Op-ed: On AI, Prescription Drugs, and Managing the Risks of Things We Don’t Understand
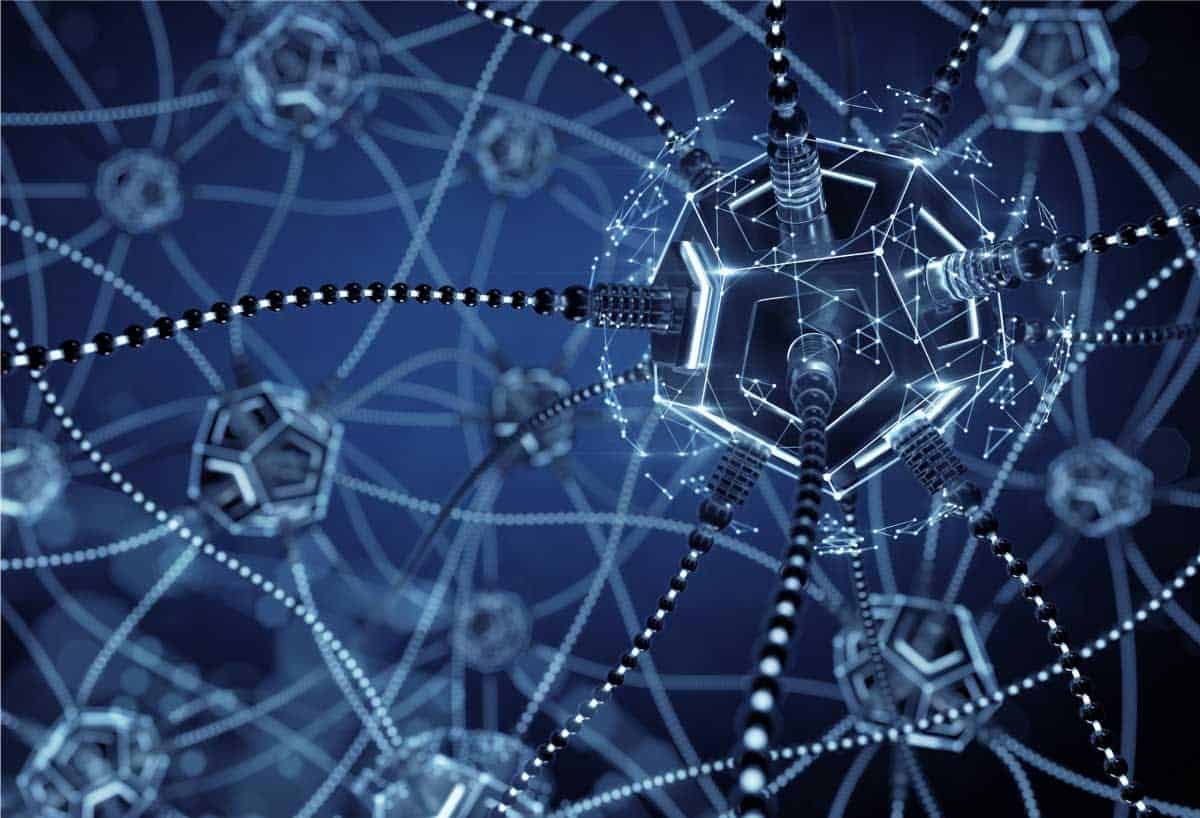
Contents
Click here to see this page in other languages : Chinese
Last month, Technology Review published a good article discussing the “dark secret at the heart of AI”–namely, that “o one really knows how the most advanced algorithms do what they do.” The opacity of algorithmic systems is something that has long drawn attention and criticism. But it is a concern that has broadened and deepened in the past few years, during which breakthroughs in “deep learning” have led to a rapid increase in the sophistication of AI. These deep learning systems operate using deep neural networks that are designed to roughly simulate the way the human brain works–or, to be more precise, to simulate the way the human brain works as we currently understand it.
Such systems can effectively “program themselves” by creating much or most of the code through which they operate. The code generated by such systems can be very complex. It can be so complex, in fact, that even the people who built and initially programmed the system may not be able to fully explain why the systems do what they do:
You can’t just look inside a deep neural network to see how it works. A network’s reasoning is embedded in the behavior of thousands of simulated neurons, arranged into dozens or even hundreds of intricately interconnected layers. The neurons in the first layer each receive an input, like the intensity of a pixel in an image, and then perform a calculation before outputting a new signal. These outputs are fed, in a complex web, to the neurons in the next layer, and so on, until an overall output is produced.
And because it is not presently possible for such a system to create a comprehensible “log” that shows the system’s reasoning, it is possible that no one can fully explain a deep learning system’s behavior.
This is, to be sure, a real concern. The opacity of modern AI systems is, as I wrote in my first article on this subject, one of the major barriers that makes it difficult to effectively manage the public risks associated with AI. In my paper, I was talking about how the opacity of such systems to regulators, industry bodies, and the general public was a problem. That problem becomes several orders of magnitude more serious if the programs are opaque even to the system’s creators.
We have already seen related concerns discussed in the world of automated trading systems, which are driven by algorithms whose workings are sufficiently mysterious as to earn them the moniker of “black-box trading” systems. The interactions between algorithmic trading systems are widely blamed for driving the Flash Crash of 2010. But, as far as I know, no one has suggested that the algorithms themselves involved in the Flash Crash were not understood by their designers. Rather, it was the interaction between those algorithmic systems that was impossible to model and predict. That makes those risks easier to manage than risks arising from technologies that are themselves poorly understood.
That being said, I don’t see even intractable ignorance as a truly insurmountable barrier or something that would make it wise to outright “ban” the development of opaque AI systems. Humans have actually been creating and using things that no one understands for centuries, if not millenia. For me, the most obvious examples probably come from the world of medicine, a science where safe and effective treatments have often been developed even when no one really understands why the treatments are safe and effective.
In the 1790s, Edward Jenner developed a vaccine to immunize people from smallpox, a disease caused by the variola virus. At the time, no one had any idea of that smallpox was caused by a virus. In fact, people at the time had not even discovered viruses. In the 1700s, the leading theory of communicable diseases was that they were transmitted by bad-smelling fumes. It would be over half a century before the germ theory of disease started to gain acceptance, and nearly a century before viruses were discovered.
Even today, there are many drugs that doctors prescribe whose mechanisms of action are either only partially understood or barely understood at all. Many psychiatric drugs fit this description, for instance. Pharmacologists have made hypotheses on how it is that tricyclic antidepressants seem to help both people with depression and people with neuropathic pain. But a hypothesis is still just, well, a hypothesis. And presumably, the designers of a deep learning system are also capable of making hypotheses regarding how the system reached its current state, even though those hypotheses may not be as well-informed as those made about tricyclic antidepressants.
As a society, we long ago decided that the mere fact that no one completely understands a drug does not mean that no one should use such drugs. But we have managed the risks associated with potential new drugs by requiring that drugs be thoroughly tested and proven safe before they hit pharmacy shelves. Indeed, aside from nuclear technology, pharmaceuticals may be the most heavily regulated technology in the world.
In the United States, many people (especially people in the pharmaceutical industry) criticize the long, laborious, and labyrinthine process that drugs must go through before they can be approved for use. But that process allows us to be pretty confident that a drug is safe (or at least “safe enough”) when it finally does hit the market. And that, in turn, means that we can live with having drugs whose workings are not well-understood, since we know that any hidden dangers would very likely have been exposed during the testing process.
What lessons might this hold for AI? Well, most obviously, it suggests a need to ensure that AI systems are rigorously tested before they can be marketed to the public, at least if the AI system cannot “show its work” in a way that permits its decision-making process to be comprehensible to, at a minimum, the people who designed it. I doubt that we’ll ever see a FDA-style uber-regulatory regime for AI systems. The FDA model would neither be practical nor effective for such a complex digital technology. But what we are likely to see is courts and legal systems rightly demanding that companies put deep learning systems through more extensive testing than has been typical for previous generations of digital technology.
Because such testing is likely to be lengthy and labor-intensive, the necessity of such testing would make it even more difficult for smaller companies to gain traction in the AI industry, further increasing the market power of giants like Google, Amazon, Facebook, Microsoft, and Apple. That is, not coincidentally, exactly what has happened in the brand-name drug industry, which has seen market power increasingly concentrated in the hands of a small number of companies. And as observers of that industry know, such concentration of market power carries risks of its own. That may be the price we have to pay if we want to reap the benefits of technologies we do not fully understand.
About the Future of Life Institute
The Future of Life Institute (FLI) is a global think tank with a team of 20+ full-time staff operating across the US and Europe. FLI has been working to steer the development of transformative technologies towards benefitting life and away from extreme large-scale risks since its founding in 2014. Find out more about our mission or explore our work.
Related content
Other posts about AI, Recent News
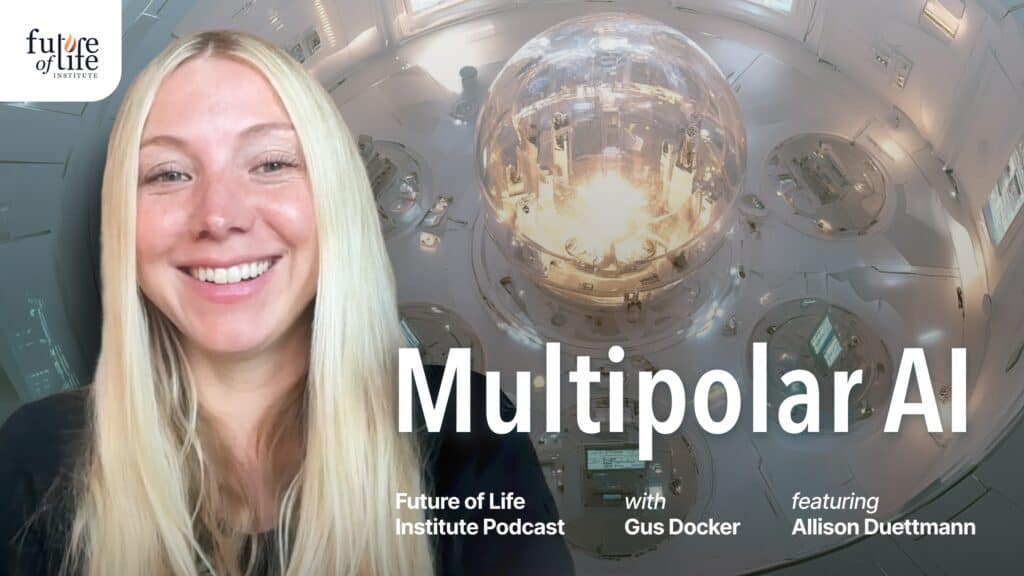
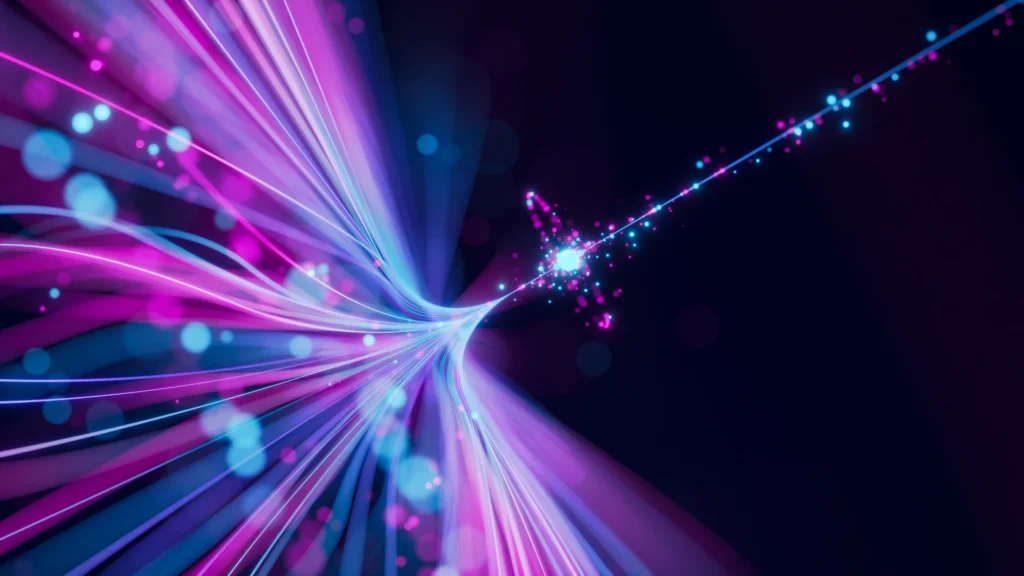