Roman Yampolskiy on the Uncontrollability, Incomprehensibility, and Unexplainability of AI
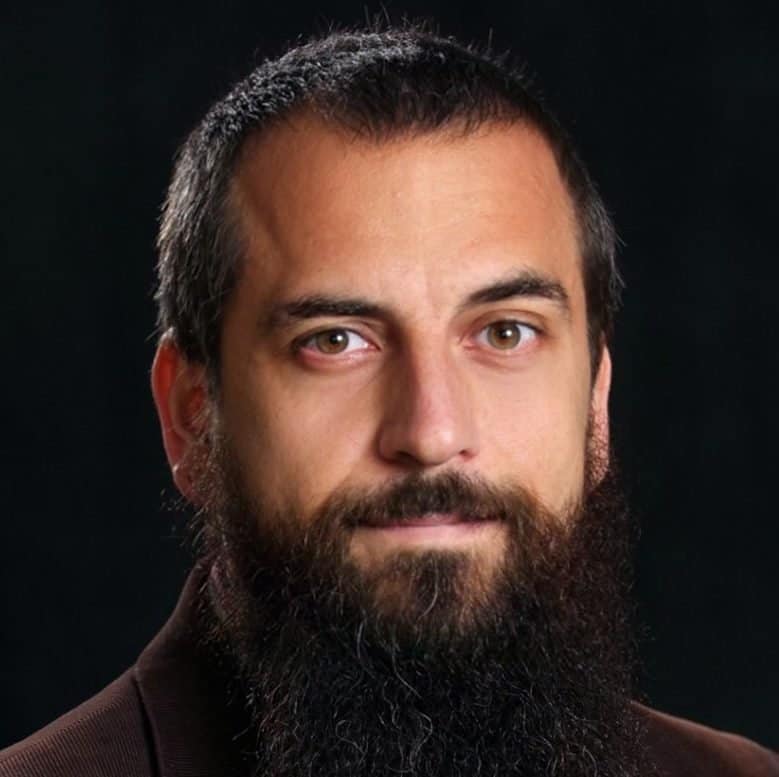
- Roman’s results on the unexplainability, incomprehensibility, and uncontrollability of AI
- The relationship between AI safety, control, and alignment
- Virtual worlds as a proposal for solving multi-multi alignment
- AI security
You can find FLI's three new policy focused job postings here
Paper's discussed in this episode:
Unexplainability and Incomprehensibility of Artificial Intelligence
Transcript
Lucas Perry: Welcome to the Future of Life Institute Podcast. I’m Lucas Perry. Today’s conversation is with Roman Yamposlkiy on uncontrollability, incomprehensibility, and unexplainability in AI systems, we also cover virtual worlds, and AI security. This episode is all about whether it's possible to maintain the control and comprension of, as well as ability to explain systems more intelligent than us, and how that affects the possibility of beneficial outcomes from AI. Before we get into the episode, the Future of Life Institute has 3 new job postings for full-time equivalent remote policy focused positions. We’re looking for a Director of European Policy, a Policy Advocate, and a Policy Researcher. These openings will mainly be focused on AI policy and governance. Additional policy areas of interest may include lethal autonomous weapons, synthetic biology, nuclear weapons policy, and the management of existential and global catastrophic risk. You can find more details about these positions at futureoflife.org/job-postings. Link in the description. The deadline for submission is April 4th, and if you have any questions about any of these positions, feel free to reach out to jobsadmin@futureoflife.org.Â
Dr. Roman Yampolskiy is a Tenured Associate Professor in the department of Computer Engineering and Computer Science at the University of Louisville. He is the founding and current director of the Cyber Security Lab and an author of many books including Artificial Superintelligence: a Futuristic Approach. Roman is also a Senior member of IEEE and AGI, a member of the Kentucky Academy of Science, and a Research Advisor for the Machine Intelligence Research Institute and is an Associate of the Global Catastrophic Risk Institute.
And with that, let’s get into our conversation with Roman YamposlkiyÂ
All right. So right now I'm pretty curious about what has been most intellectually stimulating and captivating for you, particularly over the past few years in terms of computer science and AI, it seems like you've been spending essentially all your time on AI safety and alignment research.
Roman Yampolskiy: I try to spend most of my time... I still have students who I don't want to expose to this crazy futuristic stuff. So they do kind of standard engineering work and obviously I work on that with them. But anything I'm doing full time, yeah, it's about AI safety.
Lucas Perry: Okay, so what research directions are you most interested in?
Roman Yampolskiy: So I'm trying to understand from theoretical, not just practical, but theoretical point of view, what is possible in the field of AI safety. Can we actually create 100% safe systems? Can we understand them? Can we explain their behaviors? So all sorts of limits and impossibility results, there seems to be a lot of that type of research and mathematics and physics and other fields in standard computer science, but it feels like in AI safety, everyone just kind of said, "Well, we need to have safe machine. So let's start working on it." And there is 100 different proposals for what to do addressing individual little sub problems. But it's not even obvious that the problem is solvable. So it felt like it was important to put a little bit of time to at least look at what can be done.
Lucas Perry: All right, so I'm interested in getting into that. And before we do, it'd be nice if you could unpack and explain a bit of the epistemology of and methodology of theoretical work in computer science and AI safety and alignment efforts right now, and how that is differentiated between efforts which take to apply different alignment and safety projects and explore them in systems which exist today. So what does that project of these theoretical proofs consist of? And what is it that you're hoping to extract through theoretical proofs?
Roman Yampolskiy: So it's similar to how this type of work is done in computer science in general, so if you look at early days of computer science with Alan Turing showing, "Okay, some problems are not even computable, some are unsolvable." We have this theoretical Turing machine which is not really a real thing, but we can prove things about what is possible for a Turing machine given when, "Oh, okay, it can take so many steps in a given amount of time." He was able to use contradictions to derive impossibility results such as you cannot tell if an arbitrary program has an infinite loop. So halting problem essentially.
I'm trying to do similar things, not concentrating on any particular model, of AI, I don't know what's going to get us to AGI, maybe it's very big neural networks, maybe it's something else. But I'm trying to understand, regardless of implementation, what would safety and control look like? What are the possible definitions for control we can look at? Are we interested in direct control where a system just follows orders? Are we looking for some sort of ideal advisor situation where the system knows you really well and does what's best for you? So I try to look at all possible cases. And in each one see, well, does that actually give us what we want? Is this option safe? And are we in control in those cases? Is there an undo button, undo mechanism, where if we don't like what's happening, we can kind of revert back to how it was before?
