AI Alignment Podcast: Cooperative Inverse Reinforcement Learning with Dylan Hadfield-Menell (Beneficial AGI 2019)
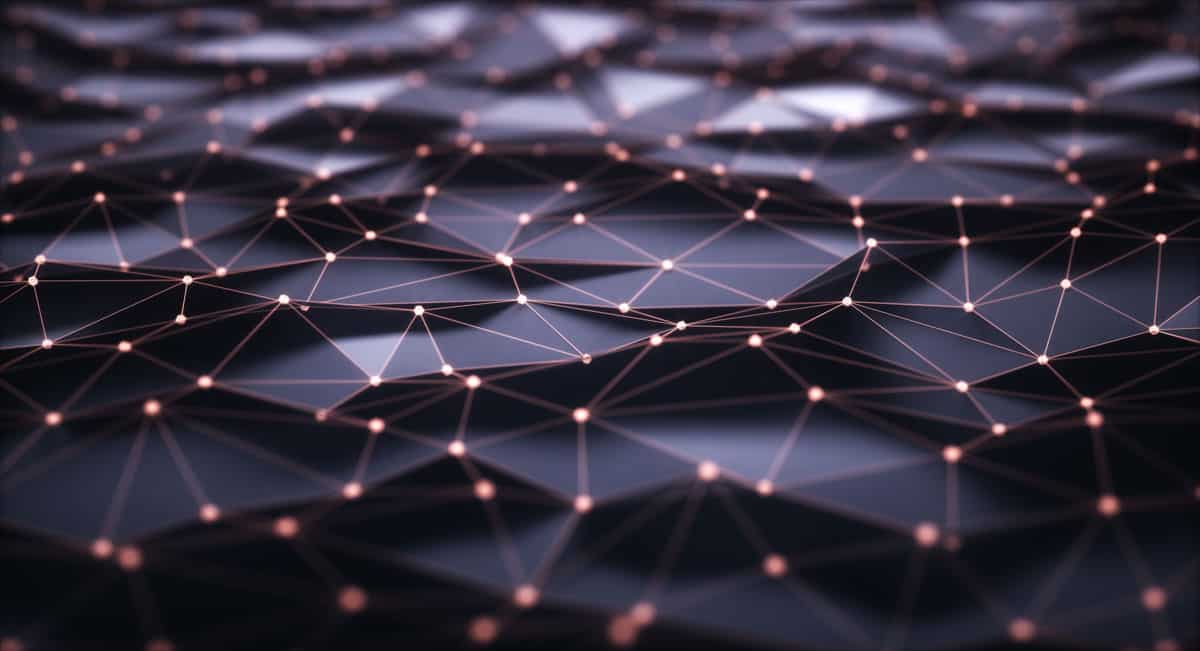
What motivates cooperative inverse reinforcement learning? What can we gain from recontextualizing our safety efforts from the CIRL point of view? What possible role can pre-AGI systems play in amplifying normative processes?
Cooperative Inverse Reinforcement Learning with Dylan Hadfield-Menell is the eighth podcast in the AI Alignment Podcast series, hosted by Lucas Perry and was recorded at the Beneficial AGI 2018 conference in Puerto Rico. For those of you that are new, this series covers and explores the AI alignment problem across a large variety of domains, reflecting the fundamentally interdisciplinary nature of AI alignment. Broadly, Lucas will speak with technical and non-technical researchers across areas such as machine learning, governance, ethics, philosophy, and psychology as they pertain to the project of creating beneficial AI. If this sounds interesting to you, we hope that you will join in the conversations by following us or subscribing to our podcasts on Youtube, SoundCloud, or your preferred podcast site/application.
If you're interested in exploring the interdisciplinary nature of AI alignment, we suggest you take a look here at a preliminary landscape which begins to map this space.
In this podcast, Lucas spoke with Dylan Hadfield-Menell. Dylan is a 5th year PhD student at UC Berkeley advised by Anca Dragan, Pieter Abbeel and Stuart Russell, where he focuses on technical AI alignment research.
Topics discussed in this episode include:
- How CIRL helps to clarify AI alignment and adjacent concepts
- The philosophy of science behind safety theorizing
- CIRL in the context of varying alignment methodologies and it's role
- If short-term AI can be used to amplify normative processes
Transcript
Lucas: Hey everyone, welcome back to the AI Alignment Podcast series. I'm Lucas Perry and today we will be speaking for a second time with Dylan Hadfield-Menell on cooperative inverse reinforcement learning, the philosophy of science behind safety theorizing, CIRL in the context of varying alignment methodologies, and if short term AI can be used to amplify normative processes. This time it just so happened to be an in person discussion and Beneficial AGI 2019, FLI's sequel to the Beneficial AI 2017 conference at Asilomar.
I have a bunch of more conversations that resulted from this conference to post soon and you can find more details about the conference in the coming weeks. As always, if you enjoy this podcast, please subscribe or follow us on your preferred listening platform. As many of you will already know, Dylan is a fifth year Ph.D. student at UC Berkeley, advised by Anca Dragan, Pieter Abbeel, and Stuart Russell, where he focuses on technical AI Alignment research. And so without further ado, I'll give you Dylan.
Thanks so much for coming on the podcast again, Dylan, that's been like a year or something. Good to see you again.
Dylan: Thanks. It's a pleasure to be here.
Lucas: So just to start off, we can go ahead and begin speaking a little bit about your work on cooperative inverse reinforcement learning and whatever sorts of interesting updates or explanation you have there.
Dylan: Thanks. For me, working in cooperative IRL has been a pretty long process, it really sort of dates back to the start of my second year in PhD when my advisor came back from a yearlong sabbatical and suggested that we entirely changed the research direction we were thinking about.
That was to think about AI Alignment and AI Safety and associated concerns that, that might bring. And our first attempt at a really doing research in that area was to try to formalize what's the problem that we're looking at, what are the space of parameters and the space of solutions that we should be thinking about in studying that problem?
And so it led us to write Cooperative Inverse Reinforcement Learning. Since then I've had a large amount of conversations where I've had incredible difficulty trying to convey what it is that we're actually trying to do here and what exactly that paper and idea represents with respect to AI Safety.
One of the big updates for me and one of the big changes since we've spoken last, is getting a little bit of a handle on really what's the value of that as the system. So for me, I've come around to the point of view that really what we were trying to do with cooperative IRL was to propose an alternative definition of what it means for an AI system to be effective or rational in some sense.
And so there's a story you can tell about artificial intelligence, which is that we started off and we observed that people were smart and they were intelligent in some way, and then we observed that we could get computers to do interesting things. And this posed the question of can we get computers to be intelligent? We had no idea what that meant, no idea how to actually nail it down and we discovered that in actually trying to program solutions that looked intelligent, we had a lot of challenges.
So one of the big things that we did as a field was to look over next door into the economics department in some sense, to look at those sort of models that they have of decision theoretic rationality and really looking at homoeconomicous as an ideal to shoot for. From that perspective, actually a lot of the field of AI has shifted to be about effective implementations of homoeconomicous.
In my terminology, this is about systems that are effectively individually rational. These are systems that are good at optimizing for their goals, and a lot of the concerns that we have about AI Safety is that systems optimizing for their own goals could actually lead to very bad outcomes for the rest of us. And so what cooperative IRL attempts to do is to understand what it would mean for a human robot system to behave as a rational agent.
In the sense, we're moving away from having a box drawn around the AI system or the artificial component of the system to having that agent box drawn around the person and the system together, and we're trying to model the sort of important parts of the value alignment problem in our formulation here. And in this case, we went with the simplest possible set of assumptions which are basically that we have a static set of preferences that are the humans preferences that they're trying to optimize. This is effectively the humans welfare.
The world is fully observable and the robot and the person are both working to maximize the humans welfare, but there is this information bottlenecking. This information asymmetry that's present that we think is a fundamental component of the value alignment problem. And so really what cooperative IRL, is it's a definition of how a human and a robot system together can be rational in the context of fixed preferences in a fully observable world state.
Lucas: There's a point of metatheory or coming up with models and theory. It seems like the fundamental issue is given how and just insanely complex AI Alignment is trying to converge on whatever the most efficacious model is, is very, very difficult. People keep flicking back and forth about theoretically how we're actually going to do this. Even in very grid world or toy environments. So it seemed very, very hard to isolate the best variables or what variables can be sort of modeled and tracked in ways that is going to help us most.
Dylan: So, I definitely think that this is not an accurate model of the world and I think that there are assumptions here which, if not appropriately reexamined, would lead to a mismatch between the real world and things that work in theory.
Lucas: Like human beings having static preferences.
Dylan: So for example, yes, I don't claim to know what human preferences really are and this theory is not an attempt to say that they are static. It is an attempt to identify a related problem to the one that we're really faced with, that we can actually make technical and theoretical progress on. That will hopefully lead to insights that may transfer out towards other situations.
I certainly recognize that what I'm calling a theta in that paper is not really the same thing that everyone talks about when we talk about preferences. I, in talking with philosophers, I've discovered, I think it's a little bit more closer to things like welfare in like a moral philosophy context, which maybe you could think about as being a more static object that you would want to optimize.
In some sense theta really is an encoding of what you would like the system to do, in general is what we're assuming there.
Lucas: Because it's static.
Dylan: Yes, and to the extent that you want to have that be changing over time, I think that there's an interesting theoretical question as to how that actually is different, and what types of changes that leads to and whether or not you can always reduce something with non-static preferences to something with static preferences from a mathematical point of view.
Lucas: I can see how moving from static to changing over time just makes it so much more insanely complex.
Dylan: Yeah, and it's also really complex of the level of its Philosophically unclear what the right thing to do.
Lucas: Yeah, that's what I mean. Yeah, you don't even know what it even means to be aligning as the values are changing, like whether or not the agent even thinks that they just moved in the right direction or not.
Dylan: Right, and I also even think I want to point out how uncertain all of these things are. We as people are hierarchical organizations have different behaviors and observation systems and perception systems. And we believe we have preferences, we have a name to that, but there is a sense in which that is ultimately a fiction of some kind.
It's a useful tool that we have to talk about ourselves to talk about others that facilitates interaction and cooperation. And so given that I do not know the answer to these philosophical questions, what can I try to do as a technical researcher to push the problem forward and to make actual progress?
Lucas: Right, and so it's sort of again, like a metatheoretical point and what people are trying to do right now in the context of AI Alignment, it seems that the best thing for people to be doing is sort of to be coming up with these theoretical models and frameworks, which have a minimum set of assumptions which may be almost like the real world but are not, and then making theoretical progress there that will hopefully in the future transfer, as you said to other problems as ML and deep learning gets better and the other tools are getting better so that it'll actually have the other tools to make it work with more complicated assumptions.
Dylan: Yes, I think that's right. The way that I view this as we had AI, is this broad, vague thing. Through the course of AI research, we kind of got to Markov decision processes as a sort of coordinating theory around what it means for us to design good agents, and cooperative IRL is an attempt to take a step from markup decision processes more closely towards the set of problems that we want to study.
Lucas: Right, and so I think this is like a really interesting point that I actually haven't talked to anyone else about and if you have a few more words about it, I think it would be really interesting. So just in terms of being a computer scientist and being someone who is working on the emerging theory of a field. I think it's often unclear what the actual theorizing process is behind how people get to CIRL. How did someone get to debate? How did someone get to iterated amplification?
It seems like you first identify problems which you see to be crucial and then there are some sorts of epistemic and pragmatic heuristics that you apply to try and begin to sculpt a model that might lead to useful insight. Would you have anything to correct or unpack here?
Dylan: I mean, I think that is a pretty good description of a pretty fuzzy process.
Lucas: But like being a scientist or whatever?
Dylan: Yeah. I don't feel comfortable speaking for scientists in general here, but I could maybe say a little bit more about my particular process, which is that I try to think about how I'm looking at the problem differently from other people based on different motivations and different goals that I have. And I try to lean into how that can push us in different directions. There's a lot of other really, really smart people who have tried to do lots of things.
You have to maintain an amount of intellectual humility about your ability to out think the historical components of the field. And for me, I think that in particular for AI Safety, it's thinking about reframing what is the goal that we're shooting towards as a field.
Lucas: Which we don't know.
Dylan: We don't know of those goals are, absolutely. And I think that there is a sense in which the field has not re-examined those goals incredibly deeply. For a little bit, I think that it's so hard to do anything that looks intelligent in the real world that we've been trying to focus on that individually rational Markov decision process model. And I think that a lot of the concerns about AI Safety are really a call for AI as a field to step back and think about what we're trying to accomplish in the world and how can we actually try to achieve beneficial outcomes for society.
Lucas: Yeah, and I guess like a sociological phenomenon within the scientists or people who are committed to empirical things. In terms of reanalyzing what the goal of AI Alignment is, the sort of area of moral philosophy and ethics and other things, which for empirical leaning rational people can be distasteful because you can't just take a telescope to the universe and see like a list of what you ought to do.
And so it seems like people like to defer on these questions. I don't know. Do you have anything else to add here?
Dylan: Yeah. I think computer scientists in particular are selected to be people who like having boxed off problems that they know how to solve and feel comfortable with, and that leaning into getting more people with a humanities bent into computer science and broadly AI in particular, AI Safety especially is really important and I think that's a broad call that we're seeing come from society generally.
Lucas: Yeah, and I think it also might be wrong though to model the humanities questions as those which are not in boxes and cannot be solved. That's sort of like a logical positivist thing to say, that on one end we have the hard things and you just have to look at the world enough and you'll figure it out and then there's the soft squishy things which deal with abstractions that I don't have real answers, but people with fluffy degrees need to come up with things that seem right but aren't really right.
Dylan: I think it would be wrong to take what I just said in that direction, and if that's what it sounds like I definitely want to correct that. I don't think there is a sense in which computer science is a place where there are easy right answers, and that the people in humanities are sort of waving their hands and sort of fluffing around.
This is sort of leaning into making this a more AI value alignment kinds of framing or thinking about it. But when I think about being AI systems into the world, I think about what things can you afford to get wrong in your specification and which things can you not afford to get wrong in your specifications.
In this sense, specifying physics incorrectly is much, much better than specifying the objective incorrectly, at least by default. And the reason for that is what happens to the world when you push it, is a question that you can answer from your observations. And so if you start off in the wrong place, as long as you're learning and adapting, I can reasonably expect my systems do correct to that. Or at least the goal of successful AI research is that your systems will effectively adapt to that.
However, the past that your system is supposed to do is sort of arbitrary in a very fundamental sense. And from that standpoint, it is on you as the system designer to make sure that objective is specified correctly. When I think about what we want to do as a field, I ended up taking a similar lens and that there's a sense in which we as researchers and people and society and philosophers and all of it are trying to figure out what we're trying to do and what we want to task the technology with, and the directions that we want to push it in. And then there are questions of what will the technology be like and how should it function that will be informed by that and shaped by that.
And I think that there is a sense in which that is arbitrary. Now, what is right? That I don't really know the answer to and I'm interested in having those conversations, but they make me feel uneasy. I don't trust myself on those questions, and that could mean that I should learn how to feel more uneasy and think about it more and in doing this research I have been kind of forced into some of those conversations.
But I also do think that for me at least I see a difference between what can we do and what should we do. And thinking about what should we do as a really, really hard question that's different than what can we do.
Lucas: Right. And so I wanna move back towards CIRL, but just to sort of wrap up here on our philosophy of science musings, a thought I had while you were going through that was, at least for now, what I think is fundamentally shared between fields that deal with things that matter, are their concepts deal with meaningfully relevant reference in the world? Like do your concepts refer to meaningful things?
Putting ontology aside, whatever love means or whatever value alignment mean. These are meaningful referents for people and I guess for now if our concepts are actually referring to meaningful things in the world, then it seems important.
Dylan: Yes, I think so. Although, I'm not totally sure I understood that.
Lucas: Sure, that's fine. People will say that humanities or philosophy doesn't have these boxes with like well-defined problems and solutions because they either don't deal with real things in the world or the concepts are so fuzzy that the problems are sort of invented and illusory. Like how many angels can stand on the head of a pin? Like the concepts don't work, aren't real and don't have real referents, but whatever.
And I'm saying the place where philosophy and ethics and computer science and AI Alignment should at least come together for now is where the referents have, where the concepts of meaningful referents in the world?
Dylan: Yes, that is something that I absolutely buy. Yes, I think there's a very real sense in which those questions are harder, but that doesn't mean they're less real or less important.
Lucas: Yes, that's because it's the only point I wanted to push against logical positivism.
Dylan: No, I don't mean to say that the answers are wrong, it's just that they are harder to prove in a real sense.
Lucas: Yeah. I mean, I don't even know if they have answers or if they do or if they're just all wrong, but I'm just open to it and like more excited about everyone coming together thing.
Dylan: Yes, I absolutely agree with that.
Lucas: Cool. So now let's turn it back into the CIRL. So you began by talking about how you and your advisers were having this conceptual shift and framing, then we got into the sort of philosophy of science behind how different models and theories of alignment go. So from here, whatever else you have to say about CIRL.
Dylan: So I think for me the upshot of concerns about advanced AI systems and negative consequence there in really is a call to recognize that the goal of our field is AI Alignment. That almost any AI that's not AI Alignment is solving a sub problem and viewing it only in solving that sub problem is a mistake.
Ultimately, we are in the business of building AI systems that integrate well with humans and human society. And if we don't take that as a fundamental tenant of the field, I think that we are potentially in trouble and I think that that is a perspective that I wish was more pervasive throughout artificial intelligence generally,
Lucas: Right, so I think I do want to move into this view where safety is a normal thing, and like Stuart Russell will say, "People who build bridges all care about safety and there aren't a subsection of bridge builders who work in bridge safety, everyone is part of the bridge safety." And I definitely want to get into that, but I also sort of want to get a little bit more into CIRL and why you think it's so motivating and why this theoretical framing and shift is important or illuminating, and what the specific content of it is.
Dylan: The key thing is that what it does is point out that it doesn't make sense to talk about how well your system is doing without talking about the way in which it was instructed and the type of information that it got. No AI system exists on its own, every AI system has a designer, and it doesn't make sense to talk about the functioning of that system without also talking about how that designer built it, evaluated it and how well it is actually serving those ends.
And I don't think this is some brand new idea that no one's ever known about, I think this is something that is incredibly obvious to practitioners in the field once you pointed out. The process whereby a robot learns to navigate a maze or vacuum a room is not, there is an objective and it optimizes it and then it does it.
What it is that there is a system designer who writes down an objective, selects an optimization algorithm, observes the final behavior of that optimization algorithm, goes back, modifies the objectives, modifies the algorithm, changes hyper parameters, and then runs it again. And there's this iterative process whereby your system eventually ends up getting to the behavior that you wanted to have. And AI researchers have tended to draw a box around. The thing that we call AI is the sort of final component of that.
Lucas: Yeah, it's because at least subjectively and I guess this is sort of illuminated by meditation and Buddhism, is that if you're a computer scientist and you're just completely identified with the process of doing computer science, you're just identified with the problem. And if you just have a little bit of mindfulness and you're like, "Okay, I'm in the context of a process where I'm an agent and trying to align another agent," and if you're not just completely identified with the process and you see the unfolding of the process, then you can do sort of like more of a meta-analysis which takes a broader view of the problem and can then, I guess hopefully work on improving it.
Dylan: Yeah, I think that's exactly right, or at least as I understand that, that's exactly right. And to be a little bit specific about this, we have had these engineering principles and skills that are not in the papers, but they are things that are passed down from Grad student to Grad student within a lab. Their institutional knowledge that exists within a company for how you actually verify and validate your systems, and cooperative IRLs and attempt to take all of that sort of structure that AI systems have existed within and try to bring that into the theoretical frameworks that we actually work with.
Lucas: So can you paint a little picture of what the CIRL model looks like?
Dylan: It exists in a sequential decision making context and we assume we have states of the world and a transition diagram that basically tells us how we get to another state given the previous state and actions from the human and the robot. But the important conceptual shift that it makes is the space of solutions that we're dealing with are combinations of a teaching strategy and a learning strategy.
There is a commitment on the side of the human designers or users of the systems to provide data that is in some way connected to the objectives that they want to be fulfilled. That data can take many forms, it could be in the form of writing down a reward function that ranks a set of alternatives, it could be in the form of providing demonstrations that you expect your system to imitate. It could be in the form of providing binary comparisons between two clearly identified alternatives.
And the other side of the problem is what is the learning strategy that we use? And this is the question of how the robot is actually committing to respond to the observations that we're giving it about what we wanted to do, in the case of a pre-specified proxy reward going to a literal interpretation and a reinforcement learning system, let's say. What the system is committing to doing is optimizing under that set of trajectory rankings and preferences based off the simulation environment that it's in, or the actual physical environment that it's exploring.
When we shift to something like inverse reward design, which is a paper that we released last year, what that says is we'd like the system to look at this ranking of alternatives and actually try to blow that up into a larger uncertainty set over the set of possible consistent rankings with that, and then when you go into deployment, you may be able to leverage that uncertainty to avoid catastrophic failures or generally just unexpected behavior.
Lucas: So this other point I think that you and I discussed briefly, maybe it was actually with Rohan, but it seems like often in terms of AI Alignment, it's almost like we're reasoning from nowhere about abstract agents and that sort of makes the problem extremely difficult. Often, if you just look at human examples, it just becomes super mundane and easy. This sort of conceptual shift can almost I think be framed super simply as like the difference between a teacher trying to teach someone and then a teacher realizing that the teacher is a person that is teaching another student and the teacher can think better about how to teach and then also the process between the teacher and the student and how to improve that at a higher level of attraction.
Dylan: I think that's the direction that we're moving in. What I would say is it's as AI practitioners, we are teaching our systems how to behave and we have developed our strategies for doing that.
And now that we've developed a bunch of strategies that sort of seem to work. I think it's time for us to develop a more rigorous theory of actually how those teaching strategies interact with the final performance of the system.
Lucas: Cool. Is there anything else here that you would like say about CIRL, or any really important points you would like people to get people who are interested in technical AI Alignment or CS students?
Dylan: I think the main point that I would make is that research and thinking about powerful AI systems is valuable, even if you don't think that that's what's going to happen. You don't need to be motivated by those sets of problems in order to recognize that this is actually just basic research into the science of artificial intelligence.
It's got an incredible amount of really interesting problems and the perspectives that you adopt from this framing can be incredibly useful as a comparative advantage over other researchers in the field. I think that'd be my final word here.
Lucas: If I might just ask you one last question. We're at beneficial AGI 2019 right now and we've heard a lot of overviews of different research agendas and methodologies and models and framings for how to best go forth with AI Alignment, which include a vast range of things which work on corrigibility and interpretability and robustness and other things, and the different sort of research agendas and methodologies of places like MIRI who is come out with this new framing on embedded agency, and also different views at OpenAI and DeepMind.
And Eric Drexler has also newly proposed these services based conception of AI where we remove the understanding of powerful AI systems or regular AI systems as agents, which sort of gets us away from a lot of the x-risky problems and global catastrophic risks problems and value alignment problems.
From your point of view, as someone who's worked a lot in CIRL and is the technical alignment researcher, how do you view CIRL in this context and how do you view all of these different emerging approaches right now in AI Alignment?
Dylan: For me, and you know, I should give a disclaimer. This is my research area and so I'm obviously pretty biased to thinking it's incredibly important and good, but for me at least, cooperative IRL is a uniting framework under which I can understand all of those different approaches. I believe that a services type solution to AI Safety or AI Alignment that's actually arguing for a particular type of learning strategy and implementation strategies of CIRL, and I think it can be framed within that system.
Similarly, I had some conversations with people about debate. I believe debate fits really nicely into the framework and we commit to a human strategy of judging debates from systems and we commit to a robot strategy and just putting yourself into two systems and working towards that direction. So for me, it's a way in which I can sort of identify the commonalities between these different approaches and compare and contrast them and then under a set of assumptions about what the world is like, what the space of possible preferences is like and what the space of strategies that people can implement possibly get out some information about which one is better or worse, or which type of strategy is vulnerable to different types of mistakes or errors.
Lucas: Right, so I agree with all of that, the only place that I might want to push back is, it seems that maybe the MIRI embedded agency stuff subsumes everything else. What do you think about that?
Because the framing is like whenever AI researchers draw these models, there are these conceptions of these information channels, right, which are selected by the researchers and which we control, but the universe is really just a big non-dual happening of stuff and agents are embedded in the environment and are almost an identical process within the environment and it's much more fuzzy where the dense causal streams are and where a little causal streams are and stuff like that. It just seems like the MIRI stuff seems to maybe subsume the CIRL and everything else a little bit more, but I don't know.
Dylan: I certainly agree that that's the one that's hardest to fit into the framework, but I would also say that in my mind, I don't know what an agent is. I don't know how to operationalize an agent, I don't actually know what that means in the physical world and I don't know what it means to be an agent. What I do know is that there is a strategy of some sort that we can think of as governing the ways that the system is perform and behave.
I want to be very careful about baking in assumptions in beforehand. And it feels to me like embedded agency is something that I don't fully understand the set of assumptions being made in that framework. I don't necessarily understand how they relate to the systems that we're actually going to build.
Lucas: When people say that an agent is like a fuzzy concept, I think that, that might be surprising to a lot of people who have thought somewhat about the problem because it's like, obviously I know what an agent is, it's different than all the other dead stuff in the world that has goals and it's physically confined and unitary.
If you just like imagine like abiogenesis, how life began. It is the first relatively self-replicating chain of hydrocarbons and agent and you can go from a really small systems to really big systems, which can exhibit certain properties or principles that feel a little bit agenty, but may not be useful. And so I guess if we're going to come up with a definition of it, it should just be something useful for us or something.
Dylan: I think I'm not sure is the most accurate word we can use here. I wish I had a better answer for what this was, maybe I can share one of the thought experiments that convinced me, I was pretty confused about what an agent is.
Lucas: Yeah, sure.
Dylan: It came from thinking about what value alignment is. So if we think about values alignment between two agents and those are both perfectly rational actors, making decisions in the world perfectly in accordance with their values, with full information. I can sort of write down a definition of value alignment, which is basically you're using the same ranking over alternatives that I am.
But a question that we really wanted to try to answer that feels really important is what does it mean to be value aligned in a partial context? If you were a bounded agent, if you're not a perfectly rational agent, what does it actually mean for you to be value aligned? That was the question that we also didn't really know how to answer.
Lucas: My initial reaction is the kind of agent that tries its best with its limited rationality to be like the former thing that you talked about.
Dylan: Right, so that leads to a question that we thought about, so as opposed I have a chess playing agent and it is my chess playing agent and so I wanted to win the game for me. Suppose it's using the correct goal test, so it is actually optimizing for my values. Let's say it's only searching out to depth three, so it's pretty dumb as far as chess players go.
Do I think that that is an agent that is value aligned with me? Maybe. I mean, certainly I can tell the story in one way that it sounds like it is. It's using the correct objective function, it's doing some sort of optimization thing. If it ever identifies a checkmate move in three moves, I will always find that get that back to me. And so that's a sense in which it feels like it is a value aligned agent.
On the other hand, what if it's using a heuristic function which is chosen poorly, or and something closer to an adversarial manner. So now it's a depth three agent that is still using the correct goal test, but it's searching in a way that is adversarially selected. Is that a partially value aligned agent?
Lucas: Sorry, I don't understand what it means to have the same objective function, but be searching in three depth in an adversarial way.
Dylan: In particular, when you're doing a chess search engine, there is your sort of goal tests that you run on your leaves of your search to see if you've actually achieved winning the game. But because you're only doing a partial search, you often have to rely on using a heuristic of some sort to like rank different positions.
Lucas: To cut off parts of the tree.
Dylan: Somewhat to cut off parts of the tree, but also just like you've got different positions, neither of which are winning and you need to choose between those.
Lucas: All right. So there's a heuristic, like it's usually good to take the center or like the queen is something that you should always probably keep.
Dylan: Or these things that are like values of pieces that you can add up was I think one of the problems ...
Lucas: Yeah, and just as like an important note now in terms of the state of machine learning, the heuristics are usually chosen by the programmer. Are system is able to collapse on heuristics themselves?
Dylan: Well, so I'd say one of the big things in like AlphaZero or AlphaGo as an approach is that they applied sort of learning on the heuristic itself and they figured out a way to use the search process to gradually improve the heuristic and have the heuristic actually improving the search process.
And so there's sort of a feedback loop set up in those types of expert iteration systems. What my point here is that when I described that search algorithm to you, I didn't mention what heuristic it was using at all. And so you had no reason to tell me whether or not that system was partially value aligned or not because actually with heuristic is 100 percent of what's going to determine the final performance of the system and whether or not it's actually helping you.
And then the sort of final point I have here that I might be able to confuse you with a little bit more is, what if we just sort of said, "Okay, forget this whole searching business. I'm just going to precompute all the solutions from my search algorithm and I'm going to give you a policy of when you're in this position, do this move. When you're in that position, do that move." And what would it mean for that policy to be values aligned with me?
Lucas: If it did everything that you would have done if you were the one playing the chess game. Like is that value alignment?
Dylan: That certainly perfect imitation, and maybe we [crosstalk 00:33:04]
Lucas: Perfect imitation isn't necessarily value alignment because you don't want it to perfectly imitate you, you want it to win the game.
Dylan: Right.
Lucas: Isn't the easiest way to just sort of understand this is that there are degrees of value alignment and value alignment is the extent to which the thing is able to achieve the goals that you want?
Dylan: Somewhat, but the important thing here is trying to understand what these intuitive notions that we're talking about actually mean for the mathematics of sequential decision making. And so there's a sense in which you and I can talk about partial value alignment and the agents that are trying to help you. But if we actually look at the math of the problem, it's actually very hard to understand how that actually translates. Like mathematically I have lots of properties that I could write down and I don't know which one of those I want to call partial value alignment.
Lucas: You know more about the math than I do, but the percentage chance of a thing achieving the goal is the degree to which its value aligned? If you're certain that the end towards which is striving, and the end towards what you want it to strive?
Dylan: Right, but that striving term is a hard one, right? Because if your goals aren't achievable then it's impossible to be value aligned with you in that sense.
Lucas: Yeah, you have to measure the degree to which the end towards which it's striving is the end towards what you want it to strive and then also measure the degree to which the way that it tries to get to what you want is efficacious or ...
Dylan: Right. I think that intuitively I agree with you and I know what you mean, but it's like I can do things like I can write down a reward function and I can say how well does this system optimize that reward function? And we could ask whether or not that means its value aligned with it or not. But to me, that just sounds like the question of like is your policy optimal and the sort of more standard context.
Lucas: All right, so have you written about how you think that CIRL subsumes all of these other methodologies? And if it does subsume these other AI Alignment methodologies. How do you think that will influence or affect the way we should think about the other ones?
Dylan: I haven't written that explicitly, but when I've tried to convey is that it's a formalization of the type of problem we're trying to solve. I think describing this subsuming them is not quite right.
Lucas: It contextualizes them and it brings light to them by providing framing.
Dylan: It gives me a way to compare those different approaches and understand what's different and what's the same between them, and in what ways are they ... like in what scenarios do we expect them to work out versus not? One thing that we've been thinking about recently is what happens when the person doesn't know immediately and what they're trying to do.
So if we imagine that there is in fact the static set of preferences, the person's trying to optimize, so we're still making that assumption, but assuming that those preferences are revealed to the person over time through experience or interaction with the world. That is a richer class of value alignment problems than cooperative IRL deals with. It's really closer to what we are attempting to do right now.
Lucas: Yeah, and I mean that doesn't even include value degeneracy, like what if I get hooked on drugs in the next three years and all my values go and my IRL agent works on assumptions that I'm always updating towards what I want, but you know ...
Dylan: Yes, and I think that's where you get these questions of changing preferences that make it hard to really think through things. I think there's a philosophical stance you're taking there, which is that your values have changed rather than your beliefs have changed there.
In the sense that wire-heading is a phenomenon that we see in people and in general learning agents, and if you are attempting to help it learning agent, you must be aware of the fact that wire-heading is a possibility and possibly bad. And then it's incredibly hard to distinguish from someone who's just found something that they really like and want to do.
When you should make that distinction or how you should make that distinction is a really challenging question, that's not a purely technical computer science question.
Lucas: Yeah, but even at the same time, I would like to demystify it a bit. If your friend got hooked on drugs, it's pretty obvious for you why it's bad, it's bad because he's losing control, it's bad because he's sacrificing all of his other values. It's bad because he's shortening his life span by a lot.
I just mean to win again, in this way, it's obvious in ways in which humans do this, so I guess if we take biological inspired approaches to understanding cognition and transferring how humans deal with these things into AI machines, at least at face value seems like a good way of doing it, I guess.
Dylan: Yes, everything that you said I agree with. My point is that those are in a very real sense, normative assumptions that you as that person's friend are able to bring to the analysis of that problem, and in in some ways there is an arbitrariness to labeling that as bad.
Lucas: Yeah, so the normative issue is obviously very contentious and needs to be addressed more, but at the same time society has come to very clear solutions to normative problems like murder is basically a solved normative problem. There's a degree to which it's super obvious that certain normative questions are just answer it and we should I guess practice epistemic humility and whatever here obviously.
Dylan: Right, and I don't disagree with you on that point, but I think what I'd say is, as a research problem there's a real question to getting a better understanding of the normative processes whereby we got to solving that question. Like what is the human normative process? It's a collective societal system. How does that system evolve and change? And then how should machines or other intelligent entities integrate into that system without either subsuming or destroying it in bad ways? I think that's what I'm trying to get at when I make these points. There is something about what we're doing here as a society that gets us to labeling these things in the ways that we do and calling them good or bad.
And on the one hand, as a person believe that there are correct answers and I know what I think is right versus what I think is wrong. And then as a scientist I want to try to take a little bit more of an outside view and try to understand like what is the process whereby we as a society or as genetic beings started doing that? Understanding what that process is and how that process evolves, and actually what that looks like in people now is a really critical research program.
Lucas: So one thing that I tried to cover in my panel yesterday on what civilization should strive for, is in the short, medium, to longterm the potential role that narrow to general AI systems might play in amplifying human moral decision making.
Solving as you were discussing this sort of deliberative, normative process that human beings undergo to total converge on an idea. I'm just curious to know like with more narrow systems, if you're optimistic about ways in which AI can sort of help and elucidate our moral decision making at work to amplify it.
And before I let you start, I guess there's one other thing that I said that I think Rohin Shah pointed out to me that was particularly helpful in one place. But beyond the moral decision making, the narrow AI systems can help us by making the moral decision make, the decisions that we implement them faster than we could.
Depending on the way a self-driving car decides to crash is like an expression of our moral decision making in like a fast computery way. I'm just saying like beyond ways in which AI systems make moral decisions for us faster than we can, I don't know, maybe in courts or other things which seem morally contentious. Are there also other ways in which they can actually help the deliberative process examining massive amounts of moral information or like a value information or analyzing something like an aggregated well-being index where we try to understand more so how policies impact the wellbeing of people or like what sorts of moral decisions lead to good outcomes, whatever. So whatever you have to say to that.
Dylan: Yes, I definitely want to echo that. We can sort of get a lot of pre-deliberation into a fast timescale reaction with AI systems and I think that that is a way for us to improve how we act in the quality of the things that we do from a moral perspective. That you do see a real path and to actually bringing that to be in the world.
In terms of helping us actually deliberate better, I think that is a harder problem that I think is absolutely worth more people thinking about but I don't know the answers here. What I do think is that if we have a better understanding of what the deliberative process is, I think there are correct questions to look at to try to get to that or not, the moral questions about what's right and what's wrong and what do we think is right and what do we think is wrong, but they are much more questions at the level of what is it about our evolutionary pathway that led us to thinking that these things are right or wrong.
What is it about society and the pressures that you're gone and faced that led us to things where murder is wrong in almost every society in the world. I will say the death penalty is the thing, it's just the type of sanctioned murder. So there is a sense in which I think it's a bit more nuanced than just that. And there's something to be said about like I guess if I had to make my claims, like what I think has sort of happened there.
So there's something about us as creatures that evolved to coordinate and perform well in groups and pressures that, that placed on us that caused us to develop these normative systems whereby we say different things are right and wrong.
Lucas: Iterated game theory over millions of years or something.
Dylan: Something like that. Yeah, but there's a sense in which us labeling things as right and wrong and developing the processes whereby we label things as right and wrong is a thing that we've been pushed towards.
Lucas: From my perspective, it feels like this is more tractable than people lead on, like AI is only going to be able to help in moral deliberation, once it's general. It already helps us in regular deliberation and moral deliberation isn't a special kind of deliberation and moral deliberation requires empirical facts about the world and in persons just like any other kind of actionable deliberation does and domains that aren't considered to have to do with moral philosophy or ethics or things like that.
So I'm not an AI researcher, but it seems to me like this is more attractable than people lead onto be. The normative aspect of AI Alignment seems to be under researched.
Dylan: Can you say a little more about what you mean by that?
Lucas: What I meant was the normative deliberative process, the difficulty in coming to normative conclusions and what the appropriate epistemic and deliberative process is for arriving at normative solutions and how narrow AI systems can take us to a beautiful world where advanced AI systems actually lead us to post human ethics.
If we ever want to get to a place where general systems take us to post human ethics, why not start today with figuring out how narrow systems can work to amplify human moral decision making and deliberative processes.
Dylan: I think the hard part there is, I don't exactly know what it means to amplify those processes. My perspective is that we as a species do not yet have a good understanding of what those deliberative processes actually represent and what formed the result actually does.
Lucas: It's just like giving more information, providing tons of data, analyzing the data, potentially pointing out biases. The part where they're literally amplifying cognitive implicit or explicit decision making process is more complicated and will require more advancement and cognition and deliberation and stuff. But yeah, I still think there are more mundane ways in which it can make us better moral reasoners and decision makers.
If I could give you like 10,000 more bits of information every day about moral decisions that you make, you would probably just be a better moral agent.
Dylan: Yes, one way to try to think about that is maybe things like VR approaches to increasing empathy. I think that that has a lot of power to make us better.
Lucas: Max always says that there's a race between wisdom and the power of our technology and it seems like people really aren't taking seriously ways in which we can amplify wisdom because wisdom is generally taken to be part of the humanities and like the soft sciences. Maybe we should be taking more seriously ways in which narrow current day AI systems can be used to amplify the progress at which the human species makes wisdom. Because otherwise we're just gonna like continue how we always continue and the wisdom is going to go really slow and then we're going to probably learn from a bunch of mistakes.
And it's just not going to be as good until we'll develop a rigorous science of making moral progress or like using technology to amplify the progress of wisdom and moral progress.
Dylan: So in principle, what you're saying, I don't really disagree with it, but I also don't know how that would change what I'm working on either. In the sense that I'm not sure what it would mean. I do not know how I would do research on amplifying wisdom. I just don't really know what that means. And that's not to say it's an impossible problem, we talked earlier about how I don't know what partial value alignment means, that something that you and I can talk about it and we can intuitively I think align on a concept, but it's not a concept I knew how to translate into actionable concrete research problems right now.
In the same way, the idea of amplifying wisdom and making people more wise is something that I think intuitively I understand what you mean, but when I try to think about how an AI system would make someone wiser, that feels difficult.
Lucas: It can seem difficult, but I feel like it would, obviously this is like an open research question, but if you were able to identify a bunch of variables that are most important for moral decision making and then if you could use AI systems to sort of gather aggregate and compile in certain ways and analyze moral information in this way, again, it just seems more tractable than people seem to be letting on.
Dylan: Yeah, although I wonder now is that different from value alignment does, we're thinking about it, right? Concrete research thing I spend a while thinking about is, how do you identify the features that a person considers to be valuable? Say, we don't know the relative tradeoffs between them.
One way you might try to solve value alignment is have a process that identifies the features that might matter in the world and then have a second process that identifies the appropriate tradeoffs between those features, and maybe something about diminishing returns or something like that. And that to me sounds like I just placed values with wisdom and I've got sort of what you're thinking about. I think both of those terms are similarly diffuse. I wonder if what we're talking about is semantics, and if it's not, I'd like to know what the difference is.
Lucas: I guess, the more mundane definition of wisdom, at least in the way that Max Tegmark would use it would be like the ways in which we use our technology. I might have specific preferences, but just because I have specific preferences that I may or may not be aligning an AI system to does not necessarily mean that that total process, this like CIRL process is actually an expression of wisdom.
Dylan: Okay, can you provide a positive description of what a process would look like? Or like basically what I'm saying is I can hear the point of I have preferences and I aligned my system to it and that's not necessarily a wise system and ...
Lucas: Yeah, like I build a fire because I want to be hot, but then the fire catches my village on fire and no longer is ... That's still might be value alignment.
Dylan: But isn't [crosstalk 00:48:39] some values that you didn't take into account when you were deciding to build the fire.
Lucas: Yeah, that's right. So I don't know. I'd probably have to think about this more because I guess this is something that I just sort of throwing out right now as a reaction to what we've been talking about. So I don't have a very good theory of it.
Dylan: And I don't wanna say that you need to know the right answers to these things to not have that be a useful direction to push people.
Lucas: We don't want to use different concepts to just reframe the same problem and just make a conceptual mess.
Dylan: That's what I'm a little bit concerned about and that's the thing I'm concerned about broadly. We've got a lot of issues that we're thinking about in dealing with that we're not really sure what they are.
For me, I think one of the really helpful things has been to frame the issue that I'm thinking about as if a person has a behavior that they want to implement into the world and that's a complex behavior that they don't know how to identify immediately. How do you actually go about building systems that allow you to implement that behavior effectively, evaluate that the behavior is actually been correctly implemented.
Lucas: Avoiding side effects, avoiding ...
Dylan: Like all of these kinds of things that we sort of concerned about in AI Safety, in my mind fall a bit more into place when we frame the problem as I have a desired behavior that I want to exist, a response function, a policy function that I want to implement into the world. What are the technological systems I can use to implement that in a computer or a robot or what have you.
Lucas: Okay. Well, do you have anything else you'd like to wrap up on?
Dylan: No, I just, I want to say thanks for asking hard questions and making me feel uncomfortable because I think it's important to do a lot of that as a scientist and in particular I think as people working on AI, we should be spending a bit more time being uncomfortable and talking about these things, because it does impact what we end up doing and it does I think impact the trajectories that we put the technology on.
Lucas: Wonderful. So if people want to read about cooperative inverse reinforcement learning, where can we find the paper or other work that you have on that? What do you think are the best resources? What are just general things you'd like to point people towards in order to follow you or keep up to date with AI Alignment?
Dylan: I tweet occasionally about AI Alignment and a bit of AI ethics questions, the Hadfield-Menell, my first initial, last name. And if you're interested in getting a technical introduction to value alignment, I would say take a look at the 2016 paper on cooperative IRL. If you'd like a more general introduction, there's a blog post from summer 2017 on the bear blog.
Lucas: All right, thanks so much Dylan, and maybe we'll be sitting in a similar room again in two years for Beneficial Artificial Super Intelligence 2021.
Dylan: I look forward to it. Thanks a bunch.
Lucas: Thanks. See you, Dylan. If you enjoyed this podcast, please subscribe, give it a like, or share it on your preferred social media platform. We'll be back again soon with another episode in the AI Alignment series.
Related episodes
