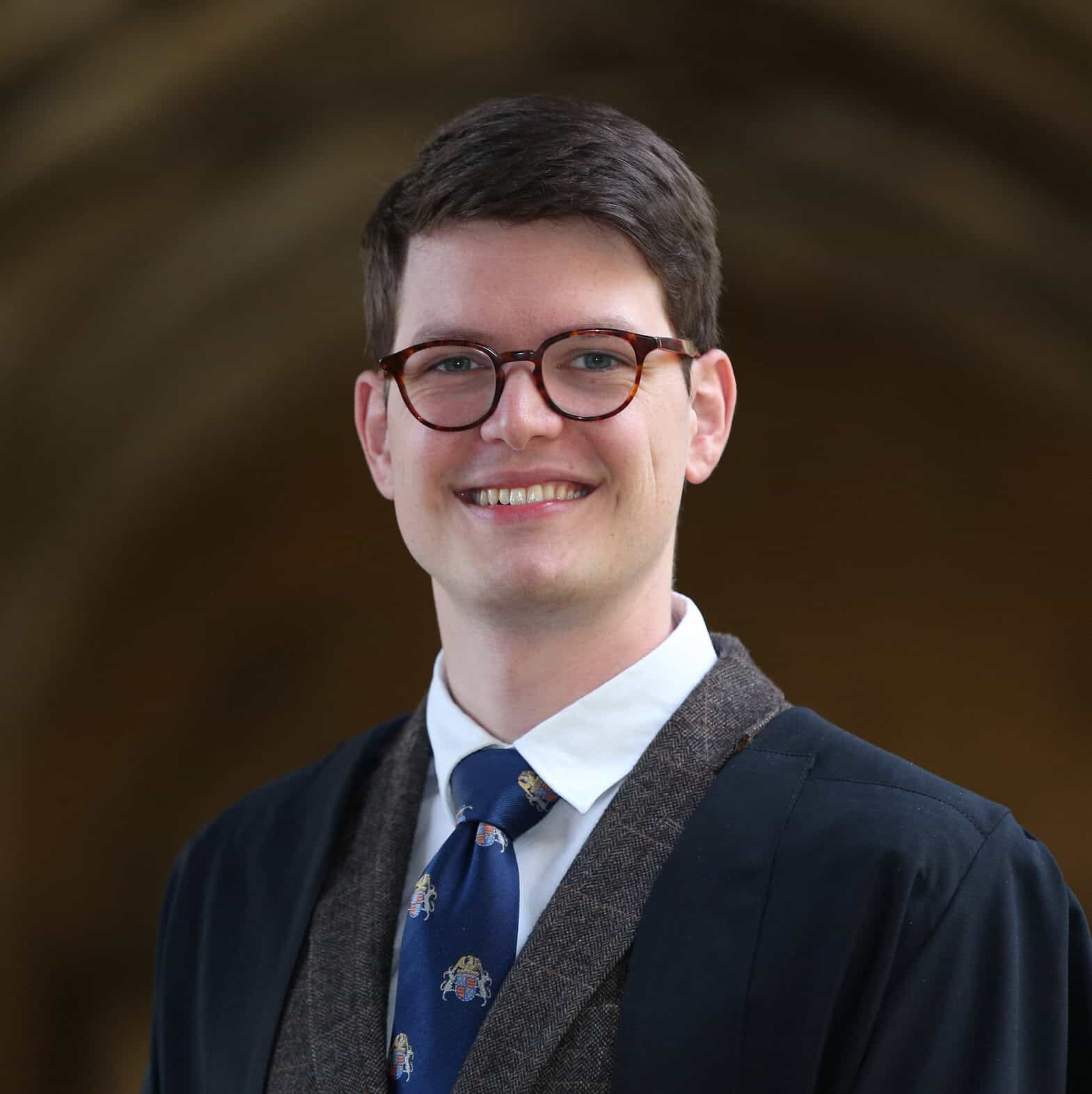
Vincent Fortuin
Why do you care about AI Existential Safety?
My strong interest in AI safety research stems from a deep-rooted recognition of AI’s potential and perils, particularly in critical applications like healthcare, finance, and autonomous driving. The reliable functionality of AI systems, even under unpredictable circumstances, is vital to prevent catastrophic failures and to foster trust among end-users. It’s imperative that AI not only makes precise decisions but also gauges and communicates the uncertainty in its predictions, especially when misjudgments could result in dire consequences. Upholding robustness and reliability, my AI safety research seeks to mitigate risks, ensuring technologies function securely and predictably. My commitment lies in championing for a future where AI is both innovative and safely interwoven into our societal fabric, guaranteeing a secure, dependable future for all.
Please give at least one example of your research interests related to AI existential safety:
My own research on AI safety connects several pivotal areas: improving uncertainty estimation, boosting interpretability in medical applications, and incorporating expert prior knowledge, while also establishing safety guarantees for deep learning models.
In the critical domain of medical applications, the repercussions of model decisions directly influence patient outcomes, emphasizing the quintessential need for reliability and trust. My endeavors in refining uncertainty estimation anchor in ensuring AI models not merely predict but also adeptly quantify and articulate the confidence, or lack thereof, in those predictions. This provides a safeguard, mitigating the risk of overconfident decisions, and securing the reliability of AI-derived medical decisions.
Interpretability in AI, especially in the medical sector, serves as another cornerstone of my research. Demystifying the complex, often opaque decision-making processes of AI models ensures that the resultant technologies are not black boxes, but transparent entities. This transparency is imperative, allowing healthcare professionals to comprehend and trust the decisions made by AI, ensuring that these technologies serve as supportive, clear, and reliable tools in clinical environments. My research thus serves to establish AI as an ally to healthcare professionals, enhancing rather than complicating their decision-making processes.
Furthermore, my research in weaving expert prior knowledge into the fabric of AI model training elucidates the path to creating AI systems deeply rooted in and informed by existing human expertise. This fusion of machine learning and expert knowledge gives rise to models that are technologically sophisticated while also being contextually informed, thereby enhancing their real-world applicability, performance, and safety.
Finally, along the theoretical axis, the development of PAC-Bayesian generalization guarantees for deep learning models in my research aims to underpin their empirical successes with a solid theoretical foundation. PAC-Bayesian frameworks provide a methodology to establish probabilistic bounds on the generalization errors of models, thereby offering a structured pathway to explore and validate the reliability and robustness of deep learning models, especially crucial in high-stakes fields like healthcare.
In essence, my research is a confluence of the theoretical and practical, ensuring AI systems are not only remarkably intelligent but also demonstrably safe, transparent, and reliable. My focus remains firmly embedded in ensuring that as AI systems weave into our societal and structural fabric, especially in vital sectors like healthcare, they bring along inherent qualities essential to safeguard and enhance our collective future, entwining advanced technology and safety in a cohesive, beneficial synthesis.