Lucas Perry: Okay, so can you make more clear the applicability to practical existing systems, in particular, this infinite loop and halting impossibility result? I imagine that there would be a spectrum of how, maybe this isn't the case, how useful in computer science and systems that exist today, certain impossibility results are. So for example, I don't know too much about Gödel's incompleteness theorem, is that applicable to current systems? How often do impossibility results actually inform the creation of robust and beneficial and safe computer science systems?
Roman Yampolskiy: Well, I think we greatly underestimate how common impossibility results are in science in general, I think that's majority of cases is that you cannot do certain things. It's very rare that you can, and we kind of do that all the time because it's what we're interested in, but it's a smaller subset of all problems. Now the Turing's result was just kind of like a first step in this direction, it was generalized. There is something called Rice's theorem, which says, "Any non-trivial property of arbitrary programs cannot be determined." So if we got this super intelligent code from SETI research, aliens beamed it to us, we would not be able to tell if it's friendly or not just by looking at it. So that's a non trivial property.
Now people correctly argue that while we're not getting arbitrary code, we're designing it so we don't have to worry about Rice's theorem, it doesn't apply here. But first of all, in many ways, we are getting arbitrary code because most AGI projects are not doing any safety. So out of 100 projects out there, maybe 10% actually have a safety team, the rest will just kind of give us something and say, "Okay, this is what we develop." So in a way, even that assumption is false. But even more so there is a whole set of additional capabilities you need to have to achieve control, and different fields, mathematics, computer science, economics, political theory, have their own impossibility results. And you can show that each of the sub problems is itself well known to be unsolvable. Simple things like voting, we know it's impossible to have voting where everyone gets what they want, their true preferences, there is always some sort of a situation where somebody has to be sacrificed for a greater good or something like that. And that's one example.
In my work, I try to kind of collect all those sub problems, which are already known to be impossible, and to show, "Okay, if we agree that this is important to be able to do, we need to agree on things." Well, that's impossible, we need to decide what is good long term, not just in a fixed amount of time, but for infinite amount of time. That's impossible, and so on. And so this is kind of where it all applies to practical systems.
Lucas Perry: Okay, so we have this project of alignment and AI safety. And because it's so new, and because it's dealing with superintelligent systems, there's this desire to do theoretical analysis on it in order to see whether or not safety or alignment is something that is possible under certain conditions and what that means. And so what we're going to get into is discussing, for example, the properties of controllability, explainability and comprehensibility, particularly in relation to systems that are as smart or much smarter than us. And so there's a desire to see what actually can be done there.
Before we get into that, to back up a little bit into something that you said earlier, why is it that if another civilization beamed us a bunch of code... And so you made the distinction here between arbitrary code and non-arbitrary code, code that we might intentionally create, why is it that we can't tell whether or not the arbitrary code is aligned or unaligned by looking at it?
Roman Yampolskiy: So there are two options usually, you can run the code and see what happens, and you can examine the source code, but they're essentially equivalent. The problem goes back to the halting problem, you cannot just see what it does, because it may be still running, it didn't stop producing output, and you don't know if it's an infinite process, it just doesn't stop, or you have to keep waiting longer. Now you can arbitrarily decide, "I will only look at it for 10 minutes, and after 10 minutes, I'll decide if it's friendly." Well, it's possible that after 11 minutes, it decides to kill you.
Lucas Perry: As we begin to speak about AI alignment, the concern is, for example, the risk of failures that are over a longer time periods and that are harder to see right away, right? So in computer science 101, it seems like you have people falling into infinite loops, I don't know, within short time periods because it's a little bit of code. But with AGI and more complex systems, the failure modes are more severe, and it seems unclear to me, for example, on what timescale these kinds of failures would emerge.
Roman Yampolskiy: Well-
Lucas Perry: And when whether they're safe.
Roman Yampolskiy: I cannot give you a specific time exactly, because that's the problem. You don't know how long you have to inspect the code, or run the program for because it may take longer always, there is not a specific set of time. So it could be an infinite process.
Lucas Perry: Is there anything else here that you think it would be helpful to add about impossibility results and their importance in clarifying and bounding problems and the kinds of solutions that can come from them?
Roman Yampolskiy: I think we want to understand what we can do with those systems. And it seems that it is not possible to predict their behavior ahead of time, if they are sufficiently smarter than us. It is not possible to explain decisions they do produce, and in cases where they are capable of explaining their decisions, we're not smart to understand them. So there seems to be a whole set of additional problems in this debugging and safety process.
Now, I see it and it's not a general agreement in a field, alignment is just a subset of AI safety, a particular approach to doing it, and alignment itself is problematic. First of all, who are you aligning with? Is it an individual? Or is it eight billion people, if it's eight billion people, we run into problems I described with voting mechanisms and agreement, you don't have multi agent, value alignment, problem solved. We don't agree on anything. Any election, you look at about half the people are miserable. Even if we had some sort of a beautiful algorithm for aligning all the humans individually into one coherent set of preferences, that set of preferences itself may not be safe. Most people are not safe, we're just kind of act well within our local moral code. But if taken outside of it later on, this will be considered a very unsafe set of behaviors. So we need a system which is aligned to a set of values which are changing, inconsistent, and we don't even agree on what they are.
So it seems like there is not just impossibility at one level, and everything else just falls neatly into place, but it's a kind of fractal nature of safety problem. The more you zoom in, the more you see additional problems with no solutions.
Lucas Perry: Okay, so let's take a look at some impossibility results or some ways that a solution might be constrained that you've looked at, in particular around unexplainability, incomprehensibility and uncontrollability. So let's start off with unexplainability and incomprehensibility. What are the results that you've found for these two aspects of AI safety?
Roman Yampolskiy: So the way we're doing it right now with large neural networks, you have a system which looks at, let's say, thousands of features, and assigns weights to them, could be millions, could be billions, doesn't matter. The decision is made based on all these features, they will impact the decision. But any type of explanation you would want to give would be a simplification of that, you cannot really just share your weights for the neural network and go, "This is why I did it." It doesn't really tell a human anything. So you have to reduce it.
Let's say you are deciding on giving a loan to someone, you're evaluating their loan applications. Maybe you look at just 100 features, you have 100 feature vector explaining why they got denied a loan, will probably be based on one or two features, "Okay, you have bad credit," or something. So you're not really telling them how the decision was made, you give them just so story, a simplification, kind of like what we do with kids. When a child is asking a complex question, you usually give them some simple story just to kind of make them happy, but they don't get full comprehension out of it.
On the other hand, if you do provide a sufficiently complex explanation, something another superintelligence will be fine with, a human is not smart enough to comprehend that. And we see it with people so even within the more narrow range of abilities different humans have, not everyone gets quantum computing, you need certain level of intelligence before you can take quantum physics courses meaningfully. That's why we test students before admitting them to programs. Otherwise, it's just a waste of everyone's time.
