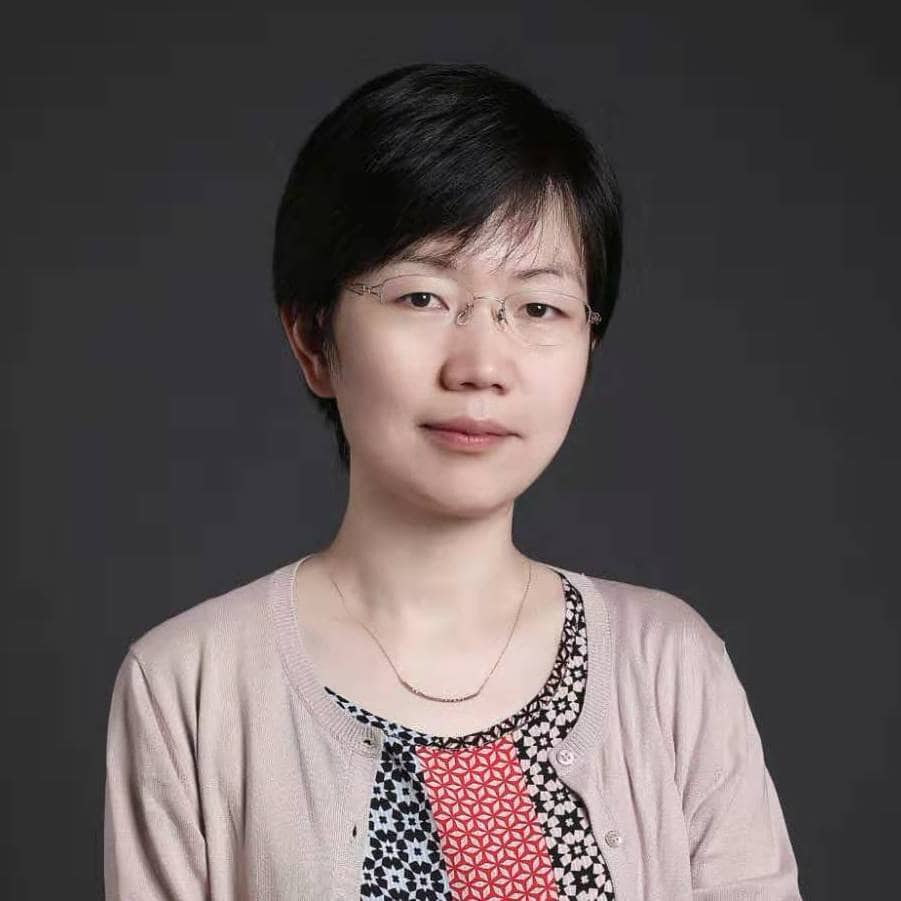
Qian Tao
Why do you care about AI Existential Safety?
I had extensive experience working in academic hospitals, where I interacted with clinicians and came across patients on a daily basis. In the clinical world, decisions were made by clinicians, through conversions with patients/families, examinations (e.g. radiology, pathology, genetics), and consultation with clinicians across departments – often a complicated, concerted effort. Practicing clinicians have gone through long professional training, which entails not only profound knowledge and hands-on practice, but also the solemn oath to respect human life, ethics, and the discipline of medicine. Both are fundamental to our trust in doctors. The recent surge of AI is transformative: in many fictions/prophecies, AI is to take over doctors, because it can be more knowledgeable and meticulous than any human being, especially with large models. This leads to my concern about AI’s path of deployment in healthcare, and more generally about existential safety. While AI is centered on optimization, healthcare transcends mere optimization, emphasizing human values. Without vigilant oversight, AI might prioritize optimization at the expense of trust fundamental to our healthcare systems.
Please give at least one example of your research interests related to AI existential safety:
I design trustworthy AI methods that harness the power of computation and data to solve real-world clinical problems, from diagnosis to intervention. I can provide two examples of my current research related to AI existential safety:
1. Uncertainty of AI prediction
I approach trustworthiness from the lens of uncertainty. We use the classical Bayesian inference framework but adapt it to be computationally tractable for huge AI models. The idea is to infer the posterior probability distributions of the AI prediction. If there is a large entropy, it implies high uncertainty, and the results need to be carefully checked by human experts. We have very exciting results showing that our uncertainty estimation is very effective in detecting MRI segmentation errors by a trained AI model, while run through massive population datasets. This research also addresses the ‘over-confidence’ issue of deep learning, which is one of the fundamental threats to safety.
2. Inductive Bias into AI
A second example is my ongoing research on inductive bias. Designing an efficient way to instil inductive bias into AI is crucial to overcoming the unexplainable error typical of the purely data-drive AI black boxes. Learning rules and principles from data is far from trivial, even with extremely large models and datasets. With proper regularization, which encodes prior knowledge (e.g., human anatomy, epidemiological distribution, etc.), we can more effectively ensure AI-generated predictions’ plausibility. A simple example is that we encode the heart anatomical model to remove wrong AI predictions of heart shape from artefact-corrupted MRI exams.
Collectively, the two research trajectories — viewed from both posterior and prior perspectives — underscore my belief that, by integrating classical statistical learning theories, we can imbue AI with certain mathematical rigor. This will be instrumental in addressing unforeseen risks, thus boosting the intrinsic safety of AI. I anticipate the opportunity to collaborate with the Future of Life community, to advance the frontier with like-minded, forward-thinking scientists.