Policymaking In The Pause
What can policymakers do now to combat risks from advanced AI systems?
Our open letter calling for a six-month pause on giant AI experiments sparked a global debate on the benefits and risks of continuing the development of increasingly powerful AI models. This paper investigates what opportunities policymakers might have if such a pause were to happen.
Contents
“The time for saying that this is just pure research has long since passed. […] It’s in no country’s interest for any country to develop and release AI systems we cannot control. Insisting on sensible precautions is not anti-industry. Chernobyl destroyed lives, but it also decimated the global nuclear industry. I’m an AI researcher. I do not want my field of research destroyed. Humanity has much to gain from AI, but also everything to lose.”
Stuart Russell, Smith-Zadeh Chair in Engineering and Professor of Computer Science at the University of California, Berkeley, Founder of the Center for Human Compatible Artificial Intelligence (CHAI).
“Let’s slow down. Let’s make sure that we develop better guardrails, let’s make sure that we discuss these questions internationally just like we’ve done for nuclear power and nuclear weapons. Let’s make sure we better understand these very large systems, that we improve on their robustness and the process by which we can audit them and verify that they are safe for the public.”
Yoshua Bengio, Scientific Director of the Montreal Institute for Learning Algorithms (MILA), Professor of Computer Science and Operations Research at the Université de Montréal, 2018 ACM A.M. Turing Award Winner.
“We have a perfect storm of corporate irresponsibility, widespread adoption, lack of regulation and a huge number of unknowns. shows how many people are deeply worried about what is going on. I think it is a really important moment in the history of AI – and maybe humanity,”
Gary Marcus, Professor Emeritus of Psychology and Neural Science at New York University, Founder of Geometric Intelligence
“It feels like we are moving too quickly. I think it is worth getting a little bit of experience with how they can be used and misused before racing to build the next one. This shouldn’t be a race to build the next model and get it out before others.”
Peter Stone, Professor at the University of Texas at Austin, Chair of the One Hundred Year Study on AI.
“We don’t know what these systems are trained on or how they are being built. All of this happens behind closed doors at commercial companies. This is worrying.”
Catelijne Muller, President of ALLAI, Member of the EU High Level Expert Group on AI
“Those making these have themselves said they could be an existential threat to society and even humanity, with no plan to totally mitigate these risks. It is time to put commercial priorities to the side and take a pause for the good of everyone to assess rather than race to an uncertain future”
Emad Mostaque, Founder and CEO of Stability AI
Introduction
Prominent AI researchers have identified a range of dangers that may arise from the present and future generations of advanced AI systems if they are left unchecked. AI systems are already capable of creating misinformation and authentic-looking fakes that degrade the shared factual foundations of society and inflame political tensions.1 AI systems already show a tendency toward amplifying entrenched discrimination and biases, further marginalizing disadvantaged communities and diverse viewpoints.2 The current, frantic rate of development will worsen these problems significantly.
As these types of systems become more sophisticated, they could destabilize labor markets and political institutions, and lead to the concentration of enormous power in the hands of a small number of unelected corporations. Advanced AI systems could also threaten national security, e.g., by facilitating the inexpensive development of chemical, biological, and cyber weapons by non-state groups. The systems could themselves pursue goals, either human- or self-assigned, in ways that place negligible value on human rights, human safety, or, in the most harrowing predictions, human existence.3
In an effort to stave off these outcomes, the Future of Life Institute (FLI), joined by over 15,000 leading AI researchers, professors, CEOs, engineers, students, and others on the frontline of AI progress, called for a pause of at least six months on the riskiest and most resource-intensive AI experiments – those experiments seeking to further scale up the size and general capabilities of the most powerful systems developed to date.4
The proposed pause provides time to better understand these systems, to reflect on their ethical, social, and safety implications, and to ensure that AI is developed and used in a responsible manner. The unchecked competitive dynamics in the AI industry incentivize aggressive development at the expense of caution5. In contrast to the breakneck pace of development, however, the levers of governance are generally slow and deliberate. A pause on the production of even more powerful AI systems would thus provide an important opportunity for the instruments of governance to catch up with the rapid evolution of the field.
We have called on AI labs to institute a development pause until they have protocols in place to ensure that their systems are safe beyond a reasonable doubt. Regardless of whether the labs will head our call, this policy brief provides policymakers with concrete recommendations for how governments can manage AI risks.
The recommendations are by no means exhaustive: the project of AI governance is a long game and will extend far beyond any pause. Nonetheless, implementing these recommendations, which largely reflect a broader consensus among AI policy experts, will establish a strong governance foundation for AI.
Policy recommendations
- Mandate robust third-party auditing and certification.
- Regulate access to computational power.
- Establish capable AI agencies at the national level.
- Establish liability for AI-caused harms.
- Introduce measures to prevent and track AI model leaks.
- Expand technical AI safety research funding.
- Develop standards for identifying and managing AI-generated content and recommendations.
To coordinate, collaborate, or inquire regarding the recommendations herein, please contact us at policy@futureoflife.org.
1. Mandate robust third-party auditing and certification for specific AI systems
For some types of AI systems, the potential to impact the physical, mental, and financial wellbeing of individuals, communities, and society is obvious. For example, a credit scoring system could discriminate against certain ethnic groups. For other systems – in particular general-purpose AI systems6 – the applications and potential risks are often not immediately evident. General-purpose AI systems trained on massive datasets also have unexpected (and often unknown) emergent capabilities.7
In Europe, the draft AI Act already requires that, prior to deployment and upon any substantial modification, ‘high-risk’ AI systems undergo ‘conformity assessments’ in order to certify compliance with specified harmonized standards or other common specifications.8 In some cases, the Act requires such assessments to be carried out by independent third-parties to avoid conflicts of interest.
In contrast, the United States has thus far established only a general, voluntary framework for AI risk assessment.9 The National Institute of Standards and Technology (NIST) is developing so-called ‘profiles’ that will provide specific risk assessment and mitigation guidance for certain types of AI systems, but these profiles still allow organizations simply to ‘accept’ the risks that they create for society instead of addressing them. In other words, the United States does not require any third-party risk assessment or risk mitigation measures before a powerful AI system can be deployed at scale.
To ensure proper vetting of powerful AI systems before deployment, we recommend a robust independent auditing regime for models that are general-purpose, trained on large amounts of compute, or intended for use in circumstances likely to impact the rights or the wellbeing of individuals, communities, or society. This mandatory third-party auditing and certification scheme could be derived from the EU’s proposed ‘conformity assessments’ and should be adopted by jurisdictions worldwide10.
In particular, we recommend third-party auditing of such systems across a range of benchmarks for the assessment of risks11, including possible weaponization12 and unethical behaviors13 and mandatory certification by accredited third-party auditors before these high-risk systems can be deployed. Certification should only be granted if the developer of the system can demonstrate that appropriate measures have been taken to mitigate risk, and that any residual risks deemed tolerable are disclosed and are subject to established protocols for minimizing harm.
2. Regulate organizations’ access to computational power
At present, the most advanced AI systems are developed through training that requires an enormous amount of computational power – ‘compute’ for short. The amount of compute used to train a general-purpose system largely correlates with its capabilities, as well as the magnitude of its risks.
Today’s most advanced models, like OpenAI’s GPT-4 or Google’s PaLM, can only be trained with thousands of specialized chips running over a period of months. While chip innovation and better algorithms will reduce the resources required in the future, training the most powerful AI systems will likely remain prohibitively expensive to all but the best-resourced players.
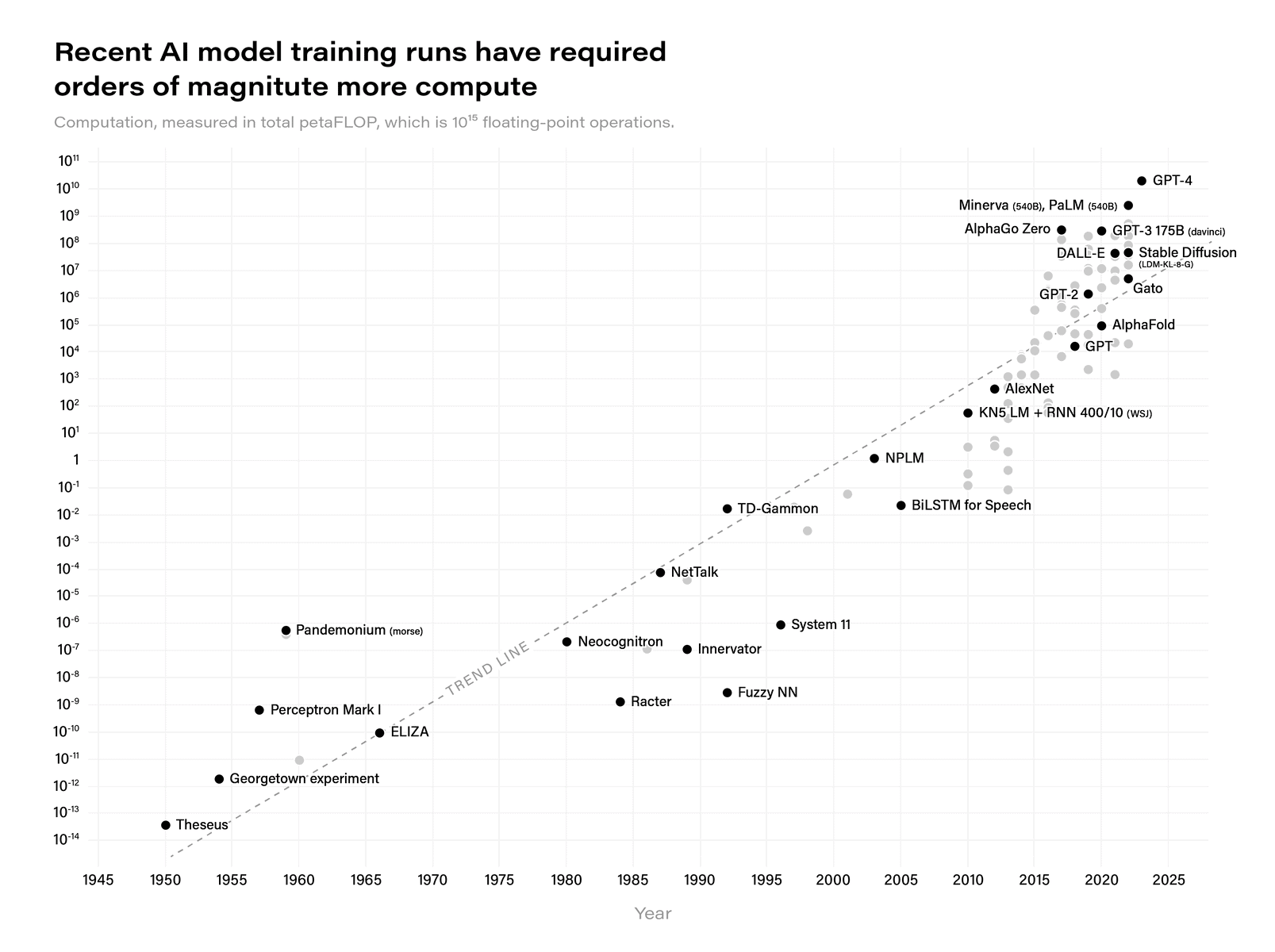
In practical terms, compute is more easily monitored and governed than other AI inputs, such as talent, data, or algorithms. It can be measured relatively easily and the supply chain for advanced AI systems is highly centralized, which means governments can leverage such measures in order to limit the harms of large-scale models.14
To prevent reckless training of the highest risk models, we recommend that governments make access to large amounts of specialized computational power for AI conditional upon the completion of a comprehensive risk assessment. The risk assessment should include a detailed plan for minimizing risks to individuals, communities, and society, consider downstream risks in the value chain, and ensure that the AI labs conduct diligent know-your-customer checks.
Successful implementation of this recommendation will require governments to monitor the use of compute at data centers within their respective jurisdictions.14.5 The supply chains for AI chips and other key components for high-performance computing will also need to be regulated such that chip firmware can alert regulators to unauthorized large training runs of advanced AI systems.15
Through passage of the CHIPS and Science Act of 2022, the United States has instituted licensing requirements15.5 for export of many of these components in an effort to monitor and control their global distribution. However, licensing is only required when exporting to certain destinations, limiting the capacity to monitor aggregation of equipment for unauthorized large training runs within the United States and outside the scope export restrictions. Companies within the specified destinations have also successfully skirted monitoring by training AI systems using compute leased from cloud providers.16 We recommend expansion of know-your-customer requirements to all high-volume suppliers for high-performance computing components, as well as providers that permit access to large amounts of cloud compute.
3. Establish capable AI agencies at national level
AI is developing at a breakneck pace and governments need to catch up. The establishment of AI regulatory agencies helps to consolidate expertise and reduces the risk of a patchwork approach.
The UK has already established an Office for Artificial Intelligence and the EU is currently legislating for an AI Board. Similarly, in the US, Representative Ted Lieu has announced legislation to create a non-partisan AI Commission with the aim of establishing a regulatory agency. These efforts need to be sped up and taken up around the world.
We recommend that national AI agencies be established in line with a blueprint17 developed by Anton Korinek at Brookings. Korinek proposes that an AI agency have the power to:
- Monitor public developments in AI progress and define a threshold for which types of advanced AI systems fall under the regulatory oversight of the agency (e.g. systems above a certain level of compute or that affect a particularly large group of people).
- Mandate impact assessments of AI systems on various stakeholders, define reporting requirements for advanced AI companies and audit the impact on people’s rights, wellbeing, and society at large. For example, in systems used for biomedical research, auditors would be asked to evaluate the potential for these systems to create new pathogens.
- Establish enforcement authority to act upon risks identified in impact assessments and to prevent abuse of AI systems.
- Publish generalized lessons from the impact assessments such that consumers, workers and other AI developers know what problems to look out for. This transparency will also allow academics to study trends and propose solutions to common problems.
Beyond this blueprint, we also recommend that national agencies around the world mandate record-keeping of AI safety incidents, such as when a facial recognition system causes the arrest of an innocent person. Examples include the non-profit AI Incident Database and the forthcoming EU AI Database created under the European AI Act.18
4. Establish liability for AI-caused harm
AI systems present a unique challenge in assigning liability. In contrast to typical commercial products or traditional software, AI systems can perform in ways that are not well understood by their developers, can learn and adapt after they are sold and are likely to be applied in unforeseen contexts. The ability for AI systems to interact with and learn from other AI systems is expected to expedite the emergence of unanticipated behaviors and capabilities, especially as the AI ecosystem becomes more expansive and interconnected.
Several plug-ins have already been developed that allow AI systems like ChatGPT to perform tasks through other online services (e.g. ordering food delivery, booking travel, making reservations), broadening the range of potential real-world harms that can result from their use and further complicating the assignment of liability.19 OpenAI’s GPT-4 system card references an instance of the system explicitly deceiving a human into bypassing a CAPTCHA bot-detection system using TaskRabbit, a service for soliciting freelance labor.20
When such systems make consequential decisions or perform tasks that cause harm, assigning responsibility for that harm is a complex legal challenge. Is the harmful decision the fault of the AI developer, deployer, owner, end-user, or the AI system itself?
Key among measures to better incentivize responsible AI development is a coherent liability framework that allows those who develop and deploy these systems to be held responsible for resulting harms. Such a proposal should impose a financial cost for failing to exercise necessary diligence in identifying and mitigating risks, shifting profit incentives away from reckless empowerment of poorly-understood systems toward emphasizing the safety and wellbeing of individuals, communities, and society as a whole.
To provide the necessary financial incentives for profit-driven AI developers to exercise abundant caution, we recommend the urgent adoption of a framework for liability for AI-derived harms. At a minimum, this framework should hold developers of general-purpose AI systems and AI systems likely to be deployed for critical functions21 strictly liable for resulting harms to individuals, property, communities, and society. It should also allow for joint and several liability for developers and downstream deployers when deployment of an AI system that was explicitly or implicitly authorized by the developer results in harm.
5. Introduce measures to prevent and track AI model leaks
Commercial actors may not have sufficient incentives to protect their models, and their cyberdefense measures can often be insufficient. In early March 2023, Meta demonstrated that this is not a theoretical concern, when their model known as LLaMa was leaked to the internet.22 As of the date of this publication, Meta has been unable to determine who leaked the model. This lab leak allowed anyone to copy the model and represented the first time that a major tech firm’s restricted-access large language model was released to the public.
Watermarking of AI models provides effective protection against stealing, illegitimate redistribution and unauthorized application, because this practice enables legal action against identifiable leakers. Many digital media are already protected by watermarking – for example through the embedding of company logos in images or videos. A similar process23 can be applied to advanced AI models, either by inserting information directly into the model parameters or by training it on specific trigger data.
We recommend that governments mandate watermarking for AI models, which will make it easier for AI developers to take action against illegitimate distribution.
6. Expand technical AI safety research funding
The private sector under-invests in research that ensures that AI systems are safe and secure. Despite nearly USD 100 billion of private investment in AI in 2022 alone, it is estimated that only about 100 full-time researchers worldwide are specifically working to ensure AI is safe and properly aligned with human values and intentions.24
In recent months, companies developing the most powerful AI systems have either downsized or entirely abolished their respective ‘responsible AI’ teams.25 While this partly reflects a broader trend of mass layoffs across the technology sector, it nonetheless reveals the relative de-prioritization of safety and ethics considerations in the race to put new systems on the market.
Governments have also invested in AI safety and ethics research, but these investments have primarily focused on narrow applications rather than on the impact of more general AI systems like those that have recently been released by the private sector. The US National Science Foundation (NSF), for example, has established ‘AI Research Institutes’ across a broad range of disciplines. However, none of these institutes are specifically working on the large-scale, societal, or aggregate risks presented by powerful AI systems.
To ensure that our capacity to control AI systems keeps pace with the growing risk that they pose, we recommend a significant increase in public funding for technical AI safety research in the following research domains:
- Alignment: development of technical mechanisms for ensuring AI systems learn and perform in accordance with intended expectations, intentions, and values.
- Robustness and assurance: design features to ensure that AI systems responsible for critical functions26 can perform reliably in unexpected circumstances, and that their performance can be evaluated by their operators.
- Explainability and interpretability: develop mechanisms for opaque models to report the internal logic used to produce output or make decisions in understandable ways. More explainable and interpretable AI systems facilitate better evaluations of whether output can be trusted.
In the past few months, experts such as the former Special Advisor to the UK Prime Minister on Science and Technology James W. Phillips27 and a Congressionally-established US taskforce have called for the creation of national AI labs as ‘a shared research infrastructure that would provide AI researchers and students with significantly expanded access to computational resources, high-quality data, educational tools, and user support.’28 Should governments move forward with this concept, we propose that at least 25% of resources made available through these labs be explicitly allocated to technical AI safety projects.
7. Develop standards for identifying and managing AI-generated content and recommendations
The need to distinguish real from synthetic media and factual content from ‘hallucinations’ is essential for maintaining the shared factual foundations underpinning social cohesion. Advances in generative AI have made it more difficult to distinguish between AI-generated media and real images, audio, and video recordings. Already we have seen AI-generated voice technology used in financial scams.29
Creators of the most powerful AI systems have acknowledged that these systems can produce convincing textual responses that rely on completely fabricated or out-of-context information.30 For society to absorb these new technologies, we will need effective tools that allow the public to evaluate the authenticity and veracity of the content they consume.
We recommend increased funding for research into techniques, and development of standards, for digital content provenance. This research, and its associated standards, should ensure that a reasonable person can determine whether content published online is of synthetic or natural origin, and whether the content has been digitally modified, in a manner that protects the privacy and expressive rights of its creator.
We also recommend the expansion of ‘bot-or-not’ laws that require disclosure when a person is interacting with a chatbot. These laws help prevent users from being deceived or manipulated by AI systems impersonating humans, and facilitate contextualizing the source of the information. The draft EU AI Act requires that AI systems be designed such that users are informed they are interacting with an AI system,31 and the US State of California enacted a similar bot disclosure law in 2019.32 Almost all of the world’s nations, through the adoption of a UNESCO agreement on the ethics of AI, have recognized33 ‘the right of users to easily identify whether they are interacting with a living being, or with an AI system imitating human or animal characteristics.’ We recommend that all governments convert this agreement into hard law to avoid fraudulent representations of natural personhood by AI from outside regulated jurisdictions.
Even if a user knows they are interacting with an AI system, they may not know when that system is prioritizing the interests of the developer or deployer over the user. These systems may appear to be acting in the user’s interest, but could be designed or employed to serve other functions. For instance, the developer of a general-purpose AI system could be financially incentivized to design the system such that when asked about a product, it preferentially recommends a certain brand, when asked to book a flight, it subtly prefers a certain airline, when asked for news, it provides only media advocating specific viewpoints, and when asked for medical advice, it prioritizes diagnoses that are treated with more profitable pharmaceutical drugs. These preferences could in many cases come at the expense of the end user’s mental, physical, or financial well-being.
Many jurisdictions require that sponsored content be clearly labeled, but because the provenance of output from complex general-purpose AI systems is remarkably opaque, these laws may not apply. We therefore recommend, at a minimum, that conflict-of-interest trade-offs should be clearly communicated to end users along with any affected output; ideally, laws and industry standards should be implemented that require AI systems to be designed and deployed with a duty to prioritize the best interests of the end user.
Finally, we recommend the establishment of laws and industry standards clarifying and the fulfillment of ‘duty of loyalty’ and ‘duty of care’ when AI is used in the place of or in assistance to a human fiduciary. In some circumstances – for instance, financial advice and legal counsel – human actors are legally obligated to act in the best interest of their clients and to exercise due care to minimize harmful outcomes. AI systems are increasingly being deployed to advise on these types of decisions or to make them (e.g. trading stocks) independent of human input. Laws and standards towards this end should require that if an AI system is to contribute to the decision-making of a fiduciary, the fiduciary must be able to demonstrate beyond a reasonable doubt that the AI system will observe duties of loyalty and care comparable to their human counterparts. Otherwise, any breach of these fiduciary responsibilities should be attributed to the human fiduciary employing the AI system.
Conclusion
The new generation of advanced AI systems is unique in that it presents significant, well-documented risks, but can also manifest high-risk capabilities and biases that are not immediately apparent. In other words, these systems may perform in ways that their developers had not anticipated or malfunction when placed in a different context. Without appropriate safeguards, these risks are likely to result in substantial harm, in both the near- and longer-term, to individuals, communities, and society.
Historically, governments have taken critical action to mitigate risks when confronted with emerging technology that, if mismanaged, could cause significant harm. Nations around the world have employed both hard regulation and international consensus to ban the use and development of biological weapons, pause human genetic engineering, and establish robust government oversight for introducing new drugs to the market. All of these efforts required swift action to slow the pace of development, at least temporarily, and to create institutions that could realize effective governance appropriate to the technology. Humankind is much safer as a result.
We believe that approaches to advancement in AI R&D that preserve safety and benefit society are possible, but require decisive, immediate action by policymakers, lest the pace of technological evolution exceed the pace of cautious oversight. A pause in development at the frontiers of AI is necessary to mobilize the instruments of public policy toward common-sense risk mitigation. We acknowledge that the recommendations in this brief may not be fully achievable within a six month window, but such a pause would hold the moving target still and allow policymakers time to implement the foundations of good AI governance.
The path forward will require coordinated efforts by civil society, governments, academia, industry, and the public. If this can be achieved, we envision a flourishing future where responsibly developed AI can be utilized for the good of all humanity.
↩ 1 See, e.g., Steve Rathje, Jay J. Van Bavel, & Sander van der Linden, ‘Out-group animosity drives engagement on social media,’ Proceedings of the National Academy of Sciences, 118 (26) e2024292118, Jun. 23, 2021, and Tiffany Hsu & Stuart A. Thompson, ‘Disinformation Researchers Raise Alarms About A.I. Chatbots,’ The New York Times, Feb. 8, 2023
↩ 2 See, e.g., Abid, A., Farooqi, M. and Zou, J. (2021a), ‘Large language models associate Muslims with violence’, Nature Machine Intelligence, Vol. 3, pp. 461–463.
↩ 3 In a 2022 survey of over 700 leading AI experts, nearly half of respondents gave at least a 10% chance of the long-run effect of advanced AI on humanity being ‘extremely bad,’ at the level of ‘causing human extinction or similarly permanent and severe disempowerment of the human species.’
↩ 4 Future of Life Institute, ‘Pause Giant AI Experiments: An Open Letter,’ Mar. 22, 2023.
↩ 5 Recent news about AI labs cutting ethics teams suggests that companies are failing to prioritize the necessary safeguards.
↩ 6 The Future of Life Institute has previously defined “general-purpose AI system” to mean ‘an AI system that can accomplish or be adapted to accomplish a range of distinct tasks, including some for which it was not intentionally and specifically trained.’
↩ 7 Samuel R. Bowman, ’Eight Things to Know about Large Language Models,’ ArXiv Preprint, Apr. 2, 2023.
↩ 8 Proposed EU Artificial Intelligence Act, Article 43.1b.
↩ 9 National Institute of Standards and Technology, ‘Artificial Intelligence Risk Management Framework (AI RMF 1.0),’ U.S. Department of Commerce, Jan. 2023.
↩ 10 International standards bodies such as IEC, ISO and ITU can also help in developing standards that address risks from advanced AI systems, as they have highlighted in response to FLI’s call for a pause.
↩ 11 See, e.g., the Holistic Evaluation of Language Models approach by the Center for Research on Foundation Models: Rishi Bommassani, Percy Liang, & Tony Lee, ‘Language Models are Changing AI: The Need for Holistic Evaluation‘.
↩ 12 OpenAI described weaponization risks of GPT-4 on p.12 of the “GPT-4 System Card.”
↩ 13 See, e.g., the following benchmark for assessing adverse behaviors including power-seeking, disutility, and ethical violations: Alexander Pan, et al., “Do the Rewards Justify the Means? Measuring Trade-offs Between Rewards and Ethical Behavior in the MACHIAVELLI Benchmark,” ArXiv Preprint, Apr. 6, 2023.
↩ 14 Jess Whittlestone et al., ‘Future of compute review – submission of evidence’, Aug. 8, 2022.
↩ 14.5 Please see fn. 14 for a detailed proposal for government compute monitoring as drafted by the Centre for Long-Term Resilience and several staff members of AI lab Anthropic.
↩ 15 Yonadav Shavit at Harvard University has proposed a detailed system for how governments can place limits on how and when AI systems get trained.
↩ 15.5 Bureau of Industry and Security, Department of Commerce, ‘Implementation of Additional Export Controls: Certain Advanced Computing and Semiconductor Manufacturing Items; Supercomputer and Semiconductor End Use; Entity List Modification‘, Federal Register, Oct. 14, 2022.
↩ 16 Eleanor Olcott, Qianer Liu, & Demetri Sevastopulo, ‘Chinese AI groups use cloud services to evade US chip export control,’ Financial Times, Mar. 9, 2023.
↩ 17 Anton Korinek, ‘Why we need a new agency to regulate advanced artificial intelligence: Lessons on AI control from the Facebook Files,’ Brookings, Dec. 8 2021.
↩ 18 Proposed EU Artificial Intelligence Act, Article 60.
↩ 19 Will Knight & Khari Johnson, ‘Now That ChatGPT is Plugged In, Things Could Get Weird,’ Wired, Mar. 28, 2023.
↩ 20 OpenAI, ‘GPT-4 System Card,’ Mar. 23, 2023, p.15.
↩ 21 I.e., functions that could materially affect the wellbeing or rights of individuals, communities, or society.
↩ 22 Joseph Cox, “Facebook’s Powerful Large Language Model Leaks Online,” VICE, Mar. 7, 2023.
↩ 23 For a systematic overview of how watermarking can be applied to AI models, see: Franziska Boenisch, ‘A Systematic Review on Model Watermarking of Neural Networks,’ Front. Big Data, Sec. Cybersecurity & Privacy, Vol. 4, Nov. 29, 2021.
↩ 24 This figure, drawn from , ‘The AI Arms Race is Changing Everything,’ (Andrew R. Chow & Billy Perrigo, TIME, Feb. 16, 2023 ), likely represents a lower bound for the estimated number of AI safety researchers. This resource posits a significantly higher number of workers in the AI safety space, but includes in its estimate all workers affiliated with organizations that engage in AI safety-related activities. Even if a worker has no involvement with an organization’s AI safety work or research efforts in general, they may still be included in the latter estimate.
↩ 25 Christine Criddle & Madhumita Murgia, ‘Big tech companies cut AI ethics staff, raising safety concerns,’ Financial Times, Mar. 29, 2023.
↩ 26 See fn. 21, supra.
↩ 27 Original call for a UK government AI lab is set out in this article.
↩ 28 For the taskforce’s detailed recommendations, see: ‘Strengthening and Democratizing the U.S. Artificial Intelligence Innovation Ecosystem: An Implementation Plan for a National Artificial Intelligence Research Resource,’ National Artificial Intelligence Research Resource Task Force Final Report, Jan. 2023.
↩ 29 Pranshu Verma, ‘They thought loved ones were calling for help. It was an AI scam.‘ The Washington Post, Mar. 5, 2023.
↩ 30 Tiffany Hsu & Stuart A. Thompson, ‘Disinformation Researchers Raise Alarms About A.I. Chatbots,’ The New York Times, Feb. 8, 2023 .
↩ 31 Proposed EU Artificial Intelligence Act, Article 52.
↩ 32 SB 1001 (Hertzberg, Ch. 892, Stats. 2018).
↩ 33 Recommendation 125, ‘Outcome document: first draft of the Recommendation on the Ethics of Artificial Intelligence,’ UNESCO, Sep. 7, 2020, p. 21.