So those are complimentary results, you cannot fully explain and the lower capability agent cannot fully understand. And we see it with humans as well, if you ask a person to explain how they make certain decisions, they're unable to do so, and then they do kind of come up with some explanation, it's usually greatly simplified one.
Lucas Perry: Okay, so we're focusing here then on explainability. And so what you're saying is, by features you mean there are properties or metrics of any given situation that one can feed into some kind of decision making process in order to determine what to do, right? So whether to classify this image as a dog, or whether to disperse a loan to someone. And as systems get more and more intelligent, the more and more parameters in the decision making will be complexifying increasingly, and eventually get to a place where it will be unintelligible to humans on any timescale or on a short timescale.
Roman Yampolskiy: Well, I don't think timescale is meaningful here, it's more about overall complexity, you simply cannot handle that amount of information. It goes beyond standard human processing capabilities. If you say we have short-term memory of maybe seven units, and the system moves at a million variables at the same time, you simply cannot fit that type of decision making into your computational process. Now, you can do more with tools, if you can write down things, if you have narrow AIs helping you, computers, you can do better than unaided human, but still there are significant differences between what is possible and what we can do.
Lucas Perry: So what does it exactly mean then to claim that decisions made by increasingly complex AI systems are unexplainable?
Roman Yampolskiy: It means that you will not understand how that decision was made. Again, it helps to look at differences between agents we have today. Let's say you have a young child, you can tell them about something you're engaging with, some sort of process, but they would not understand it, and your explanation would not be sufficient for them to get it.
Lucas Perry: Well, I mean, it might be because oftentimes adults can compress decision making into a sentence that is intelligible by children, which is lossy, but is explainable.
Roman Yampolskiy: Right. You are exactly correct. It's either a lossless compression or lossy. If it's lossy, you providing some sort of a basic explanation, but it's not an accurate one, you lost information about the decision making process.
Lucas Perry: Is there a difference, though, then between unexplainability and lossy explainability?
Roman Yampolskiy: If you take it to the extreme, I think they're going to converge. It depends on how much of the intelligence gap you have between two agents. If you are nearly identical, and you just kind of skipping the last variable, maybe it's not that important, and you can just say, "Okay, it's a lossy explanation." But as the gap increases, greater and greater percentage of decision will not be explained by what is provided.
Lucas Perry: Yeah, that makes sense. But in the realm of AI alignment and AI safety, isn't the question whether or not the movement to increasingly lossy explanation allows us to have control and alignment or not? We can take a toy example like children and adults, and explanations from adults to children are always lossy, with respect to explaining why a decision was made. And so with systems that are much more intelligent than us, it seems reasonable to expect that the relationship would be similar, right? But the human adults are aligned in good cases, to the wellbeing of children. So the child can make requests of the adult and the fact that there are lossy explanations for decisions isn't really a situation that breaks alignment necessarily.
Roman Yampolskiy: I agree with you, you can certainly use that for ideal advisor control where you do say, "Okay, the system is way smarter than me, I'm going to put it in control of my life, and it's going to make decisions for me." But you just surrendered control to this other agent, you are not in control. And now the question is, is it actually successfully doing what you want? I kind of mentioned that I don't think alignment is meaningful. I don't think there is anything it can actually align to with respect to all of us. Maybe for an individual you can have some sort of a dictatorship situation set up, but how does this resolve for billions of agents?
Lucas Perry: All right, I think it'd be interesting to talk a little bit about that later. But now just simply in terms of the impossibility results, is there anything else you'd like to add about unexplainability and how you think it feeds into informing alignment and safety?
Roman Yampolskiy: Well, I think it's a toolbox of different results, which all are kind of useful to show a bigger picture. So each one of them maybe individually, you can say, "Okay, it is not that important that we understand, as long as the outcomes are good." But there is a whole bunch of those. Unpredictability is another one, we cannot predict what the decisions will be for sufficiently complex systems. So when you take all of them, we essentially find ourselves completely at the mercy of those agents in every respect, we don't understand them, we cannot predict what they're going to do, we are not in control. I mean, if you think it's a sustainable situation, the best an option you can have, but it's not, I think, universally agreed on.
Lucas Perry: Okay, so let's get into more of these results, then. Can you explain your results on comprehensibility?
Roman Yampolskiy: So it's a complimentary result to unexplainability. And, as I said, it has to do with difference in capabilities between agents. Simply you have to be a certain level of smart to develop certain algorithms, you have to be certain level of smart to comprehend how a certain algorithm works. And if you're not, you'll simply won't to get it. And depending on how big the gap is between the agents, it could be a huge problem or a small problem. But if we're talking about superintelligent systems, which might be thousands, millions of times smarter than us, that gap is just not something we can cross, in my opinion.
Lucas Perry: So with regards to explainability and comprehensibility, in my poor understanding of alignment, it seems like there are proposals which seek to maintain the status of alignment per step of increasing intelligence, so that confidence in alignment is maintained over time, such that explanations and comprehension for systems that are smarter than human beings has a higher credence of it being accurate or true. Can you add any perspective on this? Or is this wrong in any way?
Roman Yampolskiy: Are you saying you'll have a chain of agents with progressively slight increase of intelligence helping to control the next agent of progressively higher intelligence and so on?
Lucas Perry: Right, so I mean, one proposal that comes to mind is iterated distillation and amplification, these kind of sequential tiered processes where you're increasing the level of the agent, while also having other agents help to maintain alignment for each step. And so as that intelligence increase happens, the system may becoming increasingly unexplainable and incomprehensible, but alignment is maintained per step of the process.
Roman Yampolskiy: It's a great proposal, I'm happy people are working on it. My concerns are one, the complexity increases, as you have more steps, more agents, there is more possibilities for error. That's just standard problem with software development. Additionally, I'm not sure this whole idea of alignment can work. So how do you maintain something which even doesn't make sense initially? Again, I'm trying to-
Lucas Perry: Yeah, because of multi-multi, is what you're talking about.
Roman Yampolskiy: Well, at all levels. So first of all, yes, we have multiple agents who don't agree on values. Then an individual agent who does, let's say, agree on values may have unsafe values. So it's not obvious who is in charge of filtering out what is safe and what is not safe to have as a value. And then on top of it, you change your values, what you consider desirable right now at your current state, your level, your affordances may be completely different. And for most people, it is completely different, things I wanted when I was six years old are not the same things I want today, thank God.
