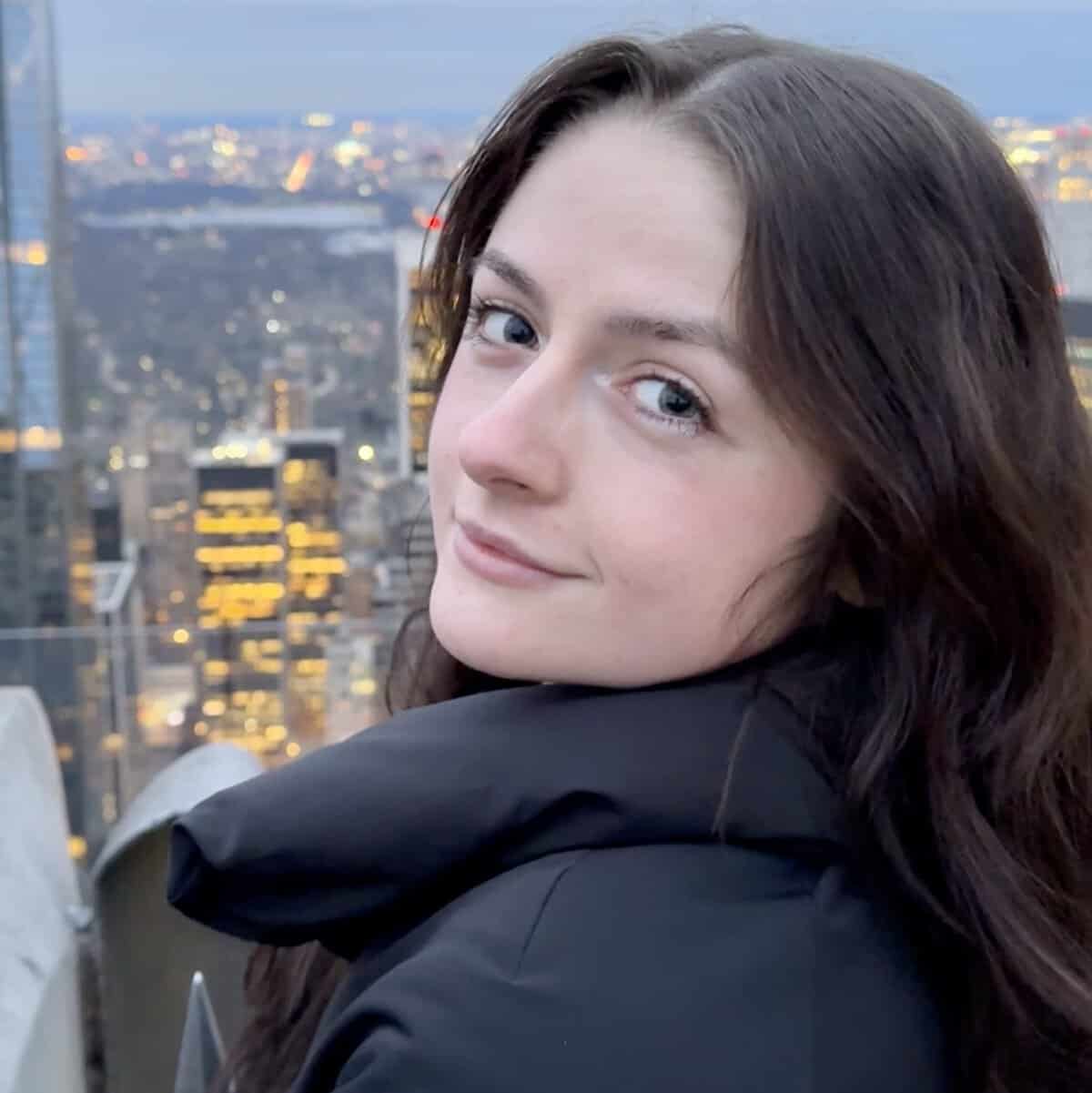
Stephanie Milani
Why do you care about AI Existential Safety?
The rapid advancement of AI capabilities raises profound questions about the long-term impacts on humanity. As we develop AI systems that can not only surpass human-level performance but are also deployed in both physical and virtual spaces, it becomes essential to ensure that these systems are aligned with human values and interests. This alignment problem is non-trivial, as human values are complex, diverse, and often conflicting. Another critical aspect of ensuring AI existential safety is interpretability. As AI systems become more complex, it’s crucial that we maintain the ability to understand and audit their decision-making processes. By focusing on interpretability and alignment, we can work towards creating AI systems that not only avoid catastrophic risks but also help us build a better future for all of humanity.
Please give at least one example of your research interests related to AI existential safety:
One of my key research interests related to AI existential safety is developing methods for learning from human feedback to align AI systems with human intentions and values. This is exemplified by my work on the MineRL BASALT (Benchmark for Agents that Solve Almost-Lifelike Tasks) Competition and Dataset. The BASALT competition and dataset focus on training AI agents to perform fuzzy tasks in the game Minecraft based solely on human demonstrations and feedback. This work is crucial for AI existential safety for several reasons:
- Alignment with human intentions: Traditional reinforcement learning often relies on pre-defined reward functions, which can lead to misaligned behavior when scaled to more complex environments. By learning directly from human demonstrations and feedback, we can create AI systems that better understand and align with human intentions, even for tasks that are difficult to specify formally.
- Handling open-ended tasks: Many real-world scenarios involve open-ended goals that are challenging to define mathematically. The BASALT framework provides a testbed for developing AI systems that can understand and pursue fuzzy, underspecified objectives – a critical capability for safe and beneficial advanced AI.
Separately, I also work on interpretability and AI for social good applications.