Optimizing AI Safety Research: An Interview With Owen Cotton-Barratt
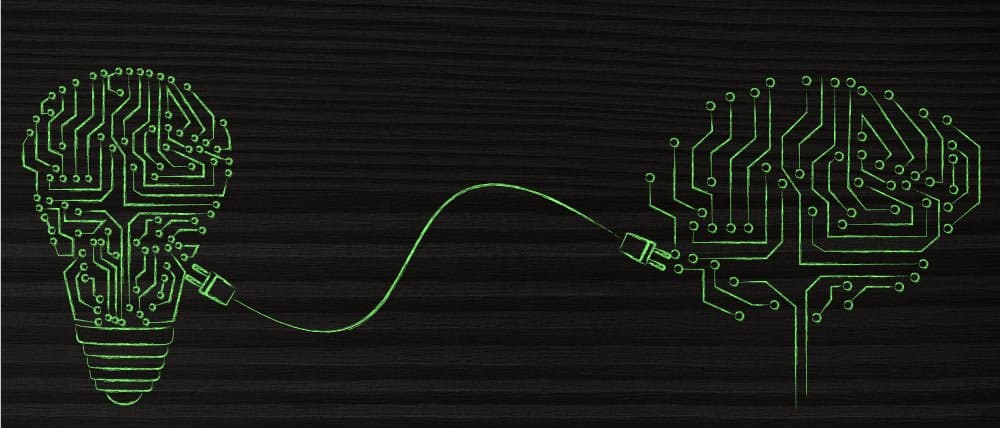
Contents
Artificial intelligence poses a myriad of risks to humanity. From privacy concerns, to algorithmic bias and “black box” decision making, to broader questions of value alignment, recursive self-improvement, and existential risk from superintelligence — there’s no shortage of AI safety issues.
AI safety research aims to address all of these concerns. But with limited funding and too few researchers, trade-offs in research are inevitable. In order to ensure that the AI safety community tackles the most important questions, researchers must prioritize their causes.
Owen Cotton-Barratt, along with his colleagues at the Future of Humanity Institute (FHI) and the Centre for Effective Altruism (CEA), looks at this ‘cause prioritization’ for the AI safety community. They analyze which projects are more likely to help mitigate catastrophic or existential risks from highly-advanced AI systems, especially artificial general intelligence (AGI). By modeling trade-offs between different types of research, Cotton-Barratt hopes to guide scientists toward more effective AI safety research projects.
Technical and Strategic Work
The first step of cause prioritization is understanding the work already being done. Broadly speaking, AI safety research happens in two domains: technical work and strategic work.
AI’s technical safety challenge is to keep machines safe and secure as they become more capable and creative. By making AI systems more predictable, more transparent, and more robustly aligned with our goals and values, we can significantly reduce the risk of harm. Technical safety work includes Stuart Russell’s research on reinforcement learning and Dan Weld’s work on explainable machine learning, since they’re improving the actual programming in AI systems.
In addition, the Machine Intelligence Research Institute (MIRI) recently released a technical safety agenda aimed at aligning machine intelligence with human interests in the long term, while OpenAI, another non-profit AI research company, is investigating the “many research problems around ensuring that modern machine learning systems operate as intended,” following suggestions from the seminal paper Concrete Problems in AI Safety.
Strategic safety work is broader, and asks how society can best prepare for and mitigate the risks of powerful AI. This research includes analyzing the political environment surrounding AI development, facilitating open dialogue between research areas, disincentivizing arms races, and learning from game theory and neuroscience about probable outcomes for AI. Yale professor Allan Dafoe has recently focused on strategic work, researching the international politics of artificial intelligence and consulting for governments, AI labs and nonprofits about AI risks. And Yale bioethicist Wendell Wallach, apart from his work on “silo busting,” is researching forms of global governance for AI.
Cause prioritization is strategy work, as well. Cotton-Barratt explains, “Strategy work includes analyzing the safety landscape itself and considering what kind of work do we think we’re going to have lots of, what are we going to have less of, and therefore helping us steer resources and be more targeted in our work.”
Who Needs More Funding?
As the graph above illustrates, AI safety spending has grown significantly since 2015. And while more money doesn’t always translate into improved results, funding patterns are easy to assess and can say a lot about research priorities. Seb Farquhar, Cotton-Barratt’s colleague at CEA, wrote a post earlier this year analyzing AI safety funding and suggesting ways to better allocate future investments.
To start, he suggests that the technical research community acquire more personal investigators to take the research agenda, detailed in Concrete Problems in AI Safety, forward. OpenAI is already taking a lead on this. Additionally, the community should go out of its way to ensure that emerging AI safety centers hire the best candidates, since these researchers will shape each center’s success for years to come.
In general, Farquhar notes that strategy, outreach and policy work haven’t kept up with the overall growth of AI safety research. He suggests that more people focus on improving communication about long-run strategies between AI safety research teams, between the AI safety community and the broader AI community, and between policymakers and researchers. Building more PhD and Masters courses on AI strategy and policy could establish a pipeline to fill this void, he adds.
To complement Farquhar’s data, Cotton-Barratt’s colleague Max Dalton created a mathematical model to track how more funding and more people working on a safety problem translate into useful progress or solutions. The model tries to answer such questions as: if we want to reduce AI’s existential risks, how much of an effect do we get by investing money in strategy research versus technical research?
In general, technical research is easier to track than strategic work in mathematical models. For example, spending more on strategic ethics research may be vital for AI safety, but it’s difficult to quantify that impact. Improving models of reinforcement learning, however, can produce safer and more robustly-aligned machines. With clearer feedback loops, these technical projects fit best with Dalton’s models.
Near-sightedness and AGI
But these models also confront major uncertainty. No one really knows when AGI will be developed, and this makes it difficult to determine the most important research. If AGI will be developed in five years, perhaps researchers should focus only on the most essential safety work, such as improving transparency in AI systems. But if we have thirty years, researchers can probably afford to dive into more theoretical work.
Moreover, no one really knows how AGI will function. Machine learning and deep neural networks have ushered in a new AI revolution, but AGI will likely be developed on architectures far different from AlphaGo and Watson.
This makes some long-term safety research a risky investment, even if, as many argue, it is the most important research we can do. For example, researchers could spend years making deep neural nets safe and transparent, only to find their work wasted when AGI develops on an entirely different programming architecture.
Cotton-Barratt attributes this issue to ‘nearsightedness,’ and discussed it in a recent talk at Effective Altruism Global this summer. Humans often can’t anticipate disruptive change, and AI researchers are no exception.
“Work that we might do for long-term scenarios might turn out to be completely confused because we weren’t thinking of the right type of things,” he explains. “We have more leverage over the near-term scenarios because we’re more able to assess what they’re going to look like.”
Any additional AI safety research is better than none, but given the unknown timelines and the potential gravity of AI’s threats to humanity, we’re better off pursuing — to the extent possible — the most effective AI safety research.
By helping the AI research portfolio advance in a more efficient and comprehensive direction, Cotton-Barratt and his colleagues hope to ensure that when machines eventually outsmart us, we will have asked — and hopefully answered — the right questions.
This article is part of a Future of Life series on the AI safety research grants, which were funded by generous donations from Elon Musk and the Open Philanthropy Project. If you’re interested in applying for our 2018 grants competition, please see this link.
About the Future of Life Institute
The Future of Life Institute (FLI) is a global non-profit with a team of 20+ full-time staff operating across the US and Europe. FLI has been working to steer the development of transformative technologies towards benefitting life and away from extreme large-scale risks since its founding in 2014. Find out more about our mission or explore our work.
Related content
Other posts about AI, AI Research, Grants Program, Recent News
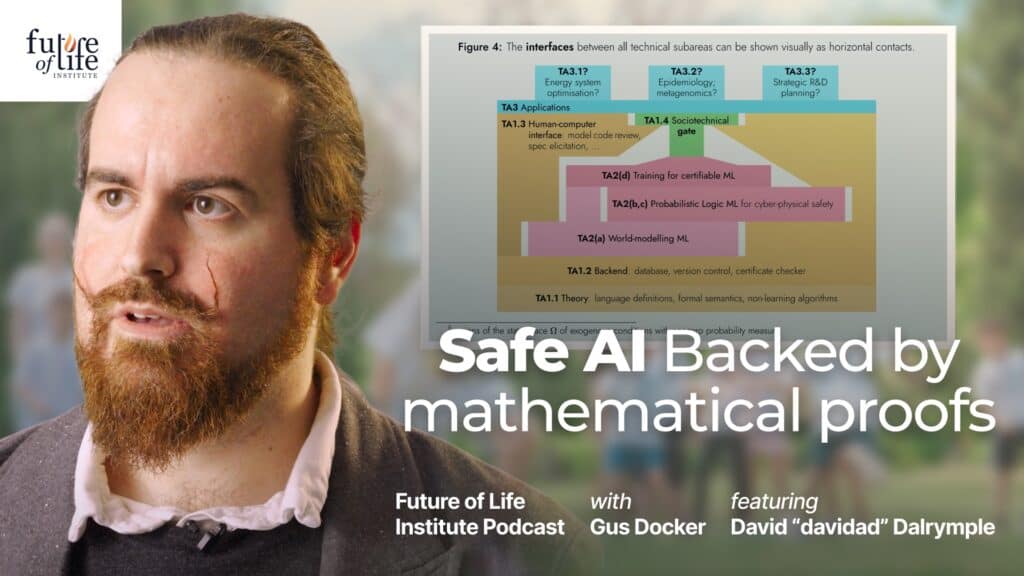
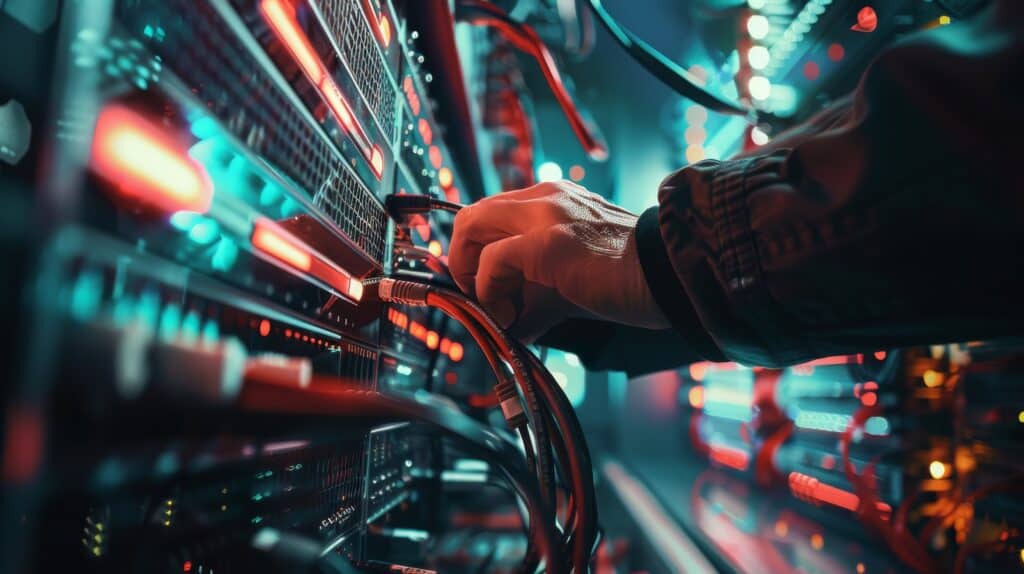