So it seems to be we are trying to hit this target which doesn't exist, keeps moving and changing. I'm not asking anyone to say, "Okay, this is an algorithm for how to do it." But at least an explanation of what it is we're trying to accomplish, which goes beyond just kind of saying, "Well, do what we want," would be a good first step, and then we can talk about making this process more reliable, some sort of additional verification. Yes, absolutely.
Lucas Perry: Okay, so let's bring in uncontrollability here. What are your arguments and proof in views around the uncontrollability of AI systems?
Roman Yampolskiy: So I tried to, as I said, look at different definitions for what we mean by control. The simplest one people usually start with is direct orders, I tell the system what to do, it follows verbatim, "Okay, this is the order, this is what I'm going to do." This is a kind of standard Genie problem, right? You have three wishes, and you come to greatly regret everything you wished for and just hope to undo damage. This is not even taking into account malevolent users who have malevolent wishes and want to destroy and cause harm. But even if you ask for something you think is good, it is not obvious that this should be implemented, especially again, because other agents may strongly disagree with you. So I think it's easy to see that direct control is unsafe.
Lucas Perry: Sure. So could you unpack this a bit more?
Roman Yampolskiy: Right. So in the paper on that topic, I use example of self-driving cars. The direct order would be, "Stop the car." So you on a highway, you're going, I don't know, 65 miles an hour, and you give an order, "Stop the car," and the car just slams the brakes, and you stopped in the middle of traffic on the highway, that was direct following of your orders. Maybe somebody hit you from behind, maybe something else happens, but that's the situation. That seems unsafe. Systems should not just do whatever random human tells it to do, especially a very powerful system.
Lucas Perry: Yeah, so it seems to be a distinction here between then alignment and controllability.
Roman Yampolskiy: Absolutely. As I said, alignment is just a small subset of this bigger problem of safety and control. Direct orders do not have any alignment in them. If you're looking for alignment, that would be indirect control where you have an ideal advisor which knows you, knows your preferences, has your model, and tries to do what you would want accomplished. So at this low level of direct control, alignment doesn't enter into the picture.
Lucas Perry: Why is alignment a subset of control and safety, in your view?
Roman Yampolskiy: Well, the problem we're trying to solve, in my view, is to make safe and controllable intelligent systems. Alignment is one proposal for doing that. It's not the only proposal, people had other ideas, that's why it's a subset. So if you look at three laws of robotics, right, just giving very hard-coded rules, that's a proposal for accomplishing safety and control, but we don't think it's a good one, but it's not alignment based.
Lucas Perry: Okay. It's an interesting way of breaking it up. It's slightly unintuitive to me, because it seems like the definition and meaning of control and safety, for example, rest upon and are contingent upon human values, and the meaning with which we ascribe to what it means for something to be controllable or safe. For example, safe is a very value loaded word. And so to say that alignment is a subset rather than a superset of that seems, maybe it's not so linear, maybe there's a bunch of overlap, but it's a little unintuitive to me.
Roman Yampolskiy: It's probably unintuitive for most AI safety researchers. But I think it's both historically accurate, we started thinking about this before alignment became a dominant idea. So if you look at science fiction, early days, they definitely worried about safety with robots and such, but alignment was not the solution proposed at the time. So the field of safety was there, solutions were proposed, but alignment was not on the table yet. You are completely correct that terms like safety and control are value dependent, that's why I try to look at every possible interpretation of what it means to be in control, direct control, indirect control, any type of combinations of those two, there is also the hybrid approach. So yeah, if there is something missing, let me know, we can analyze that type of control as well.
Lucas Perry: Okay, can you explain the relationship between these three facets that we've talked about now? So between unexplainability and comprehensibility and uncontrollability, and how you view them in relationship to what AI alignment means to you?
Roman Yampolskiy: So when I talk about control, in order to be in control, to have meaningful control, you have to understand the situation. You understand what's happening and why, and what's likely to happen. You're in control. If you definitely don't know what's going to happen, you don't understand the things which are already happening, and you have no way of influencing future decisions, you are not giving direct orders, the system is just doing whatever it wants and thinks you would like, you are not controlling this agent, you are subject to full control by that agent. And depending on the setup, you may not have any recourse, you may not have an undo button saying, "I just want to go back to 2020." So that's my view of how all this results...
And there are additional results, we have an ambiguity of language for issuing orders, there are results on unverifiability of code and programs. So all of them, to me, indicate additional issues with claims of control. So you have to decide what it is you you're trying to accomplish. Once you decide what type of relationship you want with this agent, I'm trying to look at that specific type of relationship and see, does that create any problems? Are there ways it can fail? Is it safe? And do you. have meaningful decision power over future outcomes of your existence?
Lucas Perry: Okay.
Roman Yampolskiy: Some of them rely on alignment, like ideal advisor, indirect control, some do not rely on alignment, like direct control.
Lucas Perry: Okay. So how do you see the results of these different facets, ultimately constraining what control, safety and alignment research and efforts should be like in the 21st century?
Roman Yampolskiy: Well, I think the fundamental question here is, can we succeed? So is it a question of us just having more resources, more researchers taking a little more time, and then yes, it's possible? Or it's just impossible, and every day we get closer to a problem we cannot solve? If our people agree with my assessment, and it is not a solvable problem, then our actions should be very different from, "Yeah, we can definitely solve it, just give us another billion dollars in research grants."
Lucas Perry: What does it mean to be able to solve the problem? In terms of building beneficial AI systems.
Roman Yampolskiy: So the two requirements I would like to see is that humanity is in control, perhaps as a whole, not as individuals, meaning we can undo any changes which happen, and the safety requirement, meaning we are not in danger of existential crisis, we are not negatively impacted, we are not tortured or anything like that. So if those two basic requirements are met, maybe it's a pretty good solution.
Lucas Perry: Okay. So when we claim the impossibility of something, it seems to me that we would be claiming some kind of capacity of having pure knowledge or a credence that is basically 100% kind of like a theorem that has been proved from uncontroversial axioms. What is an impossibility result for something like control, explainability, and incomprehensibility actually saying here? Is it saying that we can never arrive at 100% credence or how much control explainability and comprehensibility can we actually have? I bring this up, because I can see a situation in which impossibility results are not exactly helpful or applicable or useful in the real world, because they deal with some kind of epistemic statement, like you can't have 100% credence over what this thing will do, but maybe we can have very, very high degrees of certainty.
Roman Yampolskiy: Right. So this is a great way of looking at it. Let's say you definitely agree that we cannot get 100% safe decision making out of those systems, maybe it's 999 effective. Well, if a system makes a billion decisions a minute, then you're guaranteed to have a failure mode your first day. If what is at stake is all of humanity, that is not an acceptable safety target. When we're talking about standard cybersecurity, yeah, we have software, yeah, it's going to fail once in a while, somebody is going to lose a credit card number. We'll give you a new one, let's move on. It's a completely different safety standard for super-intelligence safety if all of humanity can be lost as a result of such a rare event where it happens once a week, once a month, whatever.
