Not Cool Ep 16: Tackling Climate Change with Machine Learning, part 1
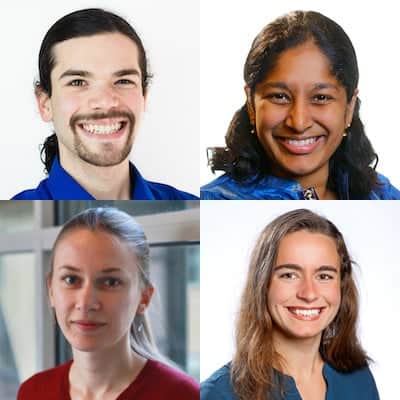
How can artificial intelligence, and specifically machine learning, be used to combat climate change? In an ambitious recent report, machine learning researchers provided a detailed overview of the ways that their work can be applied to both climate mitigation and adaptation efforts. The massive collaboration, titled “Tackling Climate Change with Machine Learning,” involved 22 authors from 16 of the world's top AI institutions. On Not Cool episodes 16 and 17, Ariel speaks directly to some of these researchers about their specific contributions, as well as the paper's significance more widely. Today, she’s joined by lead author David Rolnick; Priya Donti, author of the electricity systems chapter; Lynn Kaack, author of the transportation chapter and co-author of the buildings and cities chapter; and Kelly Kochanski, author of the climate prediction chapter. David, Priya, Lynn, and Kelly discuss the origins of the paper, the solutions it proposes, the importance of this kind of interdisciplinary work, and more.
Topics discussed include:
- Translating data to action
- Electricity systems
- Transportation
- Buildings and cities
- Climate prediction
- Adaptation
- Demand response
- Climate informatics
- Accelerated science
- Climate finance
- Responses to paper
- Next steps
- Challenges
References discussed include:
The paper is divided into three groupings: climate mitigation, adaptation, and then the third overarching grouping is tools for action — where we see machine learning providing tools for individuals or society to have an impact on climate change solutions.
~ David Rolnick
Transcript
Ariel Conn: Hi everyone, I’m Ariel Conn, your host for Not Cool: a climate podcast. And I’m excited to announce that we have a special two-part episode this week. Earlier this summer a very large paper came out called, Tackling Climate Change with Machine Learning. This paper was written by 22 authors from 16 of the world’s leading AI institutions in academia and industry. On today’s episode, which happens to be #16 of the Not Cool series, we’ll be chatting with four of the authors. We’ll talk about why machine learning can be such a valuable tool in the fight to keep global temperatures below 1.5C, as well as how machine learning can be used to help improve our electric systems, our transportation systems, our buildings and infrastructure, plus some of the challenges still to be addressed when applying ML to climate change solutions, and so much more. Then on Thursday, we’ll talk with two more of the authors about how machine learning can be used by individuals to improve their own carbon footprints and by societies to implement better climate policies. But for now, I’m going to break with tradition and let my four guests introduce themselves today. David, do you want to take it away?
David Rolnick: Thank you so much Ariel. It's a pleasure to be here. I'm David Rolnick. I'm a postdoctoral fellow with the University of Pennsylvania. I'm a mathematician and I work on the mathematical foundations of machine learning and AI. And I also obviously work on machine learning and climate change.
Priya Donti: Hi, my name is Priya Donti. I'm a PhD student in computer science and public policy at Carnegie Mellon university and I work on the intersection of electricity systems and machine learning. Specifically, how can we reduce greenhouse gas emissions from the electricity system — for instance, by helping integrate more solar power, wind power, and other low carbon electricity sources.
Lynn Kaack: Hi, my name is Lynn Kaack and I'm a postdoctoral researcher in the energy politics group at ETH Zurich. My work is centered at the intersection of climate change policy and machine learning, and I'm focusing a little more on the climate change policy side. So for example, I use machine learning to help understand how we can reduce greenhouse gas emissions from transportation. I'm also analyzing how renewable energy technologies get developed and how we can apply policies to promote that. I'm also using machine learning for that.
Kelly Kochanski: I'm Kelly Kochanski and I'm a PhD student at the University of Colorado, Boulder where I work on snow science and polar science. Recently I've been working on finding ways to integrate machine learning into climate models.
Ariel Conn: All right. Thank you all so much for joining us today. Let's just start by talking about maybe some of the really broad, basic background of the paper, as in: what brought you all together to work on this?
David Rolnick: So for a long time, researchers and practitioners across many different fields have wondered how we can use our skills to tackle problems relevant to society — and in particular, one of the greatest problems that society has ever faced, namely climate change. Over the past few years, this has become particularly urgent. This particular project started really with conversations I was having with Yoshua Bengio and others at this Institute in Montreal, Mila, where many of us were trying to identify how we can use machine learning to tackle problems posed by climate change.
Simultaneously, many other people around the world are having similar conversations. The project got started because I felt that perhaps some of these conversations could themselves be a vehicle for impact, by enabling many people with very powerful skills across the field of machine learning to use those skills and leverage them to impact problems relevant to climate change. So the project has really involved a lot of people coming together, not just from the machine learning field, but also many other fields relevant to this interdisciplinary work — because to have an impact on problems related to climate change, one really needs a lot of expertise coming in from many different areas, as you can see from the authors which we gathered to work on this paper.
Ariel Conn: How did you all find each other?
Priya Donti: So how I first heard about this project is at the NeurIPS Machine Learning Conference, David organized a lunch for climate change and machine learning, during which a lot of different people were asked to speak about their existing work in bridging these two areas. David invited me to speak, and that sparked a lot of really interesting conversations, and it seemed like there was so much passion around this project. Importantly, there was a lot of conversation about what we could do moving forward beyond that lunch, beyond a couple of presentations. How could we really put something together that would inspire broader action from the machine learning community? Later on, when David was thinking about this paper and talking about this paper, yeah, he approached me and my interest had really been sparked by that lunch.
David Rolnick: The goal of bringing together this diverse set of authors was really to cover a wide range of needed expertise. Because I realized that I certainly did not have anywhere near the field-specific expertise to cover the individual areas into which we'd have to delve, in order to assess what kinds of problems and solutions were relevant in applying machine learning to climate change. I think everyone on that author list brings their own special expertise which is necessary for tackling one facet of this problem.
Ariel Conn: Why did you focus specifically on machine learning?
David Rolnick: I don't feel that machine learning is the only tool for which such a paper should be written, or for which such an investigation should be made. It was a community that I and other authors are part of, and it is a very powerful, broadly applicable tool as a vehicle for enabling and accelerating progress. But it's far from the only such tool.
Lynn Kaack: Maybe I can also add with a perspective coming from the climate policy side. During my PhD, I felt that there was a gap because more and more big data sources were coming online and we were not addressing or taking this data into account. I personally got into machine learning and statistics on the side of my PhD, initially for fun actually. I realized that combining machine learning with these domain expertise techniques that we developed to mitigate climate change was actually a really fruitful approach.
Kelly Kochanski: I think that one of the advantages, one of the really satisfying things about working on climate and earth science, is that you get to work on problems that have a really wide global scope and interest. But one of the challenges is trying to figure out a way to translate that interest into actual impact, on rapid time scales, that can actually improve people's lives as climate change happens to us. And so for me, one of the ways that I'd like to try to increase the impact, or the time to impact, of the work that's going on in my field is to bring in new computational techniques and to help people in my field work together with larger communities and start spreading our impact further in a relatively short period of time.
So this was a way for me to work on something that had a lot of that energy and had new tools that might allow us to make improvements to what we're doing within the next few years, while we can start impacting infrastructure and policy decisions with better climate knowledge.
Priya Donti: Yeah, and I would say more broadly, climate change is a problem that affects everybody. We will need people of all expertise, stakeholders of many sorts, to come in and contribute to the issue. We felt that, as part of the machine learning community, we had something to say about how machine learning specifically could contribute. In particular, as Lynn mentioned, there are lots of areas where there's a lot of data and there isn't necessarily a lot of guidance about how we can take that data and translate it to action.
Machine learning is a particularly well suited tool for doing that. But again, machine learning doesn't exist in a vacuum: It has to be applied alongside stakeholders and alongside collaborators who understand the climate change domains that we're talking about in the paper a lot better than we might. So machine learning is one piece of a much larger effort.
