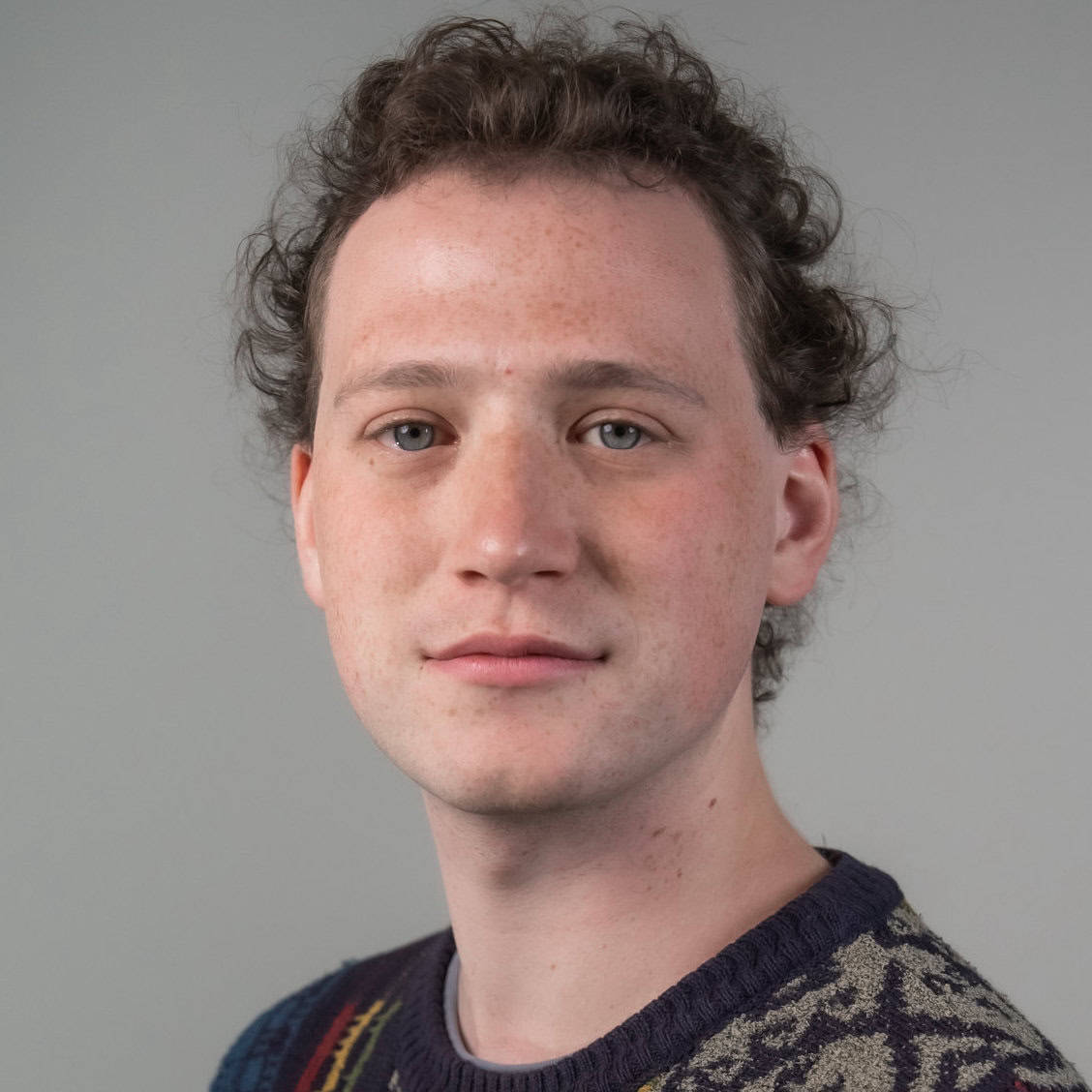
Felix Binder
Why do you care about AI Existential Safety?
In my undergraduate degree in philosophy and computer science I was engaging with arguments on existential risks from superintelligent AI systems beginning in about 2015. I have followed the literature and arguments closely since then. The topic of my PhD—agent-environment interactions during planning—was chosen with regard to the safety of deep RL agents (which seemed like the dominant paradigm in AI in 2019). Since then, the urgency of aligning AI has increased. In short, I believe that the future might be extremely good, but that the chance that all future value will be lost is considerable (~40% in this century), and I want to do what I can to ensure that the long-term flourishing of humanity is ensured.
I have a good understanding of the field, having completed the AGI Safety Course, attended philosophy courses on existential risk, organized groups and journal clubs on the topic and engage with researchers in the field.
Please give at least one example of your research interests related to AI existential safety:
My broad interest in AI safety is best described as high-level interpretability/evaluations: constructing experiments to elicit behaviors that can tell us about the inner workings of frontier models even if the inner states of the model are hard to interpret. An ability that I care about (and that I am an expert in) is planning: search over a world model to come up with intelligent actions in never-before-seen situations.
I have studied this in the context of agents embedded in a physical environment in my PhD (see my publications).
There, I investigated how the visual structure of the environment might allow for subgoal decomposition, a crucial puzzle piece in the ability to plan efficiently. In a simulated physical environment, agents with different planning strategies built towers, demonstrating that visual subgoal decomposition can indeed mitigate the computational demands of planning (paper). Behavioral experiments show that humans on the same task engage in visual subgoal decomposition, and that their subgoal choices are explained by planning cost (paper—full writeup is in preparation).
Planning requires a model of the world. To understand human and AI physical world models, I contributed in the creation of a large dataset and ran a benchmarking study to test AI models physical reasoning using ThreeDWorld. This work—Physion—was accepted at NeurIPS. The comparison to humans utilizes the Cognitive AI Benchmarking framework, which I maintain. I co-organized a workshop on Cognitive AI Benchmarking, which develops best practices around comparing the behavior of humans and AI systems with a focus on representational alignment: the degree to which humans and AIs operate on similar mental representations.
Currently, I am interested in understanding how planning and reasoning in LLMs can be investigated and supervised.
I recently developed and ran an evaluation of steganography in AI. Following this, I am working on investigating which optimization pressures might lead to the emergence of planning behaviors in models without explicit search over a world model built-in.