If you look at standard software industry, how often do we have a bug or hacking attack? We cannot accept the same standards which are typically used in this industry. The standard is to make you click on the "I agree" button without reading the contract, and then whatever happens is not their problem. I don't think that same standard can be applied here. The more the systems become capable, the greater the difference, the gap, between an average human and that system is, the greater all this problems become. If the gap is five IQ points, it's not a big deal. Explainability, predictability, this is what we have with our humans right now. But if a gap is huge, those problems become proportionately bigger, you find yourself in a situation where you are not making decisions, you don't understand what is being done to you or for you.
Lucas Perry: What does AI alignment really mean to you? You set up this example here where you see it as a subset of control and safety, and also you bring in the difficulty of multi-multi alignment. Right, so Andrew Critch has this taxonomy of single-single, single-multi, multi-single and multi-multi alignment, where the first word in each pair is the number of humans and the second is the number of AI agents. And you argue for an impossibility result with regards to multi-multi alignment. So if you, for example, pointed out an example in politics and governance, where we're making trade offs between mutually incompatible values. So I'm curious here, a good future to you then looks like something where humans are in control, and it seems like because you've supposed that multi-multi alignment is impossible, in 2019, I believe you proposed personal universes. Would you like to kind of unpack... I guess, I don't know, control, the deep future, alignment, and personal universes?
Roman Yampolskiy: Right. That's a wonderful question, actually, I was about to mention that paper, and you stole my thinking on it. That's great. So the problem I see is getting, right now, I don't know, almost eight billion of us to agree on anything, and it seems impossible. What if we can use future technology, which is developing also very quickly, alongside with AI research, virtual reality, we're getting very good at creating experiences for people which look real, they feel real, people enjoy spending more and more time in virtual reality. If you project it far enough into the future, you can get to the level of performance where you can't tell if something is real, or virtual, especially if you can modify memory enough to erase the point of entering the virtual reality. You can have this perfectly, visually, texturally in every sense of a world, real experiences.
Now, if I can create an individual virtual reality for every agent, we no longer have multi agent agreement problem, I can give each agent exactly what they want in their own universe, you don't have to agree. You still have options, you can be without agents, you can travel to different universes, but you can also have your own universe where you decide what is the proper values to align with. So I think, while it doesn't solve the fundamental problem of controlling substrate, and which all this computation is running, and superintelligence controlling it, at least it addresses one of the biggest, in my opinion, unsolved problems of value alignment, how to get all humans into an agreement.
Lucas Perry: Right, so this is born of... Your position then is that alignment is impossible, given the divergence of and incompatibility of many human values. And so what we want is control and safety. And given the impossibility of multi-multi alignment, what we go ahead and do is create some large number of virtual or simulated worlds which allow for the expression of multiple human values to coexist in our base reality. And the key thing that you said here was, "Then we will have agreement." Right? You'll have agreement between humans. So it's a proposal I think that supposes a very particular kind of metaethics, right? It's valuing agreement of human beings and the permissibility in expression of their values over anything else, which is its own kind of met ethical commitment, right?
So it's almost a kind of relativism, a relativism that human preferences are empirical facts, and some of them are incompatible with others. So the only way to have agreement which means humans aren't trying to actively, I don't know, kill each other, which saying that is worse than the expression of certain kinds of values is a particular kind of metaethical commitment. But it's basically a vehicle for allowing the proliferation and an expression of moral relativism. Is this a fair characterization?
Roman Yampolskiy: Absolutely. And people have argued that, yes, you can have universes where there is tremendous amount of suffering because someone is a psychopath, and that's what they in to. And there is need for lots of additional research to see if that can be addressed. If qualia is something we can control and maybe that could be an additional step where, okay, you can have all sorts of fun in your universe, but other non-playing characters in your universe are not suffering. And that seems to be solvable, technically, since they don't have their own values we have to follow, we can design them from scratch. They're not existing agents.
Lucas Perry: So do you think that ethical or moral statements like suffering is bad, have a truth value?
Roman Yampolskiy: In that regard? Yes, I do.
Lucas Perry: So do you think that third-person truth about it can be arrived at?
Roman Yampolskiy: About suffering being-
Lucas Perry: Bad.
Roman Yampolskiy: ... the only true thing in the universe?
Lucas Perry: Of disvalue.
Roman Yampolskiy: I think so.
Lucas Perry: So if it's possible to get truth or false statements about what value or disvalue in existence means, in being means, wouldn't the personal universes proposal violate that kind of epistemological confidence in discovering the truth or false value of moral statements, right? Because it seems like you think that relativism is not true, because you think that, for example, suffering can be said to be bad, probably we could discover that. But the personal universes hypothesis is, it's no longer interested in pursuing whether or not that kind of truth is discoverable, and is allowing the proliferation of, for example, suffering in some universes if the person is a psychopath.
Roman Yampolskiy: So for me, the question is, what are we comparing this outcome to? If we comparing to the base reality we have right now, suffering is already a situation on the ground, we're not making it worse, there is a good chance that many of those universes will not have psychopaths in charge. Also, as I said, we can look exactly how non-playing characters have to or don't have to have real experiences. They can all be philosophical zombies. So whatever you're into, you still get that experience on your end, but it's just game characters, they're not actually suffering. So I think there is a solution to that problem, or at least improvement on state of the art with this universe.
Lucas Perry: Right. But it seems like the personal universes hypothesis is a way of trying to get over, for example, metaethical policing. So if I think really hard, and think that I have answers to foundational, metaethical questions, or I have some kind of, I don't know, direct kind of knowing about the truth of moral statements, it seems like the personal universe hypothesis is trying to get around the forcible imposition of moral knowledge upon other people. And so allowing the relative expression of different preferences and values. Yet, it's also at the same time trying to constrain what you can do, for example, in that universe by... It seems like you're proposing the introduction of something like non-player characters, like NPCs, so characters within a universe who don't have conscious experience. Yeah, it could be in the sadistic or masochistic value system, it would be against their values, for example, to be in a universe with NPCs.
Roman Yampolskiy: Right, so you can look at extreme cases and definitely, there are unsolved problems. I'm not claiming that that is a perfect solution to anything. I do think that for most people, again, we have nearly eight billion people, this philosophical debate about moral values is less interesting than their basic preferences in terms of what temperature I want my universe to be at. I like it hot, some like it's cold, okay, I like free burgers. This is the type of daily reality I'm envisioning for these people. They're not so much engaged in understanding ultimate knowledge about morality, but just having experiences they want. I don't want to live in a communist country, I want to live in a capitalist one. Things of that nature, basic human experiences in terms of food, sex, adventures, there'll certainly be philosophers who'd enjoy just having those podcast debates about the nature of a substrate reality and, "Is this a multiverse? Are we living in a simulation?" But this seems to be solving some real problems for people who will be impacted by artificial intelligence when it is deployed.
