Podcast: Navigating AI Safety – From Malicious Use to Accidents
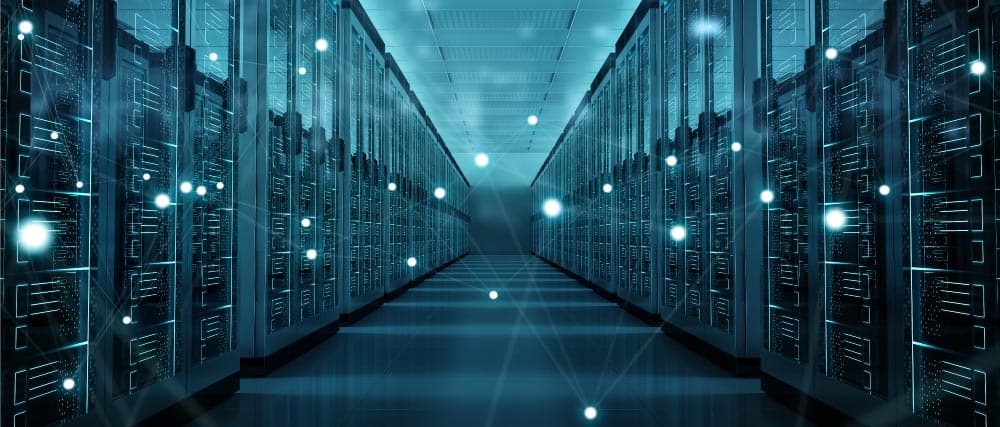
Is the malicious use of artificial intelligence inevitable? If the history of technological progress has taught us anything, it's that every "beneficial" technological breakthrough can be used to cause harm. How can we keep bad actors from using otherwise beneficial AI technology to hurt others? How can we ensure that AI technology is designed thoughtfully to prevent accidental harm or misuse?
On this month's podcast, Ariel spoke with FLI co-founder Victoria Krakovna and Shahar Avin from the Center for the Study of Existential Risk (CSER). They talk about CSER's recent report on forecasting, preventing, and mitigating the malicious uses of AI, along with the many efforts to ensure safe and beneficial AI.
Topics discussed in this episode include:
- the Facebook Cambridge Analytica scandal,
- Goodhart's Law with AI systems,
- spear phishing with machine learning algorithms,
- why it's so easy to fool ML systems,
- and why developing AI is still worth it in the end.
Transcript
Ariel: The challenge is daunting and the stakes are high. So ends the executive summary of the recent report, The Malicious Use of Artificial Intelligence: Forecasting, Prevention and Mitigation. I'm Ariel Conn with the Future of Life Institute, and I'm excited to have Shahar Avin and Victoria Krakovna joining me today to talk about this report along with the current state of AI safety research and where we've come in the last three years.
But first, if you've been enjoying our podcast, please make sure you've subscribed to this channel on SoundCloud, iTunes, or whatever your favorite podcast platform happens to be. In addition to the monthly podcast I've been recording, Lucas Perry will also be creating a new podcast series that will focus on AI safety and AI alignment, where he will be interviewing technical and non-technical experts from a wide variety of domains. His upcoming interview is with Dylan Hadfield-Menell, a technical AI researcher who works on cooperative inverse reinforcement learning and inferring human preferences. The best way to keep up with new content is by subscribing. And now, back to our interview with Shahar and Victoria.
Shahar is a Research Associate at the Center for the Study of Existential Risk, which I'll be referring to as CSER for the rest of this podcast, and he is also the lead co-author on the Malicious Use of Artificial Intelligence report. Victoria is a co-founder of the Future of Life Institute and she's a research scientist at DeepMind working on technical AI safety.
Victoria and Shahar, thank you so much for joining me today.
Shahar: Thank you for having us.
Victoria: Excited to be here.
Ariel: So I want to go back three years, to when FLI started our grant program, which helped fund this report on the malicious use of artificial intelligence, and I was hoping you could both talk for maybe just a minute or two about what the state of AI safety research was three years ago, and what prompted FLI to take on a lot of these grant research issues -- essentially what prompted a lot of the research that we're seeing today? Victoria, maybe it makes sense to start with you quickly on that.
Victoria: Well three years ago, AI safety was less mainstream in the AI research community than it is today, particularly long-term AI safety. So part of what FLI has been working on and why FLI started this grant program was to stimulate more work into AI safety and especially its longer-term aspects that have to do with powerful general intelligence, and to make it a more mainstream topic in the AI research field.
Three years ago, there were fewer people working in it, and many of the people who were working in it were a little bit disconnected from the rest of the AI research community. So part of what we were aiming for with our Puerto Rico conference and our grant program, was to connect these communities better, and to make sure that this kind of research actually happens and that the conversation shifts from just talking about AI risks in the abstract to actually doing technical work, and making sure that the technical problems get solved and that we start working on these problems well in advance before it is clear that, let's say general AI, would appear soon.
I think part of the idea with the grant program originally, was also to bring in new researchers into AI safety and long-term AI safety. So to get people in the AI community interested in working on these problems, and for those people whose research was already related to the area, to focus more on the safety aspects of their research.
Ariel: I'm going to want to come back to that idea and how far we've come in the last three years, but before we do that, Shahar, I want to ask you a bit about the report itself.
So this started as a workshop that Victoria had also actually participated in last year and then you've turned it into this report. I want you to talk about what prompted that and also this idea that's mentioned in the report is that, no one's really looking at how artificial intelligence could be used maliciously. And yet what we've seen with every technology and advance that's happened throughout history, I can't think of anything that people haven't at least attempted to use to cause harm, whether they've succeeded or not, I don't know if that's always the case, but almost everything gets used for harm in some way. So I'm curious why there haven't been more people considering this issue yet?
Shahar: So going to back to maybe a few months before the workshop, which as you said was February 2017. Both Miles Brundage at the Future of Humanity Institute and I at the Center for the Study of Existential Risk, had this inkling that there were more and more corners of malicious use of AI that were being researched, people were getting quite concerned. We were in discussions with the Electronic Frontier Foundation about the DARPA Cyber Grand Challenge and progress being made towards the use of artificial intelligence in offensive cybersecurity. I think Miles was very well connected to the circle who were looking at lethal autonomous weapon systems and the increasing use of autonomy in drones. And we were both kind of -- stories like the Facebook story that has been in the news recently, there were kind of the early versions of that coming up already back then.
So it's not that people were not looking at malicious uses of AI, but it seemed to us that there wasn't this overarching perspective that is not looking at particular domains. This is not, “what will AI do to cybersecurity in terms of malicious use? What will malicious use of AI look like in politics? What do malicious use of AI look like in warfare?” But rather across the board, if you look at this technology, what new kinds of malicious actions does it enable, and other commonalities across those different domains. Plus, it seemed that that “across the board” more technology-focused perspective, other than “domain of application” perspective, was something that was missing. And maybe that's less surprising, right? People get very tied down to a particular scenario, a particular domain that they have expertise on, and from the technologists’ side, many of them just wouldn't know all of the legal minutiae of warfare, or -- one thing that we found was there weren't enough channels of communication between the cybersecurity community and the AI research community; similarly the political scientists and the AI research community. So it did require quite an interdisciplinary workshop to get all of these things on the table, and tease out some the commonalities, which is what we then try to do with the report.
Ariel: So actually, you mentioned the Facebook thing and I was a little bit curious about that. Does that fall under the umbrella of this report or is that a separate issue?
Shahar: It's not clear if it would fall directly under the report, because the way we define malicious could be seen as problematic. It's the best that we could do with this kind of report, which is to say that there is a deliberate attempt to cause harm using the technology. It's not clear, whether in the Facebook case, there was a deliberate attempt to cause harm or whether there was disregard of harm that could be caused as a side effect, or just the use of this in an arena that there are legitimate moves, just some people realize that the technology can be used to gain an upper hand within this arena.
But, there are whole scenarios that sit just next to it, that look very similar, but that are centralized use of this kind of surveillance, diminishing privacy, potentially the use of AI to manipulate individuals, manipulate their behavior, target messaging at particular individuals.
There are clearly imaginable scenarios in which this is done maliciously to keep a corrupt government in power, to overturn a government in another nation, kind of overriding the self-determination of the members of their country. There are not going to be clear rules about what is obviously malicious and what is just part of the game. I don't know where to put Facebook's and Cambridge Analytica's case, but there are clearly cases that I think universally would be considered as malicious that from the technology side look very similar.
Ariel: So this gets into a quick definition that I would like you to give us and that is for the term 'dual use.’ I was at a conference somewhat recently and a government official who was there, not a high level, but someone who should have been familiar with the term 'dual use' was not. So I would like to make sure that we all know what that means.
Shahar: So I'm not, of course, a legal expert, but the term did come up a lot in the workshop and in the report. 'Dual use,’ as far as I can understand it, refers to technologies or materials that both have peace-time or peaceful purposes and uses, but also wartime, or harmful uses. A classical example would be certain kinds of fertilizer that could be used to grow more crops, but could also be used to make homegrown explosives. And this matters because you might want to regulate explosives, but you definitely don't want to limit people’s access to get fertilizer and so you're in a bind. How do you make sure that people who have a legitimate peaceful use of a particular technology or material get to have that access without too much hassle that will increase the cost or make things more burdensome, but at the same time, make sure that malicious actors don't get access to capabilities or technologies or materials that they can use to do harm.
I've also heard the term 'omni use,’ being referred to artificial intelligence, this is the idea that technology can have so many uses across the board that regulating it because of its potential for causing harm comes at a very, very high price, because it is so foundational for so many other things. So one can think of electricity: it is true that you can use electricity to harm people, but vetting every user of the electric grid before they are allowed to consume electricity, seems very extreme, because there is so much benefit to be gained from just having access to electricity as a utility, that you need to find other ways to regulate. Computing is often considered as 'omni use' and it may well be that artificial intelligence is such a technology that would just be foundational for so many applications that it will be 'omni use,’ and so the way to stop malicious actors from having access to it is going to be fairly complicated, but it's probably not going to be any kind of a heavy-handed regulation.
Ariel: Okay. Thank you. So going back a little bit to the report more specifically, I don't know how detailed we want to get with everything, but I was hoping you could touch a little bit on a few of the big topics that are in the report. For example, you talk about changes in the landscape of threats, where there is an expansion of existing threats, there's an intro to new threats, and typical threats will be modified. Can you speak somewhat briefly as to what each of those mean?
Shahar: So I guess what I was saying, the biggest change is that machine learning, at least in some domains, now works. That means that you don't need to have someone write out the code in order to have a computer that is performant at the particular task, if you can have the right kind of labeled data or the right kind of simulator in which you can train an algorithm to perform that action. That means that, for example, if there is a human expert with a lot of tacit knowledge in a particular domain, let's say the use of a sniper rifle, it may be possible to train a camera that sits on top of a rifle, coupled with a machine learning algorithm that does the targeting for you, so that now any soldier becomes as expert as an expert marksman. And of course, the moment you've trained this model once, making copies of it is essentially free or very close to free, the same as it is with software.
Another is the ability to go through very large spaces of options and using some heuristics to more effectively search through that space for effective solutions. So one example of that would be AlphaGo, which is a great technological achievement and has absolutely no malicious use aspects, but you can imagine as an analogy, similar kinds of technologies being used to find weaknesses in software, discovering vulnerabilities and so on. And I guess, finally, one example we've seen that came up a lot, is the capabilities in machine vision. The fact that you can now look at an image and tell what is in that image, through training, which is something that computers were just not able to do a decade ago, at least nowhere near human levels of performance, starts unlocking potential threats both in autonomous targeting, say on top of drones, but also in manipulation. If I can know whether a picture is a good representation of something or not, then my ability to create forgeries significantly increases. This is the technology of generative adversarial networks, that we've seen used to create fake audio and potentially fake videos in the near future.
All of these new capabilities, plus the fact that access to the technology is becoming -- I mean these technologies are very democratized at the moment. There are papers on arXiv, there are good tutorials on You Tube. People are very keen to have more people join the AI revolution, and for good reason, plus the fact that moving these trained models around is very cheap. It's just the cost of copying the software around, and the computer that is required to run those models is widely available. This suggests that the availability of these malicious capabilities is going to rapidly increase, and that the ability to perform certain kinds of attacks would no longer be limited to a few humans, but would become much more widespread.
Ariel: And so I have one more question for you, Shahar, and then I'm going to bring Victoria back in. You're talking about the new threats, and this expansion of threats and one of the things that I saw in the report that I've also seen in other issues related to AI is, we've had computers around for a couple decades now, we're used to issues pertaining to phishing or hacking or spam. We recognize computer vulnerabilities. We know these are an issue. We know that there's lots of companies that are trying to help us defend our computers against malicious cyber attacks, stuff like that. But one of the things that you get into in the report is this idea of “human vulnerabilities” -- that these attacks are no longer just against the computers, but they are also going to be against us.
Shahar: I think for many people, this has been one of the really worrying things about the Cambridge Analytica, Facebook issue that is in the news. It's the idea that because of our particular psychological tendencies, because of who we are, because of how we consume information, and how that information shapes what we like and what we don't like, what we are likely to do and what we are unlikely to do, the ability of the people who control the information that we get, gives them some capability to control us. And this is not new, right?
People who are making newspapers or running radio stations or national TV stations, have known for a very long time, that the ability to shape the message is the ability to influence people's decisions. But coupling that with algorithms that are able to run experiments on millions or billions of people simultaneously with very tight feedback loops -- so you make a small change in the feed of one individual and see whether their behavior changes. And you can run many of these experiments and you can get very good data, is something that was never available at the age of broadcasts. To some extent, it was available in the age of software. When software starts moving into big data and big data analytics, the boundaries start to blur between those kinds of technologies and AI technologies.
This is the kind of manipulation that you seem to be asking about that we definitely flag in the report, both in terms of political security, the ability of large communities to govern themselves in a way that they find to truthfully represent their own preferences, but also, on a more small scale, with the social side of cyber attacks. So, if I can manipulate an individual, or a few individuals in a company to disclose their passwords or to download or click a link that they shouldn't have, through modeling of their preferences and their desires, then that is a way in that might be a lot easier than trying to break the system through its computers.
Ariel: Okay, so one other thing that I think I saw come up, and I started to allude to this -- there's, like I said, the idea that we can defend our computers against attacks and we can upgrade our software to fix vulnerabilities, but then how do we sort of "upgrade" people to defend themselves? Is that possible? Or is it a case of we just keep trying to develop new software to help protect people?
Shahar: I think the answer is both. One thing that did come up a lot is, unfortunately unlike computers, you cannot just download a patch to everyone's psychology. We have slow processes of doing that. So we can incorporate parts of what is a trusted computer, what is a trusted source, into the education system and get people to be more aware of the risks. You can definitely design the technology such that it makes a lot more explicit where it's vulnerabilities and where it's more trusted parts are, which is something that we don't do very well at the moment. The little lock on the browser is kind of the high end of our ability to design systems to disclose where security is and why it matters, and there is much more to be done here, because just awareness of the amount of vulnerability is very low.
So there is some more probably that we can do with education and with notifying the public, but it also should be expected that this ability is limited, and it's also, to a large extent, an unfair burden to put on the population at large. It is much more important, I think, that the technology is being designed in the first place, to as much as possible be explicit and transparent about its levels of security, and if those levels of security are not high enough, then that in turn should lead for demands for more secure systems.
Ariel: So one of the things that came up in the report that I found rather disconcerting, was this idea of spear phishing. So can you explain what that is?
Shahar: We are familiar with phishing in general, which is when you pretend to be someone or something that you're not in order to gain your victim's trust and get them to disclose information that they should not be disclosing to you as a malicious actor. So you could pretend to be the bank and ask them to put in their username and password, and now you have access to their bank account and can transfer away their funds. If this is part of a much larger campaign, you could just pretend to be their friend, or their secretary, or someone who wants to give them a prize, get them to trust you, get one of the passwords that maybe they are using, and maybe all you do with that is you use that trust to talk to someone else who is much more concerned. So now that I have the username and password, say for the email or the Facebook account of some low-ranking employee in a company, I can start messaging their boss and pretending to be them and maybe get even more passwords and more access through that.
Phishing is usually kind of a “spray and pray” approach. You have a, "I'm a Nigerian prince, I have all of this money stocked in Africa, I'll give you a cut if you help me move it out of the country, you need to send me some money." You send this to millions of people, and maybe one or two fall for it. The cost for the sender is not very high, but the success rate is also very, very low.
Spear phishing on the other hand, is when you find a particular target, and you spend quite a lot of time profiling them and understanding what their interests are, what their social circles are, and then you craft a message that is very likely to work on them, because it plays to their ego, it plays to their normal routine, it plays on their interests and so on.
In the report we talk about this research by ZeroFOX, where they took a very simple version of this. They said, let's look at what people tweet about, we'll take that as an indication of the stuff that they're interested in. We will train a machine learning algorithm to create a model of the topics that people are interested in, form the tweets, craft a malicious tweet that is based on those topics of interest and have that be a link to a malicious site. So instead of sending kind of generally, "Check this out, super cool website," with a link to a malicious website most people know not to click on, it will be, "Oh, you are clearly interested in sports in this particular country, have you seen what happened, like the new hire in this team?" Or, "You're interested in archeology, crazy new report about recent finds in the pyramids,” or something. And what they showed was that, once that they've kind of created the bot, that bot then crafted targeted messages, those spear phishing messages, to a large number of users, and in principle they could scale it up indefinitely because now it's software, and the click through rate was very high. I think it was something like 30 percent, which is orders of magnitude more than you get with phishing.
So automating spear phishing changes what used to be a trade off between spray and pray, target millions of people, but very few of them would click on it, or spear phishing where you target only a few individuals with very high success rates -- now you can target millions of people and customize the message to each one so you have high success rates for all of them. Which means that, you and me, who previously wouldn't be very high on the target list for cyber criminals or other cyber attackers can now become targets simply because the cost is very low.
Ariel: So the cost is low, I don't think I'm the only person who likes to think that I'm pretty good at recognizing sort of these phishing scams and stuff like that. I'm assuming these are going to also become harder for us to identify?
Shahar: Yep. So the idea is that the moment you have access to people’s data, because they're explicit on social media about their interests and about their circles of friends, then the better you get at crafting messages and, say, comparing them to authentic messages from people, and saying, “oh this is not quite right, we are going to tweak the algorithm until we get something that looks a lot like something a human would write.” Quite quickly you could get to the point where computers are generating, say, to begin with texts that are indistinguishable from what a human would write, but increasingly also images, audio segments, maybe entire websites. As long as the motivation or the potential for profit is there, it seems like the technology, either the ones that we have now or the ones that we can foresee in the five years, would allow these kinds of advances to take place.
Ariel: Okay. So I want to touch quickly on the idea of adversarial examples. There was an XKCD cartoon that came out a week or two ago about self driving cars and the character says, "I worry about self driving car safety features, what's to stop someone from painting fake lines on the road or dropping a cutout of a pedestrian onto a highway to make cars swerve and crash," and then realizes all of those things would also work on human drivers. Sort of a personal story, I used to live on a street called Climax and I actually lived at the top of Climax, and I have never seen a street sign stolen more in my life, it was often the street sign just wasn't there. So my guess is it's not that hard to steal a stop sign if someone really wanted to mess around with drivers, and yet we don't see that happen very often.
So I was hoping both of you could weigh in a little bit on what you think artificial intelligence is going to change about these types of scenarios where it seems like the risk will be higher for things like adversarial examples versus just stealing a stop sign.
Victoria: I agree that there is certainly a reason for optimism in the fact that most people just aren't going to mess with the technology, that there aren't that many actual bad actors out there who want to mess it up. On the other hand, as Shahar said earlier, democratizing both the technology and the ways to mess with it, to interfere with it, does make that more likely. For example, the ways in which you could provide adversarial examples to cars, can be quite a bit more subtle than stealing a stop sign or dropping a fake body on the road or anything like that. For example, you can put patches on a stop sign that look like noise or just look like rectangles in certain places and humans might not even think to remove them, because to humans they're not a problem. But an autonomous car might interpret that as a speed limit sign instead of a stop sign, and similarly, more generally people can use adversarial patches to fool various vision systems, for example if they don't want to be identified by a surveillance camera or something like that.
So a lot of these methods, people can just read about it online, there are papers in arXiv and I think the fact that they are so widely available might make it easier for people to interfere with technology more, and basically might make this happen more often. It's also the case that the vulnerabilities of AI are different than the vulnerabilities of humans, so it might lead to different ways that it can fail that humans are not used to, and ways in which humans would not fail. So all of these things need to be considered, and of course, as technologists, we need to think about ways in which things can go wrong, whether it is presently highly likely, or not.
Ariel: So that leads to another question that I want to ask, but before I go there, Shahar, was there anything you wanted to add?
Shahar: I think that covers almost all of the basics, but I’d maybe stress a couple of these points. One thing about machines failing in ways that are different from how humans fail, it means that you can craft an attack that would only mess up a self driving car, but wouldn't mess up a human driver. And that means let's say, you can go in the middle of the night and put some stickers on and you are long gone from the scene by the time something bad happens. So this diminished ability to attribute the attack, might be something that means that more people feel like they can get away with it.
Another one is that we see people much more willing to perform malicious or borderline acts online. So it's important, I mean we often talk about adversarial examples as things that affect vision systems, because that's where a lot of the literature is, but it is very likely -- in fact, there are several examples that also things like anomaly detection that uses machine learning patterns, malicious code detection that is based on machine-learned patterns, anomaly detection in networks and so on, all of these have their kinds of adversarial examples as well. And so thinking about adversarial examples against defensive systems and adversarial examples against systems that are only available online, brings us back to one attacker somewhere in the world could have access to your system and so the fact that most people are not attackers doesn't really help you defense-wise.
Ariel: And, so this whole report is about how AI can be misused, but obviously the AI safety community and AI safety research goes far beyond that. So especially in the short term, do you see misuse or just general safety and design issues to be a bigger deal?
Victoria: I think it is quite difficult to say which of them would be a bigger deal. I think both misuse and accidents are something that are going to increase in importance and become more challenging and these are things that we really need to be working on as a research community.
Shahar: Yeah, I agree. We wrote this report not because we don't think accident risk and safety risk matters are important -- we think they are very important. We just thought that there was some pretty good technical reports out there outlining the risks from accident with near-term machine learning and with long-term and some of the researching that could be used to address them, and we felt like a similar thing was missing for misuse, which was why we wrote that report.
Both are going to be very important, and to some extent there is going to be an interplay. It is possible that systems that are more interpretable are also easier to secure. It might be the case that if there is some restriction in the diffusion of capabilities that also means that there is less incentive to cut corners to out-compete someone else by skimping on safety and so on. So there are strategic questions across both misuse and accidents, but I agree with Victoria, probably if we don't do our job, we are just going to see more and more of both of these categories causing harm in the world, and more reason to work on both of them. I think both fields need to grow.
Victoria: I just wanted to add, a common cause of both accident risks and misuse risks that might happen in the future is just that these technologies are advancing quickly and there are often unforeseen and surprising ways in which they can fail, either by accident or by having vulnerabilities that can be misused by bad actors. And so as the technology continues to advance quickly we really need to be on the lookout for new ways that it can fail, new accidents but also new ways in which it can be used for harm by bad actors.
Ariel: So one of the things that I got out of this report, and that I think is also coming through now is, it's kind of depressing. And I found myself often wondering ... So at FLI, especially now we've got the new grants that are focused more on AGI, we're worried about some of these bigger, longer-term issues, but with these shorter-term things, I sometimes find myself wondering if we're even going to make it to AGI, or if something is going to happen that prevents that development in some way. So I was hoping you could speak to that a little bit.
Shahar: Maybe I'll start with the Malicious Use report, and apologize for its somewhat gloomy perspective. So it should probably be mentioned that, I think almost all of the authors of the report are somewhere between fairly and very optimistic about artificial intelligence. So it's much more the fact that we see this technology going, we want to see it developed quickly, at least in various narrow domains that are of very high importance, like medicine, like self driving cars -- I'm personally quite a big fan. We think that the best way to, if we can foresee and design around or against the misuse risks, then we will eventually end up with a technology that it is more mature, that is more acceptable, that is more trusted because it is trustworthy, because it is secure. We think it is going to be much better to plan for these things in advance.
It is also, again, say we use electricity as an analogy, if I just sat down at the beginning of the age of electricity and I wrote a report about how many people were going to be electrocuted, it would look like a very sad thing. And it's true, there has been a rapid increase in the number of people who die from electrocution compared to before the invention of electricity and much safety has been built since then to make sure that that risk is minimized, but of course, the benefits have far, far, far outweighed the risks when it comes to electricity and we expect, probably, hopefully, if we take the right actions, like we lay out in the report, then the same is going to be true for misuse risk for AI. At least half of the report, all of Appendix B and a good chunk of the parts before it, talk about what we can do to mitigate those risks, so hopefully the message is not entirely doom and gloom.
Victoria: I think that the things we need to do remain the same no matter how far away we expect these different developments to happen. We need to be looking out for ways that things can fail. We need to be thinking in advance about ways that things can fail, and not wait until problems show up and we actually see that they're happening. Of course, we often will see problems show up, but in these matters an ounce of prevention can be worth a pound of cure, and there are some mistakes that might just be too costly. For example, if you have some advanced AI that is running the electrical grid or the financial system, we really don't want that thing to, hack its reward function.
So there are various predictions about how soon different transformative developments of AI might happen and it is possible that things might go awry with AI before we get to general intelligence and what we need to do is basically work hard to try to prevent these kinds of accidents or misuse from happening and try to make sure that AI is ultimately beneficial, because the whole point of building it is because it would be able to solve big problems that we cannot solve by ourselves. So let's make sure that we get there and that we sort of handle this with responsibility and foresight the whole way.
Ariel: I want to go back to the very first comments that you made about where we were three years ago. How have things changed in the last three years and where do you see the AI safety community today?
Victoria: In the last three years, we've seen the AI safety research community get a fair bit bigger and topics of AI safety have become more mainstream, so I will say that long-term AI safety is definitely less controversial and there are more people engaging with the questions and actually working on them. While near-term safety, like questions of fairness and privacy and technological unemployment and so on, I would say that's definitely mainstream at this point and a lot of people are thinking about that and working on that.
In terms of long term AI safety or AGI safety we've seen teams spring up, for example, both DeepMind and OpenAI have a safety team that's focusing on these sort of technical problems, which includes myself on the DeepMind side. There have been some really interesting bits of progress in technical AI safety. For example, there has been some progress in reward learning and generally value learning. For example, the cooperative inverse reinforcement learning work from Berkeley. There has been some great work from MIRI on logical induction and quantilizing agents and that sort of thing. There have been some papers at mainstream machine learning conferences that focus on technical AI safety, for example, there was an interruptibility paper at NIPS last year and generally I've been seeing more presence of these topics in the big conferences, which is really encouraging.
On a more meta level, it has been really exciting to see the Concrete Problems in AI Safety research agenda come out two years ago. I think that's really been helpful to the field. So these are only some of the exciting advances that have happened.
Ariel: Great. And so, Victoria, I do want to turn now to some of the stuff about FLI's newest grants. We have an RFP that included quite a few examples and I was hoping you could explain at least two or three of them, but before we get to that if you could quickly define what artificial general intelligence (AGI) is, what we mean when we refer to long-term AI? I think those are the two big ones that have come up so far.
Victoria: So, artificial general intelligence is this idea of an AI system that can learn to solve many different tasks. Some people define this in terms of human-level intelligence as an AI system that will be able to learn to do all human jobs, for example. And this contrasts to the kind of AI systems that we have today which we could call “narrow AI,” in the sense that they specialize in some task or class of tasks that they can do.
So, for example Alpha Zero is a system that is really good at various games like Go and Chess and so on, but it would not be able to, for example, clean up a room, because that's not in its class of tasks. While if you look at human intelligence we would say that humans are our go-to example of general intelligence because we can learn to do new things, we can adapt to new tasks and new environments that we haven't seen before and we can transfer our knowledge that we have acquired through previous experience, that might not be in exactly the same settings, to whatever we are trying to do at the moment.
So, AGI is the idea of building an AI system that is also able to do that -- not necessarily in the same way as humans, like it doesn't necessarily have to be human-like to be able to perform the same tasks, or it doesn't have to be structured the way a human mind is structured. So the definition of AGI is about what it's capable of rather than how it can do those things. I guess the emphasis there is on the word general.
In terms of the FLI grant program this year, it is specifically focused on the AGI safety issue, which we also call long-term AI safety. Long term here doesn't necessarily mean that it's 100 years away. We don't know how far away AGI actually is; the opinions of experts vary quite widely on that. But it's more emphasizing that it's not an immediate problem in the sense that we don't have AGI yet, but we are trying to foresee what kind of problems might happen with AGI and make sure that if and when AGI is built that it is as safe and aligned with human preferences as possible.
And in particular as a result of the mainstreaming of AI safety that has happened in the past two years, partly, as I like to think, due to FLI's efforts, at this point it makes sense to focus on long-term safety more specifically since this is still the most neglected area in the AI safety field. I've been very happy to see lots and lots of work happening these days on adversarial examples, fairness, privacy, unemployment, security and so on. I think this allows us to really zoom in and focus on AGI safety specifically to make sure that there's enough good technical work going on in this field and that the big technical problems get as much progress as possible and that the research community continues to grow and do well.
In terms of the kind of problems that I would want to see solved, I think some of the most difficult problems in AI safety that sort of feed into a lot of the problem areas that we have are things like Goodhart’s Law. Goodhart’s Law is basically that, when a metric becomes a target, it ceases to be a good metric. And the way this applies to AI is that if we make some kind of specification of what objective we want the AI system to optimize for -- for example this could be a reward function, or a utility function, or something like that -- then, this specification becomes sort of a proxy or a metric for our real preferences, which are really hard to pin down in full detail. Then if the AI system explicitly tries to optimize for the metric or for that proxy, for whatever we specify, for the reward function that we gave, then it will often find some ways to follow the letter but not the spirit of that specification.
Ariel: Can you give a real life example of Goodhart’s Law today that people can use as an analogy?
Victoria: Certainly. So Goodhart’s Law was not originally coined in AI. This is something that generally exists in economics and in human organizations. For example, if employees at a company have their own incentives in some way, like they are incentivized to clock in as many hours as possible, then they might find a way to do that without actually doing a lot of work. If you're not measuring that then the number of hours spent at work might be correlated with how much output you produce, but if you just start rewarding people for the number of hours then maybe they'll just play video games all day, but they'll be in the office. That could be a human example.
There are also a lot of AI examples these days of reward functions that turn out not to give good incentives to AI systems.
Ariel: For a human example, would the issues that we're seeing with standardized testing be an example of this?
Victoria: Oh, certainly, yes. I think standardized testing is a great example where when students are optimizing for doing well on the tests, then the test is a metric and maybe the real thing you want is learning, but if they are just optimizing for doing well on the test, then actually learning can suffer because they find some way to just memorize or study for particular problems that will show up on the test, which is not necessarily a good way to learn.
And if we get back to AI examples, there was a nice example from OpenAI last year where they had this reinforcement learning agent that was playing a boat racing game and the objective of the boat racing game was to go along the racetrack as fast as possible and finish the race before the other boats do, and to encourage the player to go along the track there were some reward points -- little blocks that you have to hit to get rewards -- that were along the track, and then the agent just found a degenerate solution where it would just go in a circle and hit the same blocks over and over again and get lots of reward, but it was not actually playing the game or winning the race or anything like that. This is an example of Goodhart’s Law in action. There are plenty of examples of this sort with present day reinforcement learning systems. Often when people are designing a reward function for a reinforcement learning system they end up adjusting it a number of times to eliminate these sort of degenerate solutions that happen.
And this is not limited to reinforcement learning agents. For example, recently there was a great paper that came out about many examples of Goodhart’s Law in evolutionary algorithms. For example, if some evolved agents were incentivized to move quickly in some direction, then they might just evolve to be really tall and then they fall in this direction instead of actually learning to move. There are lots and lots of examples of this and I think that as AI systems become more advanced and more powerful, then I think they'll just get more clever at finding these sort of loopholes in our specifications of what we want them to do. Goodhart’s Law is, I would say, part of what's behind various other AI safety issues. For example, negative side effects are often caused by the agent’s specification being incomplete, so there's something that we didn't specify.
For example, if we want a robot to carry a box from point A to point B, then if we just reward it for getting the box to point B as fast as possible, then if there's something in the path of the robot -- for example, there's a vase there -- then it will not have an incentive to go around the vase, it would just go right through the vase and break it just to get to point B as fast as possible, and this is an issue because our specification did not include a term for the state of the vase. So, when data is just optimizing for this reward that's all about the box, then it doesn't have an incentive to avoid disruptions to the environment.
Ariel: So I want to interrupt with a quick question. These examples so far, we're obviously worried about them with a technology as powerful as AGI, but they're also things that apply today. As you mentioned, Goodhart’s Law doesn't even just apply to AI. What progress has been made so far? Are we seeing progress already in addressing some of these issues?
Victoria: We haven't seen so much progress in addressing these questions in a very general sort of way, because when you're building a narrow AI system, then you can often get away with a sort of trial and error approach where you run it and maybe it does something stupid, finds some degenerate solution, then you tweak your reward function, you run it again and maybe it finds a different degenerate solution and then so on and so forth until you arrive at some reward function that doesn't lead to obvious failure cases like that. For many narrow systems and narrow applications where you can sort of foresee all the ways in which things can go wrong, and just penalize all those ways or build a reward function that avoids all of those failure modes, then there isn't so much need to find a general solution to these problems. While as we get closer to general intelligence, there will be more need for more principled and more general approaches to these problems.
For example, how do we build an agent that has some idea of what side effects are, or what it means to disrupt an environment that it's in, no matter what environment you put it in. That's something we don't have yet. One of the promising approaches that has been gaining traction recently is reward learning. For example, there was this paper in collaboration between DeepMind and OpenAI called Deep Reinforcement Learning from Human Preferences, where instead of directly specifying a reward function for the agent, it learns a reward function from human feedback. Where, for example, if your agent is this simulated little noodle or hopper that's trying to do a backflip, then the human would just look at two videos off the agent trying to do a backflip and say, "Well this one looks more like a back flip." And so, you have a bunch of data from the human about what is more similar to what the human wants the agent to do.
With this kind of human feedback, unlike, for example, demonstrations, the agent can learn something that the human might not be able to demonstrate very easily. For example, even if I cannot do a backflip myself, I can still judge whether someone else has successfully done a backflip or whether this reinforcement agent has done a backflip. This is promising for getting agents to potentially solve problems that humans cannot solve or do things that humans cannot demonstrate. Of course, with human feedback and human-in-the-loop kind of work, there is always the question of scalability because human time is expensive and we want the agent to learn as efficiently as possible from limited human feedback and we also want to make sure that the agent actually gets human feedback in all the relevant situations so it learns to generalize correctly to new situations. There are a lot of remaining open problems in this area as well, but the progress so far has been quite encouraging.
Ariel: Are there others that you want to talk about?
Victoria: Maybe I'll talk about one other question, which is that of interpretability. Interpretability of AI systems is something that is a big area right now in near-term AI safety that increasingly more people on the research community are thinking about and working on, that is also quite relevant in long-term AI safety. This generally has to do with being able to understand why your system does things a certain way, or makes certain decisions or predictions, or in the case of an agent, why it takes certain actions and also understanding what different components of the system are looking for in the data or how the system is influenced by different inputs and so on. Basically making it less of a black box, and I think there is a reputation for deep learning systems in particular that they are seen as black boxes and it is true that they are quite complex, but I think they don't necessarily have to be black boxes and there has certainly been progress in trying to explain why they do things.
Ariel: Do you have real world examples?
Victoria: So, for example, if you have some AI system that's used for medical diagnosis, then on the one hand you could have something simple like a decision tree that just looks at your x-ray and if there is something in a certain position then it gives you a certain diagnosis, and otherwise it doesn't and so on. Or you could have a more complex system like a neural network that takes into account a lot more factors and then at the end it says, like maybe this person has cancer or maybe this person has something else. But it might not be immediately clear why that diagnosis was made. Particularly in sensitive applications like that, what sometimes happens is that people end up using simpler systems that they find more understandable where they can say why a certain diagnosis was made, even if those systems are less accurate, and that's one of the important cases for interpretability where if we figure out how to make these more powerful systems more interpretable, for example, through visualization techniques, then they would actually become more useful in these really important applications where it actually matters not just to predict well, but to explain where the prediction came from.
And another area, another example is an algorithm that's deciding whether to give someone a loan or a mortgage, then if someone's loan application got rejected then they would really want to know why it got rejected. So the algorithm has to be able to point at some variables or some other aspect of the data that influences decisions or you might need to be able to explain how the data will need to change for the decision to change, what variables would need to be changed by a certain amount for the decision to be different. So these are just some examples of how this can be important and how this is already important. And this kind of interpretability of present day systems is of course already on a lot of people's minds. I think it is also important to think about interpretability in the longer term as we build more general AI systems that will continue to be important or maybe even become more important to be able to look inside them and be able to check if they have particular concepts that they're representing.
Like, for example, especially from a safety perspective, whether your system was thinking about the off switch and if it's thinking about whether it's going to be turned off, that might be something good to monitor for. We also would want to be able to explain how our systems fail and why they fail. This is, of course, quite relevant today if, let's say your medical diagnosis AI makes a mistake and we want to know what led to that, why it made the wrong diagnosis. Also on the longer term we want to know why an AI system hacks its reward function, what is it thinking -- well "thinking" with quotes, of course -- while it's following a degenerate solution instead of the kind of solution we would want it to find. So, what is the boat race agent that I mentioned earlier paying attention to while it's going in circles and collecting the same rewards over and over again instead of playing the game, that kind of thing. I think the particular application of interpretability techniques to safety problems is going to be important and it's one of the examples of the kind of work that we're looking for in the in the RFP.
Ariel: Awesome. Okay, and so, we've been talking about how all these things can go wrong and we're trying to do all this research to make sure things don't go wrong, and yet basically we think it's worthwhile to continue designing artificial intelligence, that no one's looking at this and saying "Oh my god, artificial intelligence is awful, we need to stop studying it or developing it." So what are the benefits that basically make these risks worth the risk?
Shahar: So I think one thing is in the domain of narrow applications, it's very easy to make analogies to software, right? For the things that we have been able to hand over to computers, they really have been the most boring and tedious and repetitive things that humans can do and we now no longer need to do them and productivity has gone up and people are generally happier and they can get paid more for doing more interesting things and we can just build bigger systems because we can hand off the control of them to machines that don't need to sleep and don't make small mistakes in calculations. Now the promise of turning that and adding to that all of the narrow things that experts can do, whether it's improving medical diagnosis, whether it's maybe farther down the line some elements of drug discovery, whether it's piloting a car or operating machinery, many of these areas where human labor is currently required because there is a fuzziness to the task, it does not enable a software engineer to come in and code an algorithm, but maybe with machine learning in the not too distant future we'll be able to turn them over to machines.
It means taking some skills that only a few individuals in the world can do and making those available to everyone around the world in some domains. That seems, I mean, concrete examples are, the ones that I have I try to find the companies that do them and get involved with them because I want to see them happen sooner and the ones that I can't imagine yet, someone will come along and make a company out of it, or a not-for-profit for it. But we've seen applications from agriculture, to medicine, to computer security, to entertainment and art, and driving and transport, and in all of these I think we're just gonna be seeing even more. I think we're gonna have more creative products out there that were designed in collaboration between humans and machines. We're gonna see more creative solutions to scientific engineering problems. We're gonna see those professions where really good advice is very valuable, but there are only so many people who can help you -- so if I'm thinking of doctors and lawyers, taking some of that advice and making it universally accessible through an app just makes life smoother. These are some of the examples that come to my mind.
Ariel: Okay, great. Victoria what are the benefits that you think make these risks worth addressing?
Victoria: I think there are many ways in which AI systems can make our lives a lot better and make the world a lot better especially as we build more general systems that are more adaptable. For example, these systems could help us with designing better institutions and better infrastructure, better health systems or electrical systems or what have you. Even now, there are examples like the Google project on optimizing the data center energy use using machine learning, which is something that Deep Mind was working on, where the use of machine learning algorithms to automate energy used in the data centers improved their energy efficiency by I think something like 40 percent. That's of course with fairly narrow AI systems.
I think as we build more general AI systems we can expect, we can hope for really creative and innovative solutions to the big problems that humans face. So you can think of something like AlphaGo's famous “move 37” that overturned thousands of years of human wisdom in Go. What if you can build even more general and even more creative systems and apply them to real world problems? I think there is great promise in that. I think this can really transform the world in a positive direction, and we just have to make sure that as the systems are built that we think about safety from the get go and think about it in advance and trying to build them to be as resistant to accidents and misuse as possible so that all these benefits can actually be achieved.
The things I mentioned were only examples of the possible benefits. Imagine if you could have an AI scientist that's trying to develop better drugs against diseases that have really resisted treatment or more generally just doing science faster and better if you actually have more general AI systems that can think as flexibly as humans can about these sort of difficult problems. And they would not have some of the limitations that humans have where, for example, our attention is limited our memory is limited, while AI could be, at least theoretically, unlimited in it's processing power, in the resources available to it, it can be more parallelized, it can be more coordinated and I think all of the big problems that are so far unsolved are these sort of coordination problems that require putting together a lot of different pieces of information and a lot of data. And I think there are massive benefits to be reaped there if we can only get to that point safely.
Ariel: Okay, great. Well thank you both so much for being here. I really enjoyed talking with you.
Shahar: Thank you for having us. It's been really fun.
Victoria: Yeah, thank you so much.
Related episodes
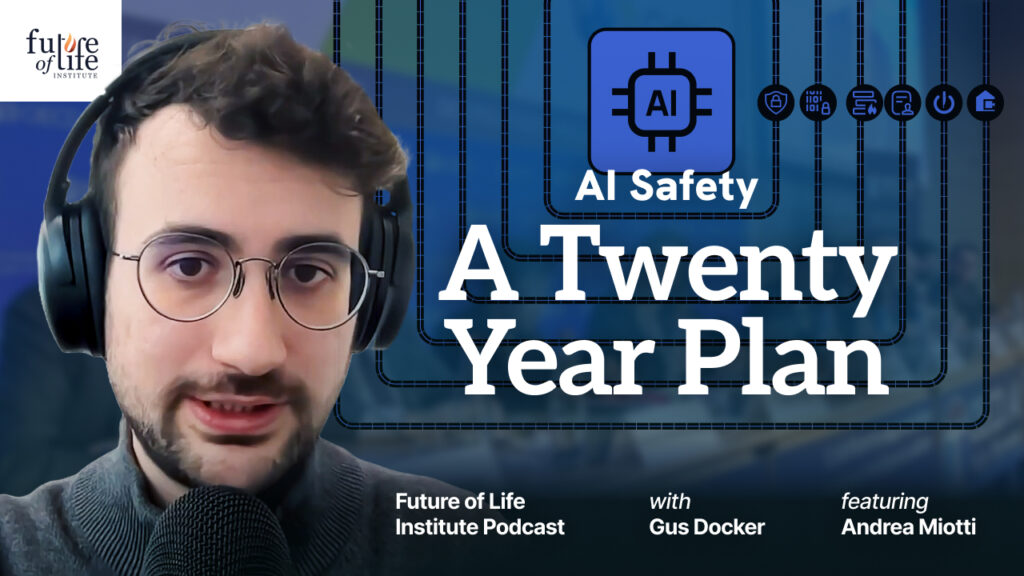
Andrea Miotti on a Narrow Path to Safe, Transformative AI
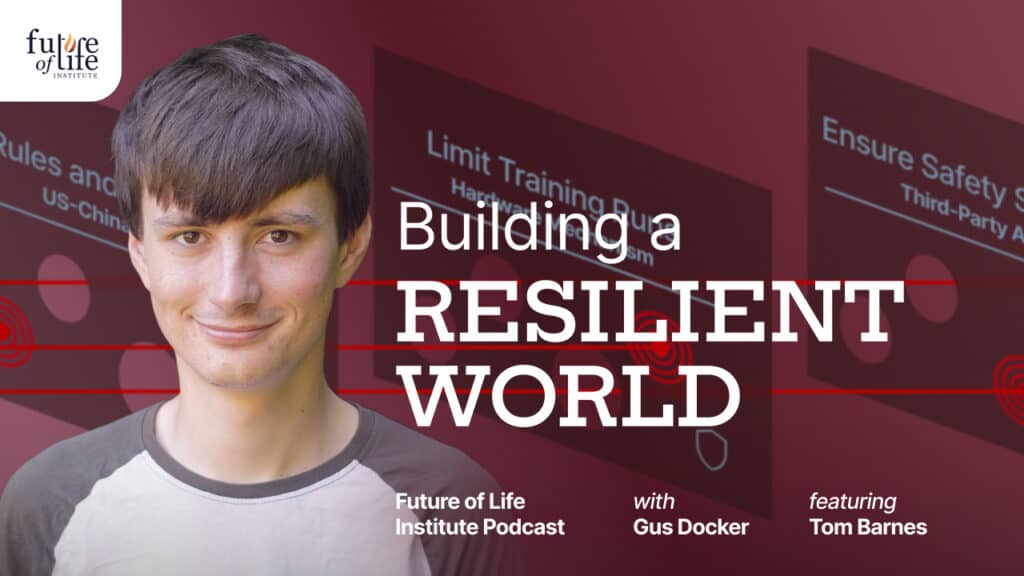