Connor Leahy on AI Progress, Chimps, Memes, and Markets
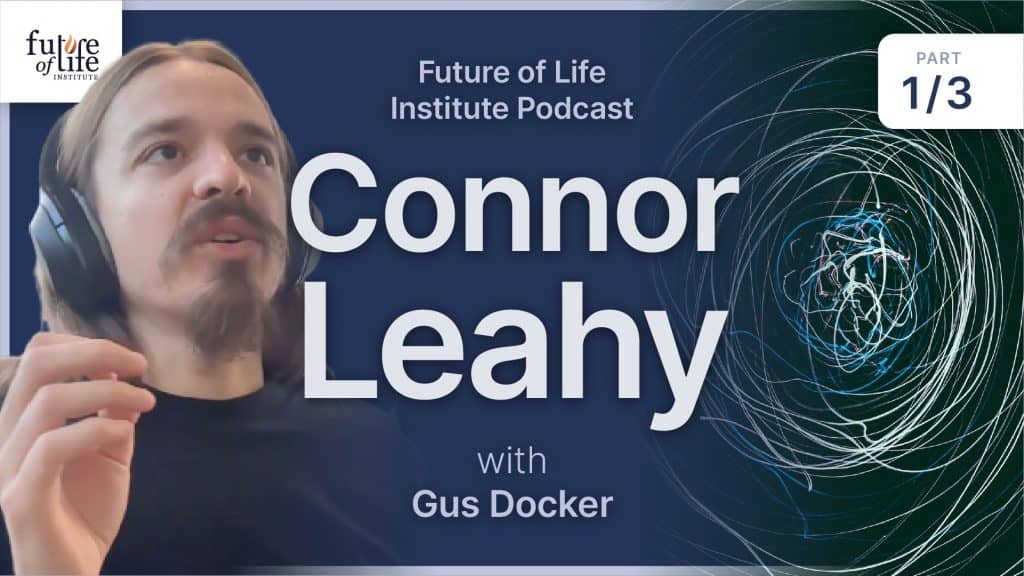
Connor Leahy from Conjecture joins the podcast to discuss AI progress, chimps, memes, and markets. Learn more about Connor's work here.
Topics covered include:
01:00 Defining artificial general intelligence
04:52 What makes humans more powerful than chimps?
17:23 Would AIs have to be social to be intelligent?
20:29 Importing humanity's memes into AIs
23:07 How do we measure progress in AI?
42:39 Gut feelings about AI progress
47:29 Connor's predictions about AGI
52:44 Is predicting AGI soon betting against the market?
57:43 How accurate are prediction markets about AGI?
Books cited in the episode include:
- The Incerto Series by Nassim Nicholas Taleb
- The Selfish Gene, Richard Dawkins
- Various books on primates and animal intelligence by Frans De Wall
- Inadequate Equilibria by Eliezer Yudkowsky
Highlights
On intelligence in humans and chimps:
We are more social because we're more intelligent and we're more intelligent because we are more social. These things are not independent variables. So at first glance, if you look at a human brain versus a chimp brain, it's basically the same thing. You see like all the same kind of structures, same kind of neurons, though a bunch of parameters are different. You see some more spindle cells, it's bigger. Human brain just has more parameters, it's just GPT-3 versus GPT-4...
But really, the difference is, is that humans have memes. And I mean this in the Richard Dawkins sense of evolved, informational, programmatic virtual concepts that can be passed around between groups. If I had to pick one niche, what is the niche that humans are evolved for?
I think the niche we're evolved for is memetic hosts.
On benchmarks and scaling laws:
Benchmarks are actually coordination technologies. They're actually social technologies. What benchmarks are fundamentally for is coordination mechanisms. The kind of mechanisms you need to use when you're trying to coordinate groups of people around certain things....
So we have these scaling laws, which I think a lot of people misunderstand. So scaling laws give you these nice curves which show how the loss of performance on the model smoothly decreases as they get larger. These are actually terrible, and these actually tell you nothing about the model. They tell you what one specific number will do. And this number doesn't mean anything. There is some value in knowing the loss. But what we actually care about is can this model do various work? Can it do various tasks? Can it reason about its environment? Can it reason about its user?...
So currently there are no predictive theories of intelligence gain or task. There is no theory that says once it reaches 74.3 billion parameters, then it will learn this task. There's no such theory. It's all empirical. And we still don't understand these things at all. I think there's, so another reason I'm kind of against benchmarks, and I'm kind of being a bit pedantic about this question is because I think they're actively misleading in the sense that people present them as if they mean something, but they just truly, truly don't. A benchmark in a vacuum means nothing.
On the dangers of having a good metric of progress towards AGI:
So this is an interesting question. And not just from a scientific perspective, but it's also interesting from a meta perspective for info-hazardous reasons. Let's assume I had a one true way of measuring whether a system is getting closer to AGI or not. I would consider that to be very dangerous. I would consider the because it basically gives you a blueprint or at least a direction in North Star of how to build more and more powerful system. We're gonna talk about this more later, I'm sure. But I consider the idea of building AGI to be very fraught with risks. I'm not saying it's not something we should do. It's also something that could be the best thing to ever happen to humanity. I'm just saying we have to do it right.
And the way things currently are looking with like our levels of AI safety and control, I think racing ahead to build AGI as fast as possible without being able to control or understand it is incredibly dangerous. So if I had such a metric, I am not saying I do or I don't, but if I did, I probably shouldn't tell you, I probably shouldn't tell people.
On the efficient market hypothesis:
The efficient market hypothesis and its consequences have been a disaster for humanity. The idea that the market is efficient is a very powerful and potent meme. And it has a lot of value in this idea. But I think people don't actually understand what it means and get confused by it...
The efficiency of a market is a contingent phenomenon. It's an observer dependent phenomenon. So if you, the reader are actually, the smartest, Jane Street, FinTech trader in the entire world, you may well be able to put $10 million into the market and take $20 million out. That's a contingent phenomenon. It's an observer dependent phenomenon. And this is also why, for example, if an alien from outer space came with 3000 million IQ, I expect they could extract arbitrary amounts of money from the market. They could money pump forever. There is nothing that can be done about that. So it is not just intelligence based either. Let us say you are the assistant of a CEO at some big company. You get a phone call and you are told the CEO had a heart attack and has died.
The market isn't so efficient that the market hypothesis says trading on this wouldn't help because surely if this was problematic, it would have already been priced in. Of course, that is silly. Someone has to price it in. You are that person. You can make a lot of money by pricing this in.
Transcript
Gus Docker: Welcome to the Future of Life Institute podcast. My name is Gus Docker. On this episode, I talk with Connor Leahy. Connor is the CEO of Conjecture, which is a new organization researching scalable AI alignment. We talk about how to find a useful definition of artificial general intelligence, what we can learn from studying the differences between humans and chimps about intelligence in general, how we should go about predicting AI progress, and why Connor expects artificial general intelligence faster than most people. This was a very fun conversation for me, and I hope you enjoy it too. Here is Connor Leahy. Connor, welcome to the podcast. Great to have you on.
Connor Leahy: Thanks for having me.
Gus: I've been looking forward to this a lot. I've been going through your stuff, as I told you, and you have lots of interesting things to say. What I want to start with is just thinking about how we, first of all, define artificial general intelligence and how we measure progress in AI. What metrics should we use to measure progress? Are we talking about economic growth or perhaps benchmarks for certain tasks solving? What's the most useful metric here?
Connor: I think I'll start by defining AGI or what I think a useful definition of AGI is. The first thing, of course, is just there is no universal definition of AGI. It's ultimately a marketing term, like anyone calls it whatever the fuck they want.
Gus: We're definitely not looking for the true definition of AGI.
Connor: Yeah, of course. Just making that clear up front. I'm not claiming I have the one true definition of intelligence or AGI or whatever. I define it more out of use. What am I trying to point at? What is the property that is interesting that I think is qualitatively different from the systems we might describe we have today? The pithiest answer I can give for that is AGI is AI that has the thing that humans have that chimps don't. Another way to put this is an AGI is not, so uh, I think it was like Yan LeCun, for example, argued that AGI is a bad concept because no system can do everything. Alphafold is really good for proteins. A GTP might be good at writing books or whatever, but you can't have a system that does everything. I think this is actually a bit confused. For me, AGI is not a system that can do everything Alphafold can do, everything GPT can do, and everything DALLY can do. It's a system that when confronted with a protein solving a folding problem, invents Alpha fold. That's what I would call AGI.
Gus: Would you say this is a pretty high standard for AGI?
Connor: It's a pretty high standard, yeah. I sometimes as a meme use the weaker definition, which is just a system that can reasonably learn any task at human or above human level with reasonable amounts of compute and effort or whatever. This has the amusing memetic side effect that I can get into great arguments with people about that I think GPT-3 already fulfills this property. GPT-3 can do most sequence-to-sequence tasks with a reasonable amount of effort. If you can code most human-level office job tasks into sequence to sequence prediction tasks, which is the case for most of them, a large GPT model with enough label training data can learn those for the most part. Not all of them, there's many of them that can't. I wouldn't say can't, that they currently haven't or we currently haven't gotten around to do.
The reason I'm using the higher standard is because people bitch when I use the lower standard one. The joke is always when something stops being AI the moment it works. I think we've had that happen with GPT-3 as well, is that if I had described what GPT-3 could do to the general public or to AI researchers and I gave them exactly what GPT-3 can do and I asked, is this AGI? I predict that most people would have said yes. But now with hindsight bias, like, well, no, it's not what we really meant. I'm using the higher standard for the point purpose of this, which is just if it encounters a protein folding problem, does it invent, implement and use out for fold?
Gus: And this is why it's interesting to even discuss what's the most useful definition of AGI, because by some definitions, GPT-3 is already there. By other definitions, if you require perhaps inventing new physics for a system to qualify as an AGI, well, then there's a large differentiator between those two extremes. What about the question, oh, let's talk about the chimp comparison, actually. So one question I have for you is, so what is it that differentiates humans from chimps? Is it our raw intelligence or could it perhaps be our ability to cooperate or the fact that we've gone through thousands of years of social evolution? So is it, are humans primarily different from chimps because we are more intelligent?
Connor: So these things are not independent. We are more social because we're more intelligent and we're more intelligent because we are more social. These things are not independent variables. So at first glance, if you look at a human brain versus a chimp brain, it's basically the same thing. You see like all the same kind of structures, same kind of neurons, kind of, sure, a bunch of parameters are different. You see some more spindle cells than not. You see some more whatever. Of course, it's bigger. Human brain is just significantly, it just has more parameters, it's just GPT-3 versus GPT-4. You know, small circle, big circle. I don't know if you've seen those memes, but it has a bunch of stupid people. They're speculating about how many parameters GPT-4 will have, which has become a meme on a EleutherAI right now.
Anyways, so humans are obviously extremely selected for parameter count. Like there's this, people want to believe that there's some fundamental difference between humans and chimps. I don't think that's true. I think like actually you could probably, like purely like genetically speaking, we should be considered a subspecies of chimp. Like we shouldn't really be considered like a whole different family, a whole different class. We're more like, you know, the third chimp, you know, there's like the classic chimp, the bonobo and then like humans, like we should be right next to the bonobo. Like we're actually super, super, super similar to chimps. And here's a small recommendation for any AI researchers listening who wants some interesting reading. I highly recommend reading at least one book about chimps and their politics. It's a very enlightening, especially like Frans De Waal's books on chimps.
Because then there's a lot of people I think have very wrong images of like how, this is a general thing. I think lots of people have wrong images about how smart animals actually are, because I think lots of, lots of studies in the past about how smart animals were done very poorly. And like, oh, they have no self concept whatsoever, they don't understand physics, they don't understand tasks or planning is obviously wrong. Like chimps obviously have theory of mind. Like if you could, you could observe chimps reasoning, not just about their environment, but about what other chimps know about the environment, and then how they should act according to that. So like chimps obviously have theory of mind, I consider this totally uncontroversial. I know some philosopher of mind will complain about this, but I don't care. Like chimps are obviously intelligent, they obviously have theory of mind.
Gus: So you think chimps are more intelligent than, more sophisticated, cognitively sophisticated than we might believe. But what differentiates humans is that humans have higher neuron count. Is that it bigger brains?
Connor: That's the that's the main physiological difference. Now there is of course, software differences. So this is the social aspect. So chimps, for example, just do not fucking trust each other. So if two chimps from two different bands meet each other, it always ends in violence or like, you know, you know, screaming or whatever, right? Like they cannot cooperate across bands, like chimps live in bands. And within a band, they can cooperate. It's actually quite impressive. Like so there's this concept of like chimp war bands. So this is like a real thing. So like there have been chimpanzee wars. So like, I love when people are like, you know, humanity, you know, stuff, the most violent species created some war. I'm like, man, have you seen what chimps do to each other? Holy shit. Like, it's awful. Chimps are just the worst. They're just like the worst possible thing. It's incredible. Like all the bad things about humans, like compressed into one little goblin creature.
Gus: And we could think about say, if humans live in these small tribes, how much could how much could we actually achieve? Could that be the limiting factor to what we achieve as a species, how powerful we are, as opposed to our raw intelligence?
Connor: So it's a mixture of both for sure. So there's a few things that come into this and sorry, I'm spending so much time with chimps, but I actually think this is really fascinating. This is actually useful for thinking about intelligence. I think when you're thinking about intelligence is really valuable to actually look at, you know, other intelligences that are out there and like, try to understand them and like how they work. So I think not enough people like pay attention to chimpanzees and like monkeys and like how smart they actually are. Also, other animals, by the way, like crows are much smarter than you think they are. Like they understand like Newtonian physics. It's actually crazy. So, but there are limitations. So like you look at chimps, right. And they like, they don't really have language. They have like some like proto language. So they have like concepts and grounds they can like change with each other. They don't really have like, like grammar or like, you know, like recursion and stuff like that. But they do have some interesting properties.
So for example, chimps are much better, like absurdly better at short term memory than humans. Like absurdly though, like to a chimp, we look like hand like mentally handicapped when it comes to short term memory tasks. But if you flash like 10 numbers for a chimp to memorize for just like one second, they'll instantly memorize it. And they can like repeat the exact sequence of numbers. And they do this like very reliably if you can get them to operate, which is another problem with the intelligence tasks is that often animals will just not want to play along with what you're trying to do. But there are, you know, there's some funny YouTube videos where you can see chimps, you know, instantly memorize, you know, like 10 digits and just like, you know, instantly re, you know, redoing them. So the human brain obviously is more optimized in other ways. Like we obviously gave up short term memory for something else.
There's obviously a lot of weird pressure, evolutionary pressure went on in humans. So the main, the main reason I say what humans I think are extremely strongly selected for large parameter counts for large brains is, I mean, look at birth in humans, like, you know, the size of the human skull is so absurdly optimized to be as big as possible without us dying during childbirth. And already, childbirth for humans is like, very abnormally dangerous for animals. Like for most animal birth is not that complicated. It's like just kind of a thing like the mother usually doesn't die. This is a complicated thing. For humans, this is a very, very complicated thing. And it's a and you know, it's really like the skull, like even when babies are born, babies are born very premature, actually, and their skulls are so squishy. It's like their, their, their, their skulls haven't fused. So they're like, literally squishy. So they can still fit, so you can fit more brain and still have the child be born. So you look at all of that. That's what it looks like when evolution is optimizing really hard myopically for one thing. So humans are obviously extremely optimized to have as big of a brain as we can fit.
We also have like some, so if there's like one adaption we have compared to like chimps and like other, and like close animals, it's that we have like much more sophisticated brain cooling, like our like blood circulatory system in the brain and our cooling system is unusually sophisticated. And so there's all these things that point pretty strongly that humans were super, super hardly heavily selected for intelligence. But why we were selected for intelligence exactly is a bit controversial, actually. So there's this very interesting phenomena in the paleolithic record, where when, when anatomically modern humans emerged, you know, they came together into groups, they developed what are called hand axes, which don't really look like axes, but they're like simple primitive stone tools. And then they did nothing. For like 70,000 years, they just kind of sat around and made hand axes. They didn't really expand that, they expanded, but like, you know, they didn't really, they didn't develop art, they didn't really have like, they didn't build buildings, they didn't have like, you know, they didn't develop like more, they didn't have spears or bows, as far as we're aware of.
You know, of course, as is always the case in archaeology, all of this could be overturned by finding, you know, one weird rock somewhere in Africa. So, you know, just for those that are unaware about the epistemological status of archaeology, it's a very frosty field to make predictions about. But in the current, you know, main telling of human history, there's this bizarre period that I feel like not enough people talk about, where humans existed everywhere. There was lots of humans, like, these are completely anatomically modern humans. And they did nothing. They had like, they didn't develop technology, they didn't develop, you know, writing or symbology or like anything, they just kind of sat around. That's really, really weird. And then, very suddenly, I think it was in East Africa, there suddenly was this explosion of like art and like new new forms of tools and, you know, all these kind of stuff just kind of written like, and the way it radiated back over, like all the other tribes that already existed outside of Africa, and kind of overtook them with this like, revolution of like new cognitive abilities.
Gus: Burial rituals, and cave paintings, and proto religions, all of these things. Yeah, yeah.
Connor: So, so this is, of course, it's, you know, very fascinating, because like, these are not anatomically different, like, it's not like their heads are suddenly twice as big or something. So the like, you look at like, like, you look at bones from like, before after this period, they look identical. You can't tell any difference, at least from bones. But the behavioral differences were massive. And like the cultural and like technological differences were massive. Sure looks like a foom, doesn't it?
Gus: What is a foom, Connor?
Connor: So a foom is a explosion in intelligence, where suddenly a system that kind of has been petering around for quite a long time, you know, suddenly gets really powerful really quickly. And so from the perspective of a human, this is still quite slow, you know, this still takes like 10s of 1000s of years and whatever, from the perspective of evolution of like, you know, some animals have existed, you know, exist for millions of years or whatever. Suddenly we had this, you know, you know, pretty sophisticated monkey, you know, it has these like rocks, it's pretty cool, you know, for like 100,000 years, and then suddenly, within like 10,000 years, so again, this is before modern history, this is still prehistory, this is still Stone Age, this is before we're talking about like, you know, you know, modern culture, this is like before Babylon before or before all of that, before all of that, we still have this some small groups. So this may have been literally one tribe, we don't know, like, it's impossible to know, they may have literally been one tribe in East Africa, that suddenly developed technology, that suddenly developed art, and just spread from there.
So it's unclear whether they spread genetically, like whether this tribe conquered the other tribe or whether it's spread memetically. So I think the second is more interesting. I have gonna have this is all wild speculation, but come on, this is a podcast, we're gonna have fun. So I'm interested in the idea of it being memetic. And so I think the main thing that is different between chimps and humans other than parameter counts, which is very important, I think parameter count gives you the hardware, this is the first step is hardware. But really, the difference is, is that humans have memes. And I mean, this in the, you know, in a Richard Dawkins sense of like, evolved, informational, like you know, programmatic virtual concepts that can be passed around between groups. If I had to pick one niche, what is the niche that humans are evolved for?
I think the niche we're evolved for is memetic hosts. We are organisms that more than any other organism on the planet is evolved to share digital information or virtual information, or just information in general. This doesn't work in chimps. There's many reasons doesn't work chimps, they have the memory capacity for it, but they don't have language. They don't really have also their voice boxes are much less sophisticated than humans. It's unclear if this is actually a bottleneck. But like, actually, another weird adaption that like, almost only humans have, is their control over our breath. So most animals can't hold their breath. This is actually an adaption that only some animals have. And humans have extremely good control over their breath. And this is actually necessary for us to speak. If you pay attention to while you speak, you'll notice that you hold your breath for like a microsecond, like all the time. And if you try to breathe while you speak, it will come out like, you know, like gibberish.
Gus: So yeah, what does all of this imply for the difference between humans and AI then? Is what we have to imbue AIs with memetic understanding for them to progress? Would there have to be AIs talking with each other in a social way for them to become more intelligent or is that extrapolating too far?
Connor: So the interesting thing here is that, so what does the memetic mean? So memetic is a new kind of evolution, which is different from genetic evolution. It's so similar in the sense that it is still, you know, selection based, right? After some kind of selection mechanism and selecting some memes to be fit and some not be fit. But what is different from genetic evolution? Speed. Memetics are very fast. You can try many memes very quickly within one generation without having to restart from scratch. I think that's the fundamental novelty, basically, that humanity has that made us work. It's that we moved our evolutionary baggage from our genes to our memes.
So the main difference between me and my paleolithic ancestor, other than me being frail and weak compared to probably how buff he was, is memetics. Like I just know things. I have concepts in my mind. I have algorithms and epistemology and culture in my mind that in many scenarios will make me much, much more capable. If you put him next to me on reasoning tasks or cultural tasks or writing or whatever, I would obviously win those tasks every single time. Of course, their brain will be specialized for other tasks that will be put back into the paleolithic. My survival chances are probably not great, but he will probably do fine. That's interesting because the different, because it's genetic. Like I could learn a lot of the things that a paleolithic hunter knows. You know, if I do enough weightlifting and I have him teach me how to hunt, I could probably learn that. And vice versa, if he was willing to, you know, I could probably teach my paleolithic ancestors quite a lot about mathematics and like how the world works.
That's not how chimps work at all. That is not at all how chimps work. And this has been tried with chimps. It has actually been tried with chimps. You can't teach this to chimps. You can't teach this to animals, but you can teach humans. So what this means is that the interesting unit of information moves from the design of the body or the implementation to the software. It moves to the training data. It moves to the algorithms. And so what this tells us about AI is that AI is really the obvious next step. It's the, it's only memes, you know, it's, it's all digital. It's all virtual. It doesn't have a body. It doesn't need a body. You know, the closest thing an AI has to a body is it's, you know, it's like network architecture or whatever, but even that can just be overwritten. Even that is just memes. It's just Python files. Those are just memes. It's just information. An AI can just write itself a new body. It can just rewrite how its brain works. Those are, there's no reason to, you know, so, you know, so humans are kind of this intermediate step between like animals and like, you know, whatever comes beyond, you know, angels, you know, we're like the intermediate stage of like, we have both, we're both animals and we have like access to the memetic, you know, spiritual realm, whatever. But then the next step is pure software.
Gus: And we have an enormous corpus of data produced by humanity, say, go back 500 years or so, that we can train these AIs on. So there is a, we can, we can very easily import our memes, so to speak, into the AI. And this, I'm guessing that what this means is that AI can very quickly catch up to where humans are.
Connor: Exactly. And that's my ultimate, that's my ultimate prediction is that, you know, I look at, yeah, took humans thousands of years, you know, bickering and tribes to figure out, you know, X, Y, and Z. I don't expect that to be a problem for AI. AI can operate much faster. I also think the, to quickly get back to the social aspect of this. So, you know, humanity, obviously, social, sociality, and like working in tribes and such was incredibly important for the development of intelligence for multiple reasons, like, you know, look at the Machiavellian intelligence hypothesis. But obviously, a big part of this was, is externalized cognition. So I think of sociality, to a large degree, as another hack to increase parameter count. You couldn't fit them inside one brain. Well, maybe we can like sort of jury rig several brains to like work as one brain. And it like sort of works a little bit. So like, you know, humans in a group are smarter, wisdom of crowds, you know, people with, you know, you know, in a society, like a society is smarter than any individual person. This is like, obviously the case, it doesn't, but it doesn't have to be that way. That's not the case with chimps. Again, if you put more chimps into a pile, it becomes less smart because they start fighting. So this is not a inherent property of groups. This is a property that humans have developed that, you know, took lots of selection to get to this nice property. And I think, again, it's a it's a hack for selecting for intelligence.
Gus: And we wouldn't necessarily need this social cooperation between AIs because we don't have the limits that humans have. AIs do not have to fit their brains through a birth canal. We can simply make the model as huge as we want to. And therefore, perhaps the social cooperation aspect is no longer needed. Do you think that's true?
Connor: Yep, that's exactly that's exactly my point. Yeah, I can just think, talk to itself. It's much smarter. Why does it need other people?
Gus: Let's, let's get back to the to the question of benchmarks and and progress. So how do you think of measuring progress in AI? And specifically, how do you think of measuring whether we are making progress in narrow AI versus whether we're making progress in generality?
Connor: So this is an interesting question. And not just from a scientific perspective, but it's also interesting from a meta perspective for info hazardous reasons. Let's assume I had a one true way of measuring whether a system is getting closer to AGI or not. I would consider that to be very dangerous. I would consider the because it basically gives you a blueprint or at least a direction in North Star of how to build more and more powerful system. We're gonna talk about this more later, I'm sure. But I consider the idea of building AGI to be very fraught with risks. I'm not, I'm not saying it's not something we should do. It's also something that, you know, as obviously could be the best thing to ever happen to humanity. I'm just saying we have to do it right.
And the way things currently are looking with like our, you know, levels of AI safety and control, I think, you know, racing ahead to build AGI as fast as possible without being able to control or understand it is incredibly dangerous. So if I had such a metric, and, you know, I am not saying I do or I don't, I, you know, but if I did, I probably shouldn't tell you, I probably shouldn't tell people. And if someone out there has a true metric, I don't think necessarily is a good idea that to, you know, help people advance as quickly as possible. I can definitely say, though, that I don't know of anyone that has a metric that I think is good. I don't know of any, I think all metrics are terrible. I think they're, they're, they all have various problems. My pet peeve worst one is like GDP and economic growth. That's obviously the worst one.
Gus: Like, and why is that?
Connor: GDP, so I recommend everyone who's interested in this to, you know, pause right now, go on to Wikipedia and look up how the GDP is actually calculated. It's not what you think it is. So you would think the GDP, you know, measures like the value created or whatever. That is not the case. So you know, Wikipedia, for example, which I consider to be one of the crowning achievements of mankind, unironically, not joking. Wikipedia is like, it's become so normal to us, but Wikipedia is an anomaly. There are most universes do not get to Wikipedia. Wikipedia is so good. It's so good. Even so it has lots of flaws, sure, whatever, but it's so good and it's free. It's a miracle that something like this exists. Like, you know, normal market dynamics do not give you the Wikipedia. It's like, you know, just like the pure unadulterated idealistic autism of, you know, thousands of, you know, dedicated nerds working together and, you know, God bless their souls. Wikipedia being free, you know, contributes zero to GDP. There is no value whatsoever recorded from two societies. One has Wikipedia, one doesn't. No difference in GDP.
Gus: GDP would measure, for example, the companies working to implement the Linux operating system, but not the decades of work creating the Linux system. And so it doesn't capture all of these contributions that are extremely important to the world.
Connor: Yeah. So basically GDP is kind of like perversely instantiated to measure the things that don't change. All the things that change and become rapidly cheaper fall out of the GDP. They no longer contribute to the GDP. So ironically, the things that change the least that become the more expensive, you know, the whole Baumol's's cost disease thing. So like, you know, as software goes cheaper, it contributes less to GDP than, for example, you know, like housing or steel or construction, whatever, because that's still slow and takes time and is expensive. And as people become richer, you know, and, you know, software becomes proliferate and everyone has software, but then, you know, hardware, you know, and like, you know, building and houses as such becomes more expensive and they contribute more to GDP. So this is like the most perverse, like, so if we're looking for a software based thing that we think will rapidly make massive amounts of things cheaper, GDP is like designed to not measure that.
Gus: Yeah. If the classic example here would be something like a smartphone that captures a radio and a GPS and a map and so on, and perhaps thereby decreased GDP in some sense, at least. But so it's an interesting discussion, whether finding a good benchmark for AI progress would actually be hazardous. I think you're onto something because scientific progress often requires having some North Star to measure progress against. And so we can't really, that's like, before there's something to measure progress against, we can't have an established scientific field. And so establishing a benchmark would perhaps help AI researchers make progress in capability. What do we do then? Because we want to talk about the rate of AI progress. We want to talk about what's called AI timelines and how far along, how close to ATI we are. So how would you have that conversation without talking about benchmarks?
Connor: There's multiple ways we can approach this and multiple ways of like how blunt I should be about it. I mean, the blunt, the most blunt thing is like, bro, that's it. That's the answer. Like, excuse me, like, please take a look outside. Of course, that's not a very scientific answer. Let me turn down the snark a little bit and try to engage with the question more directly. So what are benchmarks actually? Sorry to be pedantic, but like, what is the purpose of a benchmark? So many people will say a benchmark is a scientific tool. That is something that like, you know, helps you do science or whatever. I actually disagree. I don't think benchmarks are fundamentally versatile. They might also have, they might also be useful for that. But I do not think that's actually what benchmarks are actually for.
Benchmarks are actually coordination technologies. They're actually social technologies. Benchmarks, what benchmarks are fundamentally for is coordination mechanisms. The kind of mechanisms you need to use when you're trying to coordinate groups of people around certain things. And you want to, you know, minimize cheating and freeloading, et cetera. A lot of technology actually, in a lot of science, once this is actually, this is a tangent, but I think it's a very interesting tangent that might be worth talking about more maybe now or later, is how much I think a lot of what modern people are missing, a lot of what modern science is missing is like a missing science of coordination technology. There's like, I have like a small list of like missing sciences, where like, if we were a better society, we would have these as like established disciplines. Another one is memetics, which is like sort of a science, but not really. And another one is like coordination technology and like, you know, coordination sciences and such, which like kind of exists, but like not really.
Gus: And it's super interesting, but I think we should talk about your predictions or your way of thinking about prediction, predicting AGI before we get into coordination.
Connor: Yeah. So I actually think these are very strongly interlinked, because what is my goal of predicting things? So there's several things why I want to mind, want to predict things. It might change my behavior, or I might want to try to convince other people to change their behavior. Those are two different things. It's actually important to separate those. One is epistemological and the other is coordination. So when you're trying to look at the truth, like is AGI coming? If that's the question you're interested in, that's an epistemological question. That's a question of science. This is a question that might be solved with benchmarks, but there might be other methods that you might use to solve this. Like science is not as clean as it's presented, like, oh, you have a false hypothesis or whatever. That's not how science actually works. That's like no one who actually does science actually works that way. And the second one is coordination.
So for coordination, benchmarks can be very useful, and even bad benchmarks can be useful for this. So if I'm interested in coordinating with people, it can be useful to say, here's a bunch of tasks that humans can solve and AI couldn't solve. But oh, look, now the AI can solve them. We've crossed the threshold. For that, benchmarks are actually useful. Like I actually think this is a useful application of even very, very poor benchmarks. If you're trying to understand AI though, ultimately you need a causal model of intelligence. Like if you don't have a model of how you think intelligence works or how you think intelligence emerges, you can't build a benchmark. You can't make predictions. You need a causal model. Everything bottoms up at some point that you have to make predictions about intelligence.
So me personally, the reason I don't care particularly much for most benchmarks is that I expect intelligence to have self-reinforcing memetic properties. So I expect phase changes. And the reason I expect this is I look at chimps versus humans, early humans versus later humans, modern humans versus pre-modern humans, and you see these changes that are memetic, not implementation-wise. So we've already seen some curious phenomena of this kind, like scaling laws and such. So we have these scaling laws, which I think a lot of people misunderstand. So scaling laws give you these nice curves which show how the loss of performance, quote unquote, on the model smoothly decreases as they get larger. These are actually terrible, and these actually tell you nothing about the model really. They tell you what one specific number will do. And this number doesn't mean nothing. There is some value in knowing the loss. But what we actually care about is can this model do various work? Can it do various tasks? Can it reason about its environment? Can it reason about its user? Can it do whatever? And what we can see in many of these papers is that there are phase changes. Is that as these models get larger, some tasks are unsolvable. They'll have some formal reasoning task or math task or whatever, and almost zero performance. And once the model hits a certain regime, they go through a certain amount of training, they reach a certain amount of size, suddenly they solve the problem in a very short amount of time.
Gus: What this looks like to us is that we have a model and it can't solve a particular task. And without changing anything fundamental about the model, making it bigger, giving it more training data, suddenly it develops what feels to us like a qualitatively new capability. Suddenly it can explain complex jokes or make up new jokes that have never existed before. So what I'm guessing your answer here is that because there are these phase changes, these periods of quick progress in AI, this means that if we look at a smooth rate of progress, that's not so interesting to see that the state of the art improves every third month or so. But what we really offer is what can the model do in an absolute sense.
Connor: Yes. And we can get these smooth curves, but these smooth curves are additions of, or sums of tens of thousands or millions of S-curves. And what those S-curves are, where they lie on this graph, how to measure those S-curves. Now if we were a sophisticated, epistemologically sensible scientific species, we would be studying that and we would have lots of causal models of intelligence and how we expect these to emerge and we'd have predictive theories. So currently there are no predictive theories of intelligence gain or task. There is no theory that says, oh, once it reaches 74.3 billion parameters, then it will learn this task. There's no such theory. It's all empirical. And we still don't understand these things at all. I think there's, so another reason I'm kind of against benchmarks, and I'm kind of like being a bit pedantic about this question, sorry about that, is because I think they're actively misleading in the sense that people present them as if they mean something, but they just truly, truly don't. A benchmark in a vacuum means nothing.
A benchmark plus a theory means something. There has to be an underlying causal theory. And so what usually happens is people present benchmarks with no stated underlying causal theory. The assumptions are just implicit. And this is really bad epistemology. This is really bad science. If you don't have an underlying predictive theory, then your number means nothing. It has to have a context. There has to be a theoretical framework in which this number means something. And a curious thing that we're seeing is, is that a lot of people don't even see this as a problem. You know, like someone presents a new benchmark and they're like, you know, this measures real intelligence and, you know, look, all the models can't solve my problem, but if they could, they would be intelligent or whatever. Or they don't even say that, it's just like implicit. That's really bad epistemology. This is really bad science.
The way it should be done is like, okay, hey, I have this causal theory. This is how things intelligence works. If this theory is true, it predicts that in the future, you know, if we do this, the intelligence will do that. Here's a benchmark that measures this. Now that's good science. Like it might be wrong, to be clear. It might be wrong. Like maybe the theory is just wrong and like its predictions are falsified, but that's okay. Like, you know, I mean, it's not great, but it's like, you know, I would be like respectful of that. But I think currently there's a, most of our benchmarks are mostly marketing coordination tools. They're not of the, this is what I would consider a scientific benchmark. This is something I would consider to be a theory, like an actual science. This is not the shape that like any benchmarks that I'm aware of have.
Gus: Do we have any idea what such a causal theory of intelligence might look like?
Connor: I mean, there are ideas, but there's no good ones. Like no one, because fundamentally we don't know how intelligence works. And tell, you know, people sometimes then bring up like, you know, AIXI and whatever. And I think I see is very not relevant for reasons we can get into if you're interested.
Gus: But what you're referring to here is a, is a theoretically optimal or mathematically optimal model of intelligence that that's it's, it cannot be implemented in actual hardware.
Connor: Yes, yes. You'd need an infinitely large computer to run it. It's actually worse than that, but let's not get into too much into that. So the problem with intelligence is, is that intelligence is a, and you remember our second earlier about the missing sciences. Here's another missing science embedded systems in, in the sense of computation. So there, there's some people have been realizing this. Wolfram, Stephen Wolfram deserves credit for being one of the first people to kind of think about this and that I was personally aware of. I'm sure he's not the first one. He's never the first one, even so he always claims he is. I actually like Wolfram even so he's a bit strange sometimes. Another one is MIRI. So the machine intelligence research institute, you know, back in like 2016 or something wrote this post about embedded agency, which is so sorry to quickly explain.
Embedded agency is this idea of what are the properties of an intelligence system or reasoner that's embedded within the larger universe. Basically all our previous like mathematical theories about intelligence, such as, you know, Solomonov induction, or even just concept of Bayesianism does not work in the embedded setting. So there's like some like really cutting edge, weird work, like infrabayesianism from Vanessa Kosoy that tries to like generalize these theories to the embedded space where you are trying to reason of an environment that is larger than yourself. So you can't model the whole environment. Also because you're inside of it, so you get into these like, you know, infinite loops. If you can model the environment, well you're inside the environment, so you have to model yourself. But if you're modeling yourself, you have to model yourself, modeling yourself. But if you're modeling yourself, modeling yourself, you have to model yourself, model yourself, et cetera. So you get into these infinite loops. And, you know, very, very, you know, good alien, very, very Lubyian kind of like.
Gus: So you're thinking this is a limit to our current models of, current causal models of intelligence that they can't do this self-reference.
Connor: Yes. And it's even worse than that. It's even worse than that. We don't have a widely accepted tradition of thinking about these kinds of things. So Wolfram calls this like the fourth pillar or whatever, which is of course a very silly marketing term, but like, whatever, like he kind of, you know, sees how the first type of science was like informal. You guys kind of like made stuff up. You kind of like maybe had a little bit of logic, like Aristotelian logic, but there wasn't really mathematics. Like the second phase of science was kind of like equations. You know, this is like mathematics. You have like formulas and, you know, derivatives and like all these kinds of things. This was like really pretty useful for building models. But then in the third phase was yet computational models. So you generalize from equations to just like arbitrary programs. And now he calls the fourth paradigm multi-computation, which is the same as embedded agency ultimately, was the idea of both of having non-deterministic programs and also having observers that sample from this non-deterministic program.
Rather than having a program that you run and you get an output, you have a non-deterministic program which produces, you know, some space, some large space outputs. And then you sample from the space using some kind of smaller program. And this is what intelligence actually is. We are small embedded programs running inside of this large non-deterministic program we call the universe. And so this is the actual like correct ontology for thinking about intelligence. And this is just not something that is widely like, like even like a ontological concept that like exists in the head of many people. Like I'm not saying no people, like some scientists do think about like, you know, like distributed systems people think a lot about this kind of stuff and like various complexity sciences think about this kind of stuff. I'm not saying no one thinks about this stuff, but it's not like a widely accepted field. And at least to me, again, this is just like all hot takes, you know, podcasts speak and whatnot, but like, I think that like a good theory of intelligence has to be formulated to some degree in this kind of, some formalism like this, some kind of ontology like this. And it has, and so then it gets even worse.
So the next worst problem is, is that intelligence obviously interacts with the environment. So to have a good causal model of intelligence, you have to have a good causal model of its, of its environment. So like, for example, let's say I have a system, which is quite dumb, not very smart, but it happens to an existing environment, which includes a computer, which is super intelligent and it's helping it. Well, well now the system obviously is going to be able to figure out some like much more complicated thing because it has like a teacher, right? So if I want a predictive model of how smart the system is going to be after n steps or whatever, I'm going to have to have a model of the teacher too, because otherwise, you know, I won't be able to predict. So this is a really hard problem. And so, you know, I'll bring this all back to step one. Predicting intelligence is kind of a fool's errand. It's like, like we can make very, very weak predictions. And because just our theoretical understanding are like models, aren't pure ontologies of what intelligence is, but how it develops, that means are so primitive, you know, okay, maybe if, you know, we had to, you know, a hundred years more time to do philosophical progress and statistical, you know, theory progress or whatever. Yeah, maybe that. All right. Now I kind of trust like sensible gut feelings and like, you know, empirics more than I do any theory that I'm aware of or any predictive theory that I'm aware of. Maybe there are some I'm not aware of, but.
Gus: And in this context, you mean gut feelings in the sense of a person that's been immersed in the research and are actually reading the papers and so on, of course, as opposed to, you know, someone taking a look at this for the first time and thinking, you know, oh, where's this going? So you would, you would, oh, no.
Connor: It's complicated. Okay. I mean, the truth of the matter is, is that in my experience, a lot of people who know nothing about AI have been better at predicting AI than people who have been studying it very carefully.
Gus: See, that's interesting.
Connor: I mean, I'm being snarky, but there is a reoccurring phenomena, especially in the AI safety world, where I'll be like, I'll talk to my mom and I'll be like, so this is AI stuff, right? Intelligence. Like, yeah. And like, intelligence is super important, right? And she's like, yeah, yeah, of course. And like, you know, because you solve all those problems, like, you know, versus chimps. Like, yeah, yeah, of course. Super important. And I'm like, well, you know, you could probably teach computers how to be smart, right? I'm like, yeah, yeah, it makes sense. And then if we teach these computers to be super, super smart, they'll, you know, be doing all this, all these crazy things. Like, yeah, yeah, it seems like, yeah, well, we don't know how to control them. So, so maybe bad things will happen. Like, yeah, of course, obviously. Like, of course.
Gus: I actually, I had the same reaction when I brought up AI safety to my mom. She, she's not at all that interested in, in artificial intelligence and so on, but she actually discovered in a sense, the problem, the control problem, or the alignment problem herself from me just talking about smarter than, than human artificial intelligence. So perhaps, perhaps it's more intuitive than, than we, we, we normally think.
Connor: So this is, so this is the me being snarky part and where it's absurdly obvious, like AI safety is treated at this like, esoteric, like weird, like, you know, like requires all these like massive complex arguments to like stand up. And like, I think, I feel like I'm, I'm, I'm like, I'm being, I'm going crazy. Like, what do you mean? This is like such an obvious, simple argument that it requires so few little ontological baggage. But once you talk to actually smart people about this thing, you know, people with high IQs, let's say, not necessarily smart, let's say high IQ people. You have, you know, made this their identity to be scientists, they're AI researchers, they're pro progress, they're pro technology. A lot of them will be pretty damn resistant to these arguments.
And so this is a classic, you know, hate to call back to Kahneman, but he was right about this where, you know, as people get smarter, that doesn't mean they're more rational. That doesn't mean they're better at epistemology. It often means in fact, that they're better at tricking themselves because they're so smart that, you know, they can, you know, come up with better rationalization for what they want to believe anyways. This also goes back to the Machiavellian intelligence hypothesis that they like, you know, mentioned earlier, where one of the theories about why humans were selected so heavily. So normally in nature, when evolution selects for something so heavily, that like everything else gets thrown out. And you know, like, you know, you're like, you know, even birth becomes difficult, and it's like a massive thing. It's usually sexual selection that causes that. So sexual selection is when some arbitrary trait becomes desirable to one or both the sexes. So the other sex like optimizes for that super, there's like peacock tails. So like your peacock tails are ridiculous. Like if I was an alien and you explained evolution to me, and I was like, okay, show me some of these animals. You show me a peacock. I'd be like, what the fuck? Like what made, why is this? It's like, it's ridiculous.
So sexual selection is wild and was one of the challenges to like early Darwinian evolution actually, or like one of the things that Darwin got right later on. And people didn't really believe that like Darwin did get right. And so one of the theories is that human intelligence is actually a sexual trait is that for some reason, like for example, gossip or oratory or like storytelling or whatever became a trait that was selected for sexually. And therefore people optimize it super hard. So like one version is, you know, be like, oh, it's about art. You know, we got some, the cutesy version is humans were selected so hard to create art and sing and dance and whatever. The more, you know, pragmatic idea is that is Machiavellian is that we were selected for doing politics and for backstabbing our rivals, for making ourselves look good, lying to people, deceiving, manipulating, you know, stuff like that. It seems pretty plausible to me if I look at human, you know, general behaviors.
Gus: And but yeah, I would love to hear your take on simply how do you think about AI, AGI timelines? What is, yeah, right.
Connor: Actual topic. How do I think about timelines? It's a good question. And the obvious truth is I of course don't really know, you know, it's impossible because I also don't have, I think I have a better folk theory of intelligence than most people do, but it's still a folk theory. I'm not like under any illusions that like I actually understand intelligence. I don't actually know what's going on, but I have some folk theories, which is why you hear me talking about chimps and embedded agency and stuff. Like these are things I think a lot of people don't mention when they talk about, like, I'm assuming you taught one, you expect to be to talk about like scaling laws and neural networks and stuff.
Gus: It's often the things that come up spontaneously are the things that are most important. And so this is great.
Connor: Yeah, and it's not just, and it's not just spontaneous, obviously, like my models of intelligence are not solely informed by neural networks. My theories of intelligence are informed by humans and culture and chimps and, you know, and also neural networks and computational complexity and stuff like this. Like I think all of these things are important. So how do I think about it? Timelines. The truth is that I can't, I can't give you a full causal, my full causal model, because my full causal model includes what I think AI is currently missing. And you know, I might be totally wrong. I probably completely wrong. You know, like everyone thinks they know how to build a AGI, right. And like, I'm probably wrong about what I think, but on good info hazard practices, I should tell you like, oh, we have this many years because I expect it's gonna take two years to figure that part out and three years to do that one. But my model is close to that.
So like, I look at how I think human intelligence works. I look at like how I think it's different from chimp intelligence. I look at, you know, how the brain works, what's going on inside of it and so on. And it's just like, it's complex, sure. But it's not that complicated. Like you know, it's like, it's not like non understandable. And like the more progress you make, the more simple it seems to be. And so I look at like, okay, what are like fundamental limitations. And it's very hard for me to come up with like, limits are so hard that I like, can't have any doubt in them. And then I also look at, you know, my own timelines and previous timelines were like, every time I'm like, well, you know, surely it will take this long until this happens. And then it happens. And like, well, okay, but I can't do x, but then they do x. And then I'm like, so around 2019 or something, I was just like, okay, screw it, I'm updating all the way. And then I just updated, you know, as the Bayesian, you know, as the Bayesian, conservative conservation of expected evidence goes, I was up, I was surprised so many times by how simple things were, and how often how simple things work, how fast they go, that I was just expecting to be surprised, which is non rational, if you expect in the future, you will see evidence that will make you update in one direction, just update in that direction. And so that's what I've done.
So I'm now at very short timelines. I think that if we don't see AGI very soon, it's going to be for contingent reasons, not for fundamental reasons. I think we have hardware, I think we have most of the software, we're about we're like two to five insights away from like full blown AGI, like the strong version of AGI. Now those two to five things are not impossibly hard. You know, I expect them to be as hard as inventing transformers, which is not easy, you know, but it's doable. It's a thing humans can do. So I think you can get all five of these in one year, if you're unlucky.
You know, I think like, if you know, people search for the right direction, worked on the right things, did the right experiments, were good at epistemology and had good causal theories, we could do all of this in one year, and then you know, that would be it. But the market is very inefficient. You know, people are very inefficient, science is very inefficient. So it could also take longer. So the joke timeline I usually give people is like 30% in like the next four or five years, 50% by like 2030, or like 2035, something like that. 99% by 2100. If it's not by 2100, something truly fucked up has happened. I mean, we had like a nuclear war or something. And 1% has already happened, but we haven't noticed yet.
Gus: It's in a lab somewhere, perhaps.
Connor: It's in a lab somewhere. And like, people are like, oh, you know, it's Oh, look, it's cute. But like, you know, it's fine. Like, I mean, as I said, like, I think it's not impossible that like, if we had used GPT-3 different, or like you gave GPT-3 to aliens who have like good theories of intelligence, they could just like jury rig it into an AGI because they like know how to do that. And they were just like missing like, they're like, Oh, look, you, you did the florbal max wrong. And they just like redo that. And then it's, you know, AGI. And I'm like, 1% or less that that's true. But I can't dismiss it. Like, it does not seem impossible to me.
Gus: If you believe there's a say 25% probability that we will get to AGI within five years, are you betting against the market? Specifically, are you do you disagree with people who are valuing stocks a certain way? Should AI companies be higher have higher valuations and companies at risk of disruption have lower valuations? Yeah, because the market is often quite good at pricing assets. But you just mentioned that the market is inefficient. So so yeah, tell me about this.
Connor: The efficient market hypothesis and its consequences have been a disaster for humanity. The idea that the market is efficient is a very powerful and potent meme. And it has a lot of value in this idea. But I think people don't actually understand what it means and get confused by it. I think even the people who invented it didn't really understand it. I like like Eliezer's like writings and inadequate equilibria about like free energy and such more. But let me say a few things about efficient market. The efficiency of a market is a contingent phenomenon. It's an observer dependent phenomenon. So for you, you know, dear listener, you know, who is, you know, sitting on their couch and you know, isn't a finance person or whatever, then yes, from your perspective, the market will be efficient for the most part. The most part, you can't, you know, put $10 million into, you know, GameStop, you know, call auctions or whatever and make a bunch of money. Yes. But that is observer dependent.
So if you, the reader are actually, you know, the smartest, you know, Jane Street, FinTech trader in the entire world, you may well be able to put $10 million into the market and take $20 million out. That's a contingent phenomenon. It's an observer dependent phenomenon. And this is also why, for example, if an alien from outer space came with, you know, 3000 million IQ, I expect they could extract arbitrary amounts of money from the market. They could just money pump you forever. There's just nothing you can do about that. So it's not just intelligence based either. So let's say you're the assistant of, you know, a CEO at some big company, you get a phone call and you say the CEO had a heart attack. He died on you.
The market isn't so the efficient market hypothesis says, well, trading on this wouldn't help because surely if this was problematic, it would have already priced in. Of course, that's silly. Someone has to price it in. You're that person. You can make a lot of money for pricing this in. Of course, it would not be legal because it's insider trading, whatever. But I assume it was the market is now no longer efficient from your perspective because you have information that allows you to extract reliable money from the market by contributing that. So, of course, once you've traded on it, then it no longer then it becomes efficient again. You're trading with it makes it efficient. So yes, if I had, you know. Access to a large amount of money or like I made a lot of people rational, I expect, you know, I had my opinion. Then, yes, the market would change. The market would become efficient and would trade in regard to this.
Obviously, I think I am like the assistant in this scenario is that like, you know, I can see the CEO dead on the floor and no one else has noticed yet. And no one believes or like very few people. There is a more complex version of this as well, where, as you said, like, OK, should like AI companies be valued or whatever? That's not obviously true to me. And the obvious reason is just a control problem. Like even if the if the market was like would price my opinions, even then I would be like, hmm, maybe we should short all these companies because they're going to kill everybody. So, you know, it's not obvious how you would price these kind of things. Markets are very bad, actually, at pricing, at least the way we currently do markets. There are ways to fix these problems or at least address them the way we currently do market. But they're actually very bad at dealing with black swan scenarios like low probability, weird tail end, high impact scenarios.
A lot of this is a classic, like, you know, Nassim Taleb kind of critique of these markets and whatever is that they trade volatility, like the trade around volatility, where it looks they look more stable and they look like efficient in a short amount of time. But over long periods of time, they have blow up risks. They trade for blow up risks. And I think basically I'm saying this is the black swan. Is this like the way things are currently traded is we're like, it's a massive bubble is that like, obviously, like, assuming we survived AGI. And that was like a thing, I believe the market would survive that. Well, then, yeah, I could make an absolute killing from options right now. Like, man, I would. Oh, man, I can make a trillion dollars, you know, shorting and longing this light, you know, putting up options like the old super leverage option calls. Yeah, obviously, I just don't think the markets can survive that.
Gus: Is there a way to make the market work for us if we want to have accurate predictions here? So perhaps the best option right now is prediction markets, but those aren't there. There's not that much money in it in those markets. How seriously do you take prediction markets?
Connor: I think prediction markets, I mean, friends sometimes joke about how, like, whenever we see a thing we really like and it's not popular, we always joke it's anthropics. Is that like a sane society, you know, would have already developed AGI and already killed itself. So therefore, you know, that's why our society is insane. It's obviously a joke. Don't take that too seriously. Prediction markets are one of those things. It's like, of course, prediction markets are a good idea. Like you just look at them once and like, duh.
Like it's the fact that the US outlaws real money prediction markets is the most galaxy brain hilarious thing. Like ever since they also outlawed, you know, building houses, which is also just so unimaginably galaxy brains, you know, like Silicon Valley, biggest generator of money ever. OK, let's outlaw people living there. We did it, boys. We've saved the economy like unimaginable. So I think prediction markets are simply so there's problems with prediction markets, you know, blah, blah, blah. But like, there's so obviously a perimeter improvement on like everything they're trying to do is that the fact we don't use them is a just hilarious sign of civilization in adequacy.
Gus: Do you think that prediction markets are a bubble of people with short AGI timelines because they look they look pretty that the people who are interested in, say, meticulous, for example, whether that's a prediction market or not, we can discuss. But the people who are who are interested in these prediction markets are often also people who are into AI safety and are interested in AGI and so on. Do you think that's a bubble?
Connor: I mean, yeah, it is true that if you have inside information is usually suspiciously that people tend to have similar opinions. We see the CEO dead. Well, all the people who saw the CEO dead have been shorting this company. I think it's a bubble. So yes, it could be a bubble or they could just have information. So I've seen GPT four right. You know, I've seen what it can do and stuff. I know quite a lot about what it can do compared to like current models. So like if such markets existed, yeah, I could like make money. I'll make a lot of money there because I know a lot about it. And it's similar with like I think there's a massive price going on here, obviously, it's like, sure, there is a there is a correlation between people going on these markets and being into like short timelines and so on.
But the question is, is this that they just happen to have this arbitrary preference or is the actual causal model rational people who investigate the evidence tend to have short timelines because of this? I think it's the latter. Of course, I think that obviously, so you might dismiss this opinion. But obviously, I think it's just if you actually take these things seriously, if you actually look into what these things can actually do, if you don't just, you know, read the latest Gary Marcus, you know, whatever piece and but you actually like interact with the technology and actually think about intelligence in a causal way, you'll notice the massive mispricing going on. And it's just like massive inefficiencies. And these inefficiencies are, you know, like, they're not random, right? Like, it makes sense why these inefficiencies exist. And this is why real money prediction markets are so important.
The reason real money is so important for prediction markets is because it attracts the sociopaths. And that's super important. You can't have a good market without the sociopaths, you need them. So because the sociopaths will price anything, they don't give a shit, you know, they don't like, you know, because like, if you have markets that don't include sociopaths, you're going to get, you're always going to get mispricings based on emotions. And like aesthetics, you're always going to get that, you know, people are going to, you know, buy things because they think it's good, you know, because it looks nice, or because it's like moral or like, you know, socially acceptable, whatever. Sociopaths don't give a crap, you know, and they'll just trade you into the ground. And that's what you want from an accurate prediction market. So, you know, a lot of, you know, you know, these forecasting sites, you know, have people who are super rational, not calling them sociopaths, but I'm saying, you know, they're, they're nice and efficient, whatever. But what you really want, what I want trading on my prediction markets is, you know, just like some cutthroat, you know, you know, Wall Street, you know, Jane Street, you know, turbo, you know, you know, math sociopath, like, those are the people you actually want making these calls. And they usually do it for money.
Gus: But you're saying these options, or these, yeah, these options for betting aren't available to you. And so is there a way to set up a bet? Because you know, there's the saying that betting is a tax on BS. And it might be nice if there's someone, someone listening to this, who thinks you're totally wrong, right? What could they do to earn money from you being wrong? Or the other way around, of course?
Connor: That's a great question. I'd be happy to make predictions about, for example, what GPT-4 can do. I mean, for obvious reasons, because I have an insider advantage there. But I would also be happy to make predictions about like GPT-5, or like, like, can it do X with a certain amount of, you know, effort, we can actually formalize this in like bits of curation, if you want. So we can make that pretty precise. The truth of the matter is, I don't actually care enough of changing other people's mind to actually make these bets. If someone offered me like a pre-made like set of bets, I would probably accept it.
But truth of the matter, again, bets are coordination mechanisms. Remember we talked before about benchmarks, coordination. Bets are not actually, they're, in this case, they can be epistemological, they can be valuable, but they're also ultimately coordination mechanisms. So like, if you present me with new information that changes my mind, or like, if you present me with a bet, you know, that's like, hey, I know something that you don't know. And then I'm like, and you tell me that I just update, I'll be like, great, I'll just update. I'm like, not dogmatic here, right? If you just show me, hey, we did this experiment, we have this causal model of intelligence. Here's the causal model. Here's why it doesn't, it predicts that, you know, you know, intelligence won't increase as quickly. And I'll be like, huh, okay, I update all the way, because that's what you did, you know, as the saying goes, when I hear new facts, I change my mind. What do you do?
Gus: Fantastic.
Connor: All right.
Gus: So let's, let's end it here and then talk about AI safety next.
Related episodes
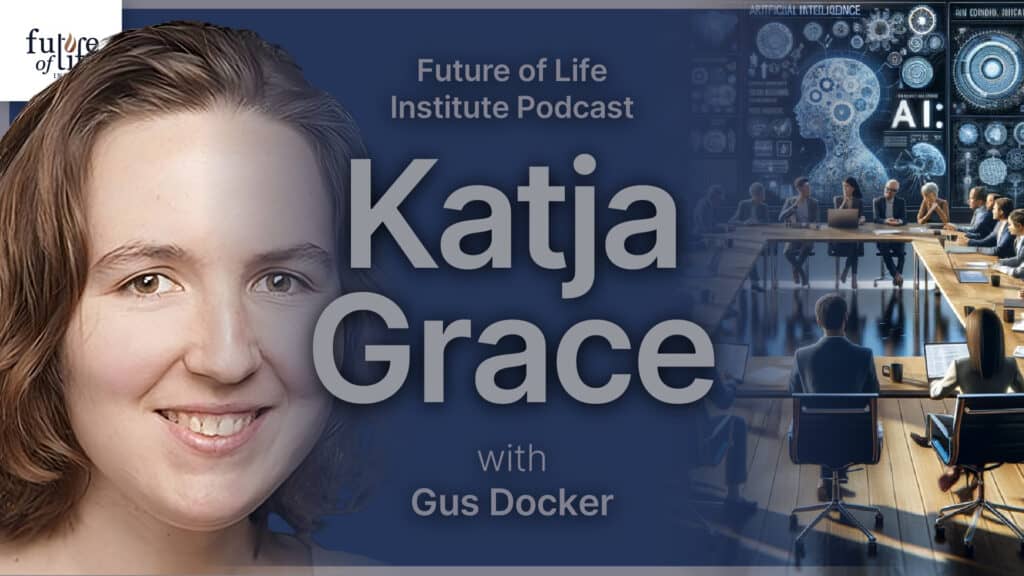
Katja Grace on the Largest Survey of AI Researchers
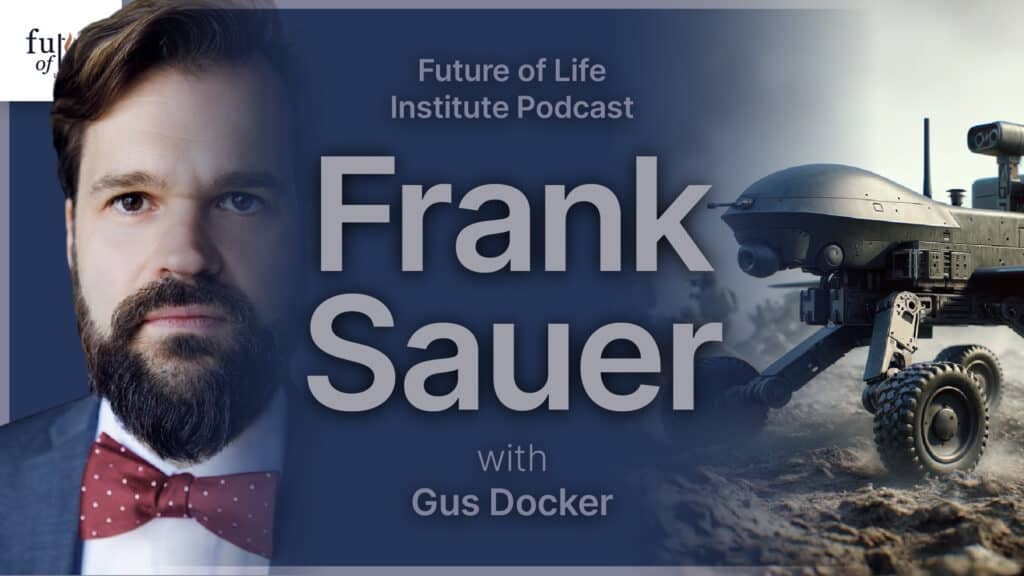
Frank Sauer on Autonomous Weapon Systems
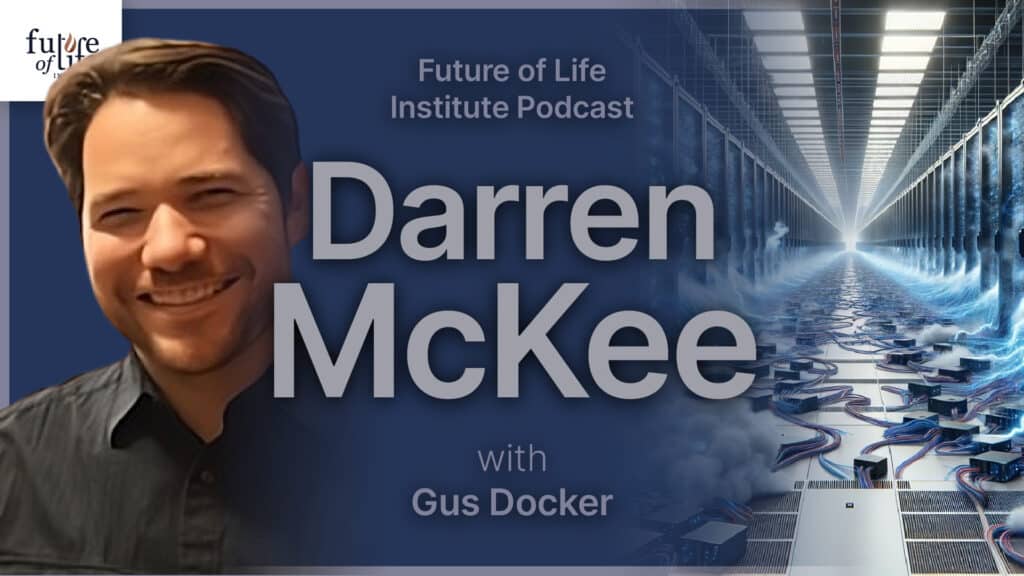