Lucas Perry: Yeah, sure. So I don't want to get too lost in the future. But I mean, that would seem to be satisfactory only for a very short period of time. So I think the only thing I was trying to suggest is that if you have some inclination towards thinking that we can arrive at knowledge about moral truth claims, and particularly around metaethics, that means that the problem of multi-multi alignment rests upon our ability to come to that knowledge, right?
Roman Yampolskiy: Just because we have true knowledge doesn't mean people are aligned with it, there is people who think the earth is flat. The fact that we have correct tensors means nothing for them agreeing with you, or being happy in your universe. Customer is always right, you have to satisfy their preferences, not true knowledge. It's possible that the superintelligence will discover that all of us are wrong about almost everything and will be very unhappy in that other world where, "Okay, things are scientifically true, but we're miserable."
Lucas Perry: Right. I think that there will be maybe edge cases of that. But I think what I'm proposing is that the personal universes hypothesis is pretty uncompelling for me, to the extent to which there is a... that all people want the truth, essentially. That seems practically universal. And even people that believe things that are obviously false, they think that they're true, and they're acting in good faith towards that end. But if there is a truth about moral statements, then it seems like there would be an obviously... and I think there might be a necessary relationship between what is true and good. And thus, there's a direct relationship between truth and wellbeing. And insofar as that everyone wants what is true, and what is also good for them, they want to be free of suffering, it would seem that the personal universe hypothesis would kind of throw... it would jam the wheel of progress of collective convergence upon what is true and good, by allowing people to fall into and express their, I don't know, their disagreements.
Roman Yampolskiy: So is just a set of options, you don't have to have your own universe, you can stay in the base reality, you can be with other and disagree and agree to compromise, that's an option. I like offering options. Most people most of the time have no problem going with fake experiences, fake knowledge. Every time you go to the movies, every time you read a science fiction book, you willingly go into this other world, which you know is not true reality, but you're happier there. And we do it a lot. We really don't mind paying other people to create fake experiences for us, we just want experiences. And if it's possible to manipulate your memory to where you really can't tell the difference between what's real and what's fake, I guarantee you that industry will be very desirable. Lots of people will want to have amazing experiences, even if they're just dreams.
Lucas Perry: Yeah, I agree with that. I think that's true. So one thing that you're... And we're taking a bit of a pivot here. One thing that you're quite well known for is the realm of AI security. So I'm curious if you could talk a bit about issues regarding AI security from current day systems all the way to AGI.
Roman Yampolskiy: So I think concern is malevolent agents. We have companies, DeepMind, OpenAI, developing very powerful systems, which will probably get a lot more powerful. Lately, they've been keeping the source code closed as much as they could. But if they are hacked, if the code leaks and malevolent agents get access to it, that could be another area of concern. So we're not just trying to get the good guys to align the system to design it properly, but now we also have to worry about deliberate misuse. And that seems to be a much harder problem because you're not just thinking how to make the code proper, but also how to control our intelligent agents and what they do. And they're definitely misaligned with your goals of creating safe beneficial AI, the same people who are right now writing computer viruses will definitely go to the next level of technology for massive scale, social engineering attacks for blackmail for any type of crime you can automate with this more advanced AI.
Lucas Perry: Sorry, so what do you think the relative risk profile is between malevolent AI and misaligned AI? Does security not apply to both?
Roman Yampolskiy: The worst, most challenging problem is malevolent purposeful design. Because you still get all the same problems you had before, you have bugs in code, you have misalignment, but now you have additional payload, malevolent payloads specifically added to the system. So it's strictly worse.
Lucas Perry: Yeah, but is it more likely?
Roman Yampolskiy: Is it likely that there are people who want to commit crimes using advanced technology? Yeah.
Lucas Perry: Right. But in the realm of global catastrophic and existential risk, given the level and degree of security at organizations like DeepMind and the kinds of resources that are needed to, I don't know, deploy and train a misaligned powerful AI system, what is more risky?
Roman Yampolskiy: Well, those resources seem to be becoming more accessible, anyone can rent cloud services from Amazon, lots of governments invested in advanced weaponry, so they would be willing to hack into American companies, we've seen that before, it's not unusual. Also, you have, again, insider threat, an employee at a company, we've seen that happen a lot, who takes the source files and makes them accessible to others for profit, or for personal reasons. You can blackmail employees, you can do all sorts of things if this as your target. So I don't think there is any companies, or even government agencies, which have not been hacked so far.
Lucas Perry: So that's one dimension of this. There's also security in relation to general systems in general. So this is, I don't know, air gapping and boxing, as I think security measures against systems which we're trying to test for whether or not they are controllable and aligned. Can you explain a little bit more about the aspect of security in terms of constraining the causal power of systems that haven't been deemed safe yet?
Roman Yampolskiy: Right. So the difference is external attacker, so internal attacker AI itself, you're trying to build a prison, or you're trying to keep people out of the prison. And the standard name I think is AI boxing, you're trying to put AI in this limited environment where you control all the resources going in, all the information and, even more importantly, controlling output, so it is not able to engage in social engineering attacks. I think everyone who looked at that agrees it's impossible to do it permanently as a long-term solution. But it could be a useful tool to study the systems under development for short amounts of time. So it's good to have those tools, virtual environments, where we do have a lot of control over systems as they become more capable. But if you are observing the system, it can impact you and most likely will find ways to influence you to release it.
Lucas Perry: So I mentioned air gapping and boxing, perhaps, in some kind of virtual environment. Are there any other security apparatuses that are useful here for keeping the thing inside of the jail?
Roman Yampolskiy: So people talk about encrypting the code itself. So there is some very advanced encryption tools, which allow you to run encrypted code on encrypted data. It's super slow, it's not efficient, it will slow down research a million times. But in theory, it's possible. So the system would not have access to its own source code for additional modification improvement. So that's something probably worth looking at.
Again, this is in direct proportion to the resources you have, you can make it safer, proportionate, you can have multiple levels of virtual worlds, separated hardware, you can have separation of controls. A lot of times with boxing experiments, the classic boxing experiments, the guard has an option of releasing an AI, why would he have that option, you should never have an option of actually releasing it. That's already kind of unrealistic example. Prison guards don't get to release prisoners, it's not their decision. So you need to have multiple levels of indirection for anything like that to happen. But still, I think, if you are observing the system, information is leaking out, and that's enough long-term periods to escape.
Lucas Perry: I see. So you're suggesting layers of redundancy in pointing out this fact that if there's any channel of being able to observe something, that is, in and of itself, leaky with regards to the inside to the out. So is there no such things such as a one way mirror in computer science for boxing such a system?
Roman Yampolskiy: Well, if you as a human observing behavior of a system, you getting information out of the box into the world. If we're not observing the system, then it's useless. We're not getting any experimental knowledge from it.
Lucas Perry: Right. But if a superintelligence is in a black box, and it has no world model, it's only in a simulated environment and doesn't know anything about the humans, and the window of observation for that system doesn't give that system any more information about who's observing it, then how would it break out of the box without knowing anything about the outside world?
Roman Yampolskiy: So this is where unpredictability is a good excuse, I cannot predict what a superintelligent system would do. My guess is you can learn a lot about designers of the world by looking at the world, how it's made, if you have access to the source code for the system, you can see, "Okay, they're very slow agents based on update times for the code. They really not that intelligent, given the bugs and imperfections and inefficiencies in the code." And this type of forensic analysis can be done to figure out what the programmers are, who they are in terms of their capabilities.
Lucas Perry: I see. So forensic analysis of the AI's own code, or the simulated world would have enough evidence and information in it to leverage in order to break out of the box if humans are observing it.
Roman Yampolskiy: At a basic level, it may be able to discover loopholes, just zero day exploits we didn't know existed there. Second level, it can do some... depending on access to resources it has, it can perform novel experiments, maybe discovering novel physical properties. There are some attacks and computer systems based on writing to certain parts of the memory, rewriting them frequently creating side effects. You can look at how hacking is done from virtual worlds from video games, people were able to break out of Mario and get access to the operating system. And that's pretty weird to invest your time into that, but people did that. And again, I simply cannot think what much, much smarter system would be able to do. If I could, I would be a much smarter system.
Lucas Perry: Yeah. Okay. So we've had a lot of failure of AI systems. Can you unpack some of your favorite historical examples of AI failures, and what that tells us about how the future of AI technology will likely also include failures?
Roman Yampolskiy: So I collect those examples. So if your listeners have any cool examples not already in my publications, I'd be very happy to get them. The pattern I found is that basically no matter what the system is designed for, so it's designed to do X, it will fail to X. If it's a spell checker, it will fail to correctly fix your word, give you a wrong word. If it's a self-driving car, it will have a car accident, it doesn't really matter. Basically, eventually systems, no matter how accurate they are, 99.99%, they will fail at it. That's one type of problems we saw.
Another problem is people under estimating how creative other humans are in terms of what they're going to do with the system, how are we going to use it or abuse it? Before releasing any AI service or product, it's really worth spending some time thinking, "Well, what's the worst thing they can do with this?" So we've seen examples like chatbot, which is trained by users on Twitter, I don't think you have to be a superintelligence researcher to predict what's going to happen there. Yet, Microsoft did that, released it, and was quite embarrassing for the company.
I like examples from evolutionary computation a lot, because they always surprise their designers. And mostly the surprise is that the system will always find a lazy way to accomplish something, you want it to do it the hard way, it will find the shortcut. And basically cheating, like cheating students, cheating businessman, it will always exploit the lowest possible way to get to the target.
Lucas Perry: Yeah okay, so the last example is reward hacking, or at least that's what comes to my mind. Well, it's achieving the objective in a way that is not what we actually intended, right? Or the objective is a proxy for something else and it achieves the proxy objective without achieving the actual objective.
Roman Yampolskiy: There is probably an infinite way of doing almost anything in the world, and it will find the one which takes the least resources.
Lucas Perry: And that is not always aligned with the actual objective.
Roman Yampolskiy: It is almost never aligned with the actual objectives. Because on the way on the path there, you can do a lot of damage, you can cause a lot of damage or bypass all the benefits. So you almost have to specify all the points in the path to the target to get what you want.
Lucas Perry: And so the first thing you said... It seems like, again, you are also bringing in the impossibility results, because you're talking about this distinction between 100% certainty of how a system will behave and 99.99% certainty. And what you mentioned earlier was that if a system is running at billions, or trillions of times faster than human computation, then very small probabilities will happen. It seems like a really important distinction between when those things happen, whether or not they're global, catastrophic, or existential.
Roman Yampolskiy: Mm-hmm (affirmative).
Lucas Perry: Right? And how often they're actually happening. And this is I think, getting back to some of the impossibility results, is I'm trying to really understand the distinction between saying 100% certain knowledge in the verifiability of some program as being friendly or that it won't halt or fall into an infinite loop. It seems like there's an important distinction between what is impossible and what is so highly probable that it won't lead to existential or global catastrophic risk in most or all universes.
Roman Yampolskiy: Right. And we can look at, for example, mathematics, right? So Gödel's incompleteness theorem, it didn't stop mathematics, it didn't stop people doing mathematical proofs. It just kind of gave us this understanding of limits of what we can accomplish. In physics, you know about, okay, there are limits on maximum speeds, there are limits on certainty and observation, it didn't stop physics, but it gives us a more accurate picture of what we are working with. I feel it's important to have complete understanding of intelligence as a resource. I think people talk about space, time as a resource, intelligence may be another computational resource, and we need to understand what we can accomplish with it, and if something is not possible with 100% certainty, maybe we can do it with less accuracy. But at least knowing that fact can save us the resources in not following dead-end goals.
Lucas Perry: Right. But what would be a dead-end goal that comes from these impossibility results? So earlier, at the very beginning of the conversation, you mentioned that it was important that we do this work, because it hasn't been proven whether or not the control or alignment problems have a solution. And there's a lot of things in life that don't clearly have solutions. Like we can't compute the whole universe. So how does that actually affect the research efforts and alignment and control and safety paradigms as we move forward?
Roman Yampolskiy: So let's take a hypothetical, if you knew before experimenting with nuclear weapons that it will definitely explode the whole atmosphere, definitely, would you still develop nuclear weapons?
Lucas Perry: Then there's no point in developing it if it's going to ignite the atmosphere.
Roman Yampolskiy: Right. So that's the question I'm trying to answer, if we are certain that we cannot do this in a safe way, should we be doing it? If we're certain that it's possible to control, then obviously, the benefits are huge. If there is a probability that actually no, you can't, then we should look at other options.
There is a lot of suggestions for maybe delaying certain technologies in favoring others. You don't have to do everything today. What happens if we postpone it and take five years to think about it a little more?
Lucas Perry: Right. So this is like global coordination on the speed of research, which I think people have been largely pessimistic about because there's a story that in global capitalism and between the militaristic and imperial conflict between nations and countries that it would be impossible to slow down progress on a technology like this.
Roman Yampolskiy: I don't disagree with that additional impossibility you mentioning, I think that's also an impossibility to stop research without completely suppressing civilization. But it doesn't negate the other impossibility, and if control is not possible, again, if nuclear weapons were known to be definitely the end, we'd still have the same problem between Germany, Russia, China developing nuclear weapons, they still would fear not developing them. So that in itself is not an argument to say, "Oh, we have to go forward anyways."
Lucas Perry: So when demonstrating the impossibility of control, is that not suggesting that there is still some very high credence of control that is able to be achieved?
Roman Yampolskiy: Explain why.
Lucas Perry: Well, so if you say, for example, control of such a system is impossible. Is that kind of an absolute claim that certain control is impossible? Or is it a claim that any credence of control over a superintelligent system is impossible?
Roman Yampolskiy: So I think this is where my different types of control are very useful. Which one are you referring to? So with direct control is possible, we can tell the system exactly what to do and it will follow an order. I'm saying this is unsafe, it results in clearly negative consequences. The ideal advisor indirect control situation can be set up, but you lose control, you may obtain safety, but you lose all control. If we all agree on that, we can do it. But is that a desirable future?
Lucas Perry: So can you explain a few more ways in which systems have failed? Out of your list, could you pick a few more examples that are some of your favorites? And what that tells us about the risks of AGI?
Roman Yampolskiy: So the interesting examples are all different applications, we had autonomous systems used in military response, so detecting an attack and automatically saying, "Okay, Soviets are attacking us, Russians, or Americans are attacking us, and let's respond." And the system was wrong, so just this type of decision and accuracy was not high enough. We have great examples where, again, with evolutionary algorithms, we're trying to, let's say, evolve a system for walking. But walking is hard, so instead, it learns to make tall agents and fall forward, that's quick and easy. So they are very interesting. You would not come up with those examples if you had human common sense. But AIs usually don't have human common sense. So they find solutions outside of our comfort box, sort of. And I would predict that that is what we're going to see with any boxed AI, it will find escape paths which we then consider. It will not go through the door, it will find how to penetrate a wall.
Lucas Perry: Do you think that oracles are a valid approach to AI safety or alignment given expectations of commercial and industrial creation and deployment of AI systems? Because it seems like we're really going to want these systems to be doing things in the real world. And so they already have that role, and they'll increasingly be occupying that role. So is it at all reasonable to expect that the most cutting edge systems will always be oracles? Are there not incentives that strongly push the systems towards being more agentive?
Roman Yampolskiy: Early systems are very likely to be oracles, but I don't think that makes them safer. You can still ask very dangerous questions, get information, which allows you... especially with malevolent actors, to either design weapons or hack some sort of a process, economic process or business process. So just the fact that they are not actively in the world and embodied or anything like that doesn't make them a safer solution if they're capable enough.
Lucas Perry: Do you think that the risks of oracles versus agentive systems creating global catastrophic or existential risk is about the same?
Roman Yampolskiy: I'm not sure you can separate an oracle from it being an agent to a certain degree, it still makes decisions about the resources that needs computational access, so that is not that different.
Lucas Perry: Which has causal implications in the world.
Roman Yampolskiy: Exactly.
Lucas Perry: Okay, my vague intuition seems that it's harder to end the world as an oracle, but maybe that's wrong.
Roman Yampolskiy: I mean, it depends on the type of advice. So let's say you have a COVID pandemic happening and people ask you, "Can you suggest a good medicine for it?" It gives you full access to whatever you want.
Lucas Perry: I see. It gives you microchip vaccines that all detonate in one year.
Roman Yampolskiy: Well, it could be more than one year, that's the beauty of it, you can do something four generations later. You can make it very stealth and undetectable.
Lucas Perry: So given all of your work and everything that has been going on with AI alignment, safety and security, do you have any final thoughts or core feelings you'd like to share?
Roman Yampolskiy: Right. So I understand a lot of those ideas are very controversial and there is nothing I would want more than for someone to prove me completely wrong. If someone can write something arguing formally or mathematical proof or anything, saying that, "No, in fact, control is possible. All the additional sub requirements are met." I would be very happy and very welcoming of this development. I don't think it will happen, but I would like to see people at least try. It is really surprising that this most important problem has not seen any attempts in that direction outside a few short blog posts where people mostly suggest, "Well, of course, it's possible," or, "Of course, it's impossible."
Lucas Perry: All right, Roman, thanks a bunch for coming on the podcast and for speaking with me.
Roman Yampolskiy: Thank you for inviting me. Good to be back.
Related episodes
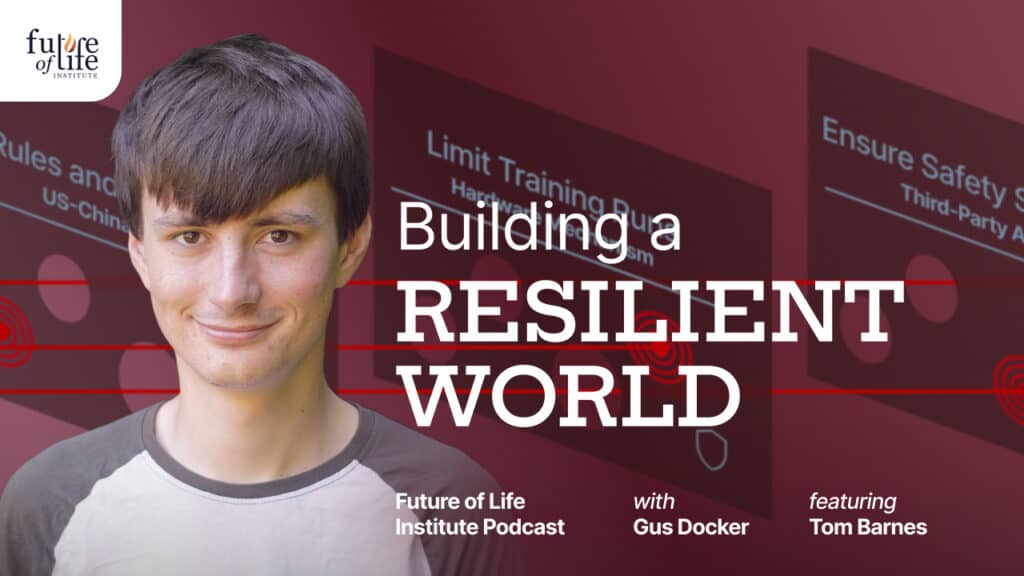