Kelly Kochanski: One of the challenges of working on this is that a lot of the tools that we can make have a pretty distant impact in time. We can build better predictions of how the climate is going to change, but putting new science into these predictions can take several years; and then those predictions aren't impactful until they've been acted on by individuals, governments, and people making, say, infrastructure decisions influenced by climate. So for me, one of the advantages of moving into machine learning is that it allows us to bring in new tools and to connect with really energetic technical communities, and start finding ways to make predictions on a more rapid scale — or make new kinds of predictions that get information out to more people faster, and let them make effective decisions.
David Rolnick: I second everything that Kelly just said, and I would add to that — but from the machine learning point of view — there are so many people desperate to do good, and use their skills for the good of society, who don't know how best to do that. Enabling them to connect with problems and people who can channel those skills to the maximum possible effect is really what we're trying to enable here.
Ariel Conn: So I think that's a really nice transition to the next question that I had for you, and that's getting a little bit more into the specifics with the paper. At the start of the paper, you have a very nice table that lists the climate change solution domains and the areas of machine learning that you see relevant to each. I was hoping you could talk a little bit about how you narrowed down all of the options to the list in this table.
David Rolnick: Sure. So some of the machine learning domains which we listed in that table we picked based upon major areas in which people had expertise; also, major areas which we see as up and coming and in need of further development within the field of machine learning. So for example, some of the areas we highlighted — computer vision, natural language processing, reinforcement learning — are areas of major research and entire sub fields of their own. Others are up and coming, and identified by many within the machine learning community as areas where great progress is being made or has the potential to be made.
For example, interpretable machine learning, or transfer learning, and few-shot learning. These are areas where we actually see the climate change applications we identify as being potentially very valuable in guiding and giving rise to improved machine learning from a research and an engineering perspective.
Lynn Kaack: We picked the climate change domains based on how this problem is usually split up in the literature. We added some sections that are typically not caught like that, so for example the tools for individuals, tools for society, which we thought were overarching themes that ML could help with, which we wanted to identify separately.
David Rolnick: Overall, the paper is divided into three large groupings. The first of those groupings is climate mitigation. That refers to reducing greenhouse gas emissions. The second overall grouping is adaptation, so helping society respond to those changes that are inevitable or are happening now. Then the third overarching grouping, which is perhaps less conventional, is tools for action — where we see machine learning having an impact, for example, in providing tools for individuals or society to have an impact on climate change solutions less directly.
Ariel Conn: All right. So I'd like to start digging in a little bit more with this paper. You mentioned the first section is mitigation. Within that, obviously, our electric systems are some of the largest causes of greenhouse gases. So Priya, did you want to start off by just explaining a little bit about the work you did on the section about electric systems?
Priya Donti: Absolutely. So electricity systems and machine learning — this intersection is something that is really fascinating to me, in particular because electricity systems touch a lot of our lives. They shape the way we work, we live, we commute — and at the same time electricity is very invisible, and the situation for electricity systems is very different depending on where in the world you are. A lot of people in the world don't have access to electricity at all. And so when you have a system that is so globally ranging and is also a huge contributor to greenhouse gas emissions — electricity supply and production contributes about a quarter of global greenhouse gas emissions — how do you even start to tackle this problem?
So there are three ways in which it's generally envisioned that we would reduce greenhouse gas emissions from electricity systems. The first is moving away from greenhouse gas emitting fossil fuels — so these are things like coal power or natural gas — and move towards low carbon electricity sources — like solar power, wind power, nuclear, geothermal, hydro — and run our system based on these low carbon sources rather than greenhouse gas producing sources.
The second method is, the transition to low carbon electricity sources isn't going to happen over night. So in the meantime we have to do something to reduce the greenhouse gas emissions from the system as it currently stands. Another method that we can think about machine learning being applied to is how, in fact, do we reduce greenhouse gas emissions from fossil fuels today. Then a third kind of overarching theme is, while we think about transitioning to low carbon sources or we think about reducing greenhouse gas emissions from our current system, we want to make sure that we think about these solutions in a global manner.
So not only in places where we have a lot of data, for example in the United States, but also in places where we may not have as much data, but that may be equally important from a climate change mitigation perspective. So machine learning can contribute to all of these strategies: to the transition to low carbon sources, to reducing emissions from our current system, and to the global implementation of these through a variety of applications. This was something I was really excited to see as I was putting the section together.
There are applications ranging from forecasting solar and wind power, to detecting methane leaks on the electricity system, to helping accelerate the search for solar fuels or to accelerate discovery in nuclear fusion, and then to also generate data in settings where we may not have a lot of traditional data streams. We may not, for example, have information about how much power each solar plant is producing in a given time, but where we may have something like satellite data that allows us to gain insights into what's going on in a particular system. So there are really a lot of different ways that machine learning can contribute to reducing greenhouse gas emissions in electricity systems.
Ariel Conn: So one of the things that I didn't really mention at the start, but it might be useful to bring up here, is there's three categories that you've all applied to some of these machine learning solutions. One is high leverage — something that you think will be especially effective. Long-term are solutions that you expect will primarily be impactful after 2040. High risk solutions are solutions that could be really helpful, but there's also some unknowns about potential side effects or other ways that they could be risky. A lot of the questions that I plan to focus on through the rest of this focus more on the high leverage.
So I don't know if you guys want to jump in on that breakdown real quick, but I was hoping to also switch to some of the sections that you found more high leverage within the electric systems work that you did.
David Rolnick: Sure. I'd be happy to briefly discuss the breakdown here. I think long-term speaks for itself. Those are the solutions which are going to have their maximal impact many years down the road, but are still extremely important. High leverage we see as those problems that have both high impact in either reducing greenhouse gas emissions or helping society adapt, but also have key elements which would strongly benefit from machine learning: so those high impact pathways forward where machine learning has very high leverage.
The third flag which we introduce is high risk, and that can refer to several kinds of risks. First, there are some solutions for which the technology is really not yet certain. For example, nuclear fusion would be a game changing technology, but has not yet been proven to be successful at any kind of scale. Second, there's sometimes uncertainty as to the impact on greenhouse gas emissions. In many situations the systems involved are very complex, so it's not certain that any particular action would have an out-sized impact on climate mitigation, reducing emissions, though it might.
Then the third kind of risk is if there are potentially unwanted side effects, what might be called negative externalities — so solutions that help from this perspective of climate change but may have other negative impacts in other ways. So for example, biofuels may be an essential component of a future climate change solution where it is necessary in many cases to have liquid fuels on which vehicles can run.
Lynn Kaack: To grow bio crops, you have large land use changes, and of course they use water, and there's the fear that they would take away from growing food on fields. So we have all these unintended side effects with technologies and it's important to take those into account.
Ariel Conn: All right, excellent. Thank you. So Priya, why don't we go back to you? Let's start with the high leverage sections in the electric systems section. One of the ones that I noted was the generation and demand forecasting. Can you talk a little bit about that and why that's important and what it means?
Priya Donti: Sure. So before talking specifically about generation and demand forecasting, let me step back and give a little bit of context. Electricity systems are a really delicate balancing act in some sense. There is electricity supply, so the production of electricity that is feeding into the electric grid; and then people are consuming electricity, so pulling electricity out of the grid. The power going into the grid has to equal the power coming out of the grid at every single moment. This is complicated by a couple of factors. On the electricity generation side, if we're looking at sources like solar power or wind power — whose production is dictated by the weather — we don't know exactly what the weather is going to look like ahead of time. So there's a little bit of uncertainty about how much solar power or how much wind power will be produced ahead of time.
If you have controllable electricity generators like coal, natural gas or nuclear, these are electricity generators that we can turn on and off explicitly, but these are still governed by some physical constraints. There's only so fast that you can turn one of these electricity generators on, or so fast that they can change how much power they produce. So you have some uncertainty or some delay — both in terms of variable electricity sources like solar and wind; and controllable electricity sources like coal, natural gas, and nuclear.
