Portfolio Approach to AI Safety Research
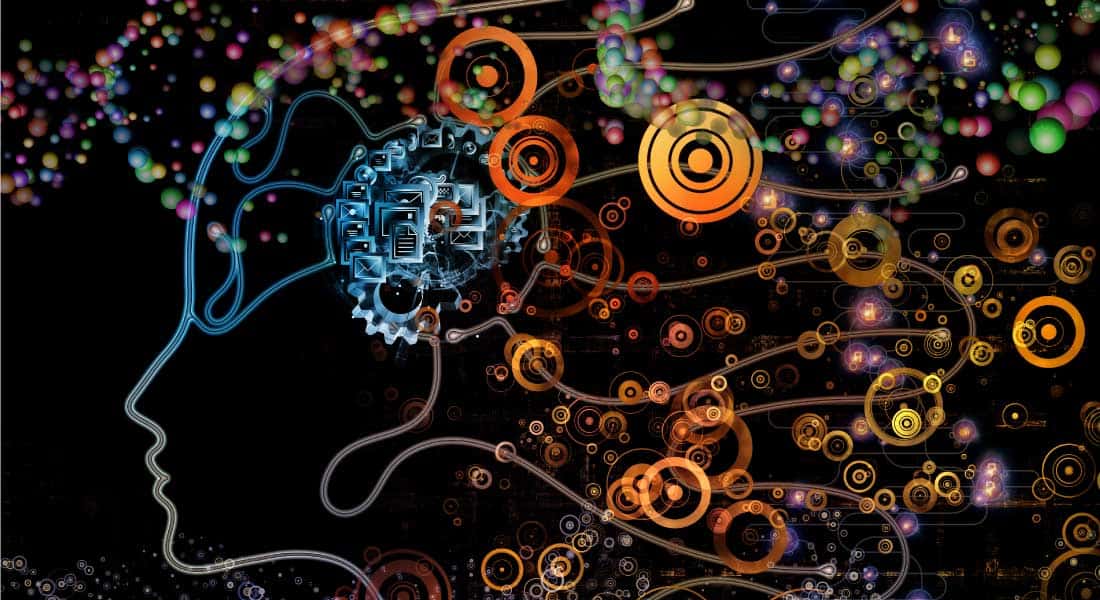
Contents
Long-term AI safety is an inherently speculative research area, aiming to ensure safety of advanced future systems despite uncertainty about their design or algorithms or objectives. It thus seems particularly important to have different research teams tackle the problems from different perspectives and under different assumptions. While some fraction of the research might not end up being useful, a portfolio approach makes it more likely that at least some of us will be right.
In this post, I look at some dimensions along which assumptions differ, and identify some underexplored reasonable assumptions that might be relevant for prioritizing safety research. (In the interest of making this breakdown as comprehensive and useful as possible, please let me know if I got something wrong or missed anything important.)
Assumptions about similarity between current and future AI systems
If a future general AI system has a similar algorithm to a present-day system, then there are likely to be some safety problems in common (though more severe in generally capable systems). Insights and solutions for those problems are likely to transfer to some degree from current systems to future ones. For example, if a general AI system is based on reinforcement learning, we can expect it to game its reward function in even more clever and unexpected ways than present-day reinforcement learning agents do. Those who hold the similarity assumption often expect most of the remaining breakthroughs on the path to general AI to be compositional rather than completely novel, enhancing and combining existing components in novel and better-implemented ways (many current machine learning advances such as AlphaGo are an example of this).
Note that assuming similarity between current and future systems is not exactly the same as assuming that studying current systems is relevant to ensuring the safety of future systems, since we might still learn generalizable things by testing safety properties of current systems even if they are different from future systems.
Assuming similarity suggests a focus on empirical research based on testing the safety properties of current systems, while not making this assumption encourages more focus on theoretical research based on deriving safety properties from first principles, or on figuring out what kinds of alternative designs would lead to safe systems. For example, safety researchers in industry tend to assume more similarity between current and future systems than researchers at MIRI.
Here is my tentative impression of where different safety research groups are on this axis. This is a very approximate summary, since views often vary quite a bit within the same research group (e.g. FHI is particularly diverse in this regard).
On the high-similarity side of the axis, we can explore the safety properties of different architectural / algorithmic approaches to AI, e.g. on-policy vs off-policy or model-free vs model-based reinforcement learning algorithms. It might be good to have someone working on safety issues for less commonly used agent algorithms, e.g. evolution strategies.
Assumptions about promising approaches to safety problems
Level of abstraction. What level of abstraction is most appropriate for tackling a particular problem. For example, approaches to the value learning problem range from explicitly specifying ethical constraints to capability amplification and indirect normativity, with cooperative inverse reinforcement learning somewhere in between. These assumptions could be combined by applying different levels of abstraction to different parts of the problem. For example, it might make sense to explicitly specify some human preferences that seem obvious and stable over time (e.g. “breathable air”), and use the more abstract approaches to impart the most controversial, unstable and vague concepts (e.g. “fairness” or “harm”). Overlap between the more and less abstract specifications can create helpful redundancy (e.g. air pollution as a form of harm + a direct specification of breathable air).
For many other safety problems, the abstraction axis is not as widely explored as for value learning. For example, most of the approaches to avoiding negative side effects proposed in Concrete Problems (e.g. impact regularizers and empowerment) are on a medium level of abstraction, while it also seems important to address the problem on a more abstract level by formalizing what we mean by side effects (which would help figure out what we should actually be regularizing, etc). On the other hand, almost all current approaches to wireheading / reward hacking are quite abstract, and the problem would benefit from more empirical work.
Explicit specification vs learning from data. Whether a safety problem is better addressed by directly defining a concept (e.g. the Low Impact AI paper formalizes the impact of an AI system by breaking down the world into ~20 billion variables) or learning the concept from human feedback (e.g. Deep Reinforcement Learning from Human Preferences paper teaches complex objectives to AI systems that are difficult to specify directly, like doing a backflip). I think it’s important to address safety problems from both of these angles, since the direct approach is unlikely to work on its own, but can give some idea of the idealized form of the objective that we are trying to approximate by learning from data.
Modularity of AI design. What level of modularity makes it easier to ensure safety? Ranges from end-to-end systems to ones composed of many separately trained parts that are responsible for specific abilities and tasks. Safety approaches for the modular case can limit the capabilities of individual parts of the system, and use some parts to enforce checks and balances on other parts. MIRI’s foundations approach focuses on a unified agent, while the safety properties on the high-modularity side has mostly been explored by Eric Drexler (more recent work is not public but available upon request). It would be good to see more people work on the high-modularity assumption.
Takeaways
To summarize, here are some relatively neglected assumptions:
- Medium similarity in algorithms / architectures
- Less popular agent algorithms
- Modular general AI systems
- More / less abstract approaches to different safety problems (more for side effects, less for wireheading, etc)
- More direct / data-based approaches to different safety problems
From a portfolio approach perspective, a particular research avenue is worthwhile if it helps to cover the space of possible reasonable assumptions. For example, while MIRI’s research is somewhat controversial, it relies on a unique combination of assumptions that other groups are not exploring, and is thus quite useful in terms of covering the space of possible assumptions.
I think the FLI grant program contributed to diversifying the safety research portfolio by encouraging researchers with different backgrounds to enter the field. It would be good for grantmakers in AI safety to continue to optimize for this in the future (e.g. one interesting idea is using a lottery after filtering for quality of proposals).
When working on AI safety, we need to hedge our bets and look out for unknown unknowns – it’s too important to put all the eggs in one basket.
(Cross-posted from Deep Safety. Thanks to Janos Kramar, Jan Leike and Shahar Avin for their feedback on this post. Thanks to Jaan Tallinn and others for inspiring discussions.)
About the Future of Life Institute
The Future of Life Institute (FLI) is a global think tank with a team of 20+ full-time staff operating across the US and Europe. FLI has been working to steer the development of transformative technologies towards benefitting life and away from extreme large-scale risks since its founding in 2014. Find out more about our mission or explore our work.
Related content
Other posts about AI, Recent News
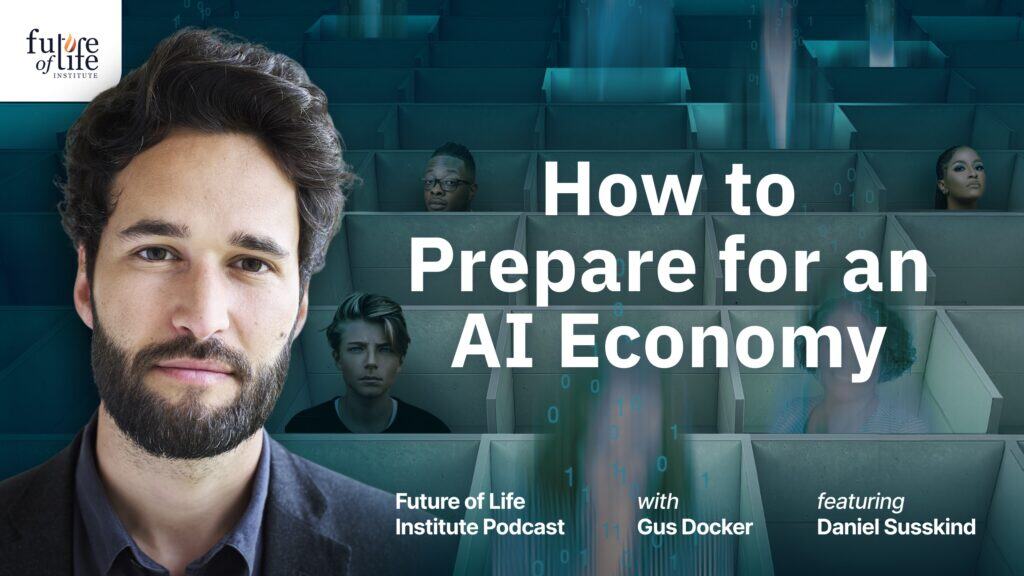