempt to mitigate them. But they have to be calibrated against what we know, taking into account our own ignorance of the future. It can be hazardous to chase the wrong worries, such as running out of petroleum, which was the big worry in the 1970s. Now we know that the problem with petroleum is too much, not too little. Overpopulation and genetically modified organisms are other examples.
If we try to fantasize too far into the future, beyond what we can reasonably predict, we can sow fear about the wrong risks. My concern about all these centers and smart people worrying about the existential risk of AI is that we are misallocating our worry budget and our intellectual resources. We should be thinking hard about how to mitigate climate change, which is a real problem. That is less true of spinning exotic scenarios about hypothetical AI systems which have been given control over the physical universe and might enslave us in cancer experiments.
Lucas Perry: All right. So wrapping up here, do you guys have final statements that you'd like to say, just if you felt like what you just said didn't fully capture what you want to end on on this issue of AI existential risk?
Steven Pinker: Despite our disagreements, most about my assessment of AI agrees with Stuart's. I personally don't think that the adjective existential is helpful in ordinary concerns over safety, which we ought to have. I think there are tremendous potential benefits to AI, and that we ought to seek at the same time as we anticipate the reasonable risks and take every effort to mitigate them.
Stuart Russell: Yep. I mean, it's hard to disagree that we should focus on the reasonable risks. The question is whether you think that the hundreds of billions of dollars that are being invested into AI research will produce systems that can have potentially global consequences.
And to me it seems self evident that it can and we can look at even simple machine algorithms like the content selection algorithms in social media because those algorithms interact with humans for hours every day and dictate what literally billions of people see and read every day. They are having substantial global impact already.
And they are very, very simple. They don't know that human beings exist at all, but they still learn to manipulate our brains to optimize the objective. I had a very interesting little Facebook exchange with Yann LeCun. And at some point in the argument, Yann said something quite similar to something Steve said earlier. He said, "There's really no risk. You'd have to be extremely stupid to put an incorrect objective into a powerful system and then deploy it on a global scale."
And I said, "Well, you mean like optimizing click-through?" And he said, "Facebook stopped using click-through years ago." And I said, "Well, why was that?" And he said, "Oh, because it was the incorrect objective."
So you did put an incorrect objective into a powerful system, deployed on a global scale. Now what does that say about Facebook? So I think just as you might have said -- and in fact the nuclear industry did say -- "It's perfectly safe. Nothing can go wrong. We're the experts. We understand safety. We understand everything."
Nonetheless, we had Chernobyl, we had Fukushima. And actually, I think there's an argument to be made that despite the massive environmental cost of foregoing nuclear power, that countries like Germany, Italy, Spain and probably a bunch of others are in the process of actually deciding that we need to phase out nuclear power because even though theoretically, it's possible to develop and operate completely safe nuclear power systems, it's beyond our capabilities and the evidence is there.
You might have argued that while Russia is corrupt, it's technology was not as great as it should have been, they cut lots of corners, but you can't argue that the Japanese nuclear industry was unsophisticated or unconcerned with safety, but they still failed. And so I think voters in those countries who said, "We don't want nuclear power because we just don't want to be in that situation even if we have the engineers making our best efforts."
These kinds of considerations suggest that we do need to pay very careful attention. I'm not saying we should stop working on climate change, but when we invented synthetic biology, we said okay, we'd better think about how do we prevent the creation of disease or new disease organisms that could produce pandemics. And we took steps. People spent a lot of time thinking about safety mechanisms for those devices. We have to do the same thing for AI.
Lucas Perry: All right. Stuart and Steven, thanks so much. I've learned a ton of stuff today. If listeners want to follow you or look into your work, where's the best place to do that? I'll start with you, Steven.
Steven Pinker: Stevenpinker.com, which has pages for ten books, including the most recent, Enlightenment Now. SAPinker on Twitter.
Lucas Perry: And Stuart.
Stuart Russell: So you can Google me. I don't really have a website or social media activity, but the book Human Compatible, which was published last October by Viking in the US and Penguin in the UK and it's being translated into lots of languages, I think that captures my views pretty well.
Lucas Perry: All right. Thanks so much for coming on. And yeah, it was a pleasure speaking.
Steven Pinker: Thanks very much, Lucas for hosting it. Thank you Stuart for the dialogue.
Stuart Russell:It was great fun, Steve. I look forward to doing it again.
Steven Pinker: Me too.
Related episodes
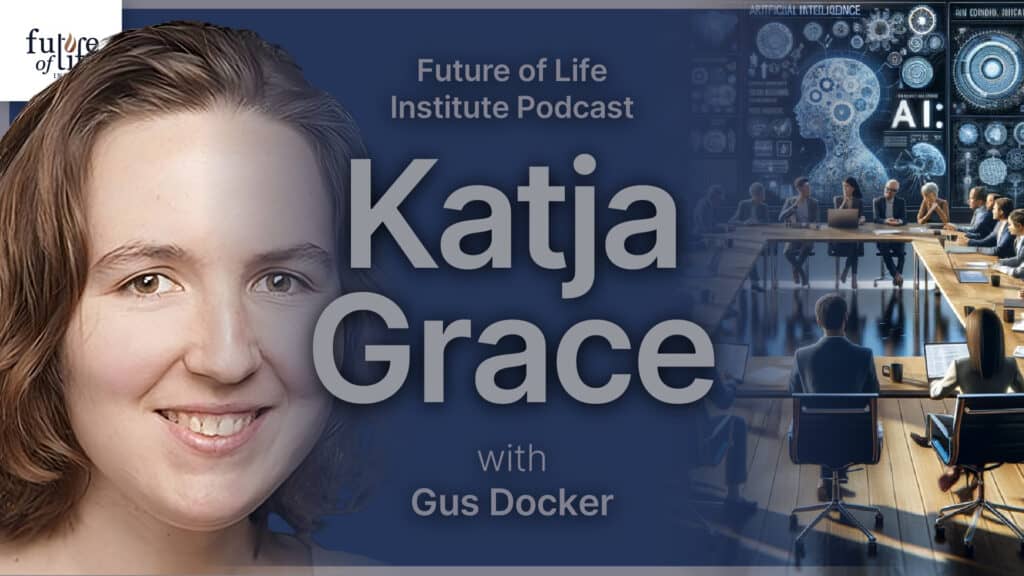
Katja Grace on the Largest Survey of AI Researchers
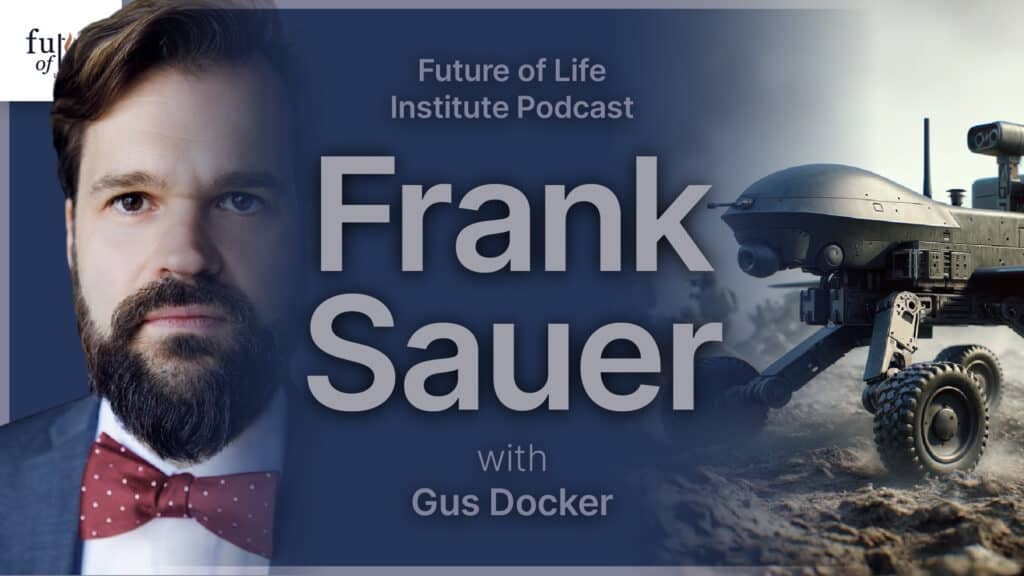
Frank Sauer on Autonomous Weapon Systems
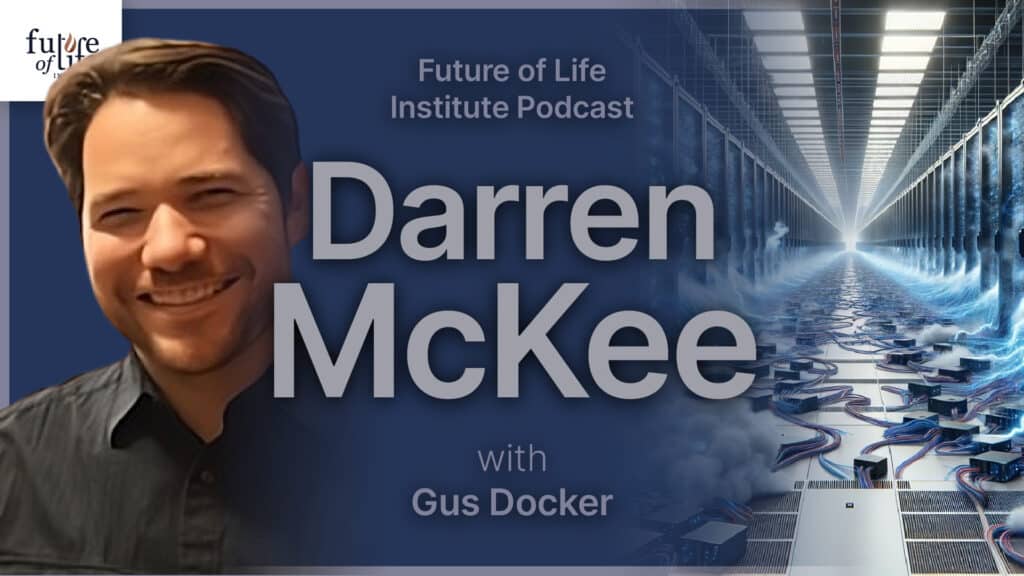