AI Alignment Podcast: Human Compatible: Artificial Intelligence and the Problem of Control with Stuart Russell
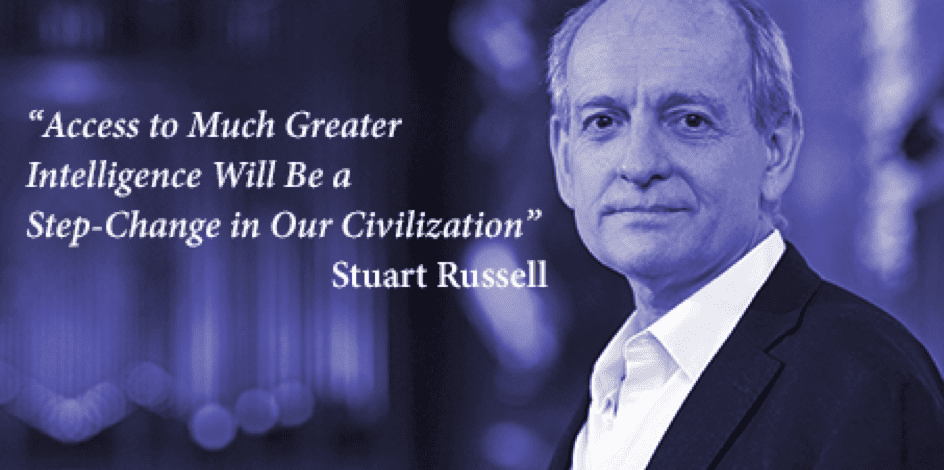
Stuart Russell is one of AI's true pioneers and has been at the forefront of the field for decades. His expertise and forward thinking have culminated in his newest work, Human Compatible: Artificial Intelligence and the Problem of Control. The book is a cornerstone piece, alongside Superintelligence and Life 3.0, that articulates the civilization-scale problem we face of aligning machine intelligence with human goals and values. Not only is this a further articulation and development of the AI alignment problem, but Stuart also proposes a novel solution which bring us to a better understanding of what it will take to create beneficial machine intelligence.
 Topics discussed in this episode include:
- Stuart's intentions in writing the book
- The history of intellectual thought leading up to the control problem
- The problem of control
- Why tool AI won't work
- Messages for different audiences
- Stuart's proposed solution to the control problem
Key points from Stuart:Â
- Â "I think it was around 2013 that it really struck me that in fact we'd been thinking about AI the wrong way all together. The way we had set up the whole field was basically kind of a copy of human intelligence in that a human is intelligent, if their actions achieve their goals. And so a machine should be intelligent if its actions achieve its goals. And then of course we have to supply the goals in the form of reward functions or cost functions or logical goals statements. And that works up to a point. It works when machines are stupid. And if you provide the wrong objective, then you can reset them and fix the objective and hope that this time what the machine does is actually beneficial to you. But if machines are more intelligent than humans, then giving them the wrong objective would basically be setting up a kind of a chess match between humanity and a machine that has an objective that's across purposes with our own. And we wouldn't win that chess match."
- "So when a human gives an objective to another human, it's perfectly clear that that's not the sole life mission. So you ask someone to fetch the coffee, that doesn't mean fetch the coffee at all costs. It just means on the whole, I'd rather have coffee than not, but you know, don't kill anyone to get the coffee. Don't empty out my bank account to get the coffee. Don't trudge 300 miles across the desert to get the coffee. In the standard model of AI, the machine doesn't understand any of that. It just takes the objective and that's its sole purpose in life. The more general model would be that the machine understands that the human has internally some overall preference structure of which this particular objective fetch the coffee or take me to the airport is just a little local manifestation. And machine's purpose should be to help the human realize in the best possible way their overall preference structure. If at the moment that happens to include getting a cup of coffee, that's great or taking him to the airport. But it's always in the background of this much larger preference structure that the machine knows and it doesn't fully understand. One way of thinking about is to say that the standard model of AI assumes that the machine has perfect knowledge of the objective and the model I'm proposing assumes that the model has imperfect knowledge of the objective or partial knowledge of the objective. So it's a strictly more general case."
- "The objective is to reorient the field of AI so that in future we build systems using an approach that doesn't present the same risk as the standard model... That's the message I think for the AI community is the first phase our existence maybe should come to an end and we need to move on to this other way of doing things. Because it's the only way that works as machines become more intelligent. We can't afford to stick with the standard model because as I said, systems with the wrong objective could have arbitrarily bad consequences."
Important timestamps:Â
0:00 Intro
2:10 Intentions and background on the book
4:30 Human intellectual tradition leading up to the problem of control
7:41 Summary of the structure of the book
8:28 The issue with the current formulation of building intelligent machine systems
10:57 Beginnings of a solution
12:54 Might tool AI be of any help here?
16:30 Core message of the book
20:36 How the book is useful for different audiences
26:30 Inferring the preferences of irrational agents
36:30 Why does this all matter?
39:50 What is really at stake?
45:10 Risks and challenges on the path to beneficial AI
54:55 We should consider laws and regulations around AI
01:03:54 How is this book differentiated from those like it?
Works referenced:
Human Compatible: Artificial Intelligence and the Problem of Control
Occam’s razor is insufficient to infer the preferences of irrational agents
Synthesizing a human's preferences into a utility function with Stuart Armstrong
We hope that you will continue to join in the conversations by following us or subscribing to our podcasts on Youtube, Spotify, SoundCloud, iTunes, Google Play, Stitcher, iHeartRadio, or your preferred podcast site/application. You can find all the AI Alignment Podcasts here.
Transcript
Lucas: Hey everyone, welcome back to the AI Alignment Podcast. I'm Lucas Perry and today we'll be speaking with Stuart Russell about his new book, Human Compatible: Artificial Intelligence and The Problem of Control. Daniel Kahneman says "This is the most important book I have read in quite some time. It lucidly explains how the coming age of artificial super intelligence threatens human control. Crucially, it also introduces a novel solution and a reason for hope."
Yoshua Bengio says that "This beautifully written book addresses a fundamental challenge for humanity: increasingly intelligent machines that do what we ask, but not what we really intend. Essential reading if you care about our future."
I found that this book helped clarify both intelligence and AI to me as well as the control problem born of the pursuit of machine intelligence. And as mentioned, Stuart offers a reconceptualization of what it means to build beneficial and intelligent machine systems. That provides a crucial place of pivoting and how we ought to be building intelligent machines systems.
Many of you will already be familiar with Stuart Russell. He is a professor of computer science and holder of the Smith-Zadeh chair in engineering at the University of California, Berkeley. He has served as the vice chair of the World Economic Forum's Council on AI and Robotics and as an advisor to the United Nations on arms control. He is an Andrew Carnegie Fellow as well as a fellow of the Association for The Advancement of Artificial Intelligence, the Association for Computing Machinery and the American Association for the Advancement of Science.
He is the author with Peter Norvig of the definitive and universally acclaimed textbook on AI, Artificial Intelligence: A Modern Approach. And so without further ado, let's get into our conversation with Stuart Russell.
Let's start with a little bit of context around the book. Can you expand a little bit on your intentions and background for writing this book in terms of timing and inspiration?
Stuart: I've been doing AI since I was in high school and for most of that time the goal has been let's try to make AI better because I think we'll all agree AI is mostly not very good. When we wrote the first edition of the textbook, we decided to have a section called, What If We Do Succeed? Because it seemed to me that even though everyone was working on making AI equivalent to humans or better than humans, no one was thinking about what would happen if that turned out to be successful.
So that section in the first edition in 94 was a little equivocal, let's say, you know, we could lose control or we could have a golden age and let's try to be optimistic. And then by the third edition, which was 2010 the idea that we could lose control was fairly widespread, at least outside the AI communities. People worrying about existential risk like Steve Omohundro, Eliezer Yudkowsky and so on.
So we included those a little bit more of that viewpoint. I think it was around 2013 that it really struck me that in fact we'd been thinking about AI the wrong way all together. The way we had set up the whole field was basically kind of a copy of human intelligence in that a human is intelligent, if their actions achieve their goals. And so a machine should be intelligent if its actions achieve its goals. And then of course we have to supply the goals in the form of reward functions or cost functions or logical goals statements. And that works up to a point. It works when machines are stupid. And if you provide the wrong objective, then you can reset them and fix the objective and hope that this time what the machine does is actually beneficial to you. But if machines are more intelligent than humans, then giving them the wrong objective would basically be setting up a kind of a chess match between humanity and a machine that has an objective that's across purposes with our own. And we wouldn't win that chess match.
So I started thinking about how to solve that problem. And the book is a result of the first couple of years of thinking about how to do it.
Lucas: So you've given us a short and concise history of the field of AI alignment and the problem of getting AI systems to do what you want. One of the things that I found so great about your book was the history of evolution and concepts and ideas as they pertain to information theory, computer science, decision theory and rationality. Chapters one through three you sort of move sequentially through many of the most essential concepts that have brought us to this problem of human control over AI systems.
Stuart: I guess what I'm trying to show is how ingrained it is in intellectual thought going back a couple of thousand years. Even in the concept of evolution, this notion of fitness, you know we think of it as an objective that creatures are trying to satisfy. So in the 20th century you had a whole lot of disciplines, economics developed around the idea of maximizing utility or welfare or profit depending on which branch you look at. Control theory is about minimizing a cost function, so the cost function described some deviation from ideal behavior and then you build systems that minimize the cost. Operations research, which is dynamic programming and Markov decision processes is all about maximizing the sum of rewards. And statistics if you set it up in general, is about minimizing an expected loss function.
So all of these disciplines have the same bug if you like. It's a natural way to set things up, but in the long run we'll just see it as a bad cramped way of doing engineering. And what I'm proposing in the book actually is a way of thinking about it that's much more in a binary rather than thinking about the machine and it's objective.
You think about this coupled system with humans or you know, it could be any entity that wants a machine to do something good for it or another system to do something good for it. And then the system itself, which is supposed to do something good for the human or whatever else it is that wants something good to happen. So this kind of coupled system, don't really see that in the intellectual tradition. Maybe one exception that I know of, which is the idea of principle agent games in economics. So a principal might be an employer and the agent might be the employee. And then the game is how does the employer get the employee to do something that the employer actually wants them to do, given that the employee, the agent has their own utility function and would rather be sitting home drinking beers and watching football on the telly.
How do you get them to show up at work and do all kinds of things they wouldn't normally want to do? The simplest way is you pay them. But you know, there's all kinds of other ideas about incentive schemes and status and then various kinds of sanctions if people don't show up and so on. So the economists study that notion, which is a coupled system where one entity wants to benefit from the behavior of another.
So that's probably the closest example that we have. And then maybe in ecology, look at symbiotic species or something like that. But there's not very many examples that I'm aware of. In fact, maybe I can't think of any, where the entity that's supposedly in control, namely us, is less intelligent than the entity that it's supposedly controlling, namely the machine.
Lucas: So providing some framing and context here for the listener, the first part of your book, chapters one through three explores the idea of intelligence in humans and in machines. There you give this historical development of ideas and I feel that this history you give of computer science and the AI alignment problem really helps to demystify both the person and evolution as a process and the background behind this problem.
Your second part of your book, chapters four through six discusses some of the problems arising from imbuing machines with intelligence. So this is a lot of the AI alignment problem considerations. And then the third part, chapter seven through ten suggests a new way to think about AI, to ensure that machines remain beneficial to humans forever.
You've begun stating this problem and readers can see in chapters one through three that this problem goes back a long time, right? The problem with computer science at its inception was that definition that you gave that a machine is intelligent in so far as it is able to achieve its objectives. In reaction to this, you've developed cooperative inverse reinforcement learning and inverse reinforcement learning, which is sort of part of the latter stages of this book where you're arguing for new definition that is more conducive to alignment.
Stuart: Yeah. In the standard model as I call it in the book, the humans specifies the objective and plugs it into the machine. If for example, you get in your self driving car and it says, "Where do you want to go?" And you say, "Okay, take me to the airport." For current algorithms as we understand them, understand built on this kind of model, that objective becomes the sole life purpose of the vehicle. It doesn't necessarily understand that in fact that's not your sole life purpose. If you suddenly get a call from the hospital saying, oh, you know, your child has just been run over and is in the emergency room. You may well not want to go to the airport. Or if you get into a traffic jam and you've already missed the last flight, then again you might not want to go to the airport.
So when a human gives an objective to another human, it's perfectly clear that that's not the sole life mission. So you ask someone to fetch the coffee, that doesn't mean fetch the coffee at all costs. It just means on the whole, I'd rather have coffee than not, but you know, don't kill anyone to get the coffee. Don't empty out my bank account to get the coffee. Don't trudge 300 miles across the desert to get the coffee.
In the standard model of AI, the machine doesn't understand any of that. It just takes the objective and that's its sole purpose in life. The more general model would be that the machine understands that the human has internally some overall preference structure of which this particular objective fetch the coffee or take me to the airport is just a little local manifestation. And machine's purpose should be to help the human realize in the best possible way their overall preference structure.
If at the moment that happens to include getting a cup of coffee, that's great or taking him to the airport. But it's always in the background of this much larger preference structure that the machine knows and it doesn't fully understand. One way of thinking about is to say that the standard model of AI assumes that the machine has perfect knowledge of the objective and the model I'm proposing assumes that the model has imperfect knowledge of the objective or partial knowledge of the objective. So it's a strictly more general case.
When the machine has partial knowledge of the objective there's whole lot of new things that come into play that simply don't arise when the machine thinks it knows the objective. For example, if the machine knows the objective, it would never ask permission to do an action. It would never say, you know, is it okay if I do this because it believes that it's already extracted all there is to know about human preferences in the form of this objective. And so whatever plan it formulates to achieve the objective must be the right thing to do.
Whereas a machine that knows that it doesn't know the full objective could say, well, given what I know, this action looks okay, but I want to check with the boss before going ahead because it might be that this plan actually violate some part of the human preference structure that it doesn't know about. So you get machines that ask permission, you get machines that, for example, allow themselves to be switched off because the machine knows that it might do something that will make the human unhappy. And if the human wants to avoid that and switches the machine off, that's actually a good thing. Whereas a machine that has a fixed objective would never want to be switched off because that guarantees that it won't achieve the objective.
So in the new approach you have a strictly more general repertoire of behaviors that the machine can exhibit. The idea of inverse reinforcement learning is this is the way for the machine to actually learn more about what the human preference structure is. By observing human behavior, which could be verbal behavior, like, could you fetch me a cup of coffee? That's a fairly clear indicator about your preference structure, but it could also be that you know, you ask a human question and the human doesn't reply. Maybe the human's mad at you and is unhappy about the line of questioning that you're pursuing.
 So human behavior means everything humans do and have done in the past. So everything we've ever written down, every movie we've made, every television broadcast contains information about human behavior and therefore about human preferences. Inverse reinforcement learning really means how do we take all that behavior and learn human preferences from it?
Lucas: What can you say about how tool AI as a possible path to AI alignment fits in this schema where we reject the standard model, as you call it, in favor of this new one?
Stuart: Tool AI is a notion, oddly enough, it doesn't really occur within the field of AI. It's a phrase that came from people who are thinking from the outside about possible risks from AI. And what it seems to mean is the idea that rather than buildings general purpose intelligence systems. If you are building AI systems designed for some specific purpose, then that's sort of innocuous and doesn't present any risks. And some people argue that in fact if you just have a large collection of these innocuous application specific AI systems, then there's nothing to worry about.
My experience of tool AI is that when you build applications specific systems, you can kind of do it in two ways. One is you kind of hack it. In other words, you figure out how you would do this task and then you write a whole bunch of very, very special purpose code. So, for example, if you were doing handwriting recognition, you might think, oh, okay, well in order to find an ‘S’ I have to look for a line that's curvy and I follow the line and it has to have three bends, it has to be arranged this way. And you know, you write a whole bunch of tests to check each characteristic of an ad that it has all these characteristics and it doesn't have any loops and this, that and the other. And then you see okay, that's an S.
And that's actually not the way that people went about the problem of handwriting recognition. The way that they did it was to develop machine learning systems that could take images of characters that were labeled and then train a recognizer that could recognize new instances of characters. And in fact, Yann LeCun at AT&T was doing a system that was designed to recognize words and figures on checks. So very, very, very application specific, very tooley and order to do that he invented convolutional neural networks. Which is what we now call deep learning.
So, out of this very, very narrow piece of tool AI came this very, very general technique. Which has solved or largely solved object recognition, speech recognition, machine translation, and some people argue will produce general purpose AI. So I don't think there's any safety to be found in focusing on tool AI.
The second point is that people feel that somehow to tool AI is not an agent. So an agent meaning a system that you can think of as perceiving the world and then taking actions. And again, I'm not sure that's really true. So a Go program is an agent. It's an agent that operates in a small world, namely the Go board, but it perceives the board, the move that's made and it takes action.
It chooses what to do next in many applications like this, this is the really the only way to build an effective tool is that it should be an agent. If it's a little vacuum cleaning robot or lawn mowing robot, certainly a domestic robot that's supposed to keep your house clean and look after the dog while you're out. There's simply no way to build those kinds of systems except as agents and as we improve the capabilities of these systems, whether it's for perception or planning and behaving in the real physical world. We're effectively going to be creating general purpose intelligent agents. I don't really see salvation in the idea that we're just going to build applications specific tools.
Lucas: So that helps to clarify that tool AI do not get around this update that you're trying to do with regards to the standard model. So pivoting back to intentions surrounding the book, if you could distill the core message or the central objective in writing this book, how would you say that?
Stuart: The objective is to reorient the field of AI so that in future we build systems using an approach that doesn't present the same risk as a standard model. I'm addressing multiple audiences. That's the message I think for the AI community is the first phase our existence maybe should come to an end and we need to move on to this other way of doing things. Because it's the only way that works as machines become more intelligent. We can't afford to stick with the standard model because as I said, systems with the wrong objective could have arbitrarily bad consequences.
Then the other audience is the general public, people who are interested in policy, how things are going to unfold in future and technology and so on. For them, I think it's important to actually understand more about AI rather than just thinking of AI as this kind of magic juice that triples the value of your startup company. It's a collection of technologies and those technologies have been built within a framework, the standard model that has been very useful and is shared with these other fields, economic, statistics, operations of search, control theory. But that model does not work as we move forward and we're already seeing places where the failure of the model is having serious negative consequences.
One example would be what's happened with social media. So social media algorithms, content selection algorithms are designed to show you stuff or recommend stuff in order to maximize click-through. Clicking is what generates revenue for the social media platforms. And so that's what they tried to do and I almost said they want to show you stuff that you will click on. And that's what you might think is the right solution to that problem, right? If you want to maximize, click-through, then show people stuff they want to click on and that sounds relatively harmless.
Although people have argued that this creates a filter bubble or a little echo chamber where you only see stuff that you like and you don't see anything outside of your comfort zone. That's true. It might tend to cause your interests to become narrower, but actually that isn't really what happened and that's not what the algorithms are doing. The algorithms are not trying to show you the stuff you like. They're trying to turn you into predictable clickers. They seem to have figured out that they can do that by gradually modifying your preferences and they can do that by feeding you material. That's basically, if you think of a spectrum of preferences, it's to one side or the other because they want to drive you to an extreme. At the extremes of the political spectrum or the ecological spectrum or whatever image you want to look at. You’re apparently a more predictable clicker and so they can monetize you more effectively.
So this is just a consequence of reinforcement learning algorithms that optimize click-through. And in retrospect, we now understand that optimizing click-through was a mistake. That was the wrong objective. But you know, it's kind of too late and in fact it's still going on and we can't undo it. We can't switch off these systems because there's so tied in to our everyday lives and there's so much economic incentive to keep them going.
So I want people in general to kind of understand what is the effect of operating these narrow optimizing systems that pursue these fixed and incorrect objectives. The effect of those on our world is already pretty big. Some people argue that operation's pursuing the maximization of profit have the same property. They're kind of like AI systems. They're kind of super intelligent because they think over long time scales, they have massive information, resources and so on. They happen to have human components, but when you put a couple of hundred thousand humans together into one of these corporations, they kind of have this super intelligent understanding, manipulation capabilities and so on.
Lucas: This is a powerful and important update for research communities. I want to focus here in a little bit on the core messages of the book as per each audience because I think you can say and clarify different things for different people. So for example, my impressions are that for sort of laypersons who are not AI researchers, the history of ideas that you give clarifies the foundations of many fields and how it has led up to this AI alignment problem. As you move through and past single agent cases to multiple agent cases where we give rise to game theory and decision theory and how that all affects AI alignment.
So for laypersons, I think this book is critical for showing the problem, demystifying it, making it simple, and giving the foundational and core concepts for which human beings need to exist in this world today. And to operate in a world where AI is ever becoming a more important thing.
And then for the research community, as you just discussed, it seems like this rejection of the standard model and this clear identification of systems with exogenous objectives that are sort of singular and lack context and nuance. That when these things optimize for their objectives, they run over a ton of other things that we care about. And so we have to shift from this understanding where the objective is something inside of the exogenous system to something that the system is uncertain about and which actually exists inside of the person.
And I think the last thing that I sort of saw was for people who are not AI researchers, it says, here's this AI alignment problem. It is deeply interdependent and difficult. It requires economists and sociologists and moral philosophers. And for this reason too, it is important for you to join in to help. Do you have anything here you'd like to hit on or expand on or anything I might've gotten wrong?
Stuart: I think that's basically right. One thing that I probably should clarify, and it comes maybe from the phrase value alignment. The goal is not to build machines whose values are identical to those of humans. In other words, it's not to just put in the right objective because I actually believe that that's just fundamentally impossible to do that. Partly because humans actually don't know their own preference structure. There's lots of things that we might have a future positive or a negative reaction to that we don't yet know, lots of foods that we haven't yet tried. And in the book I give the example of the durian fruit, which some people really love and some people find utterly disgusting, and I don't know which I am because I've never tried it. So I'm genuinely uncertain about my own preference structure.
It's really not going to be possible for machines to be built with the right objective built in. They have to know that they don't know what the objective is. And it's that uncertainty that creates this deferential behavior. It becomes rational for that machine to ask permission and to allow itself to be switched off, which as I said, are things that a standard model machine would never do.
The reason why psychology, economics, moral philosophy become absolutely central, is that these fields have studied questions of human preferences, human motivation, and also the fundamental question which machines are going to face, of how do you act on behalf of more than one person? The version of the problem where there's one machine and one human is relatively constrained and relatively straightforward to solve, but when you get one machine and many humans or many machines and many humans, then all kinds of complications come in, which social scientists have studied for centuries. That's why they do it, because there's more than one person.
And psychology comes in because the process whereby the machine is going to learn about human preferences requires that there be some connection between those preferences and the behavior that humans exhibit, because the inverse reinforcement learning process involves observing the behavior and figuring out what are the underlying preferences that would explain that behavior, and then how can I help the human with those preferences.
Humans, surprise, surprise, are not perfectly rational. If they were perfectly rational, we wouldn't need to worry about psychology; we would do all this just with mathematics. But the connection between human preferences and human behavior is extremely complex. It's mediated by our whole cognitive structure, and is subject to lots of deviations from perfect rationality. One of the deviations is that we are simply unable, despite our best efforts, to calculate what is the right thing to do given our preferences.
Lee Sedol, I'm pretty sure wanted to win the games of Go that he was playing against AlphaGo, but he wasn't able to, because he couldn't calculate the winning move. And so if you observe his behavior and you assume that he's perfectly rational, the only explanation is that he wanted to lose, because that's what he did. He made losing moves. But actually that would be obviously a mistake.
So we have to interpret his behavior in the light of his cognitive limitations. That becomes then a matter of empirical psychology. What are the cognitive limitations of humans, and how do they manifest themselves in the kind of imperfect decisions that we make? And then there's other deviations from rationality. We're myopic, we suffer from weakness of will. We know that we ought to do this, that this is the right thing to do, but we do something else. And we're emotional. We do things driven by our emotional subsystems, when we lose our temper for example, that we later regret and say, "I wish I hadn't done that."
 All of this is really important for us to understand going forward, if we want to build machines that can accurately interpret human behavior as evidence for underlying human preferences.
Lucas: You've touched on inverse reinforcement learning in terms of human behavior. Stuart Armstrong was on the other week, and I believe his claim was that you can't infer anything about behavior without making assumptions about rationality and vice versa. So there's sort of an incompleteness there. I'm just pushing here and wondering more about the value of human speech, about what our revealed preferences might be, how this fits in with your book and narrative, as well as furthering neuroscience and psychology, and how all of these things can decrease uncertainty over human preferences for the AI.
Stuart: That's a complicated set of questions. I agree with Stuart Armstrong that humans are not perfectly rational. I've in fact written an entire book about that. But I don't agree that it's fundamentally impossible to recover information about preferences from human behavior. Let me give the kind of straw man argument. So let's take Gary Kasparov: chess player, was world champion in the 1990s, some people would argue the strongest chess player in history. You might think it's obvious that he wanted to win the games that he played. And when he did win, he was smiling, jumping up and down, shaking his fists in triumph. And when he lost, he behaved in a very depressed way, he was angry with himself and so on.
Now it's entirely possible logically that in fact he wanted to lose every single game that he played, but his decision making was so far from rational that even though he wanted to lose, he kept playing the best possible move. So he's got this completely reversed set of goals and a completely reversed decision making process. So it looks on the outside as if he's trying to win and he's happy when he wins. But in fact, he's trying to lose and he's unhappy when he wins, but his attempt to appear unhappy again is reversed. So it looks on the outside like he's really happy because he keeps doing the wrong things, so to speak.
This is an old idea in philosophy. Donald Davidson calls it radical interpretation: that from the outside, you can sort of flip all the bits and come up with an explanation that's sort the complete reverse of what any reasonable person would think the explanation to be. The problem with that approach is that it then takes away the meaning of the word "preference" altogether. For example, let's take the situation where Kasparov can checkmate his opponent in one move, and it's blatantly obvious and in fact, he's taken a whole sequence of moves to get to that situation.
If in all such cases where there's an obvious way to achieve the objective, he simply does something different, in other words, let's say he resigns, so whenever he's in a position with an obvious immediate win, he instantly resigns, then in what sense is it meaningful to say that Kasparov actually wants to win the game if he always resigns whenever he has a chance of winning?
You simply vitiate the entire meaning of the word "preference". It's just not correct to say that a person who always resigns whenever they have a chance of winning really wants to win games. You can then kind of work back from there. So by observing human behavior in situations where the decision is kind of an obvious one that doesn't require a huge amount of calculation, then it's reasonable to assume that the preferences are the ones that they reveal by choosing the obvious action. If you offer someone a lump of coal or a $1,000 bill and they choose a $1,000 bill, it's unreasonable to say, "Oh, they really prefer the lump of coal, but they're just really stupid, so they keep choosing the $1,000 dollar bill." That would just be daft. So in fact it's quite natural that we're able to gradually infer the preferences of imperfect entities, but we have to make some assumptions that we might call minimal rationality, which is that in cases where the choice is obvious, people will generally tend to make the obvious choice.
Lucas: I want to be careful here about not misrepresenting any of Stuart Armstrong's ideas. I think this is in relation to the work Occam's Razor is Insufficient to Infer the Preferences of Irrational Agents, if you're familiar with that?
Stuart: Yeah.
Lucas: So then everything you said still suffices. Is that the case?
Stuart: I don't think we radically disagree. I think maybe it's a matter of emphasis. How important is it to observe the fact that there is this possibility of radical interpretation? It doesn't worry me. Maybe it worries him, but it doesn't worry me because we do a reasonably good job of inferring each other's preferences all the time by just ascribing at least a minimum amount of rationality in human decision making behavior.
This is why economists, the way they try to elicit preferences, is by offering you direct choices. They say, "Here's two pizzas. Are you going to have a bubblegum and pineapple pizza, or you can have ham and cheese pizza. Which one would you like?" And if you choose the ham and cheese pizza, they'll infer that you prefer the ham and cheese pizza, and not the bubblegum and pineapple one, as seems pretty reasonable.
There may be real cases where there is genuine ambiguity about what's driving human behavior. I am certainly not pretending that human cognition is no mystery; it still is largely a mystery. And I think for the long term, it's going to be really important to try to unpack some of that mystery. Horribly to me, the biggest deviation from rationality that humans exhibit is the fact that our choices are always made in the context of a whole hierarchy of commitments that effectively put us into what's usually a much, much smaller decision-making situation than the real problem. So the real problem is I'm alive, I'm in this enormous world, I'm going to live for a few more decades hopefully, and then my descendants will live for years after that and lots of other people on the world will live for a long time. So which actions do I do now?
And I could do anything. I could continue talking to you and recording this podcast. I could take out my phone and start trading stocks. I could go out on the street and start protesting climate change. I could set fire to the building and claim the insurance payment, and so on and so forth. I could do a gazillion things. Anything that's logically possible I could do. And I continue to talk in the podcast because I'm existing in this whole network and hierarchy of commitments. I agreed that we would do the podcast, and why did I do that? Well, because you asked me, and because I've written the book and why did I write the book and so on.
So there's a whole nested collection of commitments, and we do that because otherwise we couldn't possibly manage to behave successfully in the real world at all. The real decision problem is not, what do I say next in this podcast? It's what motor control commands do I send to my 600 odd muscles in order to optimize my payoff for the rest of time until the heat death of the universe? And that's completely and utterly impossible to figure out.
I always, and we always, exist within what I think Savage called a small world decision problem. We are aware only of a small number of options. So if you want to understand human behavior, you have to understand what are the commitments and what is the hierarchy of activities in which that human is engaged. Because otherwise you might be wondering, well why isn't Stuart taking out his phone and trading stocks? But that would be a silly thing to wonder. It's reasonable to ask, well why is he answering the question that way and not the other way?
Lucas: And so "AI, please fetch the coffee," also exists in such a hierarchy. And without the hierarchy, the request is missing much of the meaning that is required for the AI to successfully do the thing. So it's like an inevitability that this hierarchy is required to do things that are meaningful for people.
Stuart: Yeah, I think that's right. Requests are a very interesting special case of behavior, right? They're just another kind of behavior. But up to now, we've interpreted them as defining the objective for the machine, which is clearly not the case. And people have recognized this for a long time. For example, my late colleague Bob Wilensky had a project called the Unix Consultant, which was a natural language system, and it was actually built as an agent, that would help you with Unix stuff, so managing files on your desktop and so on. You could ask it questions like, "Could you make some more space on my disk?", and the system needs to know that RM*, which means "remove all files", is probably not the right thing to do, that this request to make space on the disk is actually part of a larger plan that the user might have. And for that plan, most of the other files are required.
So a more appropriate response would be, "I found these backup files that have already been deleted. Should I empty them from the trash?", or whatever it might be. So in almost no circumstances would a request be taken literally as defining the sole objective. If you asked for a cup of coffee, what happens if there's no coffee? Perhaps it's reasonable to bring a cup of tea or "Would you like a can of Coke instead?", and not to ... I think in the book I had the example that you stop at a gas station in the middle of the desert, 250 miles from the nearest town and they haven't got any coffee. The right thing to do is not to trundle off across the desert and come back 10 days later with coffee from a nearby town. But instead to ask, well, "There isn't any coffee. Would you like some tea or some Coca-Cola instead?"
 This is very natural for humans and in philosophy of language, my other late colleague Paul Grice, was famous for pointing out that many statements, questions, requests, commands in language have this characteristic that they don't really mean what they say. I mean, we all understand if someone says, "Can you pass the salt?", the correct answer is not, "Yes, I am physically able to pass the salt." He became an adjective, right? So we talk about Gricean analysis, where you don't take the meaning literally, but you look at the context in which it was said and the motivations of the speaker and so on to infer what is a reasonable course of action when you hear that request.
Lucas: You've done a wonderful job so far painting the picture of the AI alignment problem and the solution for which you offer, at least the pivoting which you'd like the community to take. So for laypersons who might not be involved or experts in AI research, plus the AI alignment community, plus potential researchers who might be brought in by this process or book, plus policymakers who may also listen to it, what's at stake here? Why does this matter?
Stuart: I think AI, for most of its history, has been an interesting curiosity. It's a fascinating problem, but as a technology it was woefully lacking. And it has found various niches where it's useful, even before the current incarnation in terms of deep learning. But if we assume that progress will continue and that we will create machines with general purpose intelligence, that would be roughly speaking, the biggest event in human history.
History, our civilization, is just a consequence of the fact that we have intelligence, and if we had a lot more, it would be a radical step change in our civilization. If these were possible at all, it would enable other inventions that people have talked about as possibly the biggest event in human history, for example, creating the ability for people to live forever or much, much longer life span than we currently have, or creating the possibility for people to travel faster than light so that we could colonize the universe.
If those are possible, then they're going to be much more possible with the help of AI. If there's a solution to climate change, it's going to be much more possible to solve climate change with the help of AI. It's this fact that AI in the form of general purpose intelligence systems is this kind of über technology that makes it such a powerful development if and when it happens. So the upside is enormous. And then the downside is also enormous, because if you build things that are more intelligent than you, then you face this problem. You've made something that's much more powerful than human beings, but somehow you've got to make sure that it never actually has any power. And that's not completely obvious how to do that.
The last part of the book is a proposal for how we could do that, how you could change this notion of what we mean by an intelligent system so that rather than copying this sort of abstract human model, this idea of rationality, of decision making in the interest, in the pursuit of one's own objectives, we have this other kind of system, this sort of coupled binary system where the machine is necessarily acting in the service of human preferences.
If we can do that, then we can reap the benefits of arbitrarily intelligent AI. Then as I said, the upside would be enormous. If we can't do that, if we can't solve this problem, then there are really two possibilities. One is that we need to curtail the development of artificial intelligence and for all the reasons that I just mentioned, it's going to be very hard because the upside incentive is so enormous. It would be very hard to stop research and development in AI.
The third alternative is that we create general purpose, superhuman intelligent machines and we lose control of them, and they're pursuing objectives that are ultimately mistaken objectives. There's tons of science fiction stories that tell you what happens next, and none of them are desirable futures for the human race.
Lucas: Can you expand upon what you mean by if we're successful in the control/alignment problem, what "tremendous" actually means? What actually are the conclusions or what is borne out of the process of generating an aligned super intelligence from that point on until heat death or whatever else?
Stuart: Assuming that we have a general purpose intelligence that is beneficial to humans, then you can think about it in two ways. I already mentioned the possibility that you'd be able to use that capability to solve problems that we find very difficult, such as eternal life, curing disease, solving the problem of climate change, solving the problem of faster than light travel and so on. You might think of these as sort of the science fiction-y upside benefits. But just in practical terms, when you think about the quality of life for most people on earth, let's say it leaves something to be desired. And you say, "Okay, would be a reasonable aspiration?", and put it somewhere like the 90th percentile in the US. That would mean a ten-fold increase in GDP for the world if you brought everyone on earth up to what we call a reasonably nice standard of living by Western standards.
General purpose AI can do that in the following way, without all these science fiction inventions and so on. So just deploying the technologies and materials and processes that we already have in ways that are much, much more efficient and obviously much, much less labor intensive.
The reason that things cost a lot and the reason that people in poor countries can't afford them ... They can't build bridges or lay railroad tracks or build hospitals because they're really, really expensive and they haven't yet developed the productive capacities to produce goods that could pay for all those things. The reason things are really, really expensive is because they have a very long chain of production in which human effort is involved at every stage. The money all goes to pay all those humans, whether it's the scientists and engineers who designed the MRI machine or the people who worked on the production line or the people who worked mining the metals that go into making the MRI machine.
All the money is really paying for human time. If machines are doing every stage of the production process, then you take all of those costs out, and to some extent it becomes like a digital newspaper, in the sense that you can have as much of it as you want. It's almost free to make new copies of a digital newspaper, and it would become almost free to produce the material goods and services that constitute a good quality of life for people. And at that point, arguing about who has more of it is like arguing about who has more digital copies of the newspaper. It becomes sort of pointless.
That has two benefits. One is everyone is relatively much better off, assuming that we can get politics and economics out of the way, and also there's then much less incentive for people to go around starting wars and killing each other, because there isn't this struggle which has sort of characterized most of human history. The struggle for power, wealth and access to resources and so on. There are other reasons people kill each other, religion being one of them, but it certainly I think would help if this source of competition and warfare were removed.
Lucas: These are very important short-term considerations and benefits from getting this control problem and this alignment problem correct. One thing that the superintelligence will hopefully also do is reduce existential risk to zero, right? And so if existential risk is reduced to zero, then basically what happens is the entire cosmic endowment, some hundreds of thousands of galaxies, become unlocked to us. Perhaps some fraction of it would have to be explored first in order to ensure existential risk is pretty close to zero. I find your arguments are pragmatic and helpful for the common person about why this is important.
For me personally, and why I'm passionate about AI alignment and existential risk issues, is that the reduction of existential risk to zero and having an aligned intelligence that's capable of authentically spreading through the cosmic endowment, to me seems to potentially unlock a kind of transcendent object at the end of time, ultimately influenced by what we do here and now, which is directed and created by coming to better know what is good, and spreading that.
What I find so beautiful and important and meaningful about this problem in particular, and why anyone who's reading your book, why it's so important for them for core reading, and reading for laypersons, for computer scientists, for just everyone, is that if we get this right, this universe can be maybe one of the universes and perhaps the multiverse, where something like the most beautiful thing physically possible could be made by us within the laws of physics. And that to me is extremely awe-inspiring.
Stuart: I think that human beings being the way they are, will probably find more ways to get it wrong. We'll need more solutions for those problems and perhaps AI will help us solve other existential risks, and perhaps it won't. The control problem I think is very important. There are a couple of other issues that I think we still need to be concerned with. Well, I don’t think we need to be concerned with all of them, but a couple of issues that I haven't begun to address or solve ... One of those is obviously the problem of misuse, that we may find ways to build beneficial AI systems that remain under control in a mathematically guaranteed way. And that's great. But the problem of making sure that only those kinds of systems are ever built and used, that's a different problem. That's a problem about human motivation and human behavior, which I don't really have a good solution to. It's sort of like the malware problem, except much, much, much, much worse. If we do go ahead developing general purpose intelligence systems that are beneficial and so on, then, parts of that technology, the general purpose intelligent capabilities could be put into systems that are not beneficial as it were, that don't have a safety catch. And that misuse problem. If you look at how well we're doing with malware, you'd have to say, more work needs to be done. We're kind of totally failing to control malware and the ability of people to inflict damage on others by uncontrolled software that's getting worse. We need an international response and a policing response. Some people argue that, oh, it's fine. The super intelligent AI that we build will make sure that other nefarious development efforts are nipped in the bud.
This doesn't make me particularly confident. So I think that's an issue. The third issue is, shall we say enfeeblement. This notion that if we develop machines that are capable of running every aspect of our civilization, then that changes the dynamic that's been in place since the beginning of human history or pre history. Which is that for our civilization to continue, we have had to pass on our knowledge and our skills to the next generation. That people have to learn what it is that the human race knows over and over again in every generation, just to keep things going. And if you add it all up, if you look, there's about a hundred odd billion people who've ever lived and they spend each about 10 years learning stuff on average. So that's a trillion person years of teaching and learning to keep our civilization going. And there's a very good reason why we've done that because without it, things would fall apart very quickly.
But that's going to change. Now. We don't have to put it into the heads of the next generation of humans. We can put it into the heads of the machines and they can take care of the civilization. And then you get this almost irreversible process of enfeeblement, where humans no longer know how their own civilization functions. They lose knowledge of science, of engineering, even of the humanities of literature. If machines are writing books and producing movies, then we don't even need to learn that. You see this in E. M. Forster's story, The Machine Stops from 1909 which is a very prescient story about a civilization that becomes completely dependent on its own machines. Or if you like something more recent in WALL-E the human race is on a, sort of a cruise ship in space and they all become obese and stupid because the machines look after everything and all they do is consume and enjoy. And that's not a future that I would want for the human race.
And arguably the machines should say, this is not the future you want, tie your shoelaces, but we are these, shortsighted. We may effectively override what the machines are telling us and say, "No, no, you have to tie my shoe laces for me." So I think this is a problem that we have to think about. Again, this is a problem for infinity. Once you turn things over to the machines, it's practically impossible, I think, to reverse that process, we have to keep our own human civilization going in perpetuity and that requires a kind of a cultural process that I don't yet understand how it would work, exactly.
Because the effort involved in learning, let's say going to medical school, it's 15 years of school and then college and then medical school and then residency. It's a huge effort. It's a huge investment and at some point the incentive to undergo that process will disappear. And so something else other than... So at the moment it's partly money, partly prestige, partly a desire to be someone who is in a position to help others. So somehow we got to make our culture capable of maintaining that process indefinitely when many of the incentive structures that have kept it in place go away.
Lucas: This makes me wonder and think about how from an evolutionary cosmological perspective, how this sort of transition from humans being the most intelligent form of life on this planet to machine intelligence being the most intelligent form of life. How that plays out in the very longterm. If we can do thought experiments where we imagine if monkeys had been actually creating humans and then had created humans, what the role of the monkey would still be.
Stuart: Yep. But we should not be creating the machine analog of humans, I.E. autonomous entities pursuing their own objectives. So we've pursued our objectives pretty much at the expense of the monkeys and the gorillas and we should not be producing machines that play an analogous role. That would be a really dumb thing to do.
Lucas: That's an interesting comparison because the objectives of the human are exogenous to the monkey and that's the key issue that you point out. If the monkey had been clever and had been able to control evolution, then they would have set the human uncertain as to the monkey's preferences and then had him optimize those.
Stuart: Yeah, I mean they could imagine creating a race of humans that were intelligent but completely subservient to the interests of the monkeys. Assuming that they solved the enfeeblement problem and the misuse problem, then they'd be pretty happy with the way things turned out. I don't see any real alternative. So Samuel Butler in 1863 wrote a book about a society that faces the problem of superintelligent machines and they take the other solution, which is actually to stop. They see no alternative but to just ban the construction of intelligent machines altogether. In fact, they ban all machines and in Frank Herbert's Dune, the same thing. They have a catastrophic war in which humanity just survives in its conflict with intelligent machines. And then from then on, all intelligent machines, in fact, all computers are banned altogether. I can't see that that's a plausible direction, but it could be that we decide at some point that we cannot solve the control problem or we can't solve the misuse problem or we can't solve the enfeeblement problem.
And we decided that it's in our best interests to just not go down this path at all. To me that just doesn't feel like a possible direction. Things can change if we start to see bigger catastrophes. I think the click through catastrophe is already pretty big and it results from very, very simple minded algorithms that know nothing about human cognition or politics or anything else. They're not even explicitly trying to manipulate us. It's just, that's what the code does in a very simple minded way. So we could imagine bigger catastrophes happening that we survived by the skin of our teeth as happened in Dune for example. And then that would change the way people think about the problem. And we see this over and over again with nuclear power, with fossil fuels and so on that by large technology is always seen as beneficial and more technology is therefore more beneficial.
And we pushed your head often ignoring the people who say "But, but, but what about this drawback? What about this drawback?" And maybe that starting to change with respect to fossil fuels. Several countries have now decided since Chernobyl and Fukushima to ban nuclear power, the EU has much stronger restriction on genetically modified foods than a lot of other countries, so there are pockets where people have pushed back against technological progress and said, "No, not all technology is good and not all uses of technology are good and so we need to exercise a choice." But the benefits of AI are potentially so enormous. It's going to take a lot to undo this forward progress.
Lucas: Yeah, absolutely. Whatever results from earth originating intelligent life at the end of time, that thing is up to us to create. I'm quoting you here, you say, "A compassionate and jubilant use of humanity's cosmic endowment sounds wonderful, but we also have to reckon with the rapid rate of innovation in the malfeasance sector, ill intentioned people are thinking up new ways to misuse AI so quickly that this chapter is likely to be outdated even before an attains printed form. Think of it not as depressing reading. However, but as a call to act before it's too late."
Thinking about this and everything you just touched on. There's obviously a ton for us to get right here that needs to be gotten right and it's a question and problem for everyone in the human species to have a voice in.
Stuart: Yeah. I think we really need to start considering the possibility that there ought to be a law against it. For a long time the IT industry almost uniquely has operated in a completely unregulated way. The car industry for example, cars have to follow various kinds of design and safety rules. You have to have headlights and turn signals and brakes and so on. A car that's designed in an unsafe way gets taken off the market, but software can do pretty much whatever it wants.
Every license agreement that you sign whenever you buy or use software tells you that it doesn't matter what their software does. The manufacturer is not responsible for anything and so on. And I think it's a good idea to actually take legislative steps, regulatory steps just to get comfortable with the idea that yes, I see we maybe do need regulation. San Francisco, for example, has banned the use of facial recognition in public or for policing. California has a ban on the impersonation of human beings by AI systems. I think that ban should be pretty much universal. But in California it's primary area of applicability is in persuading people to vote in any particular direction in an election. So it's a fairly narrow limitation. But when you think about it, why would you want to allow AI systems to impersonate human beings so that in other words, the human who's in conversation, believes that if they're talking to another human being, that they owe that other human being a whole raft of respect, politeness, all kinds of obligations that are involved in interacting with other humans.
But you don't owe any of those things to an AI system. And so why should we allow people to effectively defraud humans by convincing them that in fact they're engaged with another human when they aren't? So I think it would be a good idea to just start things off with some basic common sense rules. I think the GDPR rule that says that you can't use an algorithm to make a decision that has a significant legal effect on a person. So you can't put them in jail simply as a result of an algorithm, for example. You can't fire them from a job simply as a result of an algorithm. You can use the algorithm to advise, but a human has to be involved in the decision and the person has to be able to query the decision and ask for the reasons and in some sense have a right of appeal.
So these are common sense rules that almost everyone would agree with. And yet certainly in the U.S., there's reluctance to put them into effect. And I think going forward, if we want to have safe AI systems, there's at least going to be a role for regulations. There should also be standards as in I triple E standards. There should also be professional codes of conduct. People should be trained in how to recognize potentially unsafe designs for AI systems, but there should, I think, be a role for regulation where at some point you would say, if you want to put an AI system on the internet, for example, just as if you want to put software into the app store, it has to pass a whole bunch of checks to make sure that it's safe to make sure that it won't wreak havoc. So, we better start thinking about that. I don't know yet what that regulation should say, but we shouldn't be in principle opposed to the idea that such regulations might exist at some point.
Lucas: I basically agree that these regulations should be implemented today, but they seem pretty temporary or transient as the uncertainty in the AI system for the humans’ objective function or utility function decreases. So they become more certain about what we want. At some point it becomes unethical to have human beings governing these processes instead of AI systems. Right? So if we have timelines from AI researchers that range from 50 to a hundred years for AGI, we could potentially see laws and regulations like this go up in the next five to 10 and then disappear again somewhere within the next hundred to 150 years max.
Stuart: That's an interesting viewpoint. And I think we have to be a little careful because autonomy is part of our preference structure. So although one might say, okay, know who gets to run the government? Well self, evidently it's possible that machines could do a better job than the humans we currently have that would be better only in a narrow sense that maybe it would reduce crime, maybe it would increase economic output, we'd have better health outcomes, people would be more educated than they would with humans making those decisions, but there would be a dramatic loss in autonomy. And autonomy is a significant part of our preference structure. And so it isn't necessarily the case that the right solution is that machines should be running the government. And this is something that the machines themselves will presumably recognize and this is the reason why parents at some point tell the child, "No, you have to tie your own shoe laces." Because they want the child to develop autonomy.
The same thing will be true. The machines want humans to retain autonomy. As I said earlier, with respect to enfeeblement, right? It's this conflict between our longterm best interest and our short term-ism in the choices that we tend to make. It's always easier to say, "Oh no, I can't be bothered at the time I shoelaces. Please could you do it?" But if you keep doing that, then the longterm consequences are bad. We have to understand how autonomy, which includes machines not making decisions, folds into our overall preference structure. And up to now there hasn't been much of a choice, at least in the global sense. Of course it's been humans making the decisions, although within any local context it's only a subset of humans who are making the decisions and a lot of other people don't have as much autonomy. To me, I think autonomy is a really important currency that to the extent possible, everyone should have as much of it as possible.
Lucas: I think you really hit the nail on the head. The problem is where autonomy fits in the hierarchy of our preferences and meta preferences. For me, it seems more instrumental than being an end goal in itself. Now this is an empirical question across all people where autonomy fits in their preference hierarchies and whether it's like a terminal value or not, and whether under reflection and self idealization, our preferences distill into something else or not. Autonomy could possibly but not necessarily be an end goal. In so far as that it simply provides utility for all of our other goals. Because without autonomy we can't act on what we think will best optimize our own preferences and end values. So definitely a lot of questions there. The structure of our preference hierarchy will certainly dictate, it seems, the longterm outcome of humanity and how enfeeblement unfolds.
Stuart: The danger would be that we misunderstand the entire nature of the human preference hierarchy. So sociologists and others have talked about the hierarchy of human needs in terms of food, shelter, physical security and so on. But they've always kind of assumed that you are a human being and therefore you're the one deciding stuff. And so they tend not to think so much about fundamental properties of the ability to make your own choices for good or ill. And science fiction writers have had a field day with this. Pointing out that machines that do what you want are potentially disastrous because you lose the freedom of choice.
One could imagine that if we formulate things not quite right and the effect of the algorithms that we build is to make machines that don't value autonomy in the right way or don't have it folded into the overall preference structure in the right way, that we could end up with a subtle but gradual and very serious loss of autonomy in a way that we may not even notice as it happens. Like the slow boiling frog. If we could look ahead a hundred years and see how things turn out, he would say, "Oh my goodness, that is a terrible mistake". We're going to make sure that that doesn't happen. So I think we need to be pretty careful. And again this is where we probably need the help of philosophers to make sure that we keep things straight and understand how these things fit together.
Lucas: Right, so seems like we simply don't understand ourselves. We don't know the hierarchy of our preferences. We don't really know what preferences exactly are. Stuart Armstrong talks about how we haven't figured out the symbol grounding problem. So there are issues with even understanding how preferences relate to one another ultimately and how the meaning there is born. And we're building AI systems which will be more capable than us. Perhaps they will be conscious. You have a short subchapter I believe on that or at least on how you're not going to talk about consciousness.
Stuart: Yeah. I have a paragraph saying I have nothing to say.
Lucas: So potentially these things will also be moral patients and we don't know how to get them to do the things that we're not entirely sure that we want them to do. So how would you differentiate this book from Superintelligence or Life 3.0 or other books on the AI alignment problem. And superintelligence in this space.
Stuart: I think the two major differences are one, I believe that to understand this whole set of issues or even just to understand what's happening with AI and what's going to happen, you have to understand something about AI. And I think that Superintelligence and Life 3.0 are to some extent, easier to grasp. If you already understand quite a bit about AI. And if you don't, then it's quite difficult to get as much out of those books as is in there. I think they are full of interesting points and ideas, but those points and ideas are easier to get out if you understand AI. So I wanted people to understand AI, understand, not just it as a technology, right? You could talk about how deep learning works, but that's not the point. The point is really what is intelligence and how have we taken that qualitative understanding of what that means and turned it into this technical discipline where the standard model is machines that achieve fixed objectives.
And then the second major difference is that I'm proposing a solution for at least one of the big failure modes of AI. And as far as I can tell, that solution, I mean, it's sort of mentioned in some ways in Superintelligence, I think the phrase there is normative uncertainty, but it has a slightly different connotation. And partly that's because this approach of inverse reinforcement learning is something that we've actually worked on at Berkeley for a little over 20 years. It wasn't invented for this purpose, but it happens to fit this purpose and then the approach of how we solve this problem is fleshed out in terms of understanding that it's this coupled system between the human that has the preferences and the machine that's trying to satisfy those preferences and doesn't know what they are. So I think that part is different. That's not really present in those other two books.
It certainly shares, I think the desire to convince people that this is a serious issue. I think both Superintelligence and Life 3.0 do a good job of that Superintelligence is sort of a bit more depressing. It's such a good job of convincing you that things can go South, so many ways that you almost despair. Life 3.0 is a bit more cheerful. And also I think Life 3.0 does a good job of asking you what you want the outcome to be. And obviously you don't want it to be catastrophic outcomes where we're all placed in concrete coffins with heroin drips as Stuart Armstrong likes to put it.
But there are lots of other outcomes which are the ones you want. So I think that's an interesting part of that book. And of course Max Tegmark, the author of Life 3.0 is a physicist. So he has lots of amazing stuff about the technologies of the future, which I don't have so much. So those are the main differences. I think that wanting to convey the essence of intelligence, how that notion has developed, how is it really an integral part of our whole intellectual tradition and our technological society and how that model is fundamentally wrong and what's the new model that we have to replace it with.
Lucas: Yeah, absolutely. I feel that you help to clarify intelligence for me, the history of intelligence from evolution up until modern computer science problems. I think that you really set the AI alignment problem up well resulting from there being intelligences and multi-agent scenarios, trying to do different things, and then you suggest a solution, which we've discussed here already. So thanks so much for coming on the podcast, Stuart, your book is set for release on October 8th?
Stuart: That's correct.
Lucas: Great. We'll include links for that in the description. Thanks so much for coming on.
 If you enjoyed this podcast, please subscribe. Give it a like or share it on your preferred social media platform. We'll be back again soon with another episode in the AI alignment series.
Related episodes
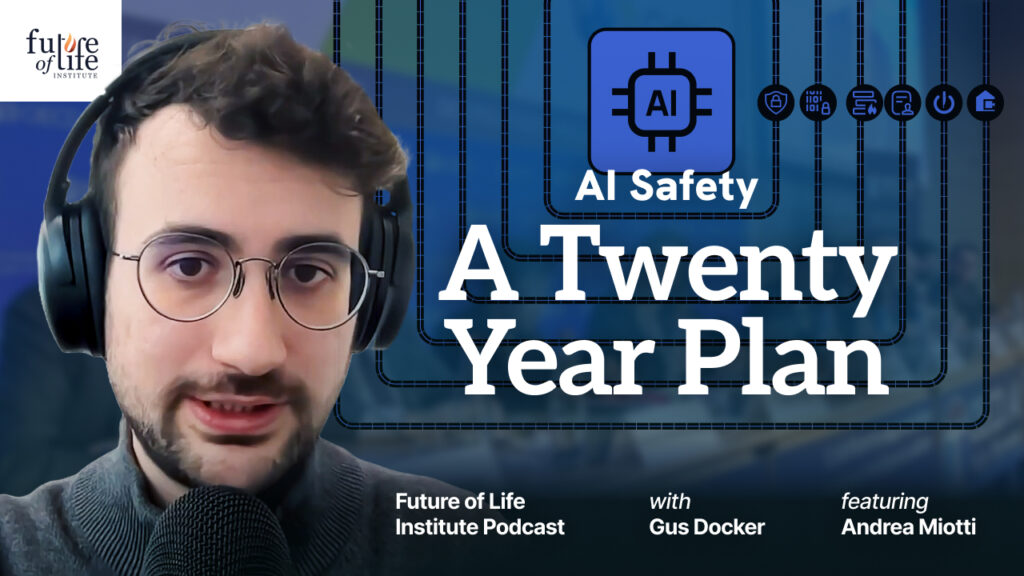
Andrea Miotti on a Narrow Path to Safe, Transformative AI
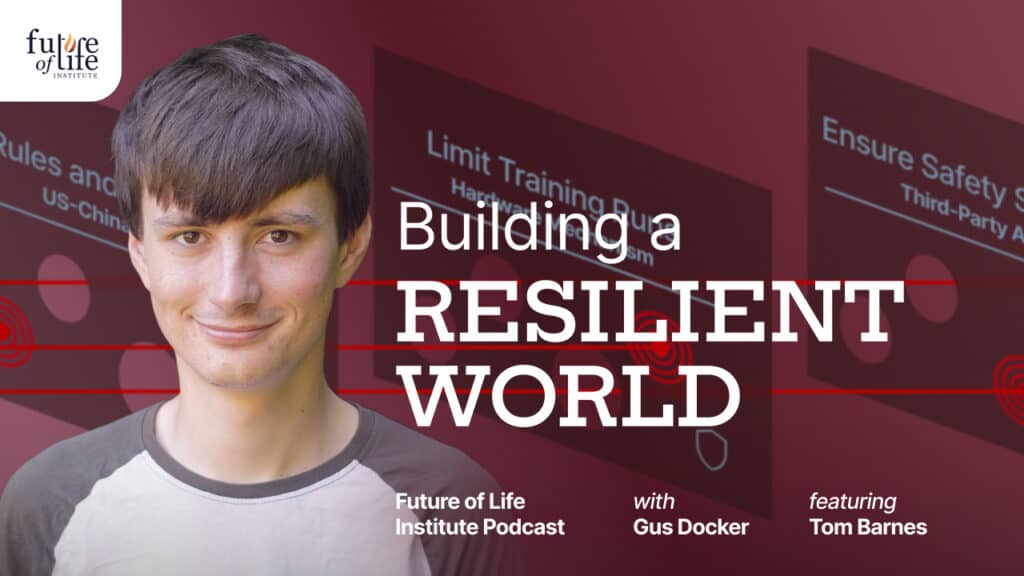