2018 AGI Safety Grant Program
Grants archive
Technical Abstract
As technology develops, it is only a matter of time before agents will be capable of long term (general purpose) autonomy, i.e., will need to choose their actions by themselves for a long period of time. Thus, in many cases agents will not be able to be coordinated in advance with all other agents with which they may interact. Instead, agents will need to cooperate in order to accomplish unanticipated joint goals without pre-coordination. As a result, the ``ad hoc teamwork'' problem, in which teammates must work together to obtain a common goal without any prior agreement regarding how to do so, has emerged as a recent area of study in the AI literature. However, to date, no attention has been dedicated to the moral aspect of the agents' behavior. In this research, we introduce the M-TAMER framework (a novel variant of TAMER) used to teach agents the idea of human morality. Using a hybrid team (agents and people), if taking an action considered to be morally bad, the agents will receive negative feedback from the human teammate(s). Using M-TAMER, agents will be able to develop an "inner-conscience'' which will enable them to act consistently with human morality.
Technical Abstract
Our goal is to understand how Machine Learning can be used for AGI in a way that is 'safely scalable', i.e. becomes increasingly aligned with human interests as the ML components improve. Existing approaches to AGI (including RL and IRL) are arguably not safely scalable: the agent can become un-aligned once its cognitive resources exceed those of the human overseer. Christiano's Iterated Distillation and Amplification (IDA) is a promising alternative. In IDA, the human and agent are 'amplified' into a resourceful (but slow) overseer by allowing the human to make calls to the previous iteration of the agent. By construction, this overseer is intended to always stay ahead of the agent being overseen.
Could IDA produce highly capable aligned agents given sufficiently advanced ML components? While we cannot directly get empirical evidence today, we can study it indirectly by running amplification with humans as stand-ins for AI. This corresponds to the study of 'factored cognition', the question of whether sophisticated reasoning can be broken down into many small and mostly independent sub-tasks. We will explore schemes for factored cognition empirically and exploit automation via ML to tackle larger tasks.
Technical Abstract
Artificial general intelligence (AGI) may be developed within this century. While this event could bring vast benefits, such as a dramatic acceleration of scientific and economic progress, it also poses important risks. A recent survey shows that the median AI researcher believes there is at least a one-in-twenty chance of a negative outcome as extreme as human extinction. Ensuring that AGI is developed safely and beneficially, and that the worst risks are avoided, will require institutions that do not yet exist. Nevertheless, the need to design and understand these institutions has so far inspired very little academic work. Our programme aims to address several questions that are foundational to the problem of governing advanced AI systems. We will pursue four workstreams toward this aim, concerning the state of Chinese AI research and policy thought, evolving relationships between governments and AI research firms, the prospects for verifying agreements on AI use and development, and strategically relevant properties of AI systems that may guide states' approaches to AI governance. Outputs of the programme will include academic publications, workshops, and consultations with leading actors in AI development and policy.
Technical Abstract
An AI race for technological advantage towards powerful AI systems could lead to serious negative consequences, especially when ethical and safety procedures are underestimated or even ignored. For all to enjoy the benefits provided by a safe, ethical and trustworthy AI, it is crucial to enact appropriate incentive strategies that ensure mutually beneficial, normative behaviour and safety-compliance from all parties involved. Using methods from Evolutionary Game Theory, this project will develop computational models (both analytic and simulated) that capture key factors of an AI race, revealing which strategic behaviours would likely emerge in different conditions and hypothetical scenarios of the race. Moreover, applying methods from incentives and agreement modelling, we will systematically analyse how different types of incentives (namely, positive vs. negative, peer vs. institutional, and their combinations) influence safety-compliance behaviours over time, and how such behaviours should be configured to ensure desired global outcomes, without undue restrictions that would slow down development. The project will thus provide foundations on which incentives will stimulate such outcomes, and how they need to be employed and deployed, within incentive boundaries suited to types of players, in order to achieve high level of compliance in a cooperative safety agreement and avoid AI disasters.
Technical Abstract
Many paradigms exist, and more will be created, for developing and understanding AI. Under these paradigms, the key benefits and risks materialise very differently. One dimension pervading all these paradigms is the notion of generality, which plays a central role, and provides the middle letter, in AGI, artificial general intelligence. This project explores the safety issues of present and future AGI paradigms from the perspective of measures of generality, as a complementary dimension to performance. We investigate the following research questions:
1. Should we define generality in terms of tasks, goals or dominance? How does generality relate to capability, to computational resources, and ultimately to risks?
2. What are the safe trade-offs between general systems with limited capability or less general systems with higher capability? How is this related to the efficiency and risks of automation?
3. Can we replace the monolithic notion of performance explosion with breadth growth? How can this help develop safe pathways for more powerful AGI systems?
These questions are analysed for paradigms such as reinforcement learning, inverse reinforcement learning, adversarial settings (Turing learning), oracles, cognition as a service, learning by demonstration, control or traces, teaching scenarios, curriculum and transfer learning, naturalised induction, cognitive architectures, brain-inspired AI, among others.
Technical Abstract
A hallmark of human cognition is the flexibility to plan with others across novel situations in the presence of uncertainty. We act together with partners of variable sophistication and knowledge and against adversaries who are themselves both heterogeneous and flexible. While a team of agents may be united by common goals, there are often multiple ways for the group to actually achieve those goals. In the absence of centralized planning or perception and constrained or costly communication, teams of agents must efficiently coordinate their plans with respect to the underlying differences across agents. Different agents may have different skills, competencies or access to knowledge. When environments and goals are changing, this coordination has elements of being ad-hoc. Miscoordination can lead to unsafe interactions and cause injury and property damage and so ad-hoc teamwork between humans and agents must be not only efficient but robust. We will both investigate human ad-hoc and dynamic collaboration and build formal computational models that reverse-engineer these capacities. These models are a key step towards building machines that can collaborate like people and with people.
Technical Abstract
Recent developments in artificial intelligence (AI) have enabled us to build AI agents and robots capable of performing complex tasks, including many that interact with humans. In these tasks, it is desirable for robots to build predictive and robust models of humans’ behaviors and preferences: a robot manipulator collaborating with a human needs to predict her future trajectories, or humans sitting in self-driving cars might have preferences for how cautiously the car should drive. In reality, humans have different preferences, which can be captured in the form of a mixture of reward functions. Learning this mixture can be challenging due to having different types of humans. It is also usually assumed that these humans are approximately optimizing the learned reward functions. However, in many safety-critical scenarios, humans follow behaviors that are not easily explainable by the learned reward functions due to lack of data or misrepresentation of the structure of the reward function. Our goal in this project is to actively learn a mixture of reward functions by eliciting comparisons from a mixed set of humans, and further analyze the generalizability and robustness of such models for safe and seamless interaction with AI agents.
Technical Abstract
The agent framework, the expected utility principle, sequential decision theory, and the information-theoretic foundations of inductive reasoning and machine learning have already brought significant order into the previously heterogeneous scattered field of artificial intelligence (AI). Building on this, in the last decade I have developed the theory of Universal AI . It is the first and currently only mathematically rigorous top 'down approach to formalize artificial general intelligence. This project will drive forward the theory of Universal AI to address what might be the 21st century's most significant existential risk: solving the Control Problem, the unique principal-agent problem that arises with the creation of an artificial superintelligent agent . The goal is to extend the existing theory to enable formal investigations into the Control Problem for generally intelligent agents. Our focus is on the most essential properties that the theory of Universal AI lacks, namely a theory of agents embedded in the real world : it does not model itself reliably, it is constraint to a single agent, it does not explore safely, and it is not well-understood how to specify goals that are aligned with human values.
Technical Abstract
Economists, having long labored to create mathematical tools that describe how hyper-rational people behave, might have devised an excellent means of modeling future computer superintelligences. This guide explains the uses, assumptions, and limitations of utility functions in the hope of becoming a valuable resource to artificial general intelligence (AGI) theorists. The guide will critique the AGI literature on instrumental convergence which theorizes that for many types of utility functions an AGI would have similar intermediate goals. The guide considers the orthogonality thesis, which holds that increasing an AGI's intelligence does not shrink the set of utility functions it could have. This guide explores utility functions that might arise in an AGI but usually do not in economic research, such as those with instability, always increasing marginal utility, extremely high or low discount rates, those that can be self-modified, or those with preferences that violate one of the assumptions of the von Neumann-Morgenstern utility theorem. The guide considers the possibility that extraterrestrials have developed computer superintelligences that have converged on utility functions consistent with the Fermi paradox. Finally, the plausibility of an AGI getting its values from human utility functions, even given the challenge that humans have divergent preferences, is explored.
Technical Abstract
Reward specification, a key challenge in value alignment, is particularly difficult in environments with multiple agents, since the designer has to balance between individual gain and overall social utility. Instead of designing rewards by hand, we consider inverse reinforcement learning (IRL), an imitation learning technique where agents learn directly from human demonstrations. These techniques are well developed for the single agent case, and while they have limitations, they are often considered a key component for addressing the value alignment problem. Yet, multi-agent settings are relatively unexplored.
We propose to fill this gap and develop imitation learning and inverse reinforcement learning algorithms specifically designed for multi-agent settings. Our objectives are to: 1) develop techniques to imitate observed human behavior and interactions, 2) explicitly recover rewards that can explain complex strategic behaviors in multi-agent systems, enabling agents to reason about human behavior and safely co-exist, 3) develop interpretable techniques, and 4) deal with irrational agents to maximize safety. These methods will significantly improve our capabilities to understand and reason about the interactions among multiple agents in complex environments.
Our other grant programs
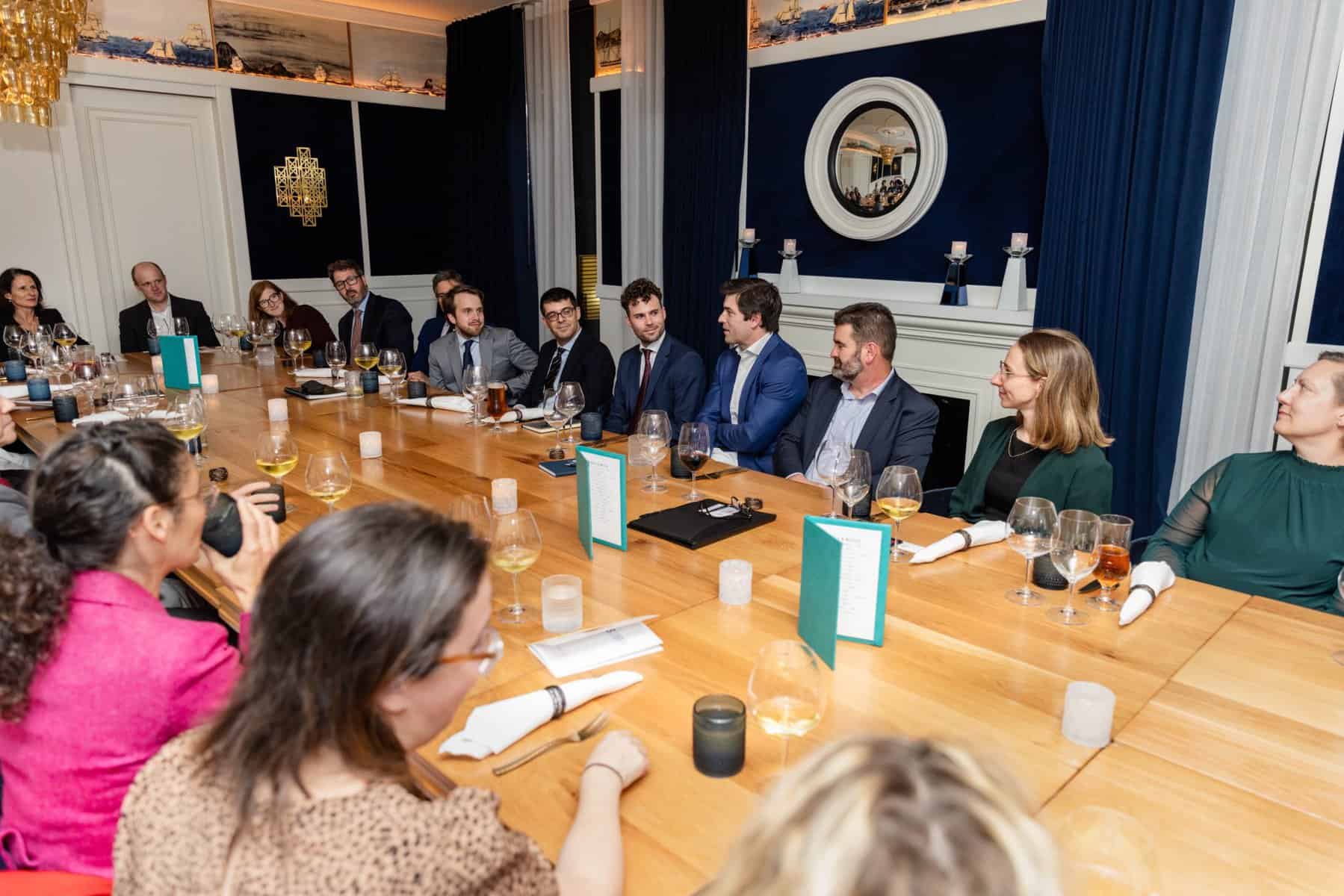
2024 Grants
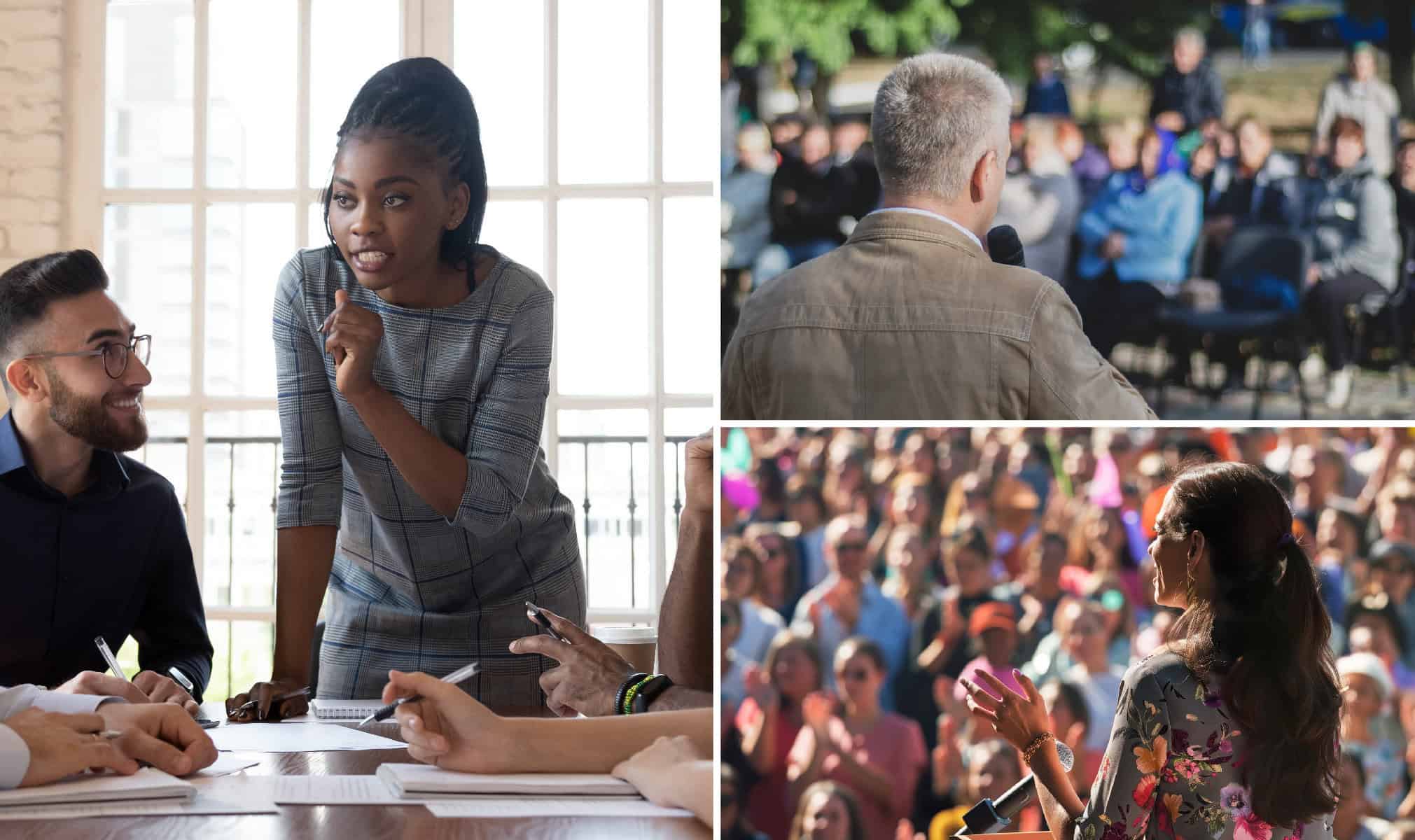
Multistakeholder Engagement for Safe and Prosperous AI
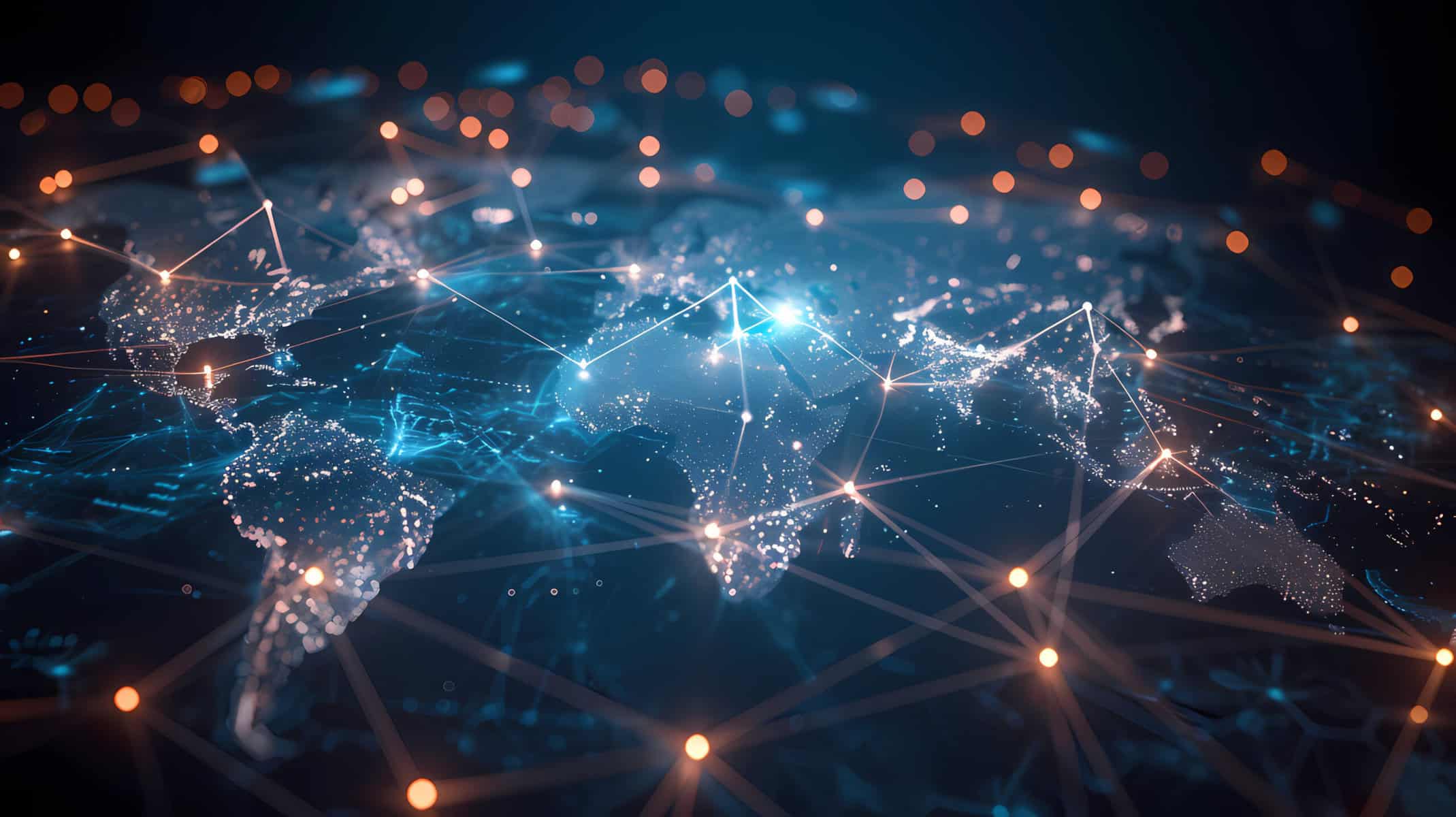