New AI Safety Research Agenda From Google Brain
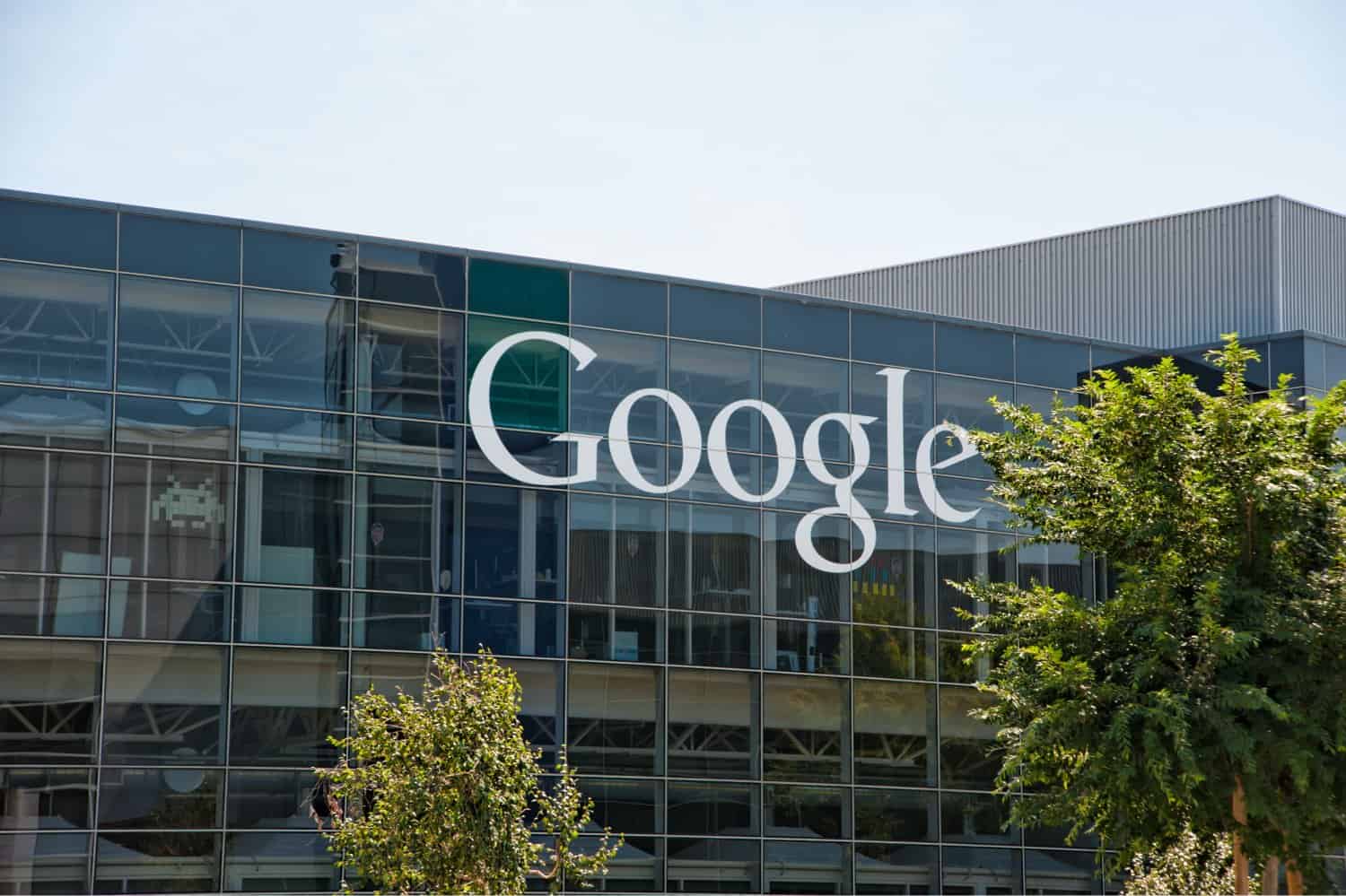
Contents
Google Brain just released an inspiring research agenda, Concrete Problems in AI Safety, co-authored by researchers from OpenAI, Berkeley and Stanford. This document is a milestone in setting concrete research objectives for keeping reinforcement learning agents and other AI systems robust and beneficial. The problems studied are relevant both to near-term and long-term AI safety, from cleaning robots to higher-stakes applications. The paper takes an empirical focus on avoiding accidents as modern machine learning systems become more and more autonomous and powerful.
Reinforcement learning is currently the most promising framework for building artificial agents – it is thus especially important to develop safety guidelines for this subfield of AI. The research agenda describes a comprehensive (though likely non-exhaustive) set of safety problems, corresponding to where things can go wrong when building AI systems:
-
Mis-specification of the objective function by the human designer. Two common pitfalls when designing objective functions are negative side-effects and reward hacking (also known as wireheading), which are likely to happen by default unless we figure out how to guard against them. One of the key challenges is specifying what it means for an agent to have a low impact on the environment while achieving its objectives effectively.
-
Extrapolation from limited information about the objective function. Even with a correct objective function, human supervision is likely to be costly, which calls for scalable oversight of the artificial agent.
-
Extrapolation from limited training data or using an inadequate model. We need to develop safe exploration strategies that avoid irreversibly bad outcomes, and build models that are robust to distributional shift – able to fail gracefully in situations that are far outside the training data distribution.
The AI research community is increasingly focusing on AI safety in recent years, and Google Brain’s agenda is part of this trend. It follows on the heels of the Safely Interruptible Agents paper from Google DeepMind and the Future of Humanity Institute, which investigates how to avoid unintended consequences from interrupting or shutting down reinforcement learning agents. We at FLI are super excited that industry research labs at Google and OpenAI are spearheading and fostering collaboration on AI safety research, and look forward to the outcomes of this work.
About the Future of Life Institute
The Future of Life Institute (FLI) is a global think tank with a team of 20+ full-time staff operating across the US and Europe. FLI has been working to steer the development of transformative technologies towards benefitting life and away from extreme large-scale risks since its founding in 2014. Find out more about our mission or explore our work.
Related content
Other posts about AI, Recent News
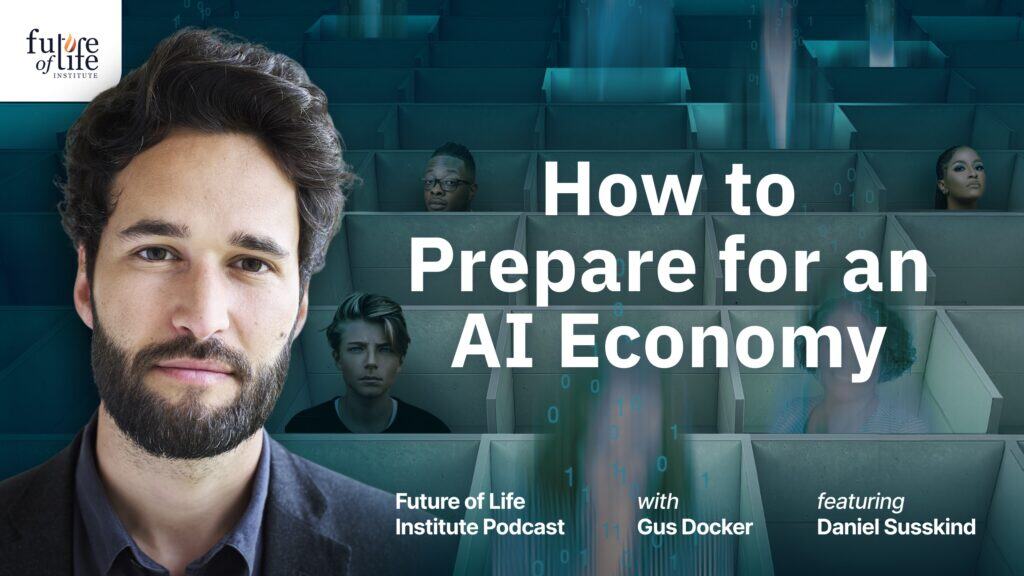