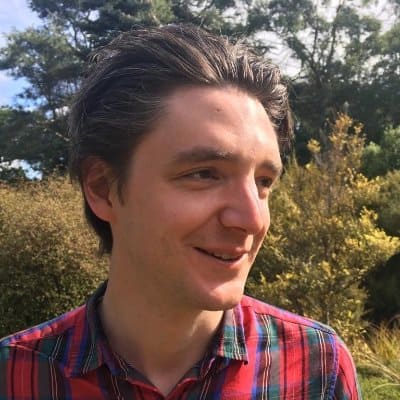
Laurence Aitchison
Why do you care about AI Existential Safety?
AI safety is a subject of profound importance to me because I am deeply invested in work that maximizes human wellbeing. The rapid advancements in artificial intelligence bring us closer to achieving strong AI, almost certainly within our lifetime. This transformative technology promises to revolutionize every aspect of society, from healthcare and education to transportation and economics. However, with such monumental potential comes significant risk. If we do not approach the development and deployment of AI with careful consideration, the consequences could be dire, impacting the trajectory of human history in unpredictable and harmful ways.
Please give at least one example of your research interests related to AI existential safety:
My research interests lie at the intersection of AI existential safety and various machine learning methods. On the theoretical side, I am deeply interested in understanding how neural networks do what they do, and these interests range from research on how networks learn representations in infinite-width networks through to mechanistic interpretability with sparse auto encoders. On the more practical side, I am interested in how to design robust instruction fine-tuning schemes, perhaps involving Bayesian inference to reason about uncertainty.
The potential for artificial intelligence to pose existential risks to humanity is a critical concern that drives my research interests. One approach to addressing these risks is to understand the operation of these networks, so we can potentially discern how and when they may begin to present safety risks. My work in this area extends back to work on the “deep kernel process/machine” programme, which gives a strong theoretical understanding of how infinite, Bayesian neural networks learn representations. At present, my work in this area extends to extending the sparse auto-encoder approach to mechanistic interpretability to obtain an understanding of the full network, and not just isolated circuits.
In the near term, perhaps the most promising approach to AI safety is instruction tuning. I am deeply interested in improving the accuracy, robustness and generalisation in instruction tuning. One approach that my group is pursuing is to leverage Bayesian uncertainty estimation so that reward models understand the regions where they have lots of data, and can therefore be certain about their reward judgements, from regions where they have little data and can be less certain. These uncertain regions may correspond to regions where generalisation is poor, or even flag adversarial inputs. The fine tuned network can then be steered away from responding in these potentially dangerous regions.