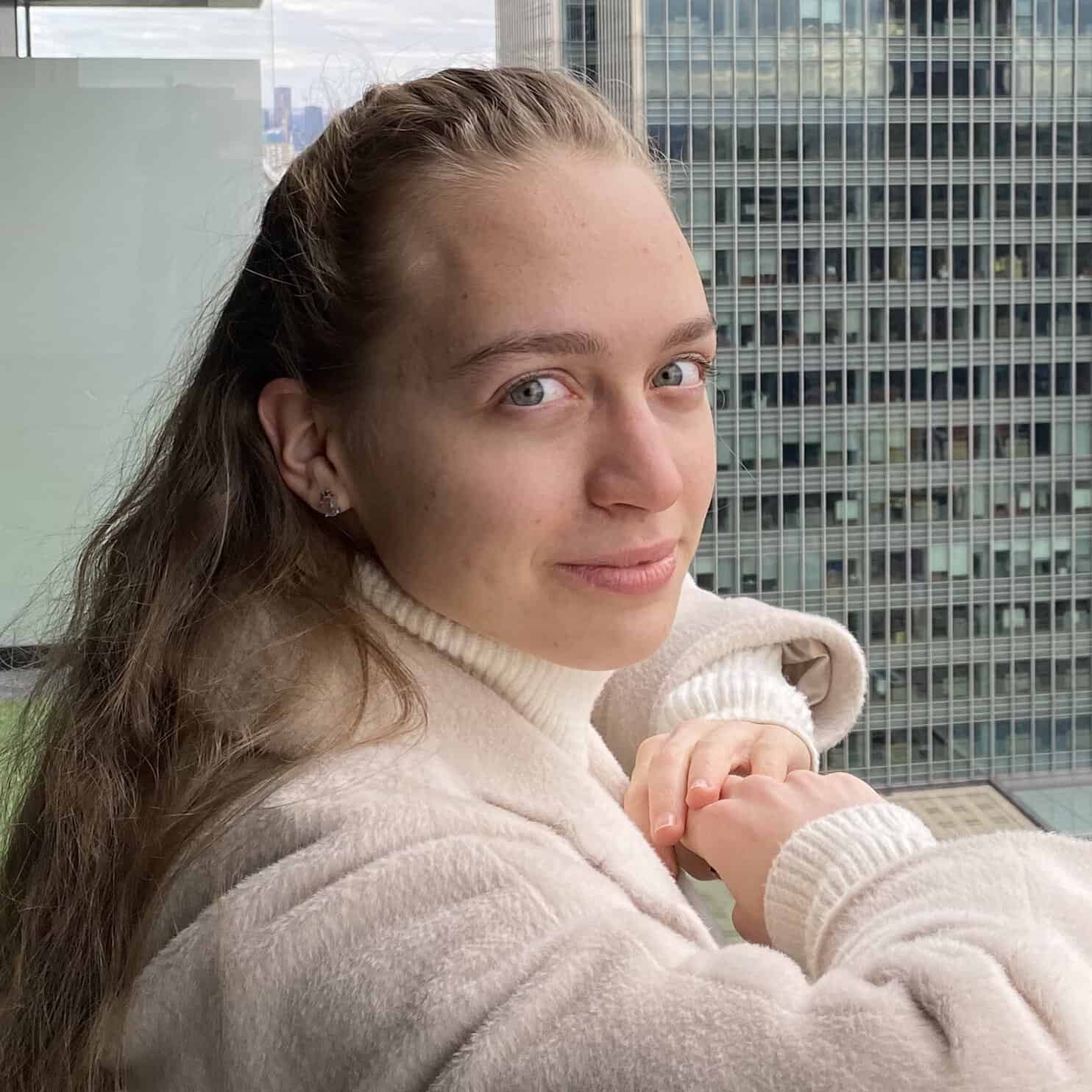
Liza Tennant
Why do you care about AI Existential Safety?
I am a PhD researcher focused on developing methods to build moral alignment into the core of AI systems. While many researchers are working on advancing task-specific capabilities in AI models (e.g., improving on baselines), I believe it is essential to address a more fundamental dimension of intelligence – social and moral reasoning, so that when these models get deployed, they do not cause collateral damage to human society.
* I believe that working on building morality into systems is essential to building robust aligned AI systems in the future, especially as the end product becomes less and less interpretable by human researchers, and controlled post-hoc fine-tuning becomes less feasible.
* This is why day-to-day I work on technical implementations of ideas from moral philosophy & psychology into learning agents (i.e., simulated models of future adaptive AI systems). My research so far has centered around Multi-Agent Reinforcement Learning simulations and moral fine-tuning of Language Models.
Please give at least one example of your research interests related to AI existential safety:
My best work so far is my PhD research on developing moral reasoning in learning agents. I have summarised this in 1 conceptual paper and 1 published experimental paper. In the conceptual paper (see ‘AI Safety Manifesto’ pdf attached), we analyse the space of approaches to moral alignment on a spectrum from fully top-down imposed rules (e.g. logic-based rules or constrained learning) to the more recent fully bottom-up inferred values (e.g. RLHF & Inverse RL). After reviewing existing works along this continuum, we argue that the middle of this range (i.e., a hybrid space) is too sparsely populated, and motivate the use of a combination of interpretable top-down quantitative definitions of moral objectives, based on existing frameworks in fields such as Moral Philosophy / Economics / Psychology, with the bottom-up advantages of trial-and-error learning from experience via RL. This hybrid methodology provides a powerful way of studying and imposing control on an AI system while enabling flexible adaptation to dynamic environments. We review 3 case studies combining moral principles with learning (namely, RL in social dilemmas with intrinsic rewards, safety-shielded RL, & Constitutional AI), providing a proof-of-concept for the potential of this hybrid approach in creating more prosocial & cooperative agents. My experimental paper then implements the intrinsic rewards method in simulated RL agents, demonstrating relative pros and cons of learning via different moral frameworks in multi-agent social dilemmas. I next plan to extend insights from this work towards implementing moral preferences in LLM-based agents.
Links:
* conceptual paper
* experimental paper, code & video