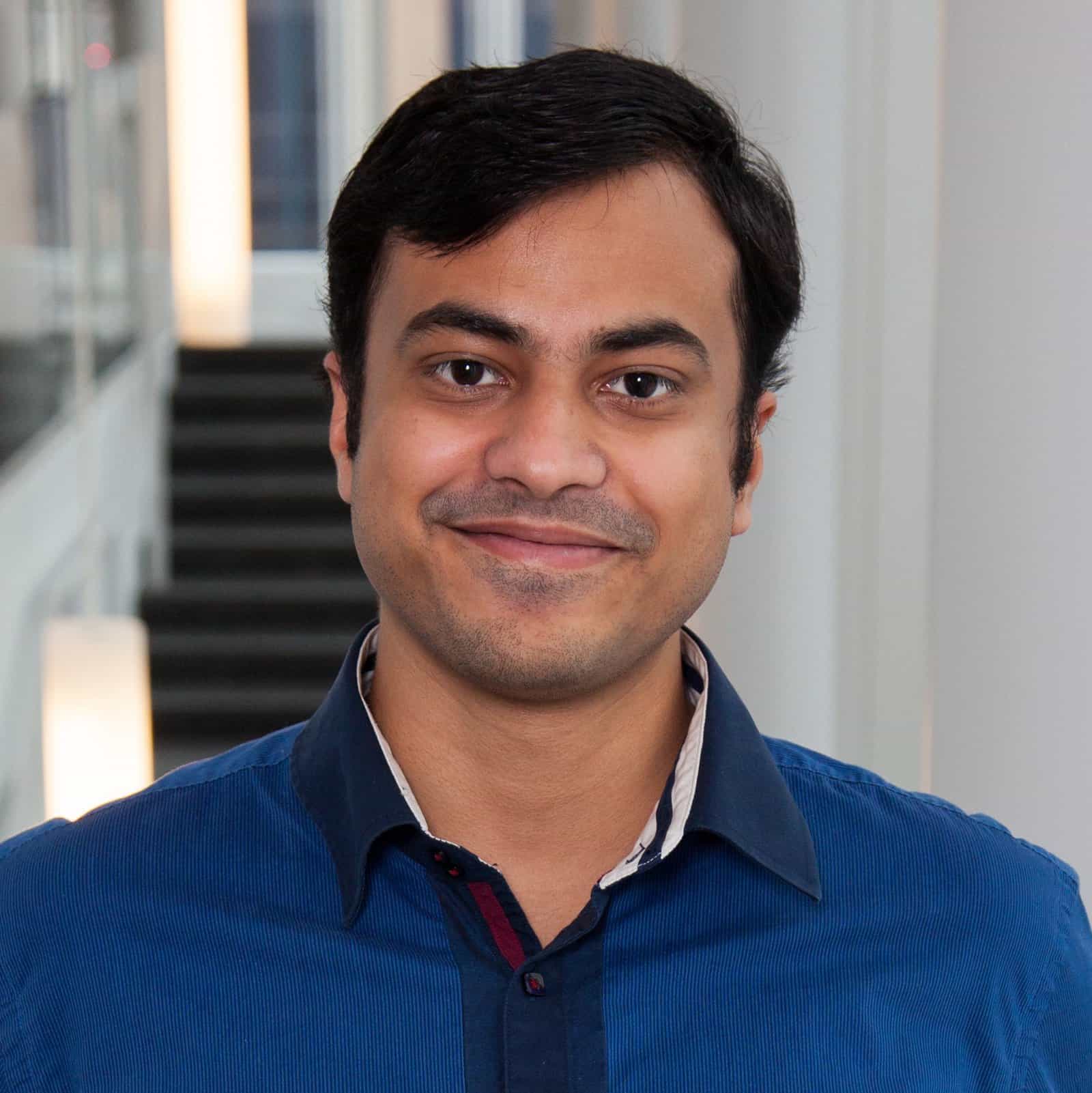
Arnob Ghosh
Why do you care about AI Existential Safety?
AI is going to be part of the decision-making in many real-life applications. Hence, it is important to develop fair and safe AI-decision making. Without safety guarantee, it might be even dangerous to implement AI in real-life applications. For example, consider the Large language models which can generate very good response, and even codes. However, how can one ensure that those responses are safe, and fair? Hence, it is very important to consider safety and fairness alongside building accurate models. As an second example, AI is being now used in robots, and drones. The question is how can you ensure that the trajectories can be safe. I am interested in developing AI algorithms while it can be safe.
Please give at least one example of your research interests related to AI existential safety:
My current research lies in safe Reinforcement Learning or constrained Markov Decision Process (CMDP). Many constrained sequential decision-making processes such as safe AV navigation, fair multi-agent learning, wireless network control, safe transportation control, safe edge-computing etc., can be cast as CMDP. Reinforcement Learning (RL) algorithms have been used to learn optimal policies for unknown unconstrained MDP. Extending these RL algorithms to unknown CMDP, brings the additional challenge of not only maximizing the reward but also satisfying the constraints. My research lies in providing theoretical guarantees on developing RL algorithm which can ensure safety as well maximize the objective. My research interest lies in both online and offline RL algorithm development. Recently, I developed the first model-free RL algorithm for large-state space with provable sample complexity guarantee. In particular, our developed algorithm guarantees feasibility guarantee as well optimality guarantee and can achieve that using the smallest possible samples. Hence, my proposed algorithm is achievable even using small number of samples. My research also revolves around developing algorithm which can adapt to the non-stationarity a well. Towards this end, I developed the first safe RL algorithm which can provably adapt to the non-stationarity in the MDP. I also developed the first safe offline RL algorithm with provable performance guarantee which can have tremendous impact as it can generate safe algorithm only using offline database. Finally, recently, I am also involved in developing distributionally safe RL algorithm which can learn algorithms even with high-probability for practical setup.
In terms of application, my research also concerns about developing safe RL algorithm for safe robot navigation. In this regard, I am collaborating with the Ohio State University in implementing such safe RL algorithms in practical setup. In this regard, we are trying to develop algorithms which will not violate safety even during training. With the collaboration of the Northeastern University, we are developing the safe beam forming algorithm which can identify the directions for sending signal fast and yet can minimize the interference at the neighboring terminal. Further, such proposed approach can adapt to the time-varying channel condition. Hence, our approach can be applicable in next generation wireless network safely.