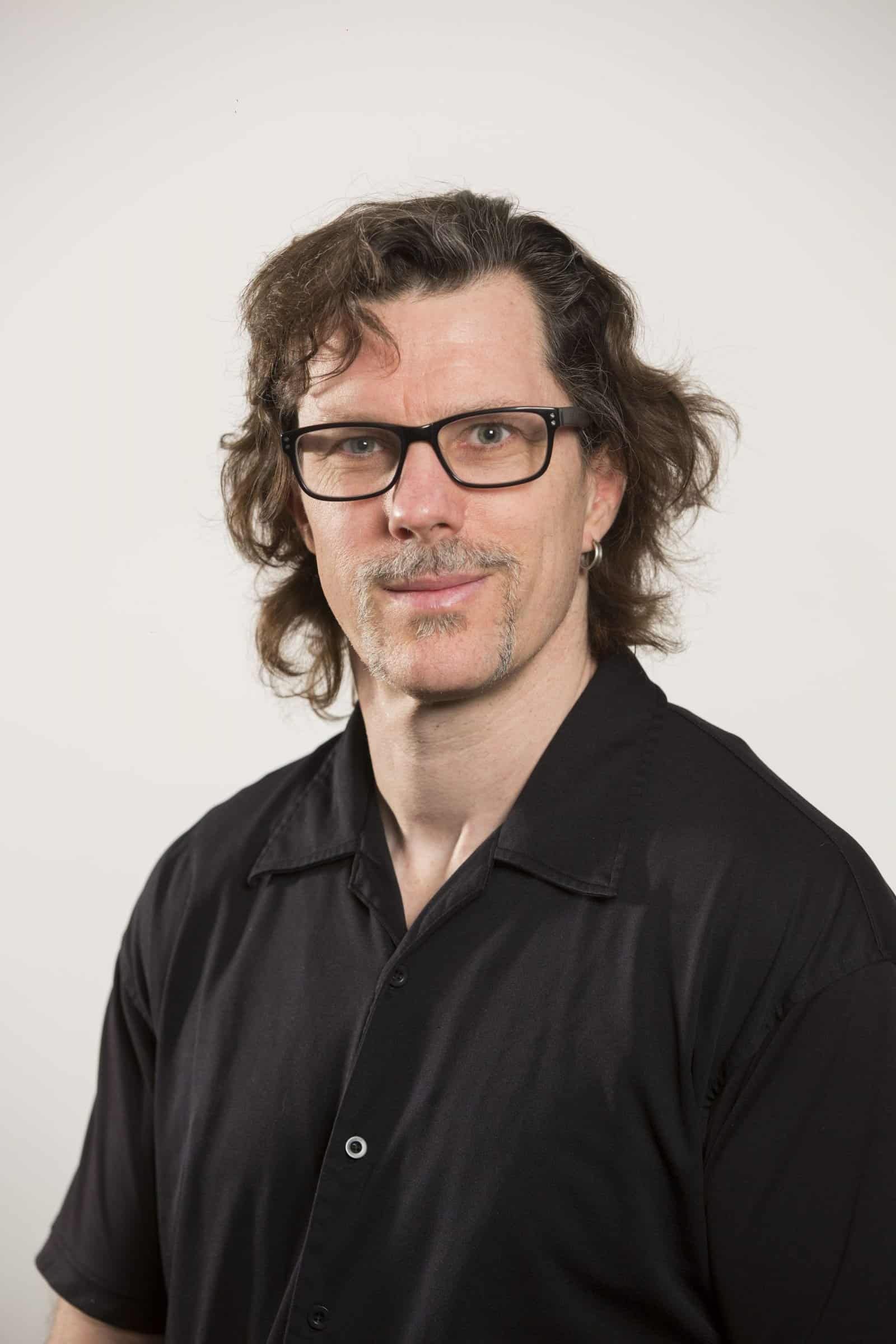
Peter Vamplew
Why do you care about AI Existential Safety?
AI has the capacity to transform our world for the better, but also the potential to cause great harm. Recent years have seen large leaps in the capabilities of AI systems (particularly those based on machine learning) and in the complexity and social impact of the problems to which they are being applied, and we are already seeing examples of the negative outcomes which can arise when the AI is biased or overlooks critical factors. As an AI researcher I believe it is vital that we focus on how to design, apply and regulate AI systems to reduce these risks and to maximise the benefits for all humanity.
Please give one or more examples of research interests relevant to AI existential safety:
My main focus is on the risks posed by AI based on unconstrained maximisation of a reward or utility measure (as in conventional reinforcement learning), and the role which multiobjective approaches to reward/utility can play in mitigating or eliminating these risks. I am also interested in reward engineering methods which will support the creation of robust reward structures which are aligned with our actual desired outcomes, and methods for automatically learning human preferences, ethics etc and incorporating these into AI agents.
Examples of my publications in this area include:
- Vamplew, P., Dazeley, R., Foale, C., Firmin, S., & Mummery, J. (2018). Human-aligned artificial intelligence is a multiobjective problem. Ethics and Information Technology, 20(1), 27-40.
- Vamplew, P., Foale, C., Dazeley, R., & Bignold, A. (2021). Potential-based multiobjective reinforcement learning approaches to low-impact agents for AI safety. Engineering Applications of Artificial Intelligence, 100, 104186.
- Mannion, P., Heintz, F., Karimpanal, T. G., & Vamplew, P. Multi-Objective Decision Making for Trustworthy AI, The 1st International Workshop on Multi-objective Decision Making