AI Alignment Podcast: Inverse Reinforcement Learning and the State of AI Alignment with Rohin Shah
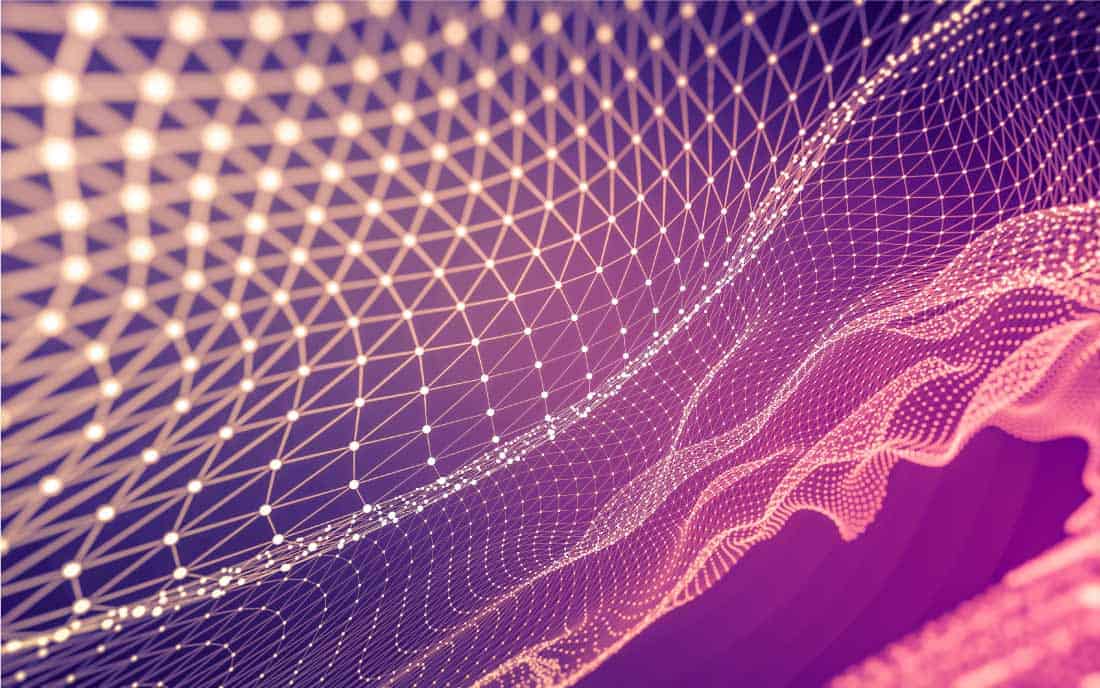
What role does inverse reinforcement learning (IRL) have to play in AI alignment? What issues complicate IRL and how does this affect the usefulness of this preference learning methodology? What sort of paradigm of AI alignment ought we to take up given such concerns?
Inverse Reinforcement Learning and the State of AI Alignment with Rohin Shah is the seventh podcast in the AI Alignment Podcast series, hosted by Lucas Perry. For those of you that are new, this series is covering and exploring the AI alignment problem across a large variety of domains, reflecting the fundamentally interdisciplinary nature of AI alignment. Broadly, we will be having discussions with technical and non-technical researchers across areas such as machine learning, governance, ethics, philosophy, and psychology as they pertain to the project of creating beneficial AI. If this sounds interesting to you, we hope that you will join in the conversations by following us or subscribing to our podcasts on Youtube, SoundCloud, or your preferred podcast site/application.
If you're interested in exploring the interdisciplinary nature of AI alignment, we suggest you take a look here at a preliminary landscape which begins to map this space.
In this podcast, Lucas spoke with Rohin Shah. Rohin is a 5th year PhD student at UC Berkeley with the Center for Human-Compatible AI, working with Anca Dragan, Pieter Abbeel and Stuart Russell. Every week, he collects and summarizes recent progress relevant to AI alignment in the Alignment Newsletter.
Topics discussed in this episode include:
Transcript
Lucas: Hey everyone, welcome back to the AI Alignment Podcast series. I'm Lucas Perry and today we will be speaking with Rohin Shah about his work on inverse reinforcement learning and his general take on the state of AI alignment efforts and theory today. Rohin is a 5th year PhD student at UC Berkeley with the Center for Human-Compatible AI, working with Anca Dragan, Pieter Abbeel and Stuart Russell. Every week, he collects and summarizes recent progress relevant to AI alignment in the Alignment Newsletter. He has also been working with effective altruism for several years. Without further ado I give you Rohin Shah.
Hey, Rohin, thank you so much for coming on the podcast. It's really a pleasure to be speaking with you.
Rohin: Hey, Lucas. Yeah. Thanks for inviting me. I'm glad to be on.
Lucas: Today I think that it would be interesting just to start off by delving into a lot of the current work that you've been looking into and practicing over the past few years. In terms of your research, it looks like you've been doing a lot of work on practical algorithms for inverse reinforcement learning that take into account, as you say, systematic cognitive biases that people have. It would be interesting if you could just sort of unpack this work that you've been doing on this and then contextualize it a bit within the AI alignment problem.
Rohin: Sure. So basically the idea with inverse reinforcement learning is you can look at the behavior of some agent, perhaps a human, and tell what they're trying to optimize, what are the things that they care about? What are their goals? And in theory this seems like a pretty nice way to do AI alignment and that intuitively you can just say, "Hey, AI, go look at the actions of humans are taking, look at what they say, look at what they do, take all of that in and figure out what humans care about." And then you could use that perhaps as a utility function for your AI system.
I think I have become less optimistic about this approach now for reasons I'll get into, partly because of my research on systematic biases. Basically one problem that you have to deal with is the fact that whatever humans are trying to optimize for, they're not going to do it perfectly. We've got all of these sorts of cognitive biases like a planning fallacy or hyperbolic time discounters, when we tend to be myopic, not looking as far into the long-term as we perhaps could.
So assuming that humans are like perfectly optimizing goals that they care about is like clearly not going to work. And in fact, basically, if you make that assumption, well, then whatever reward function you infer, once the AI system is optimizing that, it's going to simply recover the human performance because well, you assumed that it was optimal when you inferred what it was so that means whatever the humans were doing is probably the behavior that optimizes their work function that you inferred.
And we'd really like to be able to reach super human performance. We'd like our AI systems to tell us how we're wrong to get new technologies develop things that we couldn't have done ourselves. And that's not really something we can do using the sort of naive version of inverse reinforcement learning that just assumes that you're optimal. So one thing you could try to do is to learn the ways in which humans are biased, the ways in which they make mistakes, the ways in which they plan sub-optimally. And if you could learn that, then you could correct for those mistakes, take them into account when you're inferring human values.
The example I like to use is if there's a grad student who procrastinates or doesn't plan well and as a result near a paper deadline they're frantically working, but they don't get it in time and they miss the paper deadline. If you assume that they're optimal, optimizing for their goals very well I don't know what you'd infer, maybe something like grad students like to miss deadlines. Something like that seems pretty odd and it doesn't seem like you'd get something sensible out of that, but if you realize that humans are not very good at planning, they have the planning fallacy and they tend to procrastinate for reasons that they wouldn't endorse on reflection, then maybe you'd be able to say, "Oh, this was just a mistake of a grad student made. In the future I should try to help them meet their deadlines."
So that's the reason that you want to learn systematic biases. My research was basically let's just take the hammer of deep learning and apply it to this problem. So not just learn the reward function, but let's also learn the biases. It turns out that this was already known, but there is an impossibility result that says that you can't do this in general. So more, I guess I would phrase the question I was investigating, as what are a weaker set of assumptions some of than the ones that we currently use such that you can still do some reasonable form of IRL.
Lucas: Sorry. Just stepping back for like half a second. What does this impossibility theorem say?
Rohin: The impossibility theorem says that if you assume that the human is basically running some sort of planner that takes in a reward function and spits out a behavior or a policy, a thing to do over time, then if you all you see is the behavior of the human, basically any reward function is compatible with some planner. So you can't learn anything about that reward function without making any more assumptions. And intuitively, this is because for any complex behavior you see you could either call it, "Hey, the human's optimizing a reward that makes them act like that. "Or you could say, "I guess the human is biased and they're trying to do something else, but they did this instead."
The sort of extreme version of this is like if you give me an option between apples and oranges and I picked the apple, you could say, "Hey, Rohin probably likes apples and is good at maximizing his reward of getting apples." Or you could say, "Rohin probably likes oranges and he is just extremely bad at satisfying his preferences. He's got a systematic bias that always causes him to choose the opposite of what he wants." And you can't distinguish between these two cases just by looking at my behavior.
Lucas: Yeah, that makes sense. So we can pivot sort of back in here into this main line of thought that you were on.
Rohin: Yeah. So basically with that impossibility result ... When I look at the impossibility result, I sort of say that humans do this all the time, humans just sort of look at other humans and they can figure out what they want to do. So it seems like there are probably some simple set of assumptions that humans are using to infer what other humans are doing. So a simple one would be when the consequences of something or obvious to humans. Now, how you determine when that is another question, but when that's true humans tend to be close to optimal and if you have something like that, you can rule out the planner that says the human is anti-rational and always chooses the worst possible thing.
Similarly, you might say that as tasks get more and more complex or require more and more computation, the probability that the human chooses the action that best maximizes his or her goals also goes down since the task is more complex and maybe a human doesn't figure that out, figure out what's the best thing to do. Maybe with enough of these assumptions we could get some sort of algorithm that actually works.
So we looked at if you make the assumption that the human is often close to rational and a few other assumptions about humans behaving similarly or planning similarly on similar tasks, then you can maybe, kind of, sort of, in simplified settings do IRL better than if you had just assumed that the human was optimal if humans actually systematically biased, but I wouldn't say that our results are great. I don't think I would say that I definitively, conclusively said, "This will never work." Nor did I definitively conclusively say that this is great and we should definitely be putting more resources into it. Sort of somewhere in the middle, maybe more on the negative side of like this seems like a really hard problem and I'm not sure how we get around it.
Lucas: So I guess just as a point of comparison here, how is it that human beings succeed at this every day in terms of inferring preferences?
Rohin: I think humans have the benefit of being able to model the other person as being very similar to themselves. If I am trying to infer what you are doing I can sort of say, "Well, if I were in Lucas issues and I were doing this, what would I be optimizing?" And that's a pretty good answer to what you would be optimizing. Humans are just in some absolute sense very similar to each other. We have similar biases. We've got similar ways of thinking. And I think we've leveraged that similarity a lot using our own self models as a drop-in approximation of the other person's planner in this planner reward language.
And then we say, "Okay, well, if this other person thought like me and this is what they ended up doing, well then, what must they have been optimizing?" I think you'll see that when this assumption breaks down humans actually get worse at inferring goals. It's harder for me to infer what someone in a different culture is actually trying to do. They might have values that are like significantly different from mine.
I've been in both India and the US and it often seems to me that people in the US just have a hard time grasping the way that Indians see society and family expectations and things like this. So that's an example that I've observed. It's probably also true the other way around, but I was never old enough in India to actually think through this.
Lucas: Human beings sort of succeed in inferring preferences of people who they can model as having like similar values as their own or if you know that the person has similar values as your own. If inferring human preferences from inverse reinforcement learning is sort of not having the most promising results, then what do you believe to be a stronger way of inferring human preferences?
Rohin: The one thing I correct there is that I don't think humans do it by assuming that people have similar values, just that people think in similar ways. For example, I am not particularly good at dancing. If I see someone doing a lot of hip-hop or something. It's not that I value hip-hop and so I can infer they value hip-hop. It's that I know that I do things that I like and they are doing hip-hop. Therefore, they probably like doing hip-hop. But anyway, that's the minor point.
So a, just because IRL algorithms aren't doing well now, I don't think it's true that IRL algorithms couldn't do well in the future. It's reasonable to expect that they would match human performance. That said, I'm not super optimistic about IRL anyway, because even if we do figure out how to get IRL algorithms and sort of make all these implicit assumptions that humans are making that we can then run and get what a human would have thought other humans are optimizing, I'm not really happy about then going and optimizing that utility function off into the far future, which is what sort of the default assumption that we seem to have when using inverse reinforcement learning.
It may be that IRL algorithms are good for other things, but for that particular application, it seems like the utility function you infer is going to not really scale to things that super intelligence will let us do. Humans just think very differently about how they want the future to go. In some sense, the future is going to be very, very different. We're going to need to think a lot about how we want the future to go. All of our experience so far has not trained us to be able to think about what we care about in the sort of feature setting where we've got as a simple example the ability to easily copy people if they're uploaded as software.
If that's a thing that happens, well, is it okay to clone yourself? How does democracy work? All these sorts of things are somewhat value judgments. If you take egalitarianism and run with it, you basically get that one person can copy themselves millions of millions of times and just determine the outcome of all voting that way. That seems bad, but on our current values, I think that is probably what we want and we just really haven't thought this through. IRL to infer utility function that we've then just ruthlessly optimized in the long-term just seems like by the time when the world changes a bunch, the value function that we inferred is going to be weirdly wrong in strange ways that we can't predict.
Lucas: Why not run continuous updates on it as people update given the change of the world?
Rohin: It seems broadly reasonable. This is the sort of idea that you could have about how you could use IRL in a more realistic way that actually works. I think that's perfectly fine. I'm optimistic about approaches that are like, "Okay, we're going to use IRL to infer a value function or reward function or something and we're going to use that to inform what the AI does, but it's not going to be the end-all utility functions. It's just going to infer what we do now and AI system is somehow going to check with us. Maybe it's got some uncertainty over what the true reward function is. Maybe that it only keeps this reward function for a certain amount of time."
These seem like things that are worth exploring, but I don't know that we have the correct way to do it. So in the particular case that you proposed, just updating the reward function over time. The classic wire heading question is, how do we make it so that the AI doesn't say, "Okay, actually, in order to optimize the utility function I have now, it would be good for me to prevent you from changing my utility function since if you change my utility function, I'm no longer going to achieve my original utility." So that's one issue.
The other issue is maybe it starts doing some long-term plans. Maybe even if it's planning according to this utility function without expecting some changes to the utility function in the future, then it might set up some long-term plans that are going to look bad in the future, but it is hard to stop them in the future. Like you make some irreversible change to society because you didn't realize that something was going to change. These sorts of things suggest you don't want a single utility function that you're optimizing even if you're updating that utility function over time.
It could be that you have some sort of uncertainty over utility functions and that might be okay. I'm not sure. I don't think that it's settled that we don't want to do something like this. I think it's settled that we don't want to use IRL to infer a utility function and optimize that one forever. There are certain middle grounds. I don't know how well those middle grounds work. There are some intuitively there are going to be some problems, but maybe we can get around those.
Lucas: Let me try to do a quick summary just to see if I can explain this as simply as possible. There are people and people have preferences, and a good way to try and infer their preferences is through their observed behavior, except that human beings have cognitive and psychological biases, which sort of skew their actions because they're not perfectly rational epistemic agents or rational agents. So the value system or award system that they're optimizing for is imperfectly expressed through their behavior. If you're going to infer the preferences from behavior than you have to correct for biases and epistemic and rational failures to try and inferr the true reward function. Stopping there. Is that sort of like a succinct way you'd put it?
Rohin: Yeah, I think maybe another point that might be the same or might be different is that under our normal definition of what our preferences or our values are, if we would say something like, "I value egalitarianism, but it seems predictably true that in the future we're not going to have a single vote per a sentient being," or something. Then essentially what that says is that our preferences, our values are going to change over time and they depend on the environment in which we are right now.
So you can either see that as okay, I have this really big, really global, really long-term utility function that tells me how given my environment what my narrow values in that environment are. And in that case and you say, "Well okay, in that case, we're really super biased because we only really know our values in the environment. We don't know our values in future environments. We'd have to think a lot more for that." Or you can say, "We can infer our narrow values now and that has some biases thrown in, but we could probably account for those that then we have to have some sort of story for how we deal with our preferences evolving in the future."
Those are two different perspectives on the same problem, I would say, and they differ in basically what you're defining values to be. Is it the thing that tells you how to extrapolate what you want all the way into the future or is it the thing that tells you how you're behaving right now in the environment. I think our classical notion of preference or values, the one that we use when we say values in everyday language is talking about the second kind, the more narrow kind.
Lucas: There's really a lot there, I think, especially in terms of issues in that personal identity over time, commitment to values and as you said, different ideas and conceptualization of value, like what is it that I'm actually optimizing for or care about. Population ethics and tons of things about how people value future versions of themselves or whether or not they actually equally care about their value function at all times as it changes within the environment.
Rohin: That's a great description of why I am nervous around inverse reinforcement learning. You listed a ton of issues and I'm like, yeah, all of those are like really difficult issues. And with inverse reinforcement learning, it's sort of based on this premise of all of that is existent, is real and is timeless and we can infer it and then maybe we put on some hacks like continuously improving the value function over time to take into account changes, but this does feel like we're starting with some fundamentally flawed paradigm.
So mostly because of this fact that it feels like we've taken a flawed paradigm to start with, then changed it so that it doesn't have all the obvious flaws. I'm more optimistic about trying to have a different paradigm of how we want to build AI, which maybe I'll summarize as just make AIs that do what we want or what we mean at the current moment in time and then make sure that they evolve along with us as we evolve and how we think about the world.
Lucas: Yeah. That specific feature there is something that we were trying to address in inverse reinforcement learning, if the algorithm were sort of updating overtime alongside myself. I just want to step back for a moment to try to get an even grander and more conceptual understanding of the globalness of inverse reinforcement learning. So from an evolutionary and sort of more cosmological perspective, you can say that from the time that the first self-replicating organisms on the planet until today, like the entire evolutionary tree, there's sort of a global utility function across all animals that is ultimately driven by thermodynamics and the sun shining light on a planet and that this sort of global utility function of all agents across the planet, it seems like very ontologically basic and pure like what simply empirically exists. Attempting to access that through IRL is just interesting, the difficulties that arise from that. Does that sort of a picture seem accurate?
Rohin: I think I'm not super sure what exactly you're proposing here. So let me try and restate it. So if we look at the environment as a whole or the universe as a whole or maybe we're looking at evolution perhaps and we see that hey, evolution seems to have spit out all of these creatures that are interacting in this complicated way, but you can look at all of their behavior and trace it back to this objective in some sense of maximizing reproductive fitness. And so are we expecting that IRL on this very grand scale would somehow end up with maximize reproductive fitness. Is that what ... Yeah, I think I'm not totally sure what implication you're drawing from this.
Lucas: Yeah. I guess I'm not arguing that there's going to be some sort of evolutionary thing which is being optimized.
Rohin: IRL does make the assumption that there is something doing an optimization. You usually have to point it towards what that thing is. You have to say, "Look at the behavior of this particular piece of the environment and tell me what it's optimizing." Maybe if you're imagining IRL on this very grand scale, what is the thing you're pointing it at?
Lucas: Yeah, so to sort of reiterate and specify, the pointing IRL at the human species would be like to point IRL at 7 billion primates. Similarly, I was thinking that what if one pointed IRL at the ecosystem of Earth over time, you could sort of plot this evolving algorithm over time. So I was just sort of bringing to note that accessing this sort of thing, which seems quite ontologically objective and just sort of clear in this way, it's just very interesting how it's fraught with so many difficulties. Yeah, in terms of history it seems like all there really is, is the set of all preferences at each time step over time, which could be summarized in some sort of global or individual levels of algorithms.
Rohin: Got it. Okay. I think I see what you're saying right now. It seems like the intuition is like ecosystems, universe, laws of physics, very simple, very ontologically basic things, there's something more real about any value function we could infer from that. And I think this is a misunderstanding of what IRL does. IRL fundamentally requires you to have some notion of counterfactuals. You need to have a description of the action space that some agent had and then when you observe their behavior, you see that they made a choice to take one particular action instead of another particular action.
You need to be able to ask the question of what could they have done instead, which is a counterfactual. Now, with laws of physics, it's very unclear what the counterfactual would be. With evolution, you can maybe say something like, "Evolution could have chosen to make a whole bunch of mutations and I chose this particular one. And then if you use that particular model, what is IRL going to infer? It will probably infer something like maximized reproductive fitness."
On the other hand, if you model evolution as like hey, you can design the best possible organism that you can. You can just create an organism out of thin air. And then what reward function are you maximizing then, it's like super unclear. If you could just poof into existence a organism, you could just make something that's extremely intelligent, very strong, et cetera, et cetera. And you're like, well, evolution didn't do that. It took millions of years to create even humans so clearly it wasn't optimizing reproductive fitness, right?
And in fact, I think people often say that evolution is not an optimization process because of things like this. The notion of something doing optimization is very much relative to what you assume their capabilities to be and in particular what do you assume their counterfactuals to be. So if you were talking about this sort of grand scale ecosystems, universe, laws of physics, I would ask you like, "What are the counterfactuals? What could the laws of physics done otherwise or what could the ecosystem have done if it didn't do the thing that it did?" Once you have an answer to that, I imagine I could predict what IRL would do. And that part is the part that doesn't seem ontologically basic to me, which is why I don't think that IRL on this sort of thing makes very much sense.
Lucas: Okay. The part here that seems to be a little bit funny to me is where tracking from physics, whatever you take to be ontologically basic about the universe, and tracking from that to the level of whatever our axioms and pre-assumptions for IRL are. What I'm trying to say is in terms of moving from whatever is ontologically basic to the level of agents and we have some assumptions in our IRL where we're thinking about agents as sort of having theories of counterfactuals where they can choose between actions and they have some sort of reward or objective function that they're trying to optimize for over time.
It seems sort of metaphysically queer where physics stops ... Where we're going up in levels of abstraction from physics to agents and we ... Like physics couldn't have done otherwise, but somehow agents could have done otherwise. Do you see the sort of concern that I'm raising?
Rohin: Yeah, that's right. And this is perhaps another reason that I'm more optimistic about the don't try to do anything at the grand scale and just try to do something that does the right thing locally in our current time, but I think that's true. It definitely feels to me like optimization, the concept, should be ontologically basic and not a property of human thought. There's something about how a random universe is high entropy whereas the ones that humans construct is low entropy. That suggests that we're good at optimization.
It seems like it should be independent of humans. Also, on the other hand, optimization, any conception I come up with it is either specific to the way humans think about it or it seems like it relies on this notion of counterfactuals. And yeah, the laws of physics don't seem like they have counterfactuals, so I'm not really sure where that comes in. In some sense, you can see that, okay, why do we have the notion of counterfactuals on agency thinking that we could have chosen something else while we're basically ... In some sense we're just an algorithm that's continually thinking about what we could do, trying to make plans.
So we search over this space of things that could be done, and that search is implemented in physics, which has no say, it has no counterfactuals, but the search itself, which is an abstraction layer above, it's something that is running on physics. It is not itself a physics thing, that search is in fact going through multiple options and then choosing one now. It is deterministic from the point of view of physics, but from the point of view of the search, it's not deterministic. The search doesn't know which one is going to happen. I think that's why humans have this notion of choice and of agency.
Lucas: Yeah, and I mean, just in terms of understanding the universe, it's pretty interesting just how there's like these two levels of attention where at the physics level you actually couldn't have done otherwise, but as sort of like this optimization process running on physics that's searching over space and time and modeling different world scenarios and then seemingly choosing and thus, creating observed behavior for other agents to try and infer whatever reward function that thing is trying to optimize for, it's an interesting picture.
Rohin: I agree. It's definitely a sort of puzzles that keep you up at night. But I think one particularly important implication of this is that agency is about how a search process thinks about itself. It's not just about that because I can look at what someone else is doing and attribute agency to them, figure out that they are themselves running an algorithm that chooses between actions. I don’t have a great story for this. Maybe it's just humans realizing that other humans are just like them.
So this is maybe why we get acrimonious debates about whether evolution has agency, but we don't get acrimonious debates about whether humans have agency. Evolution is sufficiently different from us that we can look at the way that it “chooses” "things" and we say, "Oh well, but we understand how it chooses things." You could model it as a search process, but you could also model it is all that's happening is this deterministic or mostly deterministic which animals survived and had babies and that is how things happen. And so therefore, it's not an optimization process. There's no search. There is deterministic. And so you have these two conflicting views for evolution.
Whereas I can't really say, "Hey Lucas, I know exactly deterministically how you're going to do things." I know this at the sense of like men, there are electrons and atoms and stuff moving around in your brain and electrical signals, but that's not going to let me predict what you can do. One of the best models I can have of you is just optimizing for some goal, whereas with evolution I can have a more detailed model. And so maybe that's why I set aside the model of evolution as an optimizer.
Under this setting it's like, "Okay, maybe our views of agency and optimization are just facts about how well we can model the process, which cuts against the optimization as ontologically basic thing and it seems very difficult. It seems like a hard problem to me. I want to reiterate that most of this has just pushed me to let's try and instead have a AI alignment focus, try to do things that we understand now and not get into the metaphilosophy problems. If we just get AI systems that broadly do what we want and are asking us for clarification, helping us evolve our thoughts over time, if we can do something like that. I think there are people who would argue that like no, of course, we can't do something like that.
But if we could do something like that, that seems significantly more likely to work than something that has to have answers to all these metaphilosophical problems today. My position is just that this is doable. We should be able to make systems that are of the nature that I described.
Lucas: There's clearly a lot of philosophical difficulties that go into IRL. Now it would be sort of good if we could just sort of take a step back and you could summarize your thoughts here on inverse reinforcement learning and the place that it has in AI alignment.
Rohin: I think my current position is something like fairly confidently don't use IRL to infer a utility function that you then optimize over the long-term. In general, I would say don't have a utility function that you optimize over the long-term because it doesn't seem like that's easily definable right now. So that's like one class of things I think we should do. On the other hand I think IRL is probably good as a tool.
There is this nice property of IRL that you figure out what someone wants and then you help them do it. And this seems more robust than handwriting, the things that we care about in any particular domain, like even in a simple household robot setting, there are tons and tons of preferences that we have like don't break vases. Something like IRL could infer these sorts of things.
So I think IRL has definitely a place as a tool that helps us figure out what humans want, but I don't think the full story for alignment is going to rest on IRL in particular. It gets us good behavior in the present, but it doesn't tell us how to extrapolate on into the future. Maybe if you did IRL that let you infer how we want the AI system to extrapolate our values or to figure out IRL and our meta-preferences about how the algorithm should infer our preferences or something like this, that maybe could work, but it's not obvious to me. It seems worth trying at some point.
TLDR, don't use it for long-term utility function. Do use it as a tool to get decent behavior in the short-term. Maybe also use it as a tool to infer meta-preferences. That seems broadly good, but I don't know that we know enough about that setting yet.
Lucas: All right. Yeah, that's all just super interesting and it's sort of just great to hear how the space is unfolded for you and what your views are now. So I think that we can just sort of pivot here into the AI alignment problem more generally and so now that you've moved on from being as excited about IRL, what is essentially capturing your interests currently in the space of AI alignment?
Rohin: The thing that I'm most interested in right now is can we build an AI system that basically evolves over time with us. I'm thinking of this now is like a human AI interaction problem. You've got an AI system. We want to figure out how to make it that it broadly helps us, but also at the same time and figures out what it needs to do based on some sort of data that comes from humans. Now, this doesn't have to be the human saying something. It could be from their behavior. It could be things that they have created in the past. It could be all sorts of things. It could be a reward function that they write down.
But I think the perspective of the things that are easy to infer are the things that are specific to our current environment is pretty important. What I would like to do is build AI systems that refer to preferences in the current environment or things we want in the current environment and do those reasonably well, but don't just extrapolate to the future and let humans adapt to the future and then figure out what the humans value now and then do things based on that then.
There are a few ways that you could imagine this going. One is this notion of corrigibility in the sense that Paul Christiano writes about it, not the sense that MIRI writes about it, where the AI is basically trying to help you. And if I have an AI that is trying to help me, well, I think one of the most obvious things for someone who's trying to help me to do is make sure that I remain in effective control of any power resources that might be present that the AI might have and to ask me if my values change in the future or if what I want the AI to do changes in the future. So that's one thing that you might hope to do.
Also imagine building a norm following AI. So I think human society basically just runs on norms that we mostly all share and tend to follow. We have norms against particularly bad things like murdering people and stealing. We have norms against shoplifting. We have maybe less strong norms against littering. Unclear. And then we also have norms for things are not very consequential. We have norms against randomly knocking over a glass at a restaurant in order to break it. That is also a norm. Even though there are quite often times where I'm like, "Man, it would be fun to just break a glass at the restaurant. It's very cathartic," but it doesn't happen very often.
And so if we could build an AI system that could infer and follow those norms, it seems like this AI would behave in a more human-like fashion. This is a pretty new line of thought so I don't know whether this works, but it could be that such an AI system is simultaneously behaving in a fashion that humans would find acceptable and also lets us do pretty cool, interesting, new things like developing new technologies and stuff that humans can then deploy and the AI doesn't just unilaterally deploy without any safety checks or running it by humans or something like that.
Lucas: So let's just back up a little bit here in terms of the picture of AI alignment. So we have a system that we do not want to extrapolate too much toward possible future values. It seems that there are all these ways in which we can be using the AI first to sort of amplify our own decision making and then also different methodologies which reflect the way that human beings update their own values and preferences over time, something like as proposed by I believe Paul Christiano and Geoffrey Irving and other people at OpenAI, like alignment through debate.
And there's just all these sorts of epistemic practices of human beings with regards to sort of this world model building and how that affects shifts in value and preferences, also given how the environment changes. So yeah, it just seems like tracking overall these things, finding ways in which AI can amplify or participate in those sort of epistemic practices, right?
Rohin: Yeah. So I definitely think that something like amplification can be thought of as improving our epistemics over time. That seems like a reasonable way to do it. I haven't really thought very much about how amplification or the pay scales were changing environments. They both operate under this general like we could have a deliberation tree and in principle what we want is this exponentially sized deliberation tree where the human goes through all of the arguments and counter-arguments and breaks those down into sub-points in excruciating detail in a way that no human could ever actually do because it would take way too long.
And then amplification debate basically show you how to get the outcome that this reasoning process would have given by using an AI system to assist the human. I don't know if I would call it like improving human epistemics, but more like taking whatever epistemics you already have and running it for a long amount of time. And it's possible like in that long amount of time you actually figure out how to do better epistemics.
I'm not sure that this perspective really talks very much about how preferences change over time. You would hope that it would just naturally be robust to that in that as the environment changes, your deliberation starts looking different in that like okay, now suddenly we have to go back to my example before we have uploads and we're like egalitarianism now seems to have some really weird consequences. And then presumably the deliberation tree that amplification and debate are mimicking is going to have a bunch of thoughts about do we actually want egalitarianism now, what were the moral intuitions that pushed us towards this? Is there some equivalent principle that lets us keep our moral intuitions, but doesn't have this weird property where a single person can decide the outcome of an election, et cetera, et cetera.
I think they were not designed to do this, but by a virtue of being based off like how a human would think, what a human would do if they got a long time and a lot of helpful tools to think about it, they're essentially just inheriting these properties from the human. If the human as the environment would change would start rethinking their priorities or what they care about, then so too would amplification and debate.
Lucas: I think here it also has me thinking about what are the meta-preferences and the meta-meta-preferences and if you could imagine taking a human brain and then running it until the end, through decision and rational and logical thought trees over enough time, with enough epistemics and power behind it to try to sort of navigate its way to the end. It just raises interesting questions about like is that what we want? Is taking that over every single person and then sort of just preference aggregating it all together, is that what we want? And what is the role of moral philosophy for thinking here?
Rohin: Well, so one thing is that whatever moral philosophy you would do so would the amplification of you in theory. I think the benefit of these approaches is that they have this nice property that whatever you would have thought of it in the limit of good AI and idealizations, properly mimicking you and so on, so forth. In this sort of nice world where this all works in a nice, ideal way, it seems like any consideration you can have or you would have so would be agent produced by iterated amplification or debate.
And so if you were going to do a bunch of moral philosophy and come to some sort of decision based on that, so would iterated amplification or debate. So I think it's like basically here is how we build an AI system that solves the problems in the same way that a human would solve them. And so then if you're worried about, hey, maybe humans themselves are just not very good at solving problems. Looks like most humans in the world. Like don't do moral philosophy and don't extrapolate their values well in the future. And the only reason we have moral progress is because younger generations keep getting born and they have different views than the older generations.
That, I think, could in fact be a problem, but I think there's hope that we could like train humans to have them nice sort of properties, good epistemics, such they would provide good training data for iterated amplification if there comes a day where we think we can actually train iterated amplification to mimic human explicit reasoning. They do both have the property that they're only mimicking the explicit reasoning and not necessarily the implicit reasoning.
Lucas: Do you want to unpack that distinction there?
Rohin: Oh, yeah. Sure. So both of them require that you take your high-level question and decompose it into a bunch of sub-questions or sorry, the theoretical model of them has that. This is like pretty clear with iterated amplification. It is less clear with debate. At each point you need to have the top level agent decompose the problem into a bunch of sub-problems. And this basically requires you to be able to decompose tasks into clearly specified sub-tasks, where clearly specified could mean in natural language, but you need to make it explicit in a way that the agent you're assigning the task to can understand it without having to have your mind.
Whereas if I'm doing some sort of programming task or something, often I will just sort of know what direction to go in next, but not be able to cleanly formalize it. So you'll give me some like challenging algorithms question and I'll be like, "Oh, yeah, kind of seems like dynamic programming is probably the right thing to do here." And maybe if I consider it this particular way, maybe if I put these things in the stack or something, but even the fact that I'm saying this out in natural language is misrepresenting my process.
Really there's some intuitive not verbalizable process going on in my head. Somehow navigates to the space of possible programs and picks a thing and I think the reason I can do this is because I've been programming for a lot of time and I've trained a bunch of intuitions and heuristics that I cannot easily verbalize us some like nice decomposition. So that's sort of implicit in this thing. If you did want that to be incorporated in an iterated amplification, it would have to be incorporated in the base agent, the one that you start with. But if you start with something relatively simple, which I think is often what we're trying to do, then you don't get those human abilities and you have to rediscover them in some sense through explicit decompositional reasoning.
Lucas: Okay, cool. Yeah, that's super interesting. So now to frame all of this again, do you want to sort of just give a brief summary of your general views here?
Rohin: I wish there were a nice way to summarize this. That would mean we'd made more progress. It seems like there's a bunch of things that people have proposed. There's amplification/debate, which are very similar, IRL as a general. I think, but I'm not sure, that most of them would agree that we don't want to like infer a utility function and optimize it for the long-term. I think more of them are like, yeah, we want this sort of interactive system with the human and the AI. It's not clear to me how different these are and what they're aiming for in amplification and debate.
So here we're sort of looking at how things change over time and making that a pretty central piece of how we're thinking about it. Initially the AI is trying to help the human, human has some sort of reward function, AI trying to learn it and help them, but over time this changes, the AI has to keep up with it. And under this framing you want to think a lot about interaction, you want to think about getting as many bits about reward from the human to the AI as possible. Maybe think about control theory and how human data is in some sense of control mechanism for the AI.
You'd want to like infer norms and ways that people behave, how people relate with each other, try to have your AI systems do that as well. So that's one camp of things, have the AI interact with humans, behave generally in the way that humans would say is not crazy, update those over time. And then there's the other side which is like have an AI system that is taking human reasoning, human explicit reasoning and doing that better or doing that more, which allows it to do anything that the human would have done, which is more taking the thought process that humans go through and putting that at the center. That is the thing that we want to mimic and make better.
Sort of parts where our preferences change over time is something that you get for free in some sense by mimicking human thought processes or reasoning. Summary, those are two camps. I am optimistic about both of them, think that people should be doing research on both of them. I don't really have much more of a perspective of that, I think.
Lucas: That's excellent. I think that's a super helpful overview actually. And given that, how do you think that your views of AI alignment have changed over the past few years?
Rohin: I'll note that I've only been in this field for I think 15, 16 months now, so just over a year, but over that year I definitely came into it thinking what we want to do is infer the correct utility function and optimize it. And I have moved away quite strongly from that. I, in fact, recently started writing a value learning sequence or maybe collating is a better word. I've written a lot of posts that still have to come out, but I also took a few posts from other people.
The first part of that sequence is basically arguing seems bad to try and define a utility function and then optimize it. So I'm just trying to move away from long-term utility functions in general or long-term goals or things like this. That's probably the biggest update since starting. Other things that I've changed, a focus more on norms than on values, trying to do things that are easy to infer right now in the current environment and that making sure that we update on these over time as opposed to trying to get the one true thing that depends on us solving all the hard metaphilosophical problems. That's, I think, another big change in the way I've been thinking about it.
Lucas: Yeah. I mean, there are different levels of alignment at their core.
Rohin: Wait, I don't know exactly what you mean by that.
Lucas: There's your original point of view where you said you came into the field and you were thinking infer the utility function and maximize it. And your current view is now that you are moving away from that and beginning to be more partial towards the view which takes it that we want to be like inferring from norms in the present day just like current preferences and then optimizing that rather than extrapolating towards some ultimate end-goal and then trying to optimize for that. In terms of aligning in these different ways, isn't there a lot of room for value drift, allowing the thing to run in the real world rather than amplifying explicit human thought on a machine?
Rohin: Value drift if is an interesting question. In some sense, I do want my values to drift in that whatever I think about the correct way that the future should go or something like that today. I probably will not endorse that in the future and I endorse the fact that I won't endorse it in the future. I do want to learn more and then figure out what to do in the future based on that. You could call that value drift that is a thing. I want to happen. So in that sense then value drift wouldn't be a bad thing, but then there's also a sense in which there are ways in which my values could change in the future and ways that I don't endorse and then that one maybe is value drift. That is bad.
So yeah, if you have an AI system that's operating in the real world and changes over time as we humans change, yes, there will be changes at what the AI system is trying to achieve over time. You could call that value drift, but value drift usually has a negative connotation, whereas like this process of learning as the environment changes seems to be to me like a positive thing. It's a thing I would want to do myself.
Lucas: Yeah, sorry, maybe I wasn't clear enough. In the case of running human beings in the real world, where there are like the causes and effects of history and whatever else and how that actually will change the expression of people over time. Because if you're running this version of AI alignment where you're sort of just always optimizing the current set of values in people, progression of the world and of civilization is only as good as the best of all human like values and preferences in that moment.
It's sort of like limited by what humans are in that specific environment and time, right? If you're running that in the real world versus running some sort of amplified version of explicit human reasoning, don't you think that they're going to come to different conclusions?
Rohin: I think the amplified explicit human reasoning, I imagine that it's going to operate in the real world. It's going to see changes that happen. It might be able to predict those changes and then be able to figure out how to respond fast, before the changes even happen perhaps, but I still think of amplification as being very much embedded in the real world. Like you're asking it questions about things that happen in the real world. It's going to use explicit reasoning that it would have used if a human were in the real world and thinking about the question.
I don't really see much of a distinction here. I definitely think that even in my setting where I'm imagining AI systems that evolve over time and change based on that, that they are going to be smarter than humans, going to think through things a lot faster, be able to predict things in advance in the same way that simplified explicit reasoning would. Maybe there are differences, but value drift doesn't seem like one of them or at least I cannot predict right now how they will differ along the axis of value drift.
Lucas: So then just sort of again taking a step back to the ways in which your views have shifted over the past few years. Is there anything else there that you'd like to touch on?
Rohin: Oh man, I'm sure there is. My views changed so much because I was just so wrong initially.
Lucas: So most people listening should think that if given a lot more thought on this subject, that their views are likely to be radically different than the ones that they currently have and the conceptions that they currently have about AI alignment.
Rohin: Seems true from most listeners, yeah. Not all of them, but yeah.
Lucas: Yeah, I guess it's just an interesting fact. Do you think this is like an experience of most people who are working on this problem?
Rohin: Probably. I mean, within the first year of working on the problem that seems likely. I mean just in general if you work on the problem, if you start with near no knowledge on something and then you work on it for a year, your views should change dramatically just because you've learned a bunch of things and I think that basically explains most of my changes in view.
It's just actually hard for me to remember all the ways in which I was wrong back in the past and I focused on not using utility functions because I think that even other people in the field still believe right now. So that's where that one came from, but there are like plenty of other things that are just notably, easily, demonstrably wrong about that I'm having trouble recalling now.
Lucas: Yeah, and the utility function one I think is a very good example and I think that if it were possible to find all of these in your brain and distill them, I think it would make a very, very good infographic on AI alignment, because those misconceptions are also misconceptions that I've had and I share those and I think that I've seen them also in other people. A lot of sort of the intellectual blunders that you or I have made are probably repeated quite often.
Rohin: I definitely believe that. Yeah, I guess I could talk about the things that I'm going to very soon saying the value learning sequence. Those were definitely updates that I made, one of those a utility functions thing. Another one was thinking about what we want is for the human AI system as a whole to be optimizing for some sort of goal. And this opens up a nice space of possibilities where the AI is not optimizing a goal, only the human AI system together is. Keeping in mind that that is the goal and not just the AI itself must be optimizing some sort of goal.
The idea of corrigibility itself as a thing that we should be aiming for was a pretty big update for me, took a while for me to get to that one. I think distributional shift was a pretty key concept that I learned at some point and started applying everywhere. One way of thinking about the evolving preferences over time thing is that humans, they've been trained on the environment that we have right now and arguably we've been trained on the ancestral environment too by evolution, but we haven't been trained on whatever the future is going to be.
Or for a more current example, we haven't been trained on social media. Social media is a fairly new thing affecting us in ways that we hadn't considered in the past and this is causing us to change how we do things. So in some sense what's happening is as we go into the future, we're encountering a distributional shift and human values don't extrapolate well to that distributional shift. What do you actually need to do is wait for the humans to get to that point, let them experience it, train on it, have their values be trained on this new distribution and then figure out what they are rather than trying to do it right now when their values are just going to be wrong or going to be not what they would get if they were actually in that situation.
Lucas: Isn't that sort of summarizing coherent extrapolated volition?
Rohin: I don't know that coherent extrapolated volition explicitly talks about having the human be in a new environment. I guess you could imagine that CEV considers ... If you imagine like a really, really long process of deliberation in CEV, then you could be like, okay what would happen if I were in this environment and all these sorts of things happened. It seems like you would need to have a good model of how the world works and how physics works in order to predict what the environment would be like. Maybe you can do that and then in that case you simulate a bunch of different environments and you think about how humans would adapt and evolve and respond to those environments and then you take all of that together and you summarize it and distill it down into a single utility function.
Plausibly that could work. Doesn't seem like a thing we can actually build, but as a definition of what we might want, that seems not bad. I think that is me putting the distributional shift perspective on CEV and it was not, certainly not obvious to me from the statement of CEV itself, that you're thinking about how to mitigate the impact of distributional shift on human values. I think I've had this perspective and I've put it on CEV and I'm like, yeah, that seems fine, but it was not obvious to me from reading about CEV alone.
Lucas: Okay, cool.
Rohin: I recently posted a comment on the Alignment Forum talking about how we want to like ... I guess this is sort of in corrigibility ability too, making an AI system that tries to help us as opposed to making an AI system that is optimizing the one true utility function. So that was an update I made, basically the same update as the one about aiming for corrigibility. I guess another update I made is that while there is a phase transition or something or like a sharp change in the problems that we see when AIs become human level or super-intelligent, I think the underlying causes of the problems don't really change.
Underlying causes of problems with narrow AI systems, probably similar to the ones that underlie a super intelligent systems. Having their own reward function leads to problems both in narrow settings and in super-intelligent settings. This made me more optimistic about doing work trying to address current problems, but with an eye towards long-term problems.
Lucas: What made you have this update?
Rohin: Thinking about the problems a lot, in particular thinking about how they might happen in current systems as well. So I guess a prediction that I would make is that if it is actually true that superintelligence would end up killing us all or something like that, some like really catastrophic outcome. Then I would predict that before that, we will see some AI system that causes some other smaller scale catastrophe where I don't know what catastrophe means, it might be something like oh, you humans die or oh, the power grid went down for some time or something like that.
And then before that we will have things that sort of fail in relatively not important ways, but in ways of say that like here's an underlying problem that we need to fix with how we build AI systems. If you extrapolate all the way back to today that looks like for example to boat racing example from open AI, a reward hacking one. So generally expecting things to be more continuous. Not necessarily slow, but continuous. That update I made because of the posts arguing for slow take off from Paul Christiano and AI impacts.
Lucas: Right. And the view there is sort of that the world will be propagated with lower-level ML as we sort of start to ratchet up the capability of intelligence. So a lot of tasks will sort of be ... Already being done by systems that are slightly less intelligent than the current best system. And so all work ecosystems will already be fully flooded with AI systems optimizing within the spaces. So there won't be a lot of space for the first AGI system or whatever to really get decisive strategic advantage.
Rohin: Yeah, would I make prediction that we won't have a system that gets a decisive strategic advantage? I'm not sure about that one. It seems plausible to me that we have one AI system that is improving over time and we use those improvements in society for before it becomes super intelligent. But then by the time it becomes super intelligent, it is still the one AI system that is super intelligent. So it does gain a decisive strategic advantage.
An example of this would be if there was just one main AGI project, I would still predict that progress on AI, it would be continuous, but I would not predict a multipolar outcome in that scenario. The corresponding view is that while I still do use the terminology first AGI because it's like pointing out some intuitive concept that I think is useful, it's a very, very fuzzy concept and I don't think we'll be able to actually point at any particular system and say that was the first AGI. Rather we'll point to like a broad swath of time and say, "Somewhere in there AI had became generally intelligent."
Lucas: There are going to be all these sort of like isolated meta-epistemic reasoning tools which can work in specific scenarios, which will sort of potentially aggregate in that fuzzy space to create something fully general.
Rohin: Yep. They're going to be applied in some domains and then the percent of domains in which they apply will gradually grow grutter and eventually we'll be like, huh, looks like there's nothing left for humans to do. It probably won't be a surprise, but I don't think there will be a particular point where everyone agrees, yep, looks like AI is going to automate everything in just a few years. It's more like AI will start automating a bunch of stuff. The amount of stuff it automates will increase over time. Some people will see it coming, see full automation coming earlier, some people will be like nah, this is just a simple task that AI can do, still got a long ways to go for all the really generally intelligent stuff. People will sign on to like oh, yeah, it's actually becoming generally intelligent at different spots.
Lucas: Right. If you have a bunch of small mammalian level AIs automating a lot of stuff in industry, there would likely be a lot of people whose timelines would be skewed in the wrong direction.
Rohin: I'm not even sure this was a point of timelines. It was just a point of like which is the system that you call AGI. I claim this will not have a definitive answer. So that was also an update to how I was thinking. That one, I think, is like more generally accepted in the community. And this was more like well, all of the literature on the AI safety that's publicly available and like commonly read by EA's doesn't really talk about these sorts of points. So I just hadn't encountered these things when I started out. And then I encountered a more maybe I thought to myself, I don't remember, but like once I encountered the arguments I was like, yeah, that makes sense and maybe I should have thought of that before.
Lucas: In the sequence which you're writing, do you sort of like cover all of these items which you didn't think were in the mainstream literature?
Rohin: I cover some of them. The first few things I told you were I was just like what did I say in the sequence. There were a few I think that probably aren't going to be in that sequence just because there's a lot of stuff that people have not written down.
Lucas: It's pretty interesting because the way in which the AI alignment field is evolving is sometimes, it's often difficult to have a bird's-eye view of where it is and track avant-guard ideas being formulated in people's brains and being shared.
Rohin: Yeah. I definitely agree. I was hoping that the Alignment Newsletter, which I write, to help with that. I would say it probably speeds up the process of bit, but it's definitely not keeping you on the forefront. There are many ideas that I've heard about, that I've even read documents about that haven't made it in the newsletter yet because they haven't become public.
Lucas: So how many months behind do you think for example, the newsletter would be?
Rohin: Oh, good question. Well, let's see. There's a paper that I started writing in May or April that has not made it into the newsletter yet. There's a paper that I finished and submitted in October that has not made it to the newsletter yet, or was it September, possibly September. That one will come out soon. That suggests a three month lag. But I think many others have been longer than that. Admittedly, this is for academic researchers at CHAI. I think CHAI is like we tend to publish using papers and not blog posts and this results in the longer delay on our side.
Also because work on relative reachability, for example, I've learned about quite a bit. I learned about maybe four or five months before she released it and that's when it came out in the newsletter. And of course, she'd been working on it for longer or like AI safety by debate I think I learned about six or seven months before it was published in came out in the newsletter. So yeah, somewhere between three months and half a year for things seems likely. For things that I learned from MIRI, it's possible that they never get into the newsletter because they're never made public. So yeah, there's a fairly broad range there.
Lucas: Okay. That's quite interesting. I think that also sort of gives people a better sense of what's going on in technical AI alignment because it can seem kind of black boxy.
Rohin: Yeah. I mean, in some sense this is a thing that all fields have. I used to work in programming languages. On there we would often write a paper and submit it and then go and present about it a year later by the time we had moved on, done a whole other project and written other paper and then we'd go back and we'd talk about this. I definitely remember sometimes grad students being like, "Hey, I want to get this practice document." I say, "What's it about?" It's like some topic. And I'm like wait, but you did that. I heard about this like two years ago. And they're like, yep, just got published.
So in that sense, I think both AI is faster and AI alignment is I think even faster than AI because it's a smaller field and people can talk to each other more, and also because a lot of us write blog posts. Blog posts are great.
Lucas: They definitely play a crucial role within the community in general. So I guess just sort of tying things up a bit more here, pivoting back to a broader view. Given everything that you've learned and how your ideas have shifted, what are you most concerned about right now in AI alignment? How are the prospects looking to you and how does the problem of AI alignment look right now to Rohin Shah?
Rohin: I think it looks pretty tractable, pretty good. Most of the problems that I see are I think ones that we can see in advance, we probably can solve. None of these seem like particularly impossible to me. I think I also give more credit to the machine learning community or AI community than other researchers do. I trust in our ability where here are meaning like the AI field broadly, our ability to notice what things could go wrong and fix them in a way that maybe other researchers in the AI safety don't.
I think one of the things that feels most problematic to me right now is the problem of inner optimizers, which I'm told there will probably be a sequence on in the future because there aren't great resources on it right now. So basically this is the idea of if you run a search process over a wide space of strategies or options and you search for something that gets you good external reward or something like that, what you might end up finding is a strategy that is itself a consequentialist agent that's optimizing for its own internal reward and that internal reward will agree with the external reward on the training data because that's why it was selected, but it might diverge soon as there's any distribution shift.
And then it might start optimizing against us adversarially in the same way that you would get if you like gave a misspecified award function to and RL system today. This seems plausible to me. I've read a bit more about this and talk to people about this and things that aren't yet public, but hopefully will soon be. I definitely recommend reading that if it ever comes out, but yeah, this seems like it could be a problem. I don't think we have any instance of it being a problem yet. Seems hard to detect and I'm not sure how I would fix it right now.
But I also don't think that we've thought about the problem or I don't think I've thought about the problem that much. I don't want to say like, "Oh man, this is totally unsolvable," yet. Maybe I'm just an optimistic person by nature. I mean, that's definitely true, but maybe that's biasing my judgment here. Feels like we could probably solve that if it ends up being a problem.
Lucas: Is there anything else here that you would like to wrap up on in terms of AI alignment or inverse reinforcement learning?
Rohin: I want to continue to exhort that we should not be trying to solve all the metaphilosophical problems and we should not be trying to like infer the one true utility function and we should not be modeling an AI as pursuing a single goal over the long-term. That is a thing I want to communicate to everybody else. Apart from that I think we've covered everything at a good depth. Yeah, I don't think there's anything else I'd add to that.
Lucas: So given that I think rather succinct distillation of what we are trying not to do, could you try and offer an equally succinct distillation of what we are trying to do?
Rohin: I wish I could. That would be great, wouldn't it? I can tell you that I can't do that. I could give you like a suggestion on what we are trying to do instead, which would be try to build an AI system that is corrigible, that is doing what we want, but it's going to remain under human control in some sense. It's going to ask us, take our preferences into account, not try to go off behind our backs and optimize against us. That is a summary of a path that we could go down that I think is premised or what I would want our AI systems to be like. But that's unfortunately very sparse on concrete details because I don't know those concrete details yet.
Lucas: Right. I think that that sort of perspective shift is quite important. I think it changes the nature of the problem and how one thinks about the problem, even at the societal level.
Rohin: Yeah. Agreed.
Lucas: All right. So thank you so much Rohin, it's really been a pleasure. If people are interested in checking out some of this work that we have mentioned or following you, where's the best place to do that?
Rohin: I have a website. It is just RohinShah.com. Subscribing to the Alignment Newsletter is ... Well, it's not a great way to figure out what I personally believe. Maybe if you'd keep reading the newsletter over time and read my opinions for several weeks in a row, maybe then you'd start getting a sense of what Rohin thinks. It will soon have links to my papers and things like that, but yeah, that's probably the best way on this, like my website. I do have a Twitter, but I don't really use it.
Lucas: Okay. So yeah, thanks again Rohin. It's really been a pleasure. I think that was a ton to think about and I think that I probably have a lot more of my own thinking and updating to do based off of this conversation.
Rohin: Great. Love it when that happens.
Lucas: So yeah. Thanks so much. Take care and talk again soon.
Rohin: All right. See you soon.
Lucas: If you enjoyed this podcast, please subscribe, give it a like or share it on your preferred social media platform. We'll be back again soon with another episode in the AI Alignment series.
Related episodes
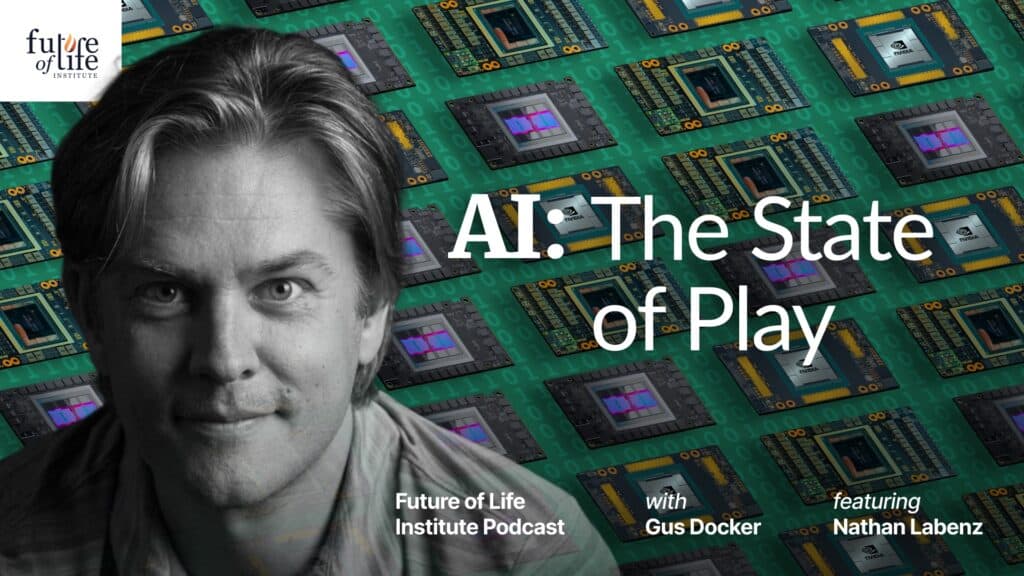
Nathan Labenz on the State of AI and Progress since GPT-4
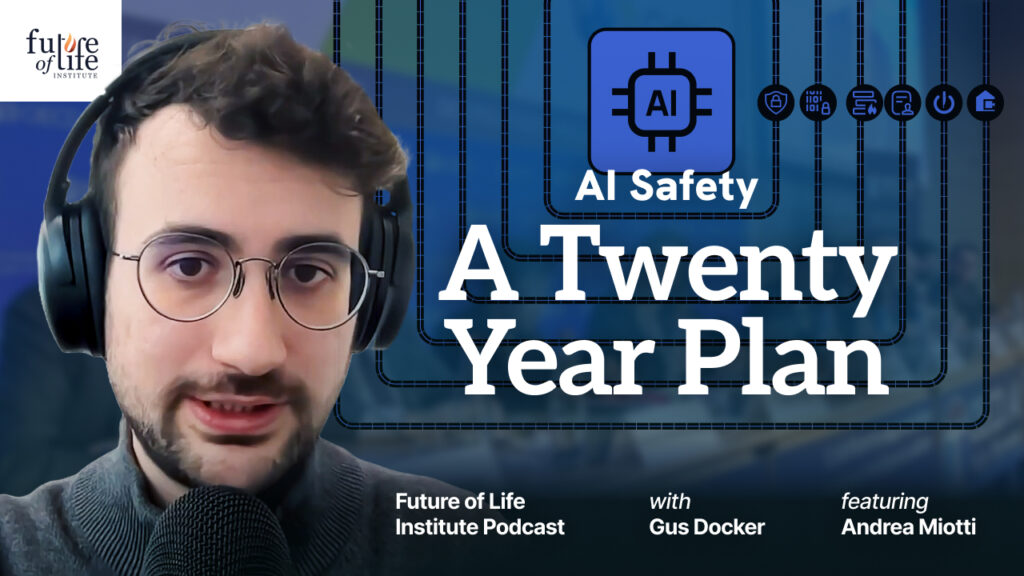