Then on the demand side, you don't always know exactly how much electricity somebody is going to use, because humans have free will: I can decide to turn on my electric kettle right now if I want to, or I don't have to. So now we have this complex balance between supply and demand, where supply is potentially unknown ahead of time, and demand is also potentially unknown ahead of time. So this is where the machine learning solution can help. Generation and demand forecasting is an application machine learning can contribute to by looking at historical weather data; by looking at information about previous demand, about previous electricity generation from wind and solar sources; by looking at is it a holiday today, and even using video data, video of clouds going over a particular solar panel or satellite data.
Machine learning can combine all of these different data streams and use them to produce forecasts of what we think solar power production or electricity demand will look like, maybe later today, or a few days from now, or a few years from now, even, if you combine it with physical models. There's some interesting technical challenges that come up here. So for example, weather is governed by physics. So is there a way to incorporate information about physics into machine learning models to enable them to make better forecasts?
There's also this question of, if you create a machine learning forecast — let's say I say my demand is going to be a certain amount tomorrow — how certain am I about that forecast? Can I give some probability estimate or some error bars on that forecast, or can I maybe explain why exactly I think that's going to be the case, so that a human electricity system operator can look at the forecast and sort of verify whether what it's saying is reasonable or not? So this is an application where generation and demand forecasting can contribute to how electricity system operators manage their electricity system, and both make them more comfortable introducing more low carbon electricity sources and also can help even today reduce the amount that they rely on fossil fuel generators to maybe buffer some of the uncertainty in supply and demand in the future.
Ariel Conn: So do you envision us being able to use machine learning to help with the transition from some of our current systems to some of the low carbon electricity sources?
Priya Donti: Yes. So in order to transition from our current system to low carbon electricity sources, one of the things we definitely need to get a handle on is how much solar power we think will be produced in the future, how much wind power will be produced in the future. Because we need this information to decide how exactly to balance our electricity system to make sure that it continues to provide electricity without, for example, collapsing or having some kind of fault. There's a lot of data that's coming into the system here — grid sensors, weather data, etc. — and making sense of this data can be very difficult for an electricity system operator.
So machine learning techniques can help here by basically giving some data-driven insights to electricity system operators, and as a result, enabling them to manage the electricity system. So I've been talking about forecasting and variable electricity sources, but I mentioned earlier that controllable electricity sources are ones that we can turn on and off as we would like, though they are subject to some physical constraints. This is why nuclear fusion is also discussed as an interesting solution, and one that machine learning people like to contribute to. Because nuclear fusion — basically I would call it mankind's quest to recreate the sun here on earth — basically takes hydrogen atoms, superheats them, and reacts them together in order to produce energy just like you would do on the surface of the sun.
You can control how much electricity comes out of a nuclear fusion reactor at any given time. And machine learning can help innovate and create these kinds of new electricity sources by helping more efficiently run experiments, or by making sure that when an experiment is run that you can do something like detect whether plasma — superheated hydrogen — is going to hit the site of a reactor, and therefore damage it. So in solutions like this, in solutions like accelerated science where machine learning can help speed up the search for new clean energy materials like solar fuels, I think there are lots of different ways that machine learning can help us transition into a low carbon system.
David Rolnick: I know I found it really surprising just how important forecasting is, both on the demand side and on the production side. It's really counterintuitive, perhaps, that not just having not enough solar power is a problem, but also having too much. So understanding exactly how to make supply and demand be equal, and route power appropriately in a grid, is just so important.
Ariel Conn: When you say that too much is a problem, do you just mean that we can't store it, or is there some other issue with having too much solar?
Priya Donti: You can put batteries, for example, or other storage devices on an electricity system — but generally speaking, you don't have as much storage as you do capability to produce solar power. So if all of my solar panels started producing at 100% and I wasn't anticipating that, I might not be able to store all of that electricity immediately. In part this is because actually electricity storage can be expensive. Then also, even if you do want to store electricity from a solar panel, you need to know when exactly you want to charge your battery. And knowing that also requires some understanding of when exactly you will have solar power production.
Ariel Conn: So the examples that you used for how machine learning can be applied with nuclear fusion and new materials are both listed as high risk, I assume because we just don't know if we can develop the technology. One, if that's incorrect, let me know. But then two, I was also curious if you could give some more examples of more certainty, where we can see machine learning being applied that would be high impact.
Priya Donti: So in this case, the reason we listed accelerated material science as high risk is, exactly, because these are technologies in development, and we don't necessarily know if or when they will pan out. So we do think these are very impactful applications for people to work on, which is why we also list them as high impact. But they are definitely, in our perception, a longer term scientific endeavor — as opposed to something that can be implemented today. So generation and demand forecasting, which we've talked about, is one application machine learning that can be implemented today and on today's systems with electricity system operators.
Another one is the detection of methane leaks. So methane is a really potent greenhouse gas. It's 30 times more efficient than carbon dioxide at capturing heat, so really potent greenhouse gas. And methane is actually the main component in natural gas — one of the fuels I mentioned earlier that is used today to produce electricity. Methane is transported to natural gas plants via pipelines or through compressor stations, and can leak out of these pipelines, out of these compressor stations in the process.
The issue with that is we don't necessarily know exactly when or where these links are occurring and we also don't necessarily know how big of an issue this is, so how much methane is leaking. The estimates vary quite broadly. Machine learning can help provide some insight here by taking information from sensors that may be on pipelines; by taking satellite data and particularly hyperspectral satellite data — so satellite data that looks at different radiative bands as opposed to just light. So you can take sensor data and hyperspectral satellite data and combine all this information to both detect where methane leaks may be occurring already, but also potentially predict where methane leak may occur so that the problem can be fixed ahead of time and a methane leak never occurs.
Ariel Conn: Excellent.
David Rolnick: I'd love to stress that really every one of our recommendations has been very carefully thought through. Even those which we don't flag as especially high leverage are still high leverage. We expect and hope that people will be working to implement all of our recommendations, because really all of them are useful and all of them are necessary.
Lynn Kaack: One thing to add there too is that there are a lot of applications that have really high impact, but just don't benefit from machine learning as much.
David Rolnick: For example, we didn't talk very much about diminishing the effect of hydrofluorocarbons, HFCs, but that's hugely impactful. It's just not something where it's very clear how the applications of machine learning could be involved.
Ariel Conn: Okay, good. I think that's a really good point to make. Were there any other applications within the electric systems section that you think are important or helpful to mention?
Priya Donti: So as I mentioned earlier, electricity systems are everywhere. They're a global phenomenon, a global issue. So it's really important that we as machine learning researchers, and also the climate community, think about how we can mitigate greenhouse gas emissions in various global contexts. And so one thing I'd like the machine learning community to keep in mind is that, as we innovate on electricity systems, we don't just want to focus on settings where we have a lot of data. We want to make sure that we're thinking about electricity systems everywhere, including systems that don't have a lot of data. One thing machine learning can potentially have a lot of leverage in doing is in producing new insights from non-traditional data streams.
For instance, we can use satellite data to detect which power plants are producing greenhouse gas emissions at a given time. Or there was one paper that used cell tower data in order to understand how much electricity people were using at a given time. So somehow leveraging these nontraditional data streams can help us innovate on electricity systems everywhere.
Ariel Conn: All right. Thank you so much. Lynn let's switch gears over to you — we're sort of going in order for the paper, and transportation was the next big section, which you wrote. So I was hoping you could just start by giving a quick overview of some of the work you did and what your findings were.
Lynn Kaack: Transportation is actually a sector that's really important to look at if you want to reduce greenhouse gas emissions. It's responsible for 14% of total greenhouse gas emissions in the world, and in the US for example, it’s about as much as those greenhouse gas emissions from electricity generation. When we look at transport, we should not only look at passenger transport, but also at add freight transport, which refers to goods being transported. Those actually account for about half of the greenhouse gas emissions from transportation. And machine learning has a number of different ways that it can help research on passenger and freight transportation. It can, for example, improve vehicle engineering — so make that more efficient. It can enable intelligent infrastructure. And it can also provide policy relevant information.
Ariel Conn: So there were a couple of things that you found high leverage, and hopefully we'll get into that. But one of the things that I found interesting was that autonomous vehicles and shared mobility are obviously things that could help with climate change, but they were also listed as high risk because they could potentially increase emissions. So I was hoping you could talk a little bit about what you found for both of those areas.
Lynn Kaack: Sure. The energy impact of those two technologies are still unknown because they are not deployed yet to that scale that we can have this data. And there are several factors that play a role. So I'm going to dive into both of these and illustrate two factors that might be reducing the greenhouse gas emissions or increasing the greenhouse gas emissions for both of these technologies.
So autonomous vehicles crucially rely on AI, and they could be good for the climate if they enable cars to drive in a more efficient way, which is also sometimes referred to as eco driving. But on the other side they might lower the barrier to using your own vehicle instead of public transportation, which actually might increase the amount of trips that people take in their own vehicle, and which also increases the greenhouse gas emissions from those vehicles. When it comes to shared mobility, this is a topic that encompasses everything from electric scooters to a group of neighbors sharing a truck. But we probably should talk about ride hailing services such as Uber and Lyft, because to date those are the most impactful applications. Perhaps a toy example here is best to illustrate why the energy impact of these technologies is still unclear.
So for example, if you want to visit a friend, you might drive there with your own car, so you drive there and back directly with your car. But instead if you use such a ride hailing service, it will come from somewhere, pick you up, drop you off, and go somewhere else to pick up a new person. So the journey that the vehicle has moved can easily be much longer than the journey you would have taken with your own car. If you’re using a normal combustion engine car, this probably increases the greenhouse gas emissions that come from that trip. But there is the possibility that these services enable a faster transition to electric vehicles, and they might also enable pooling many people in one vehicle.
Those factors would have a much better climate impact. So there's really the need for these industries to prioritize lowering greenhouse gas emissions, and I think there could be quite some potential to contribute to that.
Ariel Conn: So in the example of autonomous vehicles, that's still in the future. Shared mobility, we're seeing that now in terms of the ride sharing. What are some of the things that we can do right now to reduce greenhouse gas emissions from transportation, and how does machine learning play a role in that?
Lynn Kaack: Most greenhouse gas emissions actually come from trucking. Trucks are really hard to electrify with batteries, because those need to be very big to transport all that heavy freight. But this might not always be that way. So we hope that battery development is leading us to having lighter, cheaper, and more powerful batteries, and machine learning can be used for battery development.
There's another technology that's actually relying on autonomous vehicle technology and information communication technologies, which is called platooning. And this is an idea that you could allow trucks to drive really close to one another and that has actually less air resistance and can lead to a better fuel economy. But this technology is still kind of out in the future.
One thing we can do right now is to incentivize that more freight travels on rail instead of on trucks. Typically what happens here is that you bring the freight, probably packaged in a shipping container, to the train; and then it travels for long distances on a train; and then gets picked up again by another truck to its final destination. This is referred to as intermodal, and making intermodal faster and more reliable with machine learning could actually have quite some leverage. So for example, we can use machine learning to predict demand, predict when the vehicles are likely to arrive, try to prevent failures by predictive maintenance. We can also use it in information communication technologies too — for example, truck containers.
Ariel Conn: How much of what you're suggesting right now can be implemented either now or in the very near future?
Lynn Kaack: So people are actually beginning to implement these technologies already. This is still in the beginning, but the industry is definitely really looking at machine learning. It's really important here to optimize for greenhouse gas emissions instead of for costs. Because of course the industry will optimize for costs, but we need ways to also look at the energy side of this equation.
Ariel Conn: Do you see ways in which machine learning can be used to help these industries optimize for both cost and decreased emissions?
Lynn Kaack: Sure. I mean, there are win-win situations, of course. If you have predictive maintenance, you actually make rail cheaper and this is great for everybody. So it depends on the application. Sometimes the incentives are aligned, sometimes they're not.
Ariel Conn: So let's switch to another section. You marked modeling demand as high leverage, and I was hoping you could explain what that means and why it could be so effective.
Lynn Kaack: Many countries actually know much less about their transportation systems than you would expect, but of course this information is needed to make transport systems more efficient and also to plan new low carbon infrastructure. Machine learning can help to both make sense of data that is already collected, and it's also really great at forecasting. It can actually be used to help modeling efforts, and it can also help to infer primary data from your sources; for example, it can use satellite images to estimate how much traffic is going on.
David Rolnick: I think this is a great example of something we've seen many times in the paper: that sometimes the most impactful applications are really not sexy, but are fundamental to how stuff actually gets done in the world.
Ariel Conn: Let's come back to that, because I think that's a really important point. Lynn, I want to keep talking about transportation for another couple of minutes. Closing in on my final questions for transportation is just how machine learning can be used to improve things like electric vehicles.
Lynn Kaack: Electric vehicles are actually one of the main solutions for reducing greenhouse gas emissions from passenger transportation. There are actually a lot of things we still need to figure out about electric vehicles: how to make them better; understand how people use them and what that means for the existing infrastructure. This is especially the case since more and more electric vehicles are going to be used, and they might for example, be all charged at the same time. So understanding the time and place where they are charged both influences where we would place new charging stations, but also how to manage the grid. This ties in a little bit with Priya’s section. So we want to enable information to do better forecasting and know when vehicles are charged.
For example, it’s expected that everybody comes home from work around the same time and then plugs in their vehicle, which might actually lead to a large spike in electricity consumption. Machine learning is really great at making sense of large data, and it could help to extract such behavioral patterns. But it's also central to algorithms that help manage the way many vehicles can be charged without risking congestion, and also to manage better these large peaks and electricity demand by distributing them better over time.
Machine learning can also be used to improve electric vehicle technology — in particular, battery technology. Machine learning is currently used for predicting the battery state, the degradation, and the remaining lifetime, for example.
Ariel Conn: You also mentioned some other low carbon options that machine learning can be used for, like bike sharing and electric scooter services. Can you talk a little bit more about that as well?
Lynn Kaack: So bike sharing and electric scooter services are great examples of how fast new mobility solutions can be implemented. But they also show that there's some need to regulate those new services to make things work. There is, for example, something that’s called the bike sharing rebalancing problem, and it refers to the fact that all the bikes in the end of the day, for example, end up at the same spot — and some other bike stations or areas where you usually would expect them are empty. So machine learning or AI applications can help to predict these things happening better and help to rebalance those stations.
Ariel Conn: Excellent. I'm going to keep going with you for just a few more minutes because you also worked on the buildings and cities section, which was a very interesting section as well. So can you talk a little bit about the current impact that buildings and city infrastructure has on greenhouse gas emissions and how machine learning can address that or help address that?
Lynn Kaack: Sure. Buildings is actually really important end-use sector because about a quarter of energy related emissions are from energy that's consumed in buildings: so for example to heat or cool them, or to cook, or to power our laptops. Buildings are not only personal homes, but they also include office buildings, stores, and even industrial buildings. And we can use machine learning to reduce the energy that's consumed in buildings in two main ways. The first one is to manage the energy use and to also reduce it with intelligent control systems, which is sometimes referred to as smart home, for example.
The other way is to use machine learning to help design better policies, where policy makers need to rely on great data for example, and machine learning can provide this type of information.
Ariel Conn: You described buildings as offering some of the lowest hanging fruit to reduce emissions. Can you explain that?
Lynn Kaack: This is based on the fact that it is possible to reduce the energy consumption from buildings with existing technological solutions by quite a bit. So some people estimate there's up to 90% less energy used in those buildings. A lot of these solutions actually pay for themselves in a really short time. So the problem here is usually on the behavioral side, not on the technological side.
Ariel Conn: Can you give some more specific examples about how machine learning can help improve building efficiency? And can you talk a little bit about the extent to which we can actually turn current buildings into smart buildings? What can we do now or in the very near future?
Lynn Kaack: Machine learning as a part of intelligent control technologies can help reduce greenhouse gas emissions in two different ways. It can reduce the energy that's consumed in a building by helping to forecast and control, for example, how heating and cooling is adjusted according to the user preferences. It can also detect the occupancy in a building with sensors and turn, for example, heating and cooling off if it's not needed. Intelligent systems, for example, can decide to also ramp up cooling if it has learned that a family is likely to come home soon.
So these are all applications that could fall under the umbrella of smart home. And I think it's fairly possible to retrofit current existing buildings with smart technologies, because those are based on appliances, so they don't depend as much on the actual building. Of course, they work much better if the building is well insulated, for example, so they cannot be viewed in isolation.
Another application of these systems is by helping with integrating renewables into the electric grid. For example, many office buildings actually have cool storage for their AC, which can be imagined, and is almost exactly a large block of ice. That block of ice can be seen as a form of energy storage, where one can decide at what point in time one wants to freeze more water. So one would likely do that when it's beneficial for the grid. Today, this is often done manually by the grid operator calling a large consumer — for example, like a university campus — to stop those cooling operations when they're undesirable and to previously generate a lot amount of ice in order to have that ready.
So, for example in Germany now, there are certain times where there's so much electricity generated from renewables that actually exceeds the demand; and we would want in such moments to have this type of technology ready to be able to use that electricity beneficially, and then reuse it later for cooling buildings again. AI based algorithms can actually take the role of the operator, and instead of calling up somebody, they would give a signal, and they would optimize the way that it’s previously frozen and then use it on later.
Priya Donti: I would say that this application area of demand response is one that recurs in a couple of areas of the paper — where basically in commercial buildings, or when you have industrial equipment using electricity from the electricity system, we want to make sure that as much as possible it is using electricity when we have low carbon sources producing power. This concept of demand response, as Lynn says, is often done today by an electricity system operator literally picking up the phone and calling a building operator to say, "Please do this or please do that." This is a great application where automation and machine learning can really help make this transaction more efficient.
Ariel Conn: Do you see an application like this then also being able to be applied to maybe smaller buildings that don't necessarily have a maintenance operator, or do you see that as not an issue?
Lynn Kaack: So that's definitely the idea, and it has been done, and it is currently also being done. Residential hot water storage can be used for the same purpose. So it's definitely the idea to do that in people's homes as well.
Ariel Conn: Is there anything else that you think was really important from the buildings and cities section that's useful for either ML researchers or maybe policy makers to know about?
Lynn Kaack: Yes. There's this whole side of urban planning and also policies that help to reduce the energy that's consumed in a city. Policy makers are passing laws to make buildings more efficient, and they need to know a lot about the buildings in the city to understand how effective a policy is and how it could affect also certain populations in the city. One thing to notice here is that buildings are very heterogeneous — so they have different sizes, age, users, usage, ownership, etc. Machine learning can help to understand both energy consumption patterns if there is data available — it can help, for example, to cluster consumers into different groups that help policymakers understand how to shape a policy.
But often there's also very little data about the energy consumption in the city, and machine learning plays an important role for generating useful data. You can learn energy consumption for a whole city from all kinds of data points. People have used the sizes of houses, property class — all data they could basically find predictive for the energy consumption. And machine learning can even help to generate some of this more primary data; for example, how large buildings are from satellite images. This is really important because this kind of data is not available for many regions in the world.
Many of the mega cities in emerging economies are lying in very hot regions, so as people earn a better living, they will probably want air conditioning, for example. Designing low carbon systems for air conditioning is very desirable and will be very relevant in the near future.
Ariel Conn: Excellent. Thank you. Kelly, let's switch over to you now. You worked on the climate prediction section within the larger adaptation section. Can you talk a bit about some of the work you did in your findings, regarding climate prediction and machine learning?
Kelly Kochanski: Sure. When I wrote the climate prediction section, I was looking for applications that would give us more accurate, more usable predictions for how climate and weather are going to evolve over the next few decades. Most of this is centered around climate models. Climate models are tools that make predictions, usually on the scales of years to decades, about how the climate is going to shift. And for the last maybe 20 years now, they've been the best tool that we have for predicting what the magnitude of climate change is going to be — and increasingly, as we've developed these models and improved their resolution — for figuring out more specific trends, like rates of change of temperature and precipitation in different regions around the globe, that we can use for planning and for figuring out what kinds of changes different agricultural regions and countries and cities will have to respond to.
So when I was writing this section, I found a whole host of really exciting opportunities to use machine learning to improve the accuracy and capability of existing climate models; and also to make some new structural changes, like new models that learn rapidly from data in real time and quickly incorporate new observations from satellites, or that make hyperlocal predictions to allow individuals or businesses to figure out the future of a specific building, for example, that they might want to buy or live in. So I think there's a really wide range of applications that machine learning can be used for in this space.
Ariel Conn: One of the sections was titled “Uniting data, machine learning, and climate science.” And it seems like this is one of the most obvious applications of machine learning, since it's taking the data that we have and applying it to climate science. I was wondering if you could talk about some of the work that's already being done to unite these three things, and how much more we could be doing.
Kelly Kochanski: Sure. First off, I think it may be obvious to say that we have data; and we do have data — we have petabytes of data from satellites these days and that we should use that to make better predictions. But it's not actually obvious or easy to find ways to integrate that into climate models. So when we look at long-term climate predictions, there's a fundamental and irremediable data problem that we have, which is that the earth generates about one year's worth of data per year exactly. No matter how well we observe that and how much data we generate, we can't get more than that.
And we can't make the few years of observations that we have stretch out to give us predictions to, say, years like 2050 or 2100. So most of the highest impact solutions that I've identified have involved using that data and putting it into the framework of existing models, which usually rely less on data and much more on physical laws — like energy conservation and thermodynamics — that we trust will hold constant and allow us to make more reliable predictions.
So one of the examples that I'm most excited about right now has to do with clouds. Clouds are actually one of the biggest sources of uncertainty in climate models. You can visualize this to some extent from the ground. So for example, I live in Colorado. Most of the time it's very sunny, and that means that when a cloud comes through, it blocks the sunlight and it makes the ground cooler. But in winter, we get the opposite effect: if it's cloudy overnight, the cloud acts like a little bit of a blanket, and it traps in some of the warmth from the city in the ground, and it makes that winter night a little bit warmer.
And we see these kinds of effects happen on a global scale all the way around the world, with some clouds cooling the earth and some clouds warming the earth and blanketing it. And it turns out that the differences between the two have to do with a lot of micro cloud physics, and tiny particulates in the air, and subtleties of location and temperature and thermodynamics that are really hard to model on a global scale. But we can model them on a small scale. So some of the most exciting research on this at the moment has to do with taking really small, high resolution, expensive models of clouds — sometimes even single clouds — and training machine learning models to replicate the results of those expensive models in a relatively cheap way that we can then put into big global climate models to make our predictions better.
This is a technique that's called emulation, or sometimes surrogate modeling, where we train a machine learning model to act like a physics-based one. It's being used in an increasing number of areas in climate science, and I believe that there are still many more where it could have a great impact, where it hasn't yet been explored.
Lynn Kaack: This is, for example, also used for emulating physical models of buildings. And it has applications throughout the whole domain, which is something that we identified with this paper as well.
Priya Donti: Yeah, and it also comes up in electricity systems: this question of essentially how do we take a very large complex physical model for which we can write down all the physics that we want, but as a result it takes a really long time to simulate this on a computer — how do we take that really complex model and simplify all or parts of it using machine learning and other data-driven approaches that are trained maybe on inputs and outputs of that model?
David Rolnick: Just to feature another section written by Anna Waldman-Brown on industry: there is a notion of a digital twin of some industrial systems where you can run experiments or otherwise improve your understanding of the system by having a digital version of that system, which is simulated using machine learning.
Ariel Conn: I guess the implication in your paper then is one, it can obviously be used in more places; and two, would it be fair to say that it would be helpful to have machine learning researchers who can help optimize the systems that are already being used?
Kelly Kochanski: So I think of this as a small piece of a larger trend, which is that machine learning is going to give us a lot more options for many of the decisions that we as scientific modelers make when we're building predictive models. So in this case, the decision is whether to use an expensive model that simulates physics that we know, we understand, we trust pretty well, and use that to get something that's cheaper to run, but possibly harder to interpret. Although, interpretable ML is going to change this I think.
There are a number of other areas where these kinds of transitions are likely to take place. For example, machine learning is also changing the way that people predict which codes are going to have bugs, or handle other technical challenges — like how to represent continuous ocean and cloud movement on a grid of discretely measured computational points. In all of these areas, they’re still under development. A lot of these are whole subject areas that have only started to come up in the last couple of years. And they're going to need a lot more research by machine learning specialists, as well as a lot of testing in different application domains, before we can figure out where these techniques work and where they don't. I think once we've pinned that down, it's going to leave us with a lot of areas of scientific simulation that are suddenly much more tractable than they were before.
Ariel Conn: One of the examples that you give in this section is how machine learning can help with forecasting extreme events. Can you talk a little bit about that as well?
Kelly Kochanski: Extreme event forecasting is actually something that the machine learning community has picked up a lot on recently. So for example, the 2019 Gordon Bell Prize just went to a project which visualized extreme weather events and weather fronts in very large climate datasets. There are a lot of things people are doing — for example, taking weather datasets or climate model output and looking for hurricanes, or looking for extreme patterns of heat and cold, and using those to gather statistics either for the past or for the future; showing how often different types of extreme events come up in different places.
I think that this work is going to produce results that will be quite useful for people like urban planners and city governments as they try to figure out what types of storms, droughts, and so on, their cities are going to need to respond to in future years. Right now I think a lot of the challenges in this area involve the integration between the machine learning groups and between both scientists and city planners, in order to take the new machine learning solutions that are being developed and make them work within tools that can actually be used either by scientists to make more precise predictions or by the people on the ground who need to respond to them.
Ariel Conn: What are some of the technical challenges that machine learning researchers face working specifically with the climate data?
Kelly Kochanski: Climate data has a lot of messy characteristics that don't tend to come up in benchmark machine learning data sets. One of the classic ones with extreme events is that the data sets are extremely skewed by their nature. Extreme events are very rare, and it's hard to find a labeled training data set for a machine learning technique that includes a significant enough number of those to do good training on. Another problem that we have in earth science is that a lot of what we know about the earth is learned from inference across a lot of different physical processes and solutions that we put together in order to form a coherent picture of what the earth and its climate had looked like.
For example, if we want to figure out what past weather looked like over the last 50 years, we might be able to look at weather stations, which have used increasingly good sensor technologies as they've evolved and have been distributed in an increasingly large number of countries and places. We have to figure out how to integrate the data using all of those different sensors and observational locations in order to build up a record that's long enough, 50 to 70 years, to be anything close to comparable to the distance into the future that we want to predict.
And so it's really important to have tools that can pull data from multiple sources with different natures and different quality levels and put it all together to form that one cohesive whole before we can make predictions. As a related point, one thing that a number of people in the earth science community have thought about is building benchmark data sets for machine learning people to work on that have characteristics that are more common of the kinds of data that we deal with in earth sciences, to try to make it easier for machine learning researchers to develop tools that earth scientists can use.
Ariel Conn: Can you explain what you mean when you say benchmark?
Kelly Kochanski: So some areas of machine learning research — for example, image recognition — have involved a lot of development around specific datasets that a lot of people train on. There's one in computer vision called ImageNet. And the advantages of these datasets is that people can look at them with many different machine learning techniques, and they can test optimizations, and they can quickly compare their code and their methods to other people's code and methods to figure out what works well for tackling different problems.
One problem that a number of people in the earth science community have raised is that, since these data sets that are already in use don't resemble our data that much, we might be able to create similar benchmark datasets that have some of the characteristics that are really important to us. If machine learning researchers develop algorithms for these specific data sets, we will begin to solve problems that are really important for earth science and create tools that can be used by a lot of climate researchers. One of the challenges that I've seen a lot in areas like hurricane tracking is that the standards for successful models within the machine learning community don't always line up exactly with what's being used by city planners or by other climate scientists.
For example, if you're trying to make quantitative, useful predictions, it's very important to have a well constrained uncertainty so that you don't know just, for example, how many hurricanes you expect to have in a certain location, but whether that prediction is really good and precise or whether you might get two or three times that many. Within the climate science community, I think a large fraction of the effort that goes into modeling actually goes into modeling variability and uncertainty and accuracy and things like that. And I think the machine learning community is still working on establishing those standards.
And I think that it's really easy for people to get excited about a model that looks like it's working, and then really that's only the beginning of the work — and you need to do a lot of digging in deeper to figure out the constraints on that model, and the bounds, and when it works and when it doesn't, and when you can best trust it and make use of it.
Ariel Conn: It seems like addressing that type of problem would require exactly the type of collaboration that we're seeing with this paper that you all worked on. One, is that the case? Two, are there other things that machine learning researchers and climate scientists can be doing to come together more to create better data sets?
Kelly Kochanski: I think a lot of people on both sides of this community have realized that this is a really fruitful area of research. Just in the last few years, there's been a lot of interest by the machine learning community in climate science; and likewise within the climate science community, the interest is just growing really rapidly right now. At the big climate science conferences, we've gone from having a small number of abstracts discussing machine learning to having more than 10 sessions specifically dedicated to machine learning coming up at the next big Geophysics Conference later this year.
So the interest is really booming and a lot of people want to find ways to leverage this. And I think that they're on track to converge and start forming these collaborations. So what we're trying to do, really, is not push something that people don't want to do, but to make something that people are already interested in happen a little bit faster and easier.
Ariel Conn: Were there other sections within this chapter that you think are important to mention that we didn't get into yet?
Kelly Kochanski: I focused a lot so far on talking about the ways that machine learning can work within scientific models and with scientific datasets, but there's also been a lot of work more directly with data. There's been a lot of work to track land use cover patterns, which also comes up in another section of our paper, or crop types or reforestation from images and satellites and other remote sensing data that we have of the planet. It's a more straightforward area. It looks more like traditional computing vision problems, but I believe that it's also very promising and is also making a lot of changes in the way that we look at and measure the world.
Ariel Conn: I think that's a really nice way to transition back to looking at the rest of the paper as a whole. We've gone through the sections that each of you worked on more individually, but obviously it's a big paper. It covers a lot of topics. One of the things that David mentioned earlier that I think is really important is some of the most effective things that were discussed throughout the paper are not necessarily the — in quotes, I say they're not necessarily the most “sexy” of topics, but they could be really impactful. I was hoping maybe you could touch on a couple examples of some of the most high impact, high leverage ways in which machine learning could be applied to the climate problem.
David Rolnick: So I'd like to second what Kelly said earlier about different applications of remote sensing. I think it's a really great area of application for machine learning, partly because there are so many application domains, but also because computer vision — which is one of the main machine learning technologies involved in remote sensing, i.e processing image data coming from satellites or from planes — computer vision is a very well developed area now in machine learning. And I think it has a lot to offer almost out of the box for many of these applications.
So of course new innovations in computer vision also have the potential to lead to new innovations in these solution domains. There are just so many applications of remote sensing that it's hard to pick even just a few. But as examples: pinpointing exactly where solar panels and wind turbines are, as Priya has talked about — that's really fundamental to understanding power generation and grids, especially in situations where we don't have a lot of centralized data.
But also many other applications: so tracking deforestation, for example. There are many places in the world where deforestation is illegal, but enforcement is very hard. Satellites can be used to generate imagery at fine resolution where we can pinpoint using computer vision exactly where deforestation is happening, and thereby give more tools to law enforcement in various places to enable deforestation to be counteracted. Likewise with simply monitoring the health of different ecosystems across the world: sometimes that can be done very effectively, at least as a first cut, using aerial imagery to work out like what species of trees are there or how healthy the forest is.
Another area that I think is often under-investigated is adaptation. This is a huge area encompassing all the various ways that we can help society to be resilient to the unavoidable consequences of climate change and the consequences that are happening already. There are many different ways that machine learning can help society be more resilient to the effects of climate change — starting out with designing and implementing better infrastructure, finding places where infrastructure like water mains might fail, and creating systems that are more robust to the kinds of effects which we see happening; creating flood maps for particular cities to indicate what aspects of the urban infrastructure we should be reinforcing; then also, in the moment when a crisis happens, being there on the ground to provide real-time tools for first responders and for people who are trying to make a difference. People have used natural language processing, for example, to analyze social media, to work out where help is needed in disasters. This sounds a little bit strange, but sometimes this is the way to comb through large amounts of data to find out where help is needed.
Priya Donti: And I think adaptation illustrates one of the key points of our paper, which is that machine learning doesn't exist in a vacuum. In a lot of the examples that David just discussed, in adaptation, machine learning can help give data driven insights about, as he's mentioned, infrastructure or about disaster relief. But fundamentally, somebody has to build the infrastructure. Some city government has to create a disaster relief plan based on data driven insights that are given to them. So really a lot of the solutions that we propose here are to be done in conjunction with stakeholders like city planners or researchers in other domains or policy makers — because really we will all need to come together to address the climate crisis.
Kelly Kochanski: One of the other areas where I've seen a lot of exciting possibilities with machine learning is also to do with making more specialized and targeted predictions for individual stakeholders to learn more about how the climate is going to affect their specific area and location. I think that as we develop those tools, that will help a lot with communicating to people who are making these decisions and showing the impacts to individuals who need to figure out how to organize their lives.
Ariel Conn: This is a nice transition to a question that I have for you — and that is, now that you've written this paper, what are the next steps?
David Rolnick: We see our role as facilitating the development of this intersection of fields. One of the key components of that is — as we all have touched on — facilitating collaboration, because collaboration is the most important part of this work, and in many ways the most challenging part. So we are working on various different collaboration tools that will help stakeholders to talk to one another and form teams to address the solutions which we have outlined in our paper.
Kelly Kochanski: I'd like to chime in on that on a more personal note, which is that working on this project has opened up a lot of collaboration opportunities for me and has really encouraged me to look more for high impact work that I can be doing, and work that's connected really directly to tools that people use and are going to need in the next few years, and to work on developments that will really affect a lot of people on the ground.
Lynn Kaack: I think also it helps to illustrate maybe where I come from, because I started working on the climate change policy field, but then increasingly started to integrate machine learning into the research. And I felt always kind of isolated in my work, and since we wrote this paper I met so many people working in the space, and it feels really great to have sort of an academic home, as somebody put it. So that's really something that we're trying to provide here. Not only for academics of course, but for everybody working on this space.
David Rolnick: We've really had people coming out of the woodwork in all kinds of sectors, everything from academics to people in finance who really want to make a difference here and are eager to see that they have the ability to use their skills, and the areas in which they work, for good within the space of climate change.
Ariel Conn: So it sounds like you've mostly gotten a pretty positive response to the paper so far. Is that how you see it?
David Rolnick: We've received a hugely positive response.
Ariel Conn: Excellent. This question sort of touches on some of what you're saying. To a certain extent, I'm guessing that machine learning hasn't been applied more to address climate change issues because it's been a fairly small field for quite a while, at least relatively speaking. So you haven't had as many people with the skills to do so, and I'm guessing it probably costs money to bring them on, or it requires a lot of time and money for someone to learn. Is that the case? Is that what you've seen? If so, how do you envision getting more machine learning researchers partnering with climate scientists?
Kelly Kochanski: I think the machine learning research community in general has taken a lot of actions that actually make this easier. There've been a lot of things that have come out of it: like habitual sharing of code from landmark papers in machine learning is really fantastic from the point of view of climate scientists, because it means that we can implement a lot of exciting things and try them out with a relatively low barrier to entry. I'll also say personally that what I've found is that as I've reached out to machine learning researchers about their work, and about techniques that I think it might be interesting to apply in climate and earth science contexts, a lot of people have been really thoughtful and helpful and interested in thinking about ways that they could, for example, adapt their work slightly to make it more applicable to climate problems.
David Rolnick: I'd also like to shout out to all the communities that have been thinking about aspects of this problem, in some cases for years. So, for example, climate informatics — which combines climate science with data, statistics, and machine learning — has been around for many years and has led to many deep insights in this conjunction in different fields. Likewise, computational sustainability has been a movement that for many years has encouraged insights across sustainability, broadly defined, and different aspects of machine learning and AI.
Ariel Conn: What are some of the technical challenges that you still see for machine learning in the climate area?
Priya Donti: One of the challenges that I definitely see in bridging machine learning and climate change is in getting people together in the same room who speak different languages or come from different cultures — both literally, because this is a multi-stakeholder, multi-country problem, but also in a technical sense. People who, for example, speak the language of electricity systems, versus speaking the language of machine learning. To give a personal example from machine learning and electricity systems, there is a little bit of a culture within machine learning where the inclination is to try new solutions, in some sense move fast, break things, and if it doesn't work, just try again.
When you intersect that with electricity systems — which is a field in which we need to keep the electricity system running, because the implication of not doing that is the power going out — how do we bring together this culture of move fast versus be careful? It's something that I think we will continue to grapple with as we get different kinds of stakeholders at the table.
Lynn Kaack: Another big challenge is that we need to get better at generalizing, especially since many of the solutions need to be able to be transferred between countries, which come with very different socioeconomic realities; also, different natural circumstances, and they might also change over time. So this is something that I see over and over again in different domains and different areas of mitigation and adaptation: that we need to be able to make models that work for different settings.
Kelly Kochanski: One thing that I've seen come up again and again as well is the need for machine learning solutions that work well with constraints. By constraints, I can mean anything from, in Priya's examples of energy grid management, guaranteeing that no matter where you put your machine learning system, you're not going to make the kind of mistake that makes the power go off; or in my area of climate research, we worry a lot about constraints like energy conservation, and not building machine learning models that violate the conservation of energy.
These are both cases where we have these constraints. We want the machine learning model to satisfy these constraints, and we also want to be able to use the knowledge about the system that these constraints represent to make the machine learning model easier or faster or otherwise simpler and better to work with. Having tools to do that in any kind of general way that makes it easy to translate from our intuitive understanding of what we want from the system's behavior to the machine learning model would be very helpful.
Ariel Conn: I want to turn back to the paper as a whole. I was hoping each of you could maybe mention one or two examples from the paper that we haven't gotten into yet that really surprised you, or were exciting, that you think are important to mention.
Priya Donti: The industry section written by Anna Waldman-Brown has a lot of examples that I personally find very exciting. Some of these are optimizing supply chains; that is, the way we move goods and services from creation to retail, and how we can make these processes more efficient by, for example, forecasting how much demand that we might have for a specific retail product. Other applications in this section include reducing food waste, for example by using sensors in a bucket of food to figure out which produce is going to go bad and as a result you can remove it in order to then prevent the rest of the produce from going bad.
I think the industry section highlights a lot of interesting considerations when working in this space. Specifically, you can optimize a supply chain all you want; or you can, for example, shift electricity demand and industrial applications all you want. But fundamentally, industrial partners incentives have to be aligned here to actually reduce greenhouse gas emissions. So policies either have to come into play or there has to be some alignment between reducing greenhouse gas emissions and saving money or servicing the bottom line.
Another interesting application for me here in the industry section that also recurs throughout the paper is this application of accelerated science: so creating, for example, alternatives to cement that require fewer emissions to produce. This application also comes up in the carbon dioxide removal section; so, how can we create more efficient sorbents — essentially carbon sponges — that allow us to remove carbon dioxide from the atmosphere more efficiently? Then I previously gave the example of solar fuels in electricity systems. So this application of accelerated science is one that is cross cutting throughout the paper that I found particularly exciting.
Lynn Kaack: That's also something that surprised me quite a bit. Another thing was precision agriculture. They use a lot of data and robotic applications to really precisely treat a field according to the needs — so in terms of water or fertilizer. This both helps mitigation and adaptation, and it's actually already being deployed. It was something that I thought was very far out in the future — but through writing this paper, I've actually learned that this is a real application that might have a future.
David Rolnick: Another area that I'd like to call out is finance; the finance section of our paper was written by Sasha Luccioni. There are two aspects in which finance relates potentially to climate change and both of them have huge potential impacts in shaping forces within the system to help the overall climate crisis. So the first one is climate investment, involving finance identifying which companies, which investments are contributing positively or negatively to the climate crisis, and allowing investors the ability to choose based upon those factors.
That really has the potential to give boosts to sections of the economy that are working against the climate crisis; and also, to increase liquidity in potentially very small businesses that are having an out-sized impact in a positive way. Then the other aspect of climate finance that I want to stress is climate analytics, which looks at the potential for companies or entire industries as it relates to the climate impacts on those industries. So here, one looks at what the inevitable consequences of climate change are going to be, and then looks at how that will affect certain industries.
Accounting for that in the global financial system is really vital to making us as a society take these kinds of impacts seriously. Because sometimes when you don't put a price on it, it's very hard for society to move to take action.
Ariel Conn: All right. We've looked at a lot of specifics and some of the details of the paper. If we were to take a step back and sort of clarify a few key points that you really want people to walk away with, what would those be?
David Rolnick: Machine learning is a really powerful tool, and it can be applied to a very broad range of problems with climate impact, but it's also not a silver bullet. It's not magically going to solve the climate crisis. Also, in every way of applying machine learning to climate change, it's really essential to involve both multiple fields and multiple different stakeholders, experts in various aspects of the problem, and also end users, who will be the ones interacting with whatever is deployed.
Priya Donti: From a machine learning perspective, there are many kinds of innovations that we need in this area in order to combat the climate crisis. So there are things that researchers can do to fundamentally advance machine learning while contributing to climate change. There are interesting engineering opportunities, business opportunities, opportunities for established businesses to apply machine learning to their practices to reduce greenhouse gas emissions. And of course, there are a lot of public sector applications here. So really the kinds of people who should be interested in whether machine learning maybe can help them address their piece of the climate crisis is a very broad group of people.
Lynn Kaack: Another point is that there are really many ways to have an impact. This goes both for mitigation, where there are just so many different sources of greenhouse gas emissions and many solutions to reduce those, but also for adapting to the risk of climate change. What we would like to take away from this paper is one should have an open mind going into this field and be ready to work on problems you haven't thought of before. Even as David already put it before, those might be not as attractive at the first sight, but if you're coming with an open mind, they might open up into very interesting problems.
David Rolnick: Listen to what the domain experts tell you. Listen to what the people who have thought about this for their whole careers tell you. Talk to people who have thought about these problems their entire lives. Whatever area of solution you're thinking about, there is somebody who has spent a long time working out exactly what is needed there. If you can give it to them, that has huge potential for impact.
Kelly Kochanski: I'd like to follow up both of the previous points from Lynn and David by saying that one of the things that's been most surprising and wonderful about this project is that's given me a lot of hope for climate change. Being embedded in climate science — sometimes that can be very difficult, because we spend a lot of time measuring how things could go wrong. But working on this project has really put a lot of emphasis on solutions, on things that we can do, on the wide range of possible avenues that there are, and on the fact that all of these will have an impact. Even the small impacts will still make a difference, and we can be moving towards something that's really positive and optimistic in this area.
And finally, that there are a lot of people who are thinking about this, and who really want to make a difference, and are working hard on finding new and better ways to do it that'll make it easier for us to have a climate future that works.
Ariel Conn: That really was great. I want to open this up now to everyone else, too. How hopeful are you now that we can address climate change before it gets too bad, and did this paper impact you as it did Kelly?
Lynn Kaack: So on the one hand, I'm actually quite hopeful because I know that there are a lot of technologies that exist today that, if we would implement them, they would actually make the economy emit way less greenhouse gas emissions than we currently do. And there are also many people working on future technologies, and I really believe that we will have some solutions in the future that will enable us to go down to net zero carbon emissions.
But on the other hand, I'm extremely worried about that the society will not build up enough pressure to implement them. So our economic and political systems have, to this point, not acted fast enough, and I would really like to stress that it's important that people make their voices heard if they want to transition to a low carbon society. I think part of that is also legislation that can help to tackle climate change.
David Rolnick: I'm terrified. I'm terrified because technological solutions are only part of the ultimate solution. Through machine learning, through other technologies, you can make decisions easier for society to make — but ultimately society does have to make those decisions. But I'm not just terrified. Climate change is often thought of as an on-off switch — either we're doomed or we're saved — and it's really not about that. There are so many ways in which we can avert some of the most serious consequences and pick how far along the path we want to travel. Focusing on that, rather than on solving climate change, for all that we've talked about solutions, is really maybe the best way forward.
So focusing on reducing greenhouse gas emissions, focusing on helping society do what it can to be resilient to what is inevitable, will really help us reach a place which is as good as possible. And that's all that we can do.
Ariel Conn: What do you hope to see from people, both in response to this paper but also just more generally? We've been looking specifically at what climate scientists and machine learning researchers can do to try to address this problem. But as you've been looking at this, are there some big or little things that you'd like to see happen that any individual could start doing more of?
David Rolnick: We're trying to build tools to enable action from individuals and from organizations. On our website, climatechange.ai, you can read about some of the things that we're doing, and even sign up for a mailing list where you can receive updates about the kinds of tools that we're trying to bring out there to enable work. But I would second what people have already said from this group — that if you're trying to be involved, learn about these issues, find people to talk to who are potential collaborators, listen to them, and then make sure that whatever you're doing really gets out there in the world is deployed with impact.
Kelly Kochanski: The other thing I'd like to add with that is that it really helps to take action now, and a lot of what I think is important about this work is the speed. The faster that we can create solutions that will lower greenhouse gas emissions by any fraction, the less total warming we're going to see on earth, because the less total warming we’ll have put into the atmosphere. And so if the work that we're doing takes people who have the energy and the drive to do something and gets them moving even a month earlier than they might have been going otherwise, then I think that'll be a big success.
Priya Donti: One thing I'd like to add and to stress is that while our paper looks at how one particular kind of expertise — that is, expertise in machine learning — can be used in conjunction with climate change domain expertise to address the climate crisis, I think there are many other types of expertise that are needed. And so other fields would also, I think, benefit from examining how specifically they can contribute to the climate crisis, and to mobilize movements in their fields to do that.
Ariel Conn: All right, so I think this is really good. David, Priya, Lynn and Kelly, thank you so much for joining and talking about your paper today. It was a really great paper and it was really great talking with you.
David Rolnick: Absolutely. Thank you so much.
Priya Donti: Yeah, thanks so much for having us.
Lynn Kaack: Yeah, thank you.
Kelly Kochanski: Thank you for having us Ariel.
Ariel Conn: I really hope you enjoyed this episode, especially since we’re bringing you more machine learning and climate change in the next episode! In episode 17 of Not Cool: a climate podcast, we’ll hear more about how individuals and communities can use machine learning to improve carbon footprints and climate policies.
Tegan Maharaj: I would say in the short-term, it's often the case that climate-friendly solutions are harder to implement, because they're a change to the status quo, so that makes them a bit more expensive — but in the long-term, virtually all of the climate and environmentally friendly solutions just make economic sense.
Ariel Conn: Be sure to join us again on Thursday, and as always, if you’ve enjoyed this podcast, please take a moment to like it, share it, and maybe even leave a good review.
Related episodes
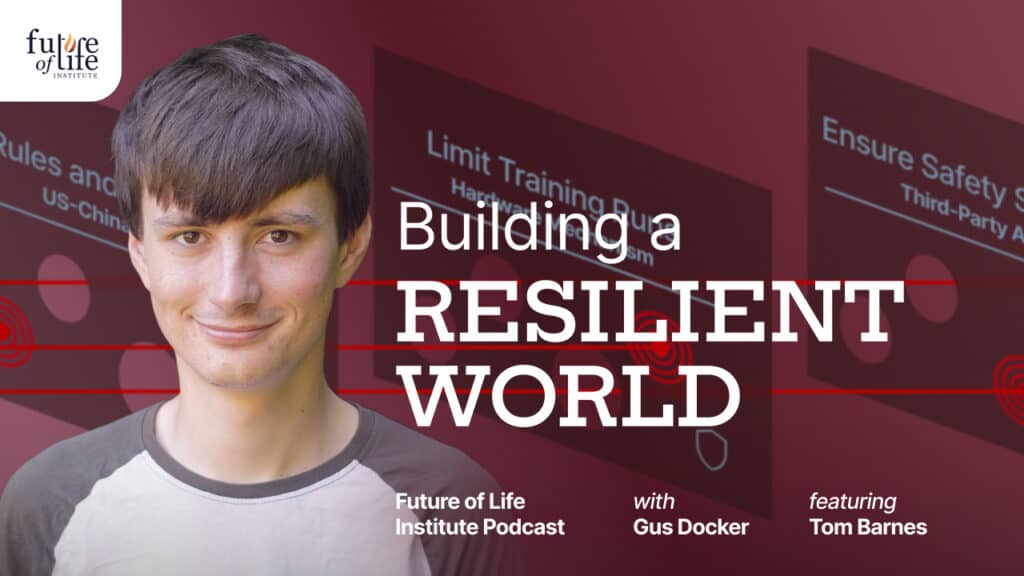