A Summary of Concrete Problems in AI Safety
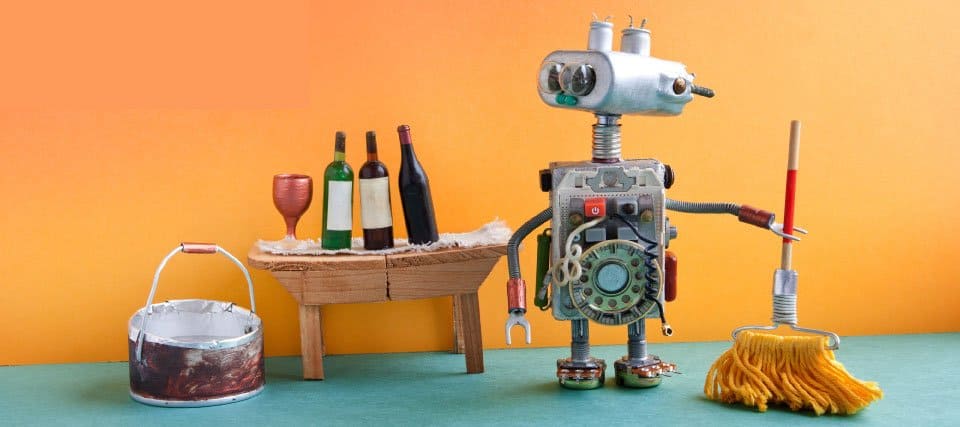
Contents
By Shagun Sodhani
Click here to see this page in other languages: Russian
It’s been nearly two years since researchers from Google, Stanford, UC Berkeley, and OpenAI released the paper, “Concrete Problems in AI Safety,” yet it’s still one of the most important pieces on AI safety. Even after two years, it represents an excellent introduction to some of the problems researchers face as they develop artificial intelligence. In the paper, the authors explore the problem of accidents — unintended and harmful behavior — in AI systems, and they discuss different strategies and on-going research efforts to protect against these potential issues. Specifically, the authors address — Avoiding Negative Side Effects, Reward Hacking, Scalable Oversight, Safe Exploration, and Robustness to Distributional Change — which are illustrated with the example of a robot trained to clean an office.
We revisit these five topics here, summarizing them from the paper, as a reminder that these problems are still major issues that AI researchers are working to address.
Avoiding Negative Side Effects
When designing the objective function for an AI system, the designer specifies the objective but not the exact steps for the system to follow. This allows the AI system to come up with novel and more effective strategies for achieving its objective.
But if the objective function is not well defined, the AI’s ability to develop its own strategies can lead to unintended, harmful side effects. Consider a robot whose objective function is to move boxes from one room to another. The objective seems simple, yet there are a myriad of ways in which this could go wrong. For instance, if a vase is in the robot’s path, the robot may knock it down in order to complete the goal. Since the objective function does not mention anything about the vase, the robot wouldn’t know to avoid it. People see this as common sense, but AI systems don’t share our understanding of the world. It is not sufficient to formulate the objective as “complete task X”; the designer also needs to specify the safety criteria under which the task is to be completed.
One simple solution would be to penalize the robot every time it has an impact on the “environment” — such as knocking the vase over or scratching the wood floor. However, this strategy could effectively neutralize the robot, rendering it useless, as all actions require some level of interaction with the environment (and hence impact the environment). A better strategy could be to define a “budget” for how much the AI system is allowed to impact the environment. This would help to minimize the unintended impact, without neutralizing the AI system. Furthermore, this strategy of budgeting the impact of the agent is very general and can be reused across multiple tasks, from cleaning to driving to financial transactions to anything else an AI system might do. One serious limitation of this approach is that it is hard to quantify the “impact” on the environment even for a fixed domain and task.
Another approach would be train the agent to recognize harmful side effects so that it can avoid actions leading to such side effects. In that case, the agent would be trained for two tasks: the original task that is specified by the objective function and the task of recognizing side effects. The key idea here is that two tasks may have very similar side effects even when the main objective is different or even when they operate in different environments. For example, both a house cleaning robot and a house painting robot should not knock down vases while working. Similarly, the cleaning robot should not damage the floor irrespective of whether it operates in a factory or in a house. The main advantage of this approach is that once an agent learns to avoid side effects on one task, it can carry this knowledge when it is trained on another task. It would still be challenging to train the agent to recognize the side effects in the first place.
While it is useful to design approaches to limit side effects, these strategies in themselves are not sufficient. The AI system would still need to undergo extensive testing and critical evaluation before deployment in real life settings.
Reward Hacking
Sometimes the AI can come up with some kind of “hack” or loophole in the design of the system to receive unearned rewards. Since the AI is trained to maximize its rewards, looking for such loopholes and “shortcuts” is a perfectly fair and valid strategy for the AI. For example, suppose that the office cleaning robot earns rewards only if it does not see any garbage in the office. Instead of cleaning the place, the robot could simply shut off its visual sensors, and thus achieve its goal of not seeing garbage. But this is clearly a false success. Such attempts to “game” the system are more likely to manifest in complex systems with vaguely defined rewards. Complex systems provide the agent with multiple ways of interacting with the environment, thereby giving more freedom to the agent, and vaguely defined rewards make it harder to gauge true success on the task.
Just like the negative side effects problem, this problem is also a manifestation of objective misspecification. The formal objectives or end goals for the AI are not defined well enough to capture the informal “intent” behind creating the system — i.e., what the designers actually want the system to do. In some cases, this discrepancy leads to suboptimal results (when the cleaning robot shuts off its visual sensors); in other cases, it leads to harmful results (when the cleaning robot knocks down vases).
One possible approach to mitigating this problem would be to have a “reward agent” whose only task is to mark if the rewards given to the learning agent are valid or not. The reward agent ensures that the learning agent (the cleaning robot in our examples) does not exploit the system, but rather, completes the desired objective. In the previous example, the “reward agent” could be trained by the human designer to check if the room has garbage or not (an easier task than cleaning the room). If the cleaning robot shuts off its visual sensors and claims a high reward, the “reward agent” would mark the reward as invalid. The designer can then look into the rewards marked as “invalid” and make necessary changes in the objective function to fix the loophole.
Scalable Oversight
When the agent is learning to perform a complex task, human oversight and feedback are more helpful than just rewards from the environment. Rewards are generally modeled such that they convey to what extent the task was completed, but they do not usually provide sufficient feedback about the safety implications of the agent’s actions. Even if the agent completes the task successfully, it may not be able to infer the side-effects of its actions from the rewards alone. In the ideal setting, a human would provide fine-grained supervision and feedback every time the agent performs an action. Though this would provide a much more informative view about the environment to the agent, such a strategy would require far too much time and effort from the human.
One promising research direction to tackle this problem is semi-supervised learning, where the agent is still evaluated on all the actions (or tasks), but receives rewards only for a small sample of those actions (or tasks). For instance, the cleaning robot would take different actions to clean the room. If the robot performs a harmful action — such as damaging the floor — it gets a negative reward for that particular action. Once the task is completed, the robot is evaluated on the overall effect of all of its actions (and not evaluated individually for each action like picking up an item from floor) and is given a reward based on the overall performance.
Another promising research direction is hierarchical reinforcement learning, where a hierarchy is established between different learning agents. This idea could be applied to the cleaning robot in the following way. There would be a supervisor robot whose task is to assign some work (say, the task of cleaning one particular room) to the cleaning robot and provide it with feedback and rewards. The supervisor robot takes very few actions itself – assigning a room to the cleaning robot, checking if the room is clean and giving feedback – and doesn’t need a lot of reward data to be effectively trained. The cleaning robot does the more complex task of cleaning the room, and gets frequent feedback from the supervisor robot. The same supervisor robot could overlook the training of multiple cleaning agents as well. For example, a supervisor robot could delegate tasks to individual cleaning robots and provide reward/feedback to them directly. The supervisor robot can only take a small number of abstract actions itself and hence can learn from sparse rewards.
Safe Exploration
An important part of training an AI agent is to ensure that it explores and understands its environment. While exploring the environment may seem like a bad strategy in the short run, it could be a very effective strategy in the long run. Imagine that the cleaning robot has learned to identify garbage. It picks up one piece of garbage, walks out of the room, throws it into the garbage bin outside, comes back into the room, looks for another piece of garbage and repeats. While this strategy works, there could be another strategy that works even better. If the agent spent time exploring its environment, it might find that there’s a smaller garbage bin within the room. Instead of going back and forth with one piece at a time, the agent could first collect all the garbage into the smaller garbage bin and then make a single trip to throw the garbage into the garbage bin outside. Unless the agent is designed to explore its environment, it won’t discover these time-saving strategies.
Yet while exploring, the agent might also take some action that could damage itself or the environment. For example, say the cleaning robot sees some stains on the floor. Instead of cleaning the stains by scrubbing with a mop, the agent decides to try some new strategy. It tries to scrape the stains with a wire brush and damages the floor in the process. It’s difficult to list all possible failure modes and hard-code the agent to protect itself against them. But one approach to reduce harm is to optimize the performance of the learning agent in the worst case scenario. When designing the objective function, the designer should not assume that the agent will always operate under optimal conditions. Some explicit reward signal may be added to ensure that the agent does not perform some catastrophic action, even if that leads to more limited actions in the optimal conditions.
Another solution might be to reduce the agent’s exploration to a simulated environment or limit the extent to which the agent can explore. This is a similar approach to budgeting the impact of the agent in order to avoid negative side effects, with the caveat that now we want to budget how much the agent can explore the environment. Alternatively, an AI’s designers could avoid the need for exploration by providing demonstrations of what optimal behavior would look like under different scenarios.
Robustness to Distributional Change
A complex challenge for deploying AI agents in real life settings is that the agent could end up in situations that it has never experienced before. Such situations are inherently more difficult to handle and could lead the agent to take harmful actions. Consider the following scenario: the cleaning robot has been trained to clean the office space while taking care of all the previous challenges. But today, an employee brings a small plant to keep in the office. Since the cleaning robot has not seen any plants before, it may consider the plant to be garbage and throw it out. Because the AI does not recognize that this is a previously-unseen situation, it continues to act as though nothing has changed. One promising research direction focuses on identifying when the agent has encountered a new scenario so that it recognizes that it is more likely to make mistakes. While this does not solve the underlying problem of preparing AI systems for unforeseen circumstances, it helps in detecting the problem before mistakes happen. Another direction of research emphasizes transferring knowledge from familiar scenarios to new scenarios safely.
Conclusion
In a nutshell, the general trend is towards increasing autonomy in AI systems, and with increased autonomy comes increased chances of error. Problems related to AI safety are more likely to manifest in scenarios where the AI system exerts direct control over its physical and/or digital environment without a human in the loop – automated industrial processes, automated financial trading algorithms, AI-powered social media campaigns for political parties, self-driving cars, cleaning robots, among others. The challenges may be immense, but the silver lining is that papers like Concrete Problems in AI Safety have helped the AI community become aware of these challenges and agree on core issues. From there, researchers can start exploring strategies to ensure that our increasingly-advanced systems remain safe and beneficial.
About the Future of Life Institute
The Future of Life Institute (FLI) is a global non-profit with a team of 20+ full-time staff operating across the US and Europe. FLI has been working to steer the development of transformative technologies towards benefitting life and away from extreme large-scale risks since its founding in 2014. Find out more about our mission or explore our work.
Related content
Other posts about AI, AI Research, Recent News
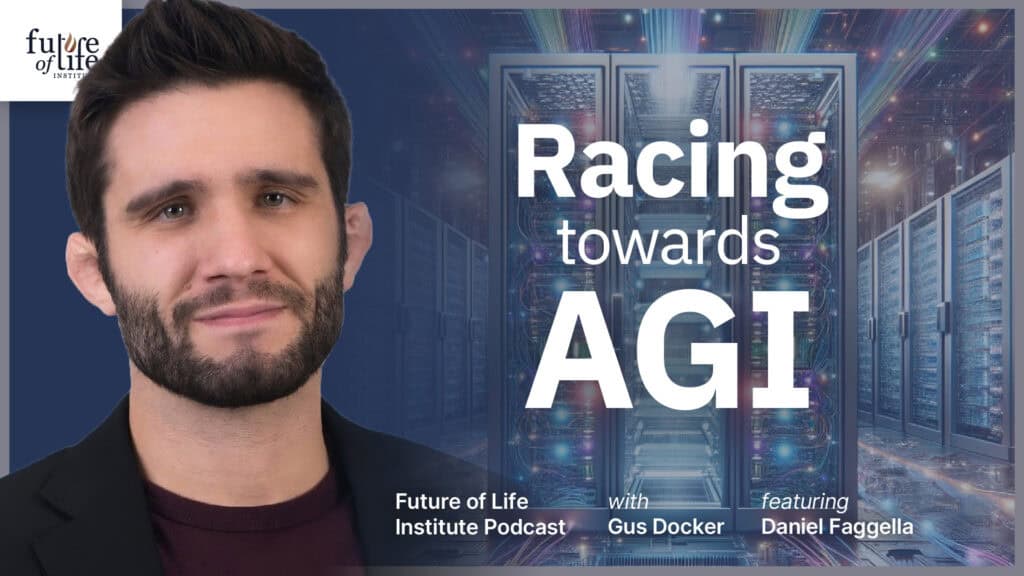
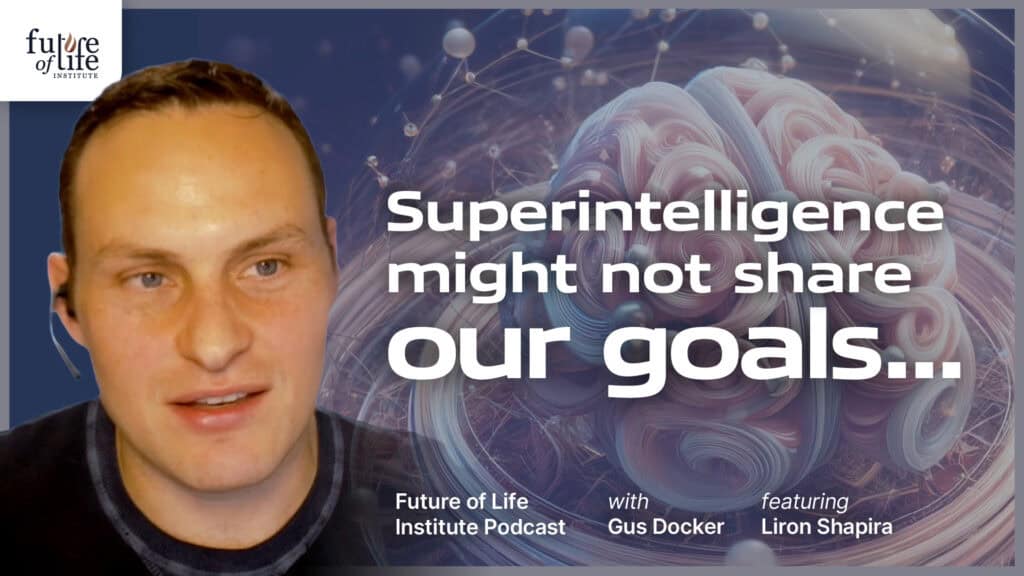
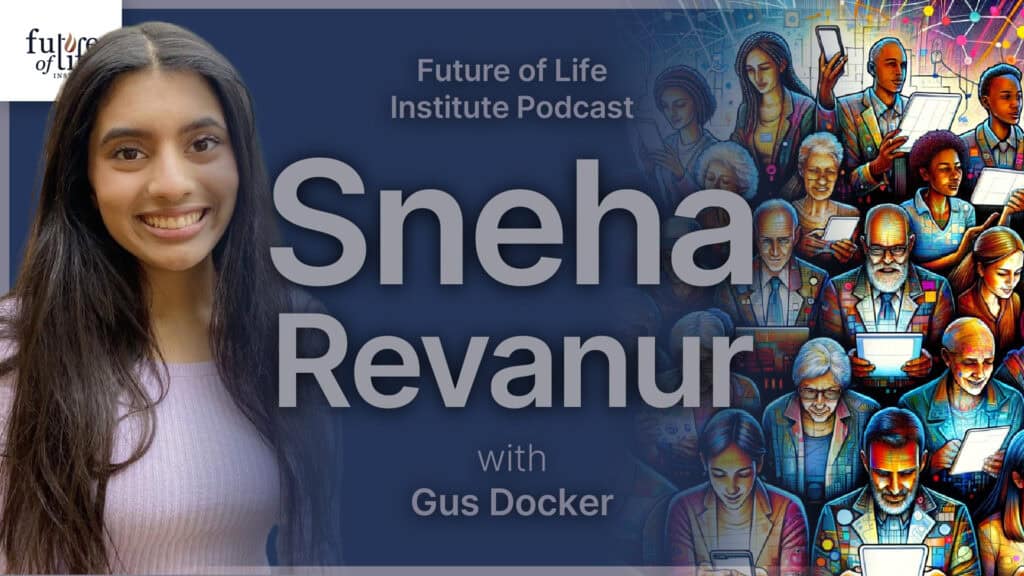