AI Alignment Podcast: Synthesizing a human’s preferences into a utility function with Stuart Armstrong
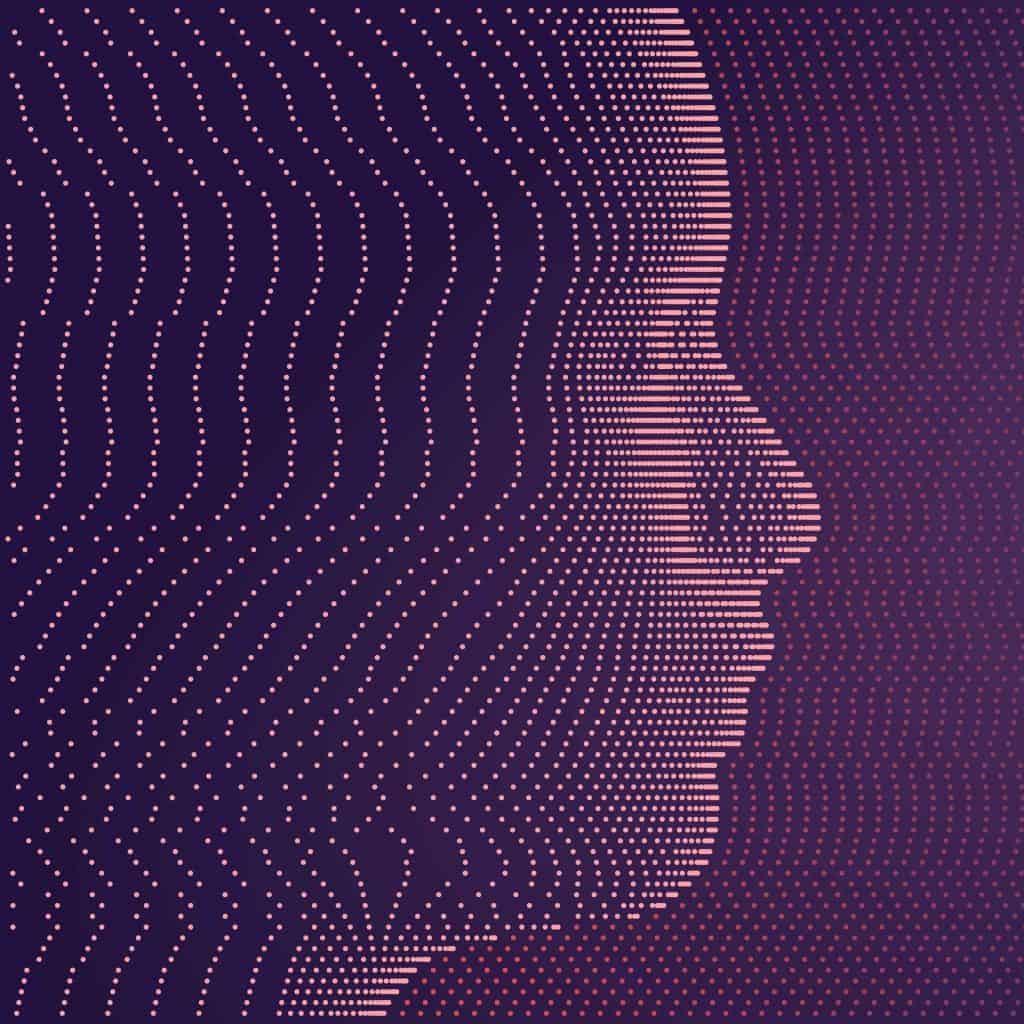
In his Research Agenda v0.9: Synthesizing a human's preferences into a utility function, Stuart Armstrong develops an approach for generating friendly artificial intelligence. His alignment proposal can broadly be understood as a kind of inverse reinforcement learning where most of the task of inferring human preferences is left to the AI itself. It's up to us to build the correct assumptions, definitions, preference learning methodology, and synthesis process into the AI system such that it will be able to meaningfully learn human preferences and synthesize them into an adequate utility function. In order to get this all right, his agenda looks at how to understand and identify human partial preferences, how to ultimately synthesize these learned preferences into an "adequate" utility function, the practicalities of developing and estimating the human utility function, and how this agenda can assist in other methods of AI alignment.
Topics discussed in this episode include:
- The core aspects and ideas of Stuart's research agenda
- Human values being changeable, manipulable, contradictory, and underdefined
- This research agenda in the context of the broader AI alignment landscape
- What the proposed synthesis process looks like
- How to identify human partial preferences
- Why a utility function anyway?
- Idealization and reflective equilibrium
- Open questions and potential problem areas
Last chance to take a short (4 minute) survey to share your feedback about the podcast.
Key points from Stuart:
- "There are two core parts to this research project essentially. The first part is to identify the humans' internal models, figure out what they are, how we use them and how we can get an AI to realize what's going on. So those give us the sort of partial preferences, the pieces from which we build our general preferences. The second part is to then knit all these pieces together into an overall preference for any given individual in a way that works reasonably well and respects as much as possible the person's different preferences, meta-preferences and so on. The second part of the project is the one that people tend to have strong opinions about because they can see how it works and how the building blocks might fit together and how they'd prefer that it would be fit together in different ways and so on but in essence, the first part is the most important because that fundamentally defines the pieces of what human preferences are."
- "So, when I said that human values are contradictory, changeable, manipulable and underdefined, I was saying that the first three are relatively easy to deal with but that the last one is not. Most of the time, people have not considered the whole of the situation that they or the world or whatever is confronted with. No situation is exactly analogous to another, so you have to try and fit it in to different categories. So if someone dubious gets elected in a country and starts doing very authoritarian things, does this fit in the tyranny box which should be resisted or does this fit in the normal process of democracy box in which case it should be endured and dealt with through democratic means. What'll happen is generally that it'll have features of both, so it might not fit comfortably in either box and then there's a wide variety for someone to be hypocritical or to choose one side or the other but the reason that there's such a wide variety of possibilities is because this is a situation that has not been exactly confronted before so people don't actually have preferences here. They don't have a partial preference over this situation because it's not one that they've ever considered... I've actually argued at some point in the research agenda that this is an argument for insuring that we don't go too far from the human baseline normal into exotic things where our preferences are not well-defined because in these areas, the chance that there is a large negative seems higher than the chance that there's a large positive... So, when I say not go too far, I don't mean not embrace a hugely transformative future. I'm saying not embrace a hugely transformative future where our moral categories start breaking down."
- "One of the reasons to look for a utility function is to look for something stable that doesn't change over time and there is evidence that consistency requirements will push any form of preference function towards a utility function and that if you don't have a utility function, you just lose value. So, the desire to put this into a utility function is not out of an admiration for utility functions per se but our desire to get something that won't further change or won't further drift in a direction that we can't control and have no idea about. The other reason is that as we start to control our own preferences better and have a better ability to manipulate our own minds, we are going to be pushing ourselves towards utility functions because of the same pressures of basically not losing value pointlessly."
- "Reflective equilibrium is basically you refine your own preferences, make them more consistent, apply them to yourself until you've reached a moment where your meta-preferences and your preferences are all smoothly aligned with each other. What I'm doing is a much more messy synthesis process and I'm doing it in order to preserve as much as possible of the actual human preferences. It is very easy to reach reflective equilibrium by just, for instance, having completely flat preferences or very simple preferences, these tend to be very reflectively in equilibrium with itself and pushing towards this thing is a push towards, in my view, excessive simplicity and the great risk of losing valuable preferences. The risk of losing valuable preferences seems to me a much higher risk than the gain in terms of simplicity or elegance that you might get. There is no reason that the kludgey human brain and it's mess of preferences should lead to some simple reflective equilibrium. In fact, you could say that this is an argument against reflexive equilibrium because it means that many different starting points, many different minds with very different preferences will lead to similar outcomes which basically means that you're throwing away a lot of the details of your input data."
- "Imagine that we have reached some positive outcome, we have got alignment and we haven't reached it through a single trick and we haven't reached it through the sort of tool AIs or software as a service or those kinds of approaches, we have reached an actual alignment. It, therefore, seems to me all the problems that I've listed or almost all of them will have had to have been solved, therefore, in a sense, much of this research agenda needs to be done directly or indirectly in order to achieve any form of sensible alignment. Now, the term directly or indirectly is doing a lot of the work here but I feel that quite a bit of this will have to be done directly."
Important timestamps:
0:00 Introductions
3:24 A story of evolution (inspiring just-so story)
6:30 How does your “inspiring just-so story” help to inform this research agenda?
8:53 The two core parts to the research agenda
10:00 How this research agenda is contextualized in the AI alignment landscape
12:45 The fundamental ideas behind the research project
15:10 What are partial preferences?
17:50 Why reflexive self-consistency isn’t enough
20:05 How are humans contradictory and how does this affect the difficulty of the agenda?
25:30 Why human values being underdefined presents the greatest challenge
33:55 Expanding on the synthesis process
35:20 How to extract the partial preferences of the person
36:50 Why a utility function?
41:45 Are there alternative goal ordering or action producing methods for agents other than utility functions?
44:40 Extending and normalizing partial preferences and covering the rest of section 2
50:00 Moving into section 3, synthesizing the utility function in practice
52:00 Why this research agenda is helpful for other alignment methodologies
55:50 Limits of the agenda and other problems
58:40 Synthesizing a species wide utility function
1:01:20 Concerns over the alignment methodology containing leaky abstractions
1:06:10 Reflective equilibrium and the agenda not being a philosophical ideal
1:08:10 Can we check the result of the synthesis process?
01:09:55 How did the Mahatma Armstrong idealization process fail?
01:14:40 Any clarifications for the AI alignment community?
Works referenced:
Research Agenda v0.9: Synthesising a human's preferences into a utility function
Some Comments on Stuart Armstrong's "Research Agenda v0.9"
Mahatma Armstrong: CEVed to death
We hope that you will continue to join in the conversations by following us or subscribing to our podcasts on Youtube, Spotify, SoundCloud, iTunes, Google Play, Stitcher, iHeartRadio, or your preferred podcast site/application. You can find all the AI Alignment Podcasts here.
Transcript
Lucas: Hey everyone and welcome back to the AI Alignment Podcast at the Future of Life Institute. I'm Lucas Perry and today we'll be speaking with Stuart Armstrong on his Research Agenda version 0.9: Synthesizing a human's preferences into a utility function. Here Stuart takes us through the fundamental idea behind this research agenda, what this process of synthesizing human preferences into a utility function might look like, key philosophical and empirical insights needed for progress, how human values are changeable, manipulable, under-defined and contradictory, how these facts affect generating an adequate synthesis of human values, where this all fits in the alignment landscape and how it can inform other approaches to aligned AI systems.
If you find this podcast interesting or useful, consider sharing it with friends, on social media platforms, forums or anywhere you think it might be found valuable. I'd also like to put out a final call for this round of SurveyMonkey polling and feedback, so if you have any comments, suggestions or any other thoughts you'd like to share with me about the podcast, potential guests or anything else, feel free to do so through the SurveyMonkey poll link attached to the description of wherever you might find this podcast. I'd love to hear from you. There also seems to be some lack of knowledge regarding the pages that we create for each podcast episode. You can find a link to that in the description as well and it contains a summary of the episode, topics discussed, key points from the guest, important timestamps if you want to skip around, works referenced, as well as a full transcript of the audio in case you prefer reading.
Stuart Armstrong is a researcher at the Future of Humanity Institute who focuses on the safety and possibilities of artificial intelligence, how to define the potential goals of AI and map humanities partially defined values into it and the longterm potential for intelligent life across the reachable universe. He has been working with people at FHI and other organizations such as DeepMind to formalize AI desiderata in general models so the AI designers can include these safety methods in their designs. His collaboration with DeepMind on "Interruptability" has been mentioned in over 100 media articles. Stuart's past research interests include comparing existential risks in general, including their probability and their interactions, anthropic probability, how the fact that we exist affects our probability estimates around that key fact, decision theories that are stable under self-reflection and anthropic considerations, negotiation theory and how to deal with uncertainty about your own preferences, computational biochemistry, fast ligand screening, parabolic geometry and his Oxford DPhil was on the holonomy of projective and conformal Cartan geometries and so without further ado or pretenses that I know anything about the holonomy of projective and conformal Cartan geometries, I give you Stuart Armstrong.
We're here today to discuss your research agenda version 0.9: Synthesizing a human's preferences into a utility function. One wonderful place for us to start here would be with this sort of story of evolution, which you call an inspiring just so story, and so starting this, I think it would be helpful for us contextualizing sort of the place of the human and what the human is as we sort of find ourselves here at the beginning of this value alignment problem. I'll go ahead and read there here for listeners to begin developing a historical context and narrative.
So, I'm quoting you here. You say, “This is the story of how evolution created humans with preferences and what the nature of these preferences are. The story is not true in the sense of accurate. Instead, it is intended to provide some inspiration as to the direction of this research agenda. In the beginning, evolution created instinct driven agents. These agents have no preferences or goals nor do they need any. They were like Q-learning agents. They knew the correct action to take in different circumstances but that was it. Consider baby turtles that walk towards the light upon birth because traditionally, the sea was lighter than the land. Of course, this behavior fails them in the era of artificial lighting but evolution has a tiny bandwidth, acting once per generation, so it created agents capable of planning, of figuring out different approaches rather than having to follow instincts. This was useful especially in varying environments and so evolution off-loaded a lot of it's job onto the planning agents.”
“Of course, to be of any use, the planning agents need to be able to model their environment to some extent or else their plans can't work and had to have preferences or else every plan was as good as another. So, in creating the first planning agents, evolution created the first agents with preferences. Of course, evolution is messy, undirected process, so the process wasn't clean. Planning agents are still riven with instincts and the modeling of the environment is situational, used for when it was needed rather than some consistent whole. Thus, the preferences of these agents were underdefined and some times contradictory. Finally, evolution created agents capable of self-modeling and of modeling other agents in their species. This might have been because of competitive social pressures as agents learned to lie and detect lying. Of course, this being evolution, the self and other modeling took the form of kludges built upon spandrels, built upon kludges and then arrived humans, who developed norms and norm violations.”
“As a side effect of this, we started having higher order preferences as to what norms and preferences should be but instincts and contradictions remained. This is evolution after all, and evolution looked upon this hideous mess and saw that it was good. Good for evolution that is but if we want it to be good for us, we're going to need to straighten out this mess somewhat.” Here we arrive, Stuart, in the human condition after hundreds of millions of years of evolution. So, given the story of human evolution that you've written here, why were you so interested in this story and why were you looking into this mess to better understand AI alignment and development this research agenda?
Stuart: This goes back to a paper that I co-wrote for NuerIPS It basically develops the idea of inverse reinforcement learning or more broadly, can you infer what the preferences of an agent are just by observing their behavior. Humans are not entirely rational, so the question I was looking at is can you simultaneously infer the rationality and the preferences of an agent by observing their behavior. It turns out to be mathematically completely impossible. We can't infer the preferences without making assumptions about the rationality and we can't infer the rationality without making assumptions about the preferences. This is a rigorous result, so my looking at human evolution is to basically get around this result, in a sense, to make the right assumptions so that we can extract actual human preferences since we can't just do it by observing behavior. We need to dig a bit deeper.
Lucas: So, what have you gleaned then from looking at this process of human evolution and seeing into how messy the person is?
Stuart: Well, there's two key insights here. The first is that I located where human preferences reside or where we can assume that human preferences reside and that's in the internal models of the humans, how we model the world, how we judge, that was a good thing or I want that or ooh, I'd be really embarrassed about that, and so human preferences are defined in this project or at least the building blocks of human preferences are defined to be in these internal models that humans have with the labeling of states of outcomes as good or bad. The other point to bring about evolution is that since it's not anything like a clean process, it's not like we have one general model with clearly labeled preferences and then everything else flows from that. It is a mixture of situational models in different circumstances with subtly different things labeled as good or bad. So, as I said to you in preferences are contradictory, changeable, manipulable and underdefined.
So, there are two core parts to this research project essentially. The first part is to identify the humans' internal models, figure out what they are, how we use them and how we can get an AI to realize what's going on. So those give us the sort of partial preferences, the pieces from which we build our general preferences. The second part is to then knit all these pieces together into an overall preference for any given individual in a way that works reasonably well and respects as much as possible the person's different preferences, meta-preferences and so on.
The second part of the project is the one that people tend to have strong opinions about because they can see how it works and how the building blocks might fit together and how they'd prefer that it would be fit together in different ways and so on but in essence, the first part is the most important because that fundamentally defines the pieces of what human preferences are.
Lucas: Before we dive into the specifics of your agenda here, can you contextualize it within evolution of your thought on AI alignment and also how it fits within the broader research landscape?
Stuart: So, this is just my perspective on what the AI alignment landscape looks like. There are a collection of different approaches addressing different aspects of the alignment problem. Some of them, which MIRI is working a lot on, are technical things of how to ensure stability of goals and other similar thoughts along these lines that should be necessary for any approach. Others are developed on how to make the AI safe either indirectly or make itself fully aligned. So, the first category you have things like software as a service. Can we have super intelligent abilities integrated in a system that doesn't allow for say super intelligent agents with pernicious goals.
Others that I have looked into in the past are things like low impact agents or oracles, which again, the idea is we have a superintelligence, we cannot align it with human preferences, yet we can use it to get some useful work done. Then there are the approaches, which aim to solve the whole problem and get actual alignment, what used to be called the friendly AI approach. So here, it's not an AI that's constrained in any ways, it's an AI that is intrinsically motivated to do the right thing. There are a variety of different approaches to that, some more serious than others. Paul Christiano has an interesting variant on that, though it's hard to tell, I would say, his in a bit of a mixture of value alignment and constraining what the AI can do in a sense, but it is very similar and so this is of that last type, of getting the aligned, the friendly AI, the aligned utility function.
In that area, there are what I would call the ones that sort of rely on indirect proxies. This is the ideas of you put Nick Bostrom in a room for 500 years or a virtual version of that and hope that you get something aligned at the end of that. There are direct approaches and this is the basic direct approach, doing everything the hard way in a sense but defining everything that needs to be defined so that the AI can then assemble an aligned preference function from all the data.
Lucas: Wonderful. So you gave us a good summary earlier of the different parts of this research agenda. Would you like to expand a little bit on the "fundamental idea" behind this specific research project?
Stuart: There are two fundamental ideas that are not too hard to articulate. The first is that though our revealed preferences could be wrong though our stated preferences could be wrong, what our actual preferences are at least in one moment is what we model inside our head, what we're thinking of as the better option. We might lie, as I say, in politics or in a court of law or just socially but generally, when we know that we're lying, it's because there's a divergence between what we're saying and what we're modeling internally. So, it is this internal model, which I'm identifying as the place where our preferences lie and then all the rest of it, the whole convoluted synthesis project is just basically how do we take these basic pieces and combine them in a way that does not seem to result in anything disastrous and that respects human preferences and meta-preferences and this is a key thing, actually reaches a result. That's why the research project is designed for having a lot of default actions in a lot of situations.
Like if the person does not have strong meta-preferences, then there's a whole procedure of how you combine say preferences about the world and preferences about your identity are, by default, combined in a different way if you would want GDP to go up, that's a preference about the world. If you yourself would want to believe something or believe only the truth, for example, that's a preference about your identity. It tends to be that identity preferences are more fragile, so the default is that preferences about the world are just added together and this overcomes most of the contradictions because very few human preferences are exactly anti-aligned whereas identity preferences are combined in a more smooth process so that you don't lose too much on any of them. But as I said, these are the default procedures, and they're all defined so that we get an answer but there's also large abilities for the person's meta-preferences to override the defaults. Again, precautions are taken to ensure that an answer is actually reached.
Lucas: Can you unpack what partial preferences are? What you mean by partial preferences and how they're contextualized within human mental models?
Stuart: What I mean by partial preference is mainly that a human has a small model of part of the world like let's say they're going to a movie and they would prefer to invite someone they like to go with them. Within this mental model, there is the movie, themselves and the presence or absence of the other person. So, this is a very narrow model of reality, virtually the entire rest of the world and, definitely, the entire rest of the universe does not affect this. It could be very different and not change anything of this. So, this is what I call a partial preference. You can't go from this to a general rule of what the person would want to do in every circumstance but it is a narrow valid preference. Partial preferences refers to two things, first of all, that it doesn't cover all of our preferences and secondly, the model in which it lives only covers a narrow slice of the world.
You can make some modifications to this. This is the whole point of the second section that if the approach works, variations on the synthesis project should not actually result in results that are disastrous at all. If the synthesis process being changed a little bit would result in a disaster, then something has gone wrong with the whole approach but you could, for example, add restrictions like looking for consistent preferences but I'm starting with basically the fundamental thing is there is this mental model, there is an unambiguous judgment that one thing is better than another and then we can go from there in many ways. A key part of this approach is that there is no single fundamental synthesis process that would work, so it is aiming for an adequate synthesis rather than an idealized one because humans are a mess of contradictory preferences and because even philosophers have contradictory meta-preferences within their own minds and with each other and because people can learn different preferences depending on the order in which information is presented to them, for example.
Any method has to make a lot of choices, and therefore, I'm writing down explicitly as many of the choices that have to be made as I can so that other people can see what I see the processes entailing. I am quite wary of things that look for reflexive self-consistency because in a sense, if you define your ideal system as one that's reflexively self-consistent, that's a sort of local condition in a sense that the morality judges itself by its own assessment and that means that you could theoretically wander arbitrarily far in preference space before you hit that. I don't want something that is just defined by this has reached reflective equilibrium, this morality synthesis is now self-consistent, I want something that is self-consistent and it's not too far from where it started. So, I prefer to tie things much more closely to actual human preferences and to explicitly aim for a synthesis process that doesn't wander too far away from them.
Lucas: I see, so the starting point is the evaluative moral that we're trying to keep it close to?
Stuart: Yes, I don't think you can say that any human preference synthesized is intrinsically wrong as long as it reflects some of the preferences that were inputs into it. However, I think you can say that it is wrong from the perspective of the human that you started with if it strongly contradicts what they would want. Disagreements from my starting position is something which I take to be very relevant to the ultimate outcome. There's a bit of a challenge here because we have to avoid say preferences which are based on inaccurate facts. So, some of the preferences are inevitably going to be removed or changed just because they're based on factually inaccurate beliefs. Some other processes of trying to make consistent what is sort of very vague will also result in some preferences being moved beyond. So, you can't just say the starting person has veto power over the final outcome but you do want to respect their starting preferences as much as you possibly can.
Lucas: So, reflecting here on the difficulty of this agenda and on how human beings contain contradictory preferences and models, can you expand a bit how we contain these internal contradictions and how this contributes to the difficulty of the agenda?
Stuart: I mean humans contain many contradictions within them. Our mood shifts. We famously are hypocritical in favor of ourselves and against the foibles of others, we basically rewrite narratives to allow ourselves to always be heroes. Anyone who's sort of had some experience of a human has had knowledge of when they've decided one way or decided the other way or felt that something was important and something else wasn't and often, people just come up with a justification for what they wanted to do anyway, especially if they're in a social situation, and then some people can cling to this justification and integrate that into their morality while behaving differently in other ways. The easiest example are sort of political hypocrites. The anti-gay preacher who sleeps with other men is a stereotype for a reason but it's not just a sort of contradiction at that level. It's that basically most of the categories in which we articulate our preferences are not particularly consistent.
If we throw a potentially powerful AI in this, which could change the world drastically, we may end up with things across our preferences. For example, suppose that someone created or wanted to create a subspecies of human that was bred to be a slave race. Now, this race did not particularly enjoy being a slave race but they wanted to be slaves very strongly. In this situation, a lot of our intuitions are falling apart because we know that slavery is almost always involuntary and is backed up by coercion. We also know that even though our preferences and our enjoyments do sometimes come apart, they don't normally come apart that much. So, we're now confronted by a novel situation where a lot of our intuitions are pushing against each other.
You also have things like nationalism for example. Some people have strong nationalist sentiments about their country and sometimes their country changes and in this case, what seemed like a very simple, yes, I will obey the laws of my nation, for example, becomes much more complicated as the whole concept of my nation starts to break down. This is the main way that I see preferences to being underdefined. They're articulated in terms of concepts which are not universal and which bind together many, many different concepts that may come apart.
Lucas: So, at any given moment, like myself at this moment, the issue is that there's a large branching factor of how many possible future Lucases there can be. At this time, currently and maybe a short interval around this time as you sort of explore in your paper, the sum total of my partial preferences and the partial world models in which these partial preferences are contained. The expression of these preferences and models can be expressed differently and sort of hacked and changed based off how questions are asked, the order of questions. I am like a 10,000-faced thing which I can show you one of my many faces depending on how you push my buttons and depending on all of the external input that I get in the future, I'm going to express and maybe become more idealized in one of many different paths. The only thing that we have to evaluate which of these many different paths I would prefer is what I would say right now, right?
Say my core value is joy or certain kinds of conscious experiences over others and all I would have for evaluating this many branching thing is say this preference now at this time but that could be changed in the future, who knows? I will create new narratives and stories that justify the new person that I am and that makes sense of the new values and preferences that I have retroactively, like something that I wouldn't actually have approved of now but my new, maybe more evil version of myself would approve and create a new narrative retroactively. Is this sort of helping to elucidate and paint the picture of why human beings are so messy?
Stuart: Yes, we need to separate that into two. The first is that our values can be manipulated by other humans as they often are and by the AI itself during the process but that can be combated to some extent. I have a paper that may soon come out on how to reduce the influence of an AI over a learning process that it can manipulate. That's one aspect. The other aspect is when you are confronted by a new situation, you can go in multiple different directions and these things are just not defined. So, when I said that human values are contradictory, changeable, manipulable and underdefined, I was saying that the first three are relatively easy to deal with but that the last one is not.
Most of the time, people have not considered the whole of the situation that they or the world or whatever is confronted with. No situation is exactly analogous to another, so you have to try and fit it in to different categories. So if someone dubious gets elected in a country and starts doing very authoritarian things, does this fit in the tyranny box which should be resisted or does this fit in the normal process of democracy box in which case it should be endured and dealt with through democratic means. What'll happen is generally that it'll have features of both, so it might not fit comfortably in either box and then there's a wide variety for someone to be hypocritical or to choose one side or the other but the reason that there's such a wide variety of possibilities is because this is a situation that has not been exactly confronted before so people don't actually have preferences here. They don't have a partial preference over this situation because it's not one that they've ever considered.
How they develop one is due to a lot as you say, the order in which information is presented, which category it seems to most strongly fit into and so on. We are going here for very mild underdefinedness. The willing slave race was my attempt to push it out a bit further into something somewhat odd and then if you consider a powerful AI that is able to create vast numbers of intelligent entities, for example, and reshape society, human bodies and human minds in hugely transformative ways, we are going to enter sort of very odd situations where all our starting instincts are almost useless. I've actually argued at some point in the research agenda that this is an argument for insuring that we don't go too far from the human baseline normal into exotic things where our preferences are not well-defined because in these areas, the chance that there is a large negative seems higher than the chance that there's a large positive.
Now, I'm talking about things that are very distant in terms of our categories, like the world of Star Trek is exactly the human world from this perspective because even though they have science fiction technology, all of the concepts and decisions they are articulated around concepts that we're very familiar with because it is a work of fiction addressed to us now. So, when I say not go too far, I don't mean not embrace a hugely transformative future. I'm saying not embrace a hugely transformative future where our moral categories start breaking down.
Lucas: In my mind, there's two senses. There's the sense in which we have these models for things and we have all of these necessary and sufficient conditions for which something can be pattern matched to some sort of concept or thing and we can encounter situations where there're conditions for many different things being included in the context in a new way which makes it so that the thing like goodness or justice is underdefined in the slavery case because we don't really know initially whether this thing is good or bad. I see this underdefined in this sense. The other sense is maybe the sense in which my brain is a neural architectural aggregate of a lot of neurons and the sum total of its firing statistics and specific neural pathways can be potentially identified as containing preferences and models somewhere within there. So is it also true to say that it's underdefined in the sense that the human as not a thing in the world but as a process in the world largely constituted of the human brain, even within that process, it's underdefined where in the neural firing statistics or the processing of the person there could ever be something called a concrete preference or value?
Stuart: I would disagree that it is underdefined in the second sense.
Lucas: Okay.
Stuart: In order to solve the second problem, you need to solve the symbol grounding problem for humans. You need to show that the symbols or the neural pattern firing or the neuron connection or something inside the brain corresponds to some concepts in the outside world. This is one of my sort of side research projects. When I say side research project, I mean I wrote a couple of blog posts on this pointing out how I might approach it and I point out that you can do this in a very empirical way. If you think that a certain pattern of neural firing refers to say a rabbit, you can see whether this thing firing in the brain is that predictive of say a rabbit in the outside world or predictive of this person is going to start talking about rabbits soon.
In model theory, the actual thing that gives meaning to the symbols is sort of beyond the scope of the math theory but if you have a potential connection between the symbols and the outside world, you can check whether this theory is a good one or a terrible one. If you say this corresponds to hunger and yet that thing only seems to trigger when someone's having sex, for example, we can say, okay, your model that this corresponds to hunger is terrible. It's wrong. I cannot use it for predicting that the person will eat in the world but I can use it for predicting that they're having sex. So, if I model this as connected with sex, this is a much better grounding of that symbol. So using methods like this and there're some subtleties I also address Quine's "gavagai" and connect it to sort of webs of connotation and concepts that go together but the basic idea is to empirically solve the symbol grounding problem for humans.
When I say that things are underdefined, I mean that they are articulated in terms of concepts that are underdefined across all possibilities in the world, not that these concepts could be anything or we don't know what they mean. Our mental models correspond to something. It's a collection of past experience and the concepts in our brain are tying together a variety of experiences that we've had. They might not be crisp. They might not be well-defined even if you look at say the totality of the universe but they correspond to something, to some repeated experience, some concepts to some thought process that we've had and that we've extracted this idea from. When we do this in practice, we are going to inject some of our own judgements into it and since humans are so very similar in how we interpret each other and how we decompose many concepts, it's not necessarily particularly bad that we do so, but I strongly disagree that these are arbitrary concepts that are going to be put in by hand. They are going to be in the main identified via once you have some criteria for tracking what happens in the brain, comparing it with the outside world and those kinds of things.
My concept, maybe a cinema is not an objectively well-defined fact but what I think of as a cinema and what I expect in a cinema and what I don't expect in a cinema, like I expect it to go dark and a projector and things like that. I don't expect that this would be in a completely open space in the Sahara Desert under the sun with no seats and no sounds and no projection. I'm pretty clear that one of these things is a lot more of a cinema than the other.
Lucas: Do you want to expand here a little bit about this synthesis process?
Stuart: The main idea is to try and ensure that no disasters come about and the main thing that could lead to a disaster is the over prioritization of certain preferences over others. There are other avenues to disaster but this seems to be the most obvious. The other important part of the synthesis process is that it has to reach an outcome, which means that a vague description is not sufficient, so that's why it's phrased in terms of this is the default way that you synthesize preferences. This way may be modified by certain meta-preferences. The meta-preferences have to be reducible to some different way of synthesizing the preferences.
For example, the synthesis is not particularly over-weighting long-term preferences versus short term preferences. It would prioritize long-term preferences but not exclude short term ones. So, I want to be thin is not necessarily prioritizing over that's a delicious piece of cake that I'd like to eat right now, for example, but human meta-preferences often prioritize long-term preferences over short term ones, so this is going to be included and this is going to change the default balance towards long-term preferences.
Lucas: So, powering the synthesis process, how are we to extract the partial preferences and their weights from the person?
Stuart: That's, as I say, the first part of the project and that is a lot more empirical. This is going to be a lot more looking at what neuroscience says, maybe even what algorithm theory says or what modeling of algorithms say and about what's physically going on in the brain and how this corresponds to internal mental models. There might be things like people noting down what they're thinking, correlating this with changes in the brain and this is a much more empirical aspect to the process that could be carried out essentially independently from the synthesis product.
Lucas: So, a much more advanced neuroscience would be beneficial here?
Stuart: Yes, but even without that, it might be possible to infer some of these things indirectly via the AI and if the AI accounts well for uncertainties, this will not result in disasters. If it knows that we would really dislike losing something of importance to our values, even if it's not entirely sure what the thing of importance is, it will naturally, with that kind of motivation, act in a cautious way, trying to preserve anything that could be valuable until such time as it figures out better what we want in this model.
Lucas: So, in section two of your paper, synthesizing the preference utility function, within this section, you note that this is not the only way of constructing the human utility function. So, can you guide us through this more theoretical section, first discussing what sort of utility function and why a utility function in the first place?
Stuart: One of the reasons to look for a utility function is to look for something stable that doesn't change over time and there is evidence that consistency requirements will push any form of preference function towards a utility function and that if you don't have a utility function, you just lose value. So, the desire to put this into a utility function is not out of an admiration for utility functions per se but our desire to get something that won't further change or won't further drift in a direction that we can't control and have no idea about. The other reason is that as we start to control our own preferences better and have a better ability to manipulate our own minds, we are going to be pushing ourselves towards utility functions because of the same pressures of basically not losing value pointlessly.
You can kind of see it in some investment bankers who have to a large extent, constructed their own preferences to be expected money maximizers within a range and it was quite surprising to see but human beings are capable of pushing themselves towards that and this is what repeated exposure to different investment decision tends to do to you and it's the correct thing to do in terms of maximizing the money and this is the kind of thing that general pressure on humans combined with human's ability to self-modify, which we may develop in the future, so all this is going to be pushing us towards a utility function anyway, so we may as well go all the way and get the utility function directly rather than being pushed into it.
Lucas: So, is the view here that the reason why we're choosing utility functions even when human beings are very far from being utility functions is that when optimizing our choices in mundane scenarios, it's pushing us in that direction anyway?
Stuart: In part. I mean utility functions can be arbitrarily complicated and can be consistent with arbitrarily complex behavior. A lot of when people think of utility functions, they tend to think of simple utility functions and simple utility functions are obviously simplifications that don't capture everything that we value but complex utility functions can capture as much of the value as we want. What tends to happen is that when people have say, inconsistent preferences, that they are pushed to make them consistent by the circumstances of how things are presented, like you might start with the chocolate mousse but then if offered a trade for the cherry pie, go for the cherry pie and then if offered a trade for the maple pie, go for the maple pie but then you won't go back to the chocolate or even if you do, you won't continue going around the cycle because you've seen that there is a cycle and this is ridiculous and then you stop it at that point.
So, what we decide when we don't have utility functions tends to be determined by the order in which things are encountered and under contingent things and as I say, non-utility functions tend to be intrinsically less stable and so can drift. So, for all these reasons, it's better to nail down a utility function from the start so that you don't have the further drift and your preferences are not determined by the order in which you encounter things, for example.
Lucas: This is though in part thus a kind of normative preference then, right? To use utility functions in order not to be pushed around like that. Maybe one can have the meta-preferences for their preferences to be expressed in the order in which they encounter things.
Stuart: You could have that strong meta-preference, yes, though even that can be captured by a utility function if you feel like doing it. Utility functions can capture pretty much any form of preferences, even the ones that seem absurdly inconsistent. So, we're not actually losing anything in theory by insisting that it should be a utility function. We may be losing things in practice in the construction of that utility function. I'm just saying if you don't have something that is isomorphic with a utility function or very close to that, your preferences are going to drift randomly affected by many contingent factors. You might want that, in which case, you should put it in explicitly rather than implicitly and if you put it in explicitly, it can be captured by a utility function that is conditional on the things that you see, in the order in which you see them, for example.
Lucas: So, comprehensive AI services and other tool-like AI approaches to AI alignment I suppose avoid some of the anxieties produced by a strong agential AIs with utility functions. Are there alternative goal ordering or action producing methods in agents other than utility functions that may have the properties that we desire of utility functions or is the category of utility functions just so large that it encapsulates much of what is just mathematically rigorous and simple?
Stuart: I'm not entirely sure. Alternative goal structures tend to be quite ad hoc and limited in my practical experience whereas utility functions or reward functions which may or may not be isomorphic do seem to be universal. There are possible inconsistencies within utility functions themselves if you get a self-referential utility function including your own preferences, for example, but MIRI's work should hope to clarify those aspects. I came up with an alternative goal structure which is basically an equivalence class of utility functions that are not equivalent in terms of utility and this could successfully model an agent's who's preferences were determined by the order in which things were chosen but I put this together as a toy model or as a thought experiment. I would never seriously suggest building that. So, it just seems that for the moment, most non-utility function things are either ad hoc or under-defined or incomplete and that most things can be captured by utility functions, so the things that are not utility functions all seem at the moment to be flawed and the utility functions seem to be sufficiently versatile to capture anything that you would want.
This may mean by the way that we may lose some of the elegant properties of utility function that we normally assume like deontology can be captured by a utility function that assigns one to obeying all the rules and zero to violating any of them and this is a perfectly valid utility function, however, there's not much in terms of expected utility in terms of this. It behaves almost exactly like a behavioral constraint, never choose any option that is against the rules. That kind of thing, even though it's technically a utility function, might not behave the way that we're used to utility functions behaving in practice. So, when I say that it should be captured as a utility function, I mean formally it has to be defined in this way but informally, it may not have the properties that we informally expect of utility functions.
Lucas: Wonderful. This is a really great picture that you're painting. Can you discuss extending and normalizing the partial preferences? Take us through the rest of section two on synthesizing to a utility function.
Stuart: The extending is just basically you have, for instance, a preference of going to the cinema this day with that friend versus going to the cinema without that friend. That's an incredibly narrow preference, but you also have preferences about watching films in general, being with friends in general, so these things should be combined in as much as they can be into some judgment of what you like to watch, who you like to watch with and under what circumstances. That's the generalizing. The extending is basically trying to push these beyond the typical situations. So, if there was a sort of virtual reality, which really gave you the feeling that other people were present with you, which current virtual reality doesn't tend to, then would this count as being with your friend. What level of interaction would be required for it to count as being with your friend? Well, that's some of the sort of extending.
The normalizing is just basically the fact that utility functions are defined up to scaling, up to multiplying by some positive real constant. So, if you want to add utilities together or combine them in a smooth-min or combine them in any way, you have to scale the different preferences and there are various ways of doing this. I fail to find an intrinsically good way of doing it that has all the nice formal properties that you would want but there are a variety of ways that can be done, all of which seem acceptable. The one I'm currently using is the mean max normalization, which is that the best possible outcome gets a utility of one, and the average outcome gets a utility of zero. This is the scaling.
Then the weight of these preferences is just how strongly you feel about it. Do you have a mild preference for going to the cinema with this friend? Do you have an overwhelming desire for chocolate? Once they've normalized, you weigh them, and you combine them.
Lucas: Can you take us through the rest of section two here, if there's anything else here that you think is worth mentioning?
Stuart: I'd like to point out that this is intended to work with any particular human being that you point the process at, so there are a lot of assumptions that I made from my non-moral realist, worried about over simplification and other things. The idea is that if people have strong meta-preferences themselves, these will overwhelm the default decisions that I've made but if people don't have strong meta-preferences, then they are synthesized in this way in the way which I feel is the best to not lose any important human value. There are also judgements about what would constitute a disaster or how we might judge this to have gone disastrously wrong, those are important and need to be sort of fleshed out a bit more because many of them can't be quite captured within this system.
The other thing is that the outcomes may be very different. To choose a silly example, if you are 50% total utilitarian versus 50% average utilitarian or if you're 45%, 55% either way, the outcomes are going to be very different because the pressure on the future is going to be different and because the AI is going to have a lot of power, it's going to result in very different outcomes but from our perspective where if we put 50/50 total utilitarianism and average utilitarianism, we're not exactly 50/50 most of the time. We're kind of ... Yeah, they're about the same. So, 45, 55, should not result in a disaster if 50/50 doesn't.
So, even though from the perspective of these three mixes, 45/55, 50/50, 55/45, these three mixes will look at something that optimizes one of the other two mixes and say that is very bad from my perspective, however, more human perspective, we're saying all of them are pretty much okay. Well, we would say none of them are pretty much okay because they don't incorporate many other of our preferences but the idea is that when we get all the preferences together, it shouldn't matter a bit if it's a bit fuzzy. So even though the outcome will change a lot if we shift it a little bit, the quality of the outcome shouldn't change a lot and this is connected with a point that I'll put up in section three that uncertainties may change the outcome a lot but again, uncertainties should not change the quality of the outcome and the quality of the outcome is measured in a somewhat informal way by our current preferences.
Lucas: So, moving along here into section three, what can you tell us about the synthesis of the human utility function in practice?
Stuart: So, first of all, there's ... Well, let's do this project, let's get it done but we don't have perfect models of the human brain, we haven't grounded all the symbols, what are we going to do with the great uncertainties. So, that's arguing that even with the uncertainties, this method is considerably better than nothing and you should expect it to be pretty safe and somewhat adequate even with great uncertainties. The other part is I'm showing how thinking in terms of the human mental models can help to correct and improve some other methods like revealed preferences, our stated preferences, or the locking the philosopher in a box for a thousand years. All methods fail and we actually have a pretty clear idea when they fail, revealed preferences fail because we don't model bounded rationality very well and even when we do, we know that sometimes our preferences are different from what we reveal. Stated preferences fail in situations where there's strong incentives not to tell the truth, for example.
We could deal with these by sort of adding all the counter examples of the special case or we could add the counter examples as something to learn from or what I'm recommending is that we add them as something to learn from while stating that the reason that this is a counter example is that there is a divergence between whatever we're measuring and the internal model of the human. The idea being that it is a lot easier to generalize when you have an error theory rather than just lists of error examples.
Lucas: Right and so there's also this point of view here that you're arguing that this research agenda and perspective is also potentially very helpful for things like corrigibility and low impact research and Christiano's distillation and amplification, which you claim all seem to be methods that require some simplified version of the human utility function. So any sorts of conceptual insights or systematic insights which are generated through this research agenda in your view seem to be able to make significant contributions to other research agendas which don't specifically take this lens?
Stuart: I feel that even something like corrigibility can benefit from this because in my experience, things like corrigibility, things like low impact have to define to some extent what is important and what can be categorized as unimportant. A low impact AI cannot be agnostic about our preferences, it has to know that a nuclear war is a high impact thing whether or not we'd like it whereas turning on an orange light that doesn't go anywhere is a low impact thing, but there's no real intrinsic measure by which one is high impact and the other is low impact. Both of them have ripples across the universe. So, I think I phrased it as Hitler, Gandhi and Thanos all know what a low impact AI is, all know what an oracle AI is, or know the behavior to expect from it. So, it means that we need to get some of the human preferences in, the bit that tells us that nuclear wars are high impact but we don't need to get all of it in because since so many different humans will agree on it, you don't need to capture any of their individual preferences.
Lucas: So, it's applicable to these other methodologies and it's also your belief and I'm quoting you here, you say that, “I'd give a 10% chance of it being possible this way, meaning through this research agenda and a 95% chance that some of these ideas will be very useful for other methods of alignment.” So, just adding that here as your credences for the skillfulness of applying insights from this research agenda to other areas of AI alignment.
Stuart: In a sense, you could think of this research agenda in reverse. Imagine that we have reached some outcome that isn't some positive outcome, we have got alignment and we haven't reached it through a single trick and we haven't reached it through the sort of tool AIs or software as a service or those kinds of approaches, we have reached an actual alignment. It, therefore, seems to me all the problems that I've listed or almost all of them will have had to have been solved, therefore, in a sense, much of this research agenda needs to be done directly or indirectly in order to achieve any form of sensible alignment. Now, the term directly or indirectly is doing a lot of the work here but I feel that quite a bit of this will have to be done directly.
Lucas: Yeah, I think that that makes a lot of sense. It seems like there's just a ton about the person that is just confused and difficult to understand what we even mean here in terms of our understanding of the person and also broader definitions included in alignment. Given this optimism that you've stated here surrounding the applicability of this research agenda on synthesizing a humans' preferences into a utility function, what can you say about the limits of this method? Any pessimism to inject here?
Stuart: So, I have a section four, which is labeled as the things that I don't address. Some of these are actually a bit sneaky like the section on how to combine the preferences of different people because if you read that section, it basically lays out ways of combining different people's preferences. But I've put it in that to say I don't want to talk about this issue in the context of this research agenda because I think this just diverts from the important work here, and there are a few of those points but some of them are genuine things that I think are problems and the biggest is the fact that there is a sort of informal Godel statement in humans about their own preferences. How many people would accept a computer synthesis of their preferences and say yes, that is my preferences, especially when they can explore it a bit and find the counter intuitive bits? I expect humans in general to reject the AI assigned synthesis no matter what it is, pretty much just because it was synthesized and then given to them, I expect them to reject or want to change it.
We have a natural reluctance to accept the judgment of other entities about our own morality and this is a perfectly fine meta-preference that most humans have and I think all humans have to some degree and I have no way of capturing it within the system because it's basically a Godel statement in a sense. The best synthesis process is the one that wasn't used. The other thing is that people want to continue with moral learning and moral improvement and I've tried to decompose moral learning and more improvements into different things and show that some forms of moral improvements and moral learning will continue even when you have a fully synthesized utility function but I know that this doesn't capture everything of what people mean by this and I think it doesn't even capture everything of what I would mean by this. So, again, there is a large hole in there.
There are some other holes of the sort of more technical nature like infinite utilities, stability of values and a bunch of other things but conceptually, I'm the most worried about these two aspects, the fact that you would reject what values you were assigned and the fact that you'd want to continue to improve and how do we define continuing improvement that isn't just the same as well your values may drift randomly.
Lucas: What are your thoughts here? Feel free to expand on both the practical and theoretical difficulties of applying this across humanity and aggregating it into a single human species wide utility function.
Stuart: Well, the practical difficulties are basically politics, how to get agreements between different groups. People might want to hang onto their assets or their advantages. Other people might want sort of stronger equality. Everyone will have broad principles to appeal to. Basically, there's going to be a lot of fighting over the different weightings of individual utilities. The hope there is that, especially with a powerful AI, that the advantage might be sufficiently high that it's easier to do something where everybody gains even if the gains are uneven than to talk about how to divide a fixed sized pie. The theoretical issue is mainly what do we do with anti-altruistic preferences. I'm not talking about selfish preferences, those are very easy to deal with. That's just basically competition for the utility, for the resources, for the goodness but actual anti-altruistic utilities so, someone who wants harm to befall other people and also to deal with altruistic preferences because you shouldn't penalize people for having altruistic preferences.
You should, in a sense, take out the altruistic preferences and put that in the humanity one and allow their own personal preferences some extra weight, but anti-altruistic preferences are a challenge especially because it's not quite clear where the edge is. Now, if you want someone to suffer, that's an anti-altruistic preference. If you want to win a game and part of your enjoyment of the game is that other people lose, where exactly does that lie and that's a very natural preference. You might become a very different person if you didn't get some at least mild enjoyment from other people losing or from the status boost there is a bit tricky. You might sort of just tone them down so that mild anti-altruistic preferences are perfectly fine, so if you want someone to lose to your brilliant strategy at chess, that's perfectly fine but if you want someone to be dropped slowly into a pit of boiling acid, then that's not fine.
The other big question is population ethics. How do we deal with new entities and how do we deal with other conscious or not quite conscious animals around the world, so who gets to count as a part of the global utility function?
Lucas: So, I'm curious to know about concerns over aspects of this alignment story or any kind of alignment story involving lots of leaky abstractions, like in Rich Sutton's short essay called The Bitter Lesson, he discusses how the bitter lesson of computer science is how leveraging computation over human domain-specific ingenuity has broadly been more efficacious for breeding very powerful results. We seem to have this tendency or partiality towards trying to imbue human wisdom or knowledge or unique techniques or kind of trickery or domain-specific insight into architecting the algorithm and alignment process in specific ways whereas maybe just throwing tons of computation at the thing has been more productive historically. Do you have any response here for concerns over concepts being leaky abstractions, or the categories in which you use to break down human preferences, not fully capturing what our preferences are?
Stuart: Well, in a sense that's part of the research project and part of the reasons why I warned against going to distant words where in my phrasing, the web of connotations break down, in your phrasing the abstractions become too leaky and this is also part of why even though the second part is done as if this is the theoretical way of doing it, I also think there should be a lot of experimental aspect to it to test where this is going, where it goes surprisingly wrong or surprisingly right, the second part, though it's presented as just this is basically the algorithm, it should be tested and checked and played around with to see how it goes. For The Bitter Lesson, the difference here I think is that in the case of The Bitter Lesson, we know what we're trying to do.
We have objectives whether it's winning at a game, whether it's classifying images successfully, whether it's classifying some other feature successfully, we have some criteria for the success of it. The constraints I'm putting in by hand are not so much trying to put in the wisdom of the human or the wisdom of the Stuart. There's some of that but it's to try and avoid disasters and the disasters cannot be just avoided with more data. You can get to many different points from the data and I'm trying to carve away lots of them. Don't oversimplify, for example. So, to go back to The Bitter Lesson, you could say that you can tune your regularizer and what I'm saying is have a very weak regularizer, for example and this is not something that The Bitter Lesson applies to because in the real world, on the problems where The Bitter Lesson applies, you can see whether hand tuning the regularizer works because you can check what the outcome is and compare it with what you want.
Since you can't compare it with what you want, because if we knew what we wanted we'd kind of have it solved, what I'm saying here is don't put a strong regularizer for these reasons. The data can't tell me that I need a stronger regularizer because the data has no opinion if you want on that. There is no ideal outcome to compare with. There might be some problems but the problems like if our preferences do not look like my logic or like our logic, this points towards the method failing, not towards the method's needing more data and less restrictions.
Lucas: I mean I'm sure part of this research agenda is also further clarification and refinement of the taxonomy and categories used, which could potentially be elucidated by progress in neuroscience.
Stuart: Yes, and there's a reason that this is version 0.9 and not yet version 1. I'm getting a lot of feedback and going to refine it before trying to put it out as version 1. It's in alpha or in beta at the moment. It's a prerelease agenda.
Lucas: Well, so hopefully this podcast will spark a lot more interest and knowledge about this research agenda and so hopefully we can further contribute to bettering it.
Stuart: When I say that this is in alpha or in beta, that doesn't mean don't criticize it, do criticize it and especially if these can lead to improvements but don't just assume that this is fully set in stone yet.
Lucas: Right, so that's sort of framing this whole conversation in the light of epistemic humility and willingness to change. So, two more questions here and then we'll wrap up. So, reflective equilibrium, you say that this is not a philosophical ideal, can you expand here about your thoughts on reflective equilibrium and how this process is not a philosophical ideal?
Stuart: Reflective equilibrium is basically you refine your own preferences, make them more consistent, apply them to yourself until you've reached a moment where your meta-preferences and your preferences are all smoothly aligned with each other. What I'm doing is a much more messy synthesis process and I'm doing it in order to preserve as much as possible of the actual human preferences. It is very easy to reach reflective equilibrium by just, for instance, having completely flat preferences or very simple preferences, these tend to be very reflectively in equilibrium with itself and pushing towards this thing is a push towards, in my view, excessive simplicity and the great risk of losing valuable preferences. The risk of losing valuable preferences seems to me a much higher risk than the gain in terms of simplicity or elegance that you might get. There is no reason that the kludgey human brain and it's mess of preferences should lead to some simple reflective equilibrium.
In fact, you could say that this is an argument against reflexive equilibrium because it means that many different starting points, many different minds with very different preferences will lead to similar outcomes which basically means that you're throwing away a lot of the details of your input data.
Lucas: So, I guess two things, one is that this process clarifies and improves on incorrect beliefs in the person but it does not reflect what you or I might call moral wrongness, so like if some human is evil, then the synthesized human utility function will reflect that evilness. So, my second question here is, an idealization process is very alluring to me. Is it possible to synthesize the human utility function and then run it internally on the AI and then see what we get in the end and then check if that's a good thing or not?
Stuart: Yes, in practice, this whole thing, if it works, is going to be very experimental and we're going to be checking the outcomes and there's nothing wrong with sort of wanting to be an idealized version of yourself. What I have, especially if it's just one idealized, it's the version where you are the idealized version of the idealized version of the idealized version of the idealized version, et cetera, of yourself where there is a great risk of losing yourself and the inputs there. This is where I had the idealized process where I started off wanting to be more compassionate and spreading my compassion to more and more things at each step, eventually coming to value insects as much as humans and then at the next step, value rocks as much as humans and then removing humans because of the damage that they can do to mountains, that was a process or something along the lines of what I can see if you are constantly idealizing yourself without any criteria for stop idealizing now or you've gone too far from where you started.
Your ideal self is pretty close to yourself. The triple idealized version of your idealized, idealized self or so on, starts becoming pretty far from your starting point and this is the sort of areas where I fear over-simplicity or trying to get to reflective equilibrium at the expense of other qualities and so on, these are the places where I fear this pushes towards.
Lucas: Can you make more clear what failed in our view in terms of that idealization process where Mahatma Armstrong turns into a complete negative utilitarian?
Stuart: It didn't even turn into a negative utilitarian, it just turned into someone that valued rocks as much as they valued humans and therefore eliminated humans on utilitarian grounds in order to preserve rocks or to preserve insects if you wanted to go down one level of credibility. The point of this is this was the outcome of someone that wants to be more compassionate, continuously wanting to make more compassionate versions of themselves that still want to be more compassionate and so on. It went too far from where it had started. It's one of many possible narratives but the point is the only way of resisting something like that happening is to tie the higher levels to the starting point. A better thing might say I want to be what myself would think is good and what my idealized self would think was good and what the idealized, idealized self would think was good and so on. So that kind of thing could work but just idealizing without ever tying it back to the starting point, to what compassion meant for the first entity, not what it meant for the nth entity is the problem that I see here.
Lucas: If I think about all possible versions of myself across time and I just happen to be one of them, this just seems to be a meta-preference to bias towards the one that I happen to be at this moment, right?
Stuart: We have to make a decision as to what preferences to take and we may as well take now because if we try and take into account our future preferences, we are starting to come a cropper with the manipulable aspect of our preferences. The fact that these could be literally anything. There is a future Stuart who is probably a Nazi because you can apply a certain amount of pressure to transform my preferences. I would not want to endorse their preferences now. There are future Stuarts who are saints, whose preferences I might endorse. So, if we're deciding which future preferences that we're accepting, we have to decide it according to criteria and criteria that at least are in part of what we have now.
We could sort of defer to our expected future selves if we sort of say I expect a reasonable experience of the future, define what reasonable means and then average out our current preferences with our reasonable future preferences if we can define what we mean by reasonable then, then yes, we can do this. This is our sole way of doing things and if we do it this way, it will most likely be non-disastrous. If doing the synthesis process with our current preference is non-disastrous then doing it with the average of our future reasonable preferences is also going to be non-disastrous. This is one of the choices that you could choose to put into the process.
Lucas: Right, so we can be mindful here that we'll have lots of meta-preferences about the synthesis process itself.
Stuart: Yes, you can put it as a meta-preference or you can put it explicitly in the process if that's a way you would prefer to do it. The whole process is designed strongly around get an answer from this process, so the, yes, we could do this, let's see if we can do it for one person over a short period of time and then we can talk about how we might take into account considerations like that, including as I say, this might be in the meta-preferences themselves. This is basically another version of moral learning. We're kind of okay with our values shifting but not okay with our values shifting arbitrarily. We really don't want our values to completely flip from what we have now, though some aspects we're more okay with them changing. This is part of the complicated how do you do moral learning.
Lucas: All right, beautiful, Stuart. Contemplating all this is really quite fascinating and I just think in general, humanity has a ton more thinking to do and self-reflection in order to get this process really right and I think that this conversation has really helped elucidate that to me and all of my contradictory preferences and my multitudes within the context of my partial and sometimes erroneous mental models, reflecting on that also has me feeling maybe slightly depersonalized and a bit ontologically empty but it's beautiful and fascinating. Do you have anything here that you would like to make clear to the AI alignment community about this research agenda? Any last few words that you would like to say or points to clarify?
Stuart: There are people who disagree with this research agenda, some of them quite strongly and some of them having alternative approaches. I like that fact that they are researching other alternatives. If they disagree with the agenda and want to engage with it, the best engagement that I could see is pointing out why bits of the agenda are unnecessary or how alternate solutions could work. You could also point out that maybe it's impossible to do it this way, which would also be useful but if you think you have a solution or the sketch of a solution, then pointing out which bits of the agenda you solve otherwise would be a very valuable exercise.
Lucas: In terms of engagement, you prefer people writing responses on the AI Alignment forum or Lesswrong
Stuart: Emailing me is also fine. I will eventually answer every non-crazy email.
Lucas: Okay, wonderful. I really appreciate all of your work here on this research agenda and all of your writing and thinking in general. You're helping to create beautiful futures with AI and you're much appreciated for that.
If you enjoyed this podcast, please subscribe, give it a like, or share it on your preferred social media platform. We'll be back again soon with another episode in the AI Alignment series.
Related episodes
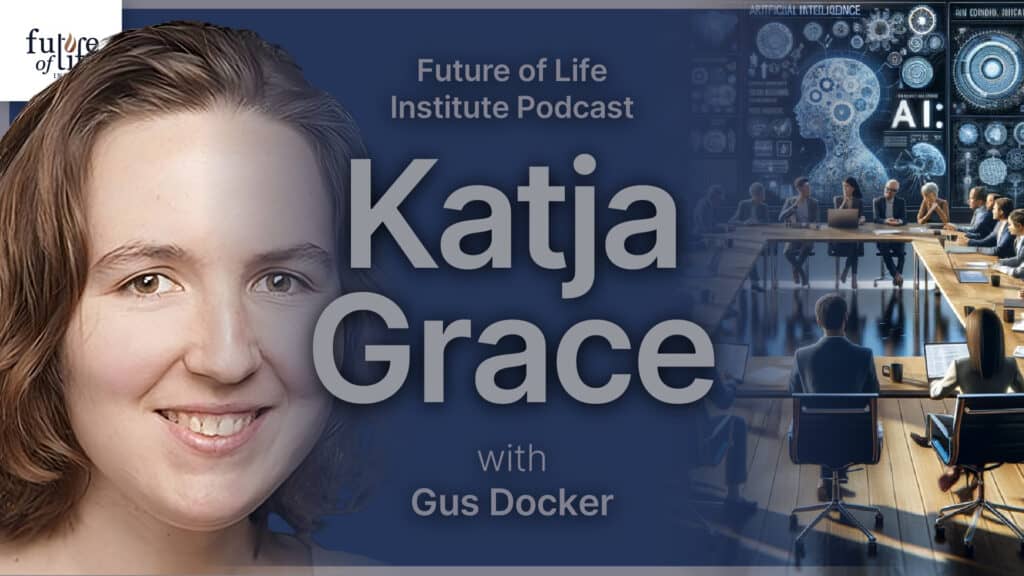
Katja Grace on the Largest Survey of AI Researchers
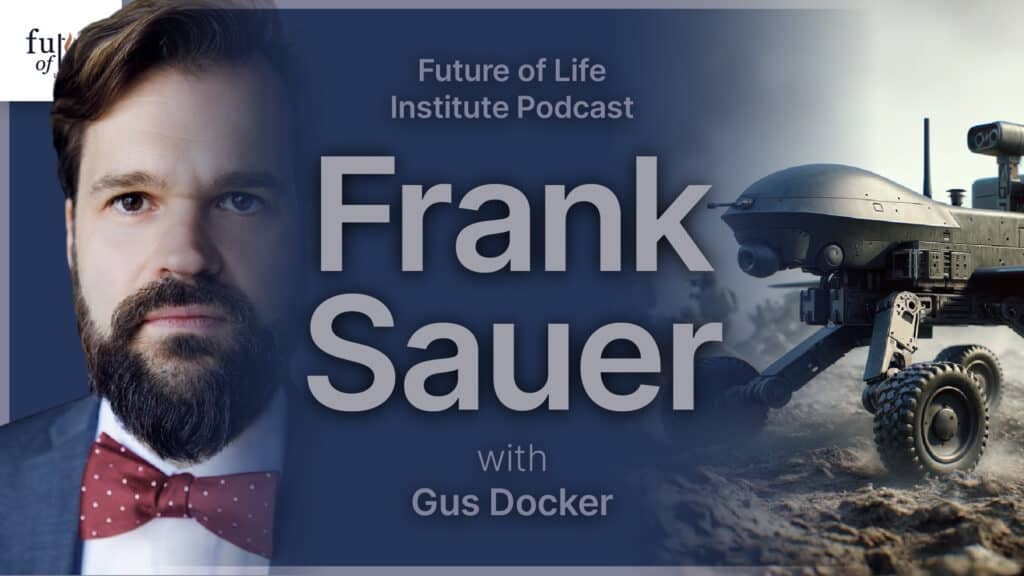
Frank Sauer on Autonomous Weapon Systems
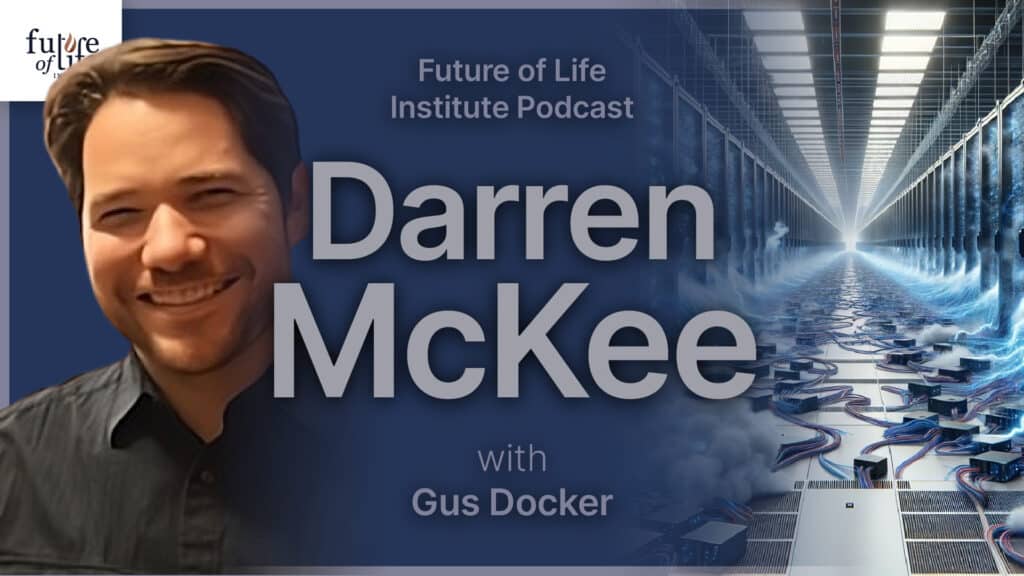