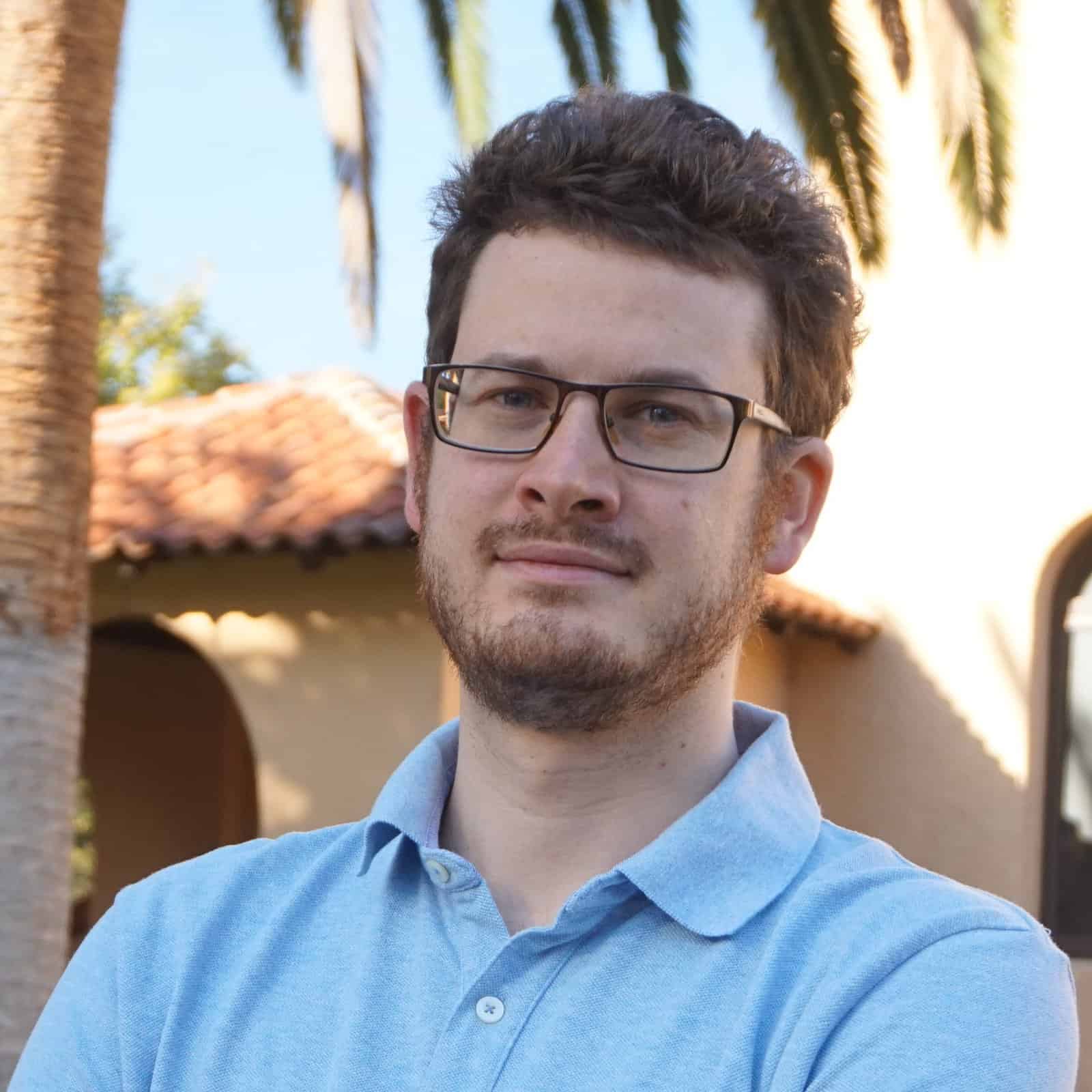
Florian Tramer
Why do you care about AI Existential Safety?
Recent years have shown that AI systems can exhibit a sort of threshold behavior, with a sudden emergence of new capabilities. We can thus go from systems that are nearly useless to possible wide-range deployments in a very short time frame. This makes it hard to predict what capabilities AI systems might have in the near-future. It is thus important to start thinking about existential safety now, and to canvas possible ways of identifying and mitigating such threats before they fully manifest. At the same time, research on AI existential safety can already be useful today. Current AI systems already pose several risks. While these risks are not necessarily existential in nature, they can still pose serious harms to society (e.g., automated weapons, misinformation, increased inequality, etc). By studying ways to “stifle” AI systems we might be able to address some of these contemporary issues. while simultaneously laying the groundwork for understanding and preventing existential threats that may arise in the future.
Please give one or more examples of research interests relevant to AI existential safety:
How can we adequately measure the performance and robustness of AI systems with super-human capabilities? Concretely, how would we create a “test set”, if we don’t even know how to label the data? It seems clear that AI systems that pose existential threats will have some kind of superhuman abilities (indeed, no sole human seems to have ever posed an existential threat to civilization). It thus is important to think about how we would even evaluate such systems. – How do we enable AI systems to be audited by external parties (e.g., “white hats”) for security flaws, misalignment, etc? AI systems that are likely to pose existential threats will probably be created by groups outside of public scrutiny. As we see time and time again in computer security, such public scrutiny is actually incredibly important for surfacing severe flaws and vulnerabilities. How can we design AI systems so as to facilitate such external auditability? – can we define “security boundaries” for machine learning systems, in the same way as for other computer systems? That is, can we design systems that have certain capabilities but that cannot access others? Can we design AI systems that keep other AI systems in check, or does this simply “kick the can down the road”?