Artificial Intelligence: The Challenge to Keep It Safe
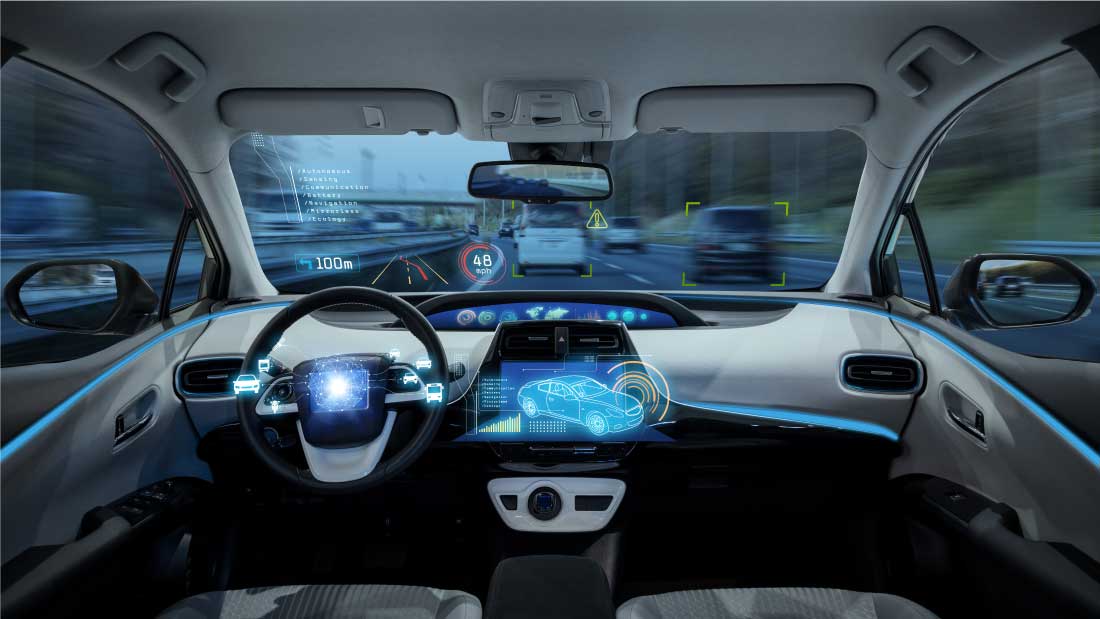
Contents
Safety Principle: AI systems should be safe and secure throughout their operational lifetime and verifiably so where applicable and feasible.
When a new car is introduced to the world, it must pass various safety tests to satisfy not just government regulations, but also public expectations. In fact, safety has become a top selling point among car buyers.
And it’s not just cars. Whatever the latest generation of any technology happens to be — from appliances to airplanes — manufacturers know that customers expect their products to be safe from start to finish.
Artificial intelligence is no different. So, on the face of it, the Safety Principle seems like a “no brainer,” as Harvard psychologist Joshua Greene described it. It’s obviously not in anyone’s best interest for an AI product to injure its owner or anyone else. But, as Greene and other researchers highlight below, this principle is much more complex than it appears at first glance.
“This is important, obviously,” said University of Connecticut philosopher Susan Schneider, but she expressed uncertainty about our ability to verify that we can trust a system as it gets increasingly intelligent. She pointed out that at a certain level of intelligence, the AI will be able to rewrite its own code, and with superintelligent systems “we may not even be able to understand the program to begin with.”
What Is AI Safety?
This principle gets to the heart of the AI safety research initiative: how can we ensure safety for a technology that is designed to learn how to modify its own behavior?
Artificial intelligence is designed so that it can learn from interactions with its surroundings and alter its behavior accordingly, which could provide incredible benefits to humanity. Because AI can address so many problems more effectively than people, it has huge potential to improve health and wellbeing for everyone. But it’s not hard to imagine how this technology could go awry. And we don’t need to achieve superintelligence for this to become a problem.
Microsoft’s chatbot, Tay, is a recent example of how an AI can learn negative behavior from its environment, producing results quite the opposite from what its creators had in mind. Meanwhile, the Tesla car accident, in which the vehicle mistook a white truck for a clear sky, offers an example of an AI misunderstanding its surrounding and taking deadly action as a result.
Researchers can try to learn from AI gone astray, but current designs often lack transparency, and much of today’s artificial intelligence is essentially a black box. AI developers can’t always figure out how or why AIs take various actions, and this will likely only grow more challenging as AI becomes more complex.
However, Ian Goodfellow, a research scientist at Google Brain, is hopeful, pointing to efforts already underway to address these concerns.
“Applying traditional security techniques to AI gives us a concrete path to achieving AI safety,” Goodfellow explains. “If we can design a method that prevents even a malicious attacker from causing an AI to take an undesirable action, then it is even less likely that the AI would choose an undesirable action independently.”
AI safety may be a challenge, but there’s no reason to believe it’s insurmountable. So what do other AI experts say about how we can interpret and implement the Safety Principle?
What Does ‘Verifiably’ Mean?
‘Verifiably’ was the word that caught the eye of many researchers as a crucial part of this Principle.
John Havens, an Executive Director with IEEE, first considered the Safety Principle in its entirety, saying, “I don’t know who wouldn’t say AI systems should be safe and secure. … ‘Throughout their operational lifetime’ is actually the more important part of the sentence, because that’s about sustainability and longevity.”
But then, he added, “My favorite part of the sentence is ‘and verifiably so.’ That is critical. Because that means, even if you and I don’t agree on what ‘safe and secure’ means, but we do agree on verifiability, then you can go, ‘well, here’s my certification, here’s my checklist.’ And I can go, ‘Great, thanks.’ I can look at it, and say, ‘oh, I see you got things 1-10, but what about 11-15?’ Verifiably is a critical part of that sentence.”
AI researcher Susan Craw noted that the Principle “is linked to transparency.” She explained, “Maybe ‘verifiably so’ would be possible with systems if they were a bit more transparent about how they were doing things.”
Greene also noted the complexity and challenge presented by the Principle when he suggested:
“It depends what you mean by ‘verifiably.’ Does ‘verifiably’ mean mathematically, logically proven? That might be impossible. Does ‘verifiably’ mean you’ve taken some measures to show that a good outcome is most likely? If you’re talking about a small risk of a catastrophic outcome, maybe that’s not good enough.”
Safety and Value Alignment
Any consideration of AI safety must also include value alignment: how can we design artificial intelligence that can align with the global diversity of human values, especially taking into account that, often, what we ask for is not necessarily what we want?
“Safety is not just a technical problem,” Patrick Lin, a philosopher at California Polytechnic told me. “If you just make AI that can align perfectly with whatever values you set it to, well the problem is, people can have a range of values, and some of them are bad. Just merely matching AI, aligning it to whatever value you specify I think is not good enough. It’s a good start, it’s a good big picture goal to make AI safe, and the technical element is a big part of it; but again, I think safety also means policy and norm-setting.”
And the value-alignment problem becomes even more of a safety issue as the artificial intelligence gets closer to meeting — and exceeding — human intelligence.
“Consider the example of the Japanese androids that are being developed for elder care,” said Schneider. “They’re not smart; right now, the emphasis is on physical appearance and motor skills. But imagine when one of these androids is actually engaged in elder care … It has to multitask and exhibit cognitive flexibility. … That raises the demand for household assistants that are AGIs. And once you get to the level of artificial general intelligence, it’s harder to control the machines. We can’t even make sure fellow humans have the right goals; why should we think AGI will have values that align with ours, let alone that a superintelligence would.”
Defining Safety
But perhaps it’s time to reconsider the definition of safety, as Lin alluded to above. Havens also requested “words that further explain ‘safe and secure,’” suggesting that we need to expand the definition beyond “physically safe” to “provide increased well being.”
Anca Dragan, an associate professor at UC Berkeley, was particularly interested in the definition of “safe.”
“We all agree that we want our systems to be safe,” said Dragan. “More interesting is what do we mean by ‘safe’, and what are acceptable ways of verifying safety.
“Traditional methods for formal verification that prove (under certain assumptions) that a system will satisfy desired constraints seem difficult to scale to more complex and even learned behavior. Moreover, as AI advances, it becomes less clear what these constraints should be, and it becomes easier to forget important constraints. … we need to rethink what we mean by safe, perhaps building in safety from the get-go as opposed to designing a capable system and adding safety after.”
What Do You Think?
What does it mean for a system to be safe? Does it mean the owner doesn’t get hurt? Are “injuries” limited to physical ailments, or does safety also encompass financial or emotional damage? And what if an AI is being used for self-defense or by the military? Can an AI harm an attacker? How can we ensure that a robot or software program or any other AI system remains verifiably safe throughout its lifetime, even as it continues to learn and develop on its own? How much risk are we willing to accept in order to gain the potential benefits that increasingly intelligent AI — and ultimately superintelligence — could bestow?
This article is part of a series on the 23 Asilomar AI Principles. The Principles offer a framework to help artificial intelligence benefit as many people as possible. But, as AI expert Toby Walsh said of the Principles, “Of course, it’s just a start. … a work in progress.” The Principles represent the beginning of a conversation, and now we need to follow up with broad discussion about each individual principle. You can read the discussions about previous principles here.
About the Future of Life Institute
The Future of Life Institute (FLI) is a global think tank with a team of 20+ full-time staff operating across the US and Europe. FLI has been working to steer the development of transformative technologies towards benefitting life and away from extreme large-scale risks since its founding in 2014. Find out more about our mission or explore our work.
Related content
Other posts about AI, AI Safety Principles, Recent News
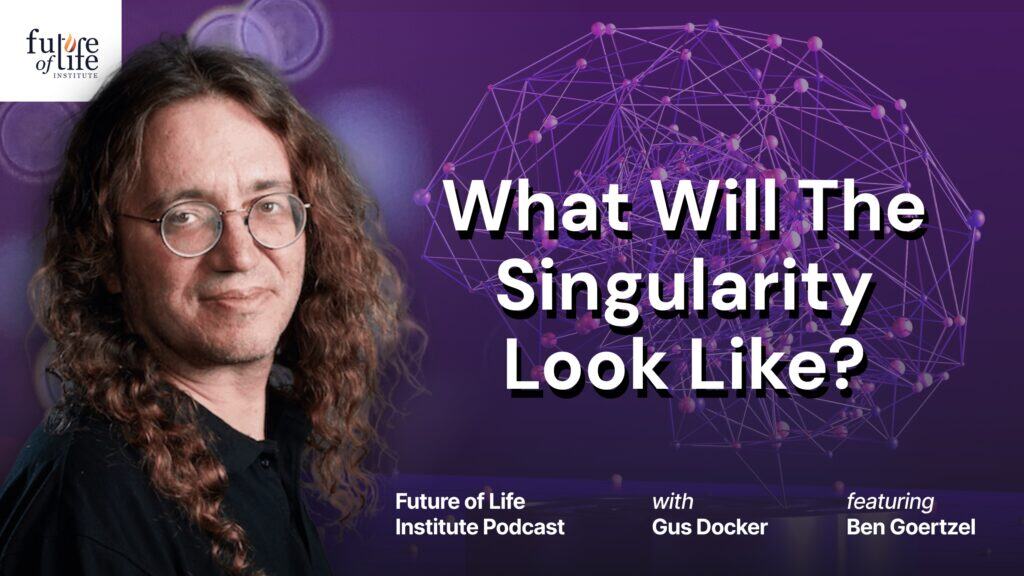
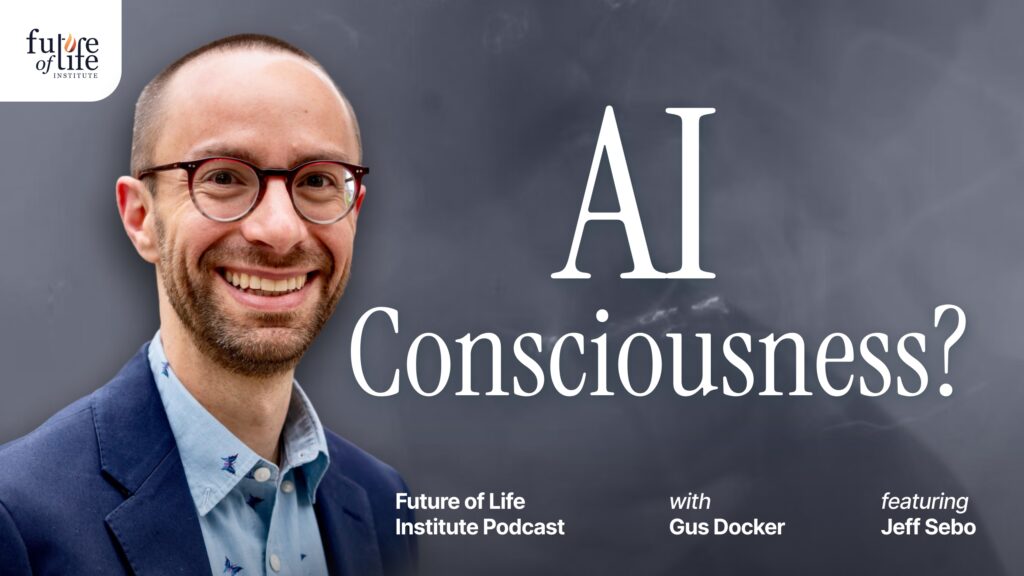