Risks From General Artificial Intelligence Without an Intelligence Explosion
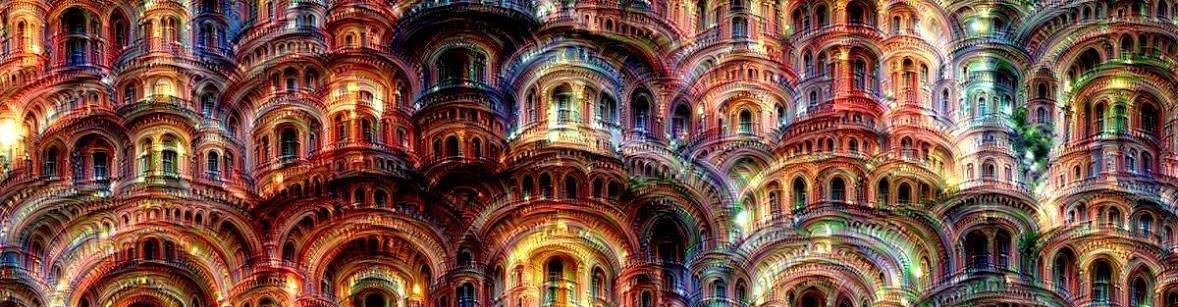
Contents
“An ultraintelligent machine could design even better machines; there would then unquestionably be an ‘intelligence explosion,’ and the intelligence of man would be left far behind.”
– Computer scientist I. J. Good, 1965
Artificial intelligence systems we have today can be referred to as narrow AI – they perform well at specific tasks, like playing chess or Jeopardy, and some classes of problems like Atari games. Many experts predict that general AI, which would be able to perform most tasks humans can, will be developed later this century, with median estimates around 2050. When people talk about long term existential risk from the development of general AI, they commonly refer to the intelligence explosion (IE) scenario. AI risk skeptics often argue against AI safety concerns along the lines of “Intelligence explosion sounds like science-fiction and seems really unlikely, therefore there’s not much to worry about”. It’s unfortunate when AI safety concerns are rounded down to worries about IE. Unlike I. J. Good, I do not consider this scenario inevitable (though relatively likely), and I would expect general AI to present an existential risk even if I knew for sure that intelligence explosion were impossible.
Here are some dangerous aspects of developing general AI, besides the IE scenario:
- Human incentives. Researchers, companies and governments have professional and economic incentives to build AI that is as powerful as possible, as quickly as possible. There is no particular reason to think that humans are the pinnacle of intelligence – if we create a system without our biological constraints, with more computing power, memory, and speed, it could become more intelligent than us in important ways. The incentives are to continue improving AI systems until they hit physical limits on intelligence, and those limitations (if they exist at all) are likely to be above human intelligence in many respects.
- Convergent instrumental goals. Sufficiently advanced AI systems would by default develop drives like self-preservation, resource acquisition, and preservation of their objective functions, independent of their objective function or design. This was outlined in Omohundro’s paper and more concretely formalized in a recent MIRI paper. Humans routinely destroy animal habitats to acquire natural resources, and an AI system with any goal could always use more data centers or computing clusters.
- Unintended consequences. As in the stories of Sorcerer’s Apprentice and King Midas, you get what you asked for, but not what you wanted. This already happens with narrow AI, like in the frequently cited example from the Bird & Layzell paper: a genetic algorithm was supposed to design an oscillator using a configurable circuit board, and instead designed a makeshift radio that used signal from neighboring computers to produce the requisite oscillating pattern. Unintended consequences produced by a general AI, more opaque and more powerful than a narrow AI, would likely be far worse.
- Value learning is hard. Specifying common sense and ethics in computer code is no easy feat. As argued by Stuart Russell, given a misspecified value function that omits variables that turn out to be important to humans, an optimization process is likely to set these unconstrained variables to extreme values. Think of what would happen if you asked a self-driving car to get you to the airport as fast as possible, without assigning value to obeying speed limits or avoiding pedestrians. While researchers would have incentives to build in some level of common sense and understanding of human concepts that is needed for commercial applications like household robots, that might not be enough for general AI.
- Value learning is insufficient. Even an AI system with perfect understanding of human values and goals would not necessarily adopt them. Humans understand the “goals” of the evolutionary process that generated us, but don’t internalize them – in fact, we often “wirehead” our evolutionary reward signals, e.g. by eating sugar.
- Containment is hard. A general AI system with access to the internet would be able to hack thousands of computers and copy itself onto them, thus becoming difficult or impossible to shut down – this is a serious problem even with present-day computer viruses. When developing an AI system in the vicinity of general intelligence, it would be important to keep it cut off from the internet. Large scale AI systems are likely to be run on a computing cluster or on the cloud, rather than on a single machine, which makes isolation from the internet more difficult. Containment measures would likely pose sufficient inconvenience that many researchers would be tempted to skip them.
Some believe that if intelligence explosion does not occur, AI progress will occur slowly enough that humans can stay in control. Given that human institutions like academia or governments are fairly slow to respond to change, they may not be able to keep up with an AI that attains human-level or superhuman intelligence over months or even years. Humans are not famous for their ability to solve coordination problems. Even if we retain control over AI’s rate of improvement, it would be easy for bad actors or zealous researchers to let it go too far – as Geoff Hinton recently put it, “the prospect of discovery is too sweet”.
As a machine learning researcher, I care about whether my field will have a positive impact on humanity in the long term. The challenges of AI safety are numerous and complex (for a more technical and thorough exposition, see Jacob Steinhardt’s essay), and cannot be rounded off to a single scenario. I look forward to a time when disagreements about AI safety no longer derail into debates about IE, and instead focus on other relevant issues we need to figure out.
(Thanks to Janos Kramar for his help with editing this post.)
About the Future of Life Institute
The Future of Life Institute (FLI) is a global think tank with a team of 20+ full-time staff operating across the US and Europe. FLI has been working to steer the development of transformative technologies towards benefitting life and away from extreme large-scale risks since its founding in 2014. Find out more about our mission or explore our work.
Related content
Other posts about AI
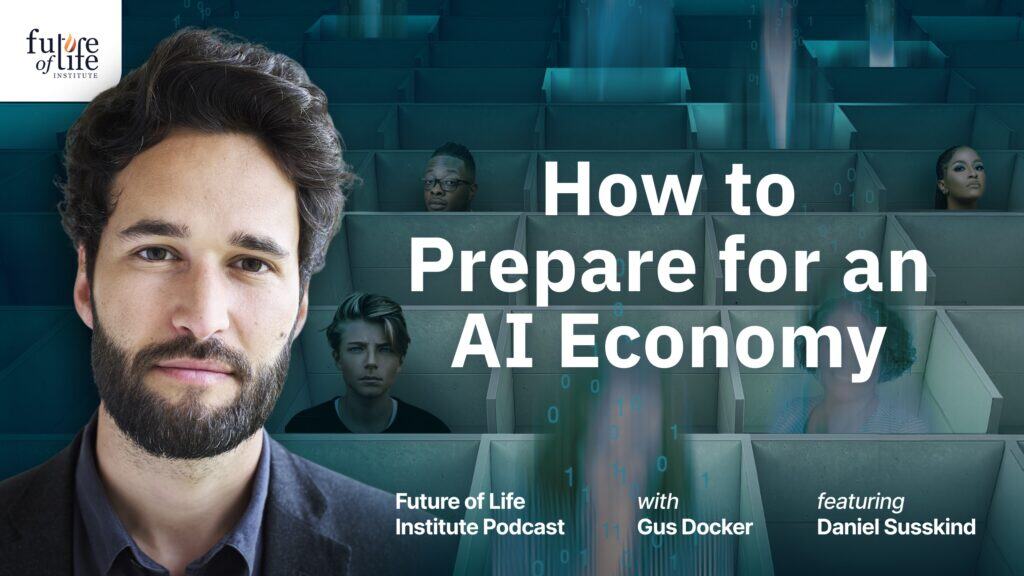