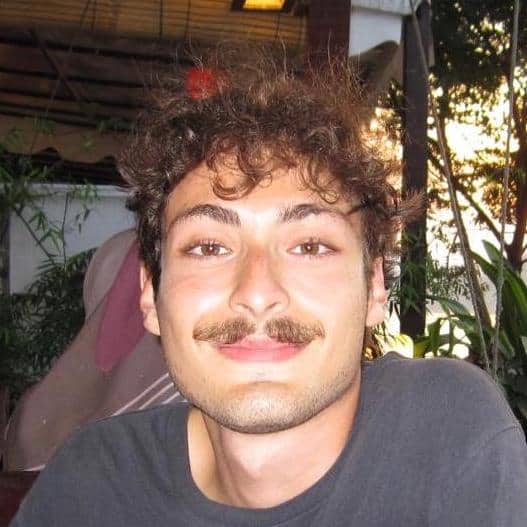
Stephane Hatgis-Kessell
Why do you care about AI Existential Safety?
I enjoy critically analyzing prior work, identifying nuances in prior results, and then formulating and tackling open problems. My enthusiasm for the research process and my keenness for building technology that benefits society have led to my interest in researching Existential Safety methods during my Ph.D. I believe that the intelligent agents set to be deployed soon, and perhaps even current decision-making systems, present an existential risk to humanity if proper precautions are not taken. While organizations and governments are developing AI regulatory frameworks and ethics principles to lay the foundations for these precautions, a pressing gap remains between these goals and actionable methodologies.
Please give at least one example of your research interests related to AI existential safety:
Problem Description: When training reinforcement learning (RL) agents, incorrectly specified reward functions – or those that are unaligned with the desires of human stakeholders – can lead to catastrophic failures. For example, a reward function for a vacuum cleaning robot that encodes the desire to “maximize dust collected off the floor” seems sensible but may result in a robot that dumps dust onto the floor in order to immediately pick it up again. Manually specifying reward functions limits the utility of RL systems and may pose dangers to end users or even existential risks. Instead, I intend to develop methodologies for both learning human-aligned reward functions and detecting unaligned reward functions or behaviors in advance. My particular emphasis is on creating tools that enable the regulation of complex decision-making systems.
Scope: The problem of incorrectly specified objectives plagues any optimization framework. In RL, this objective is encoded via a reward function. While I focus on aligning reward functions with human values, my solutions will likely be extendable to many other areas.
Proposal: I contend that learning aligned objectives and detecting unaligned behaviors should be an iterative, human-in-the-loop process. I aim to develop a collaborative framework that involves two distinct, repeating interactions: using human feedback to learn a reward function and enabling the human(s) to detect misalignment during training. This framework reframes the objective learning problem as a collaborative human-agent task. While I identify these challenges, I would be thrilled to contribute to any project aimed at enhancing the safety and autonomy of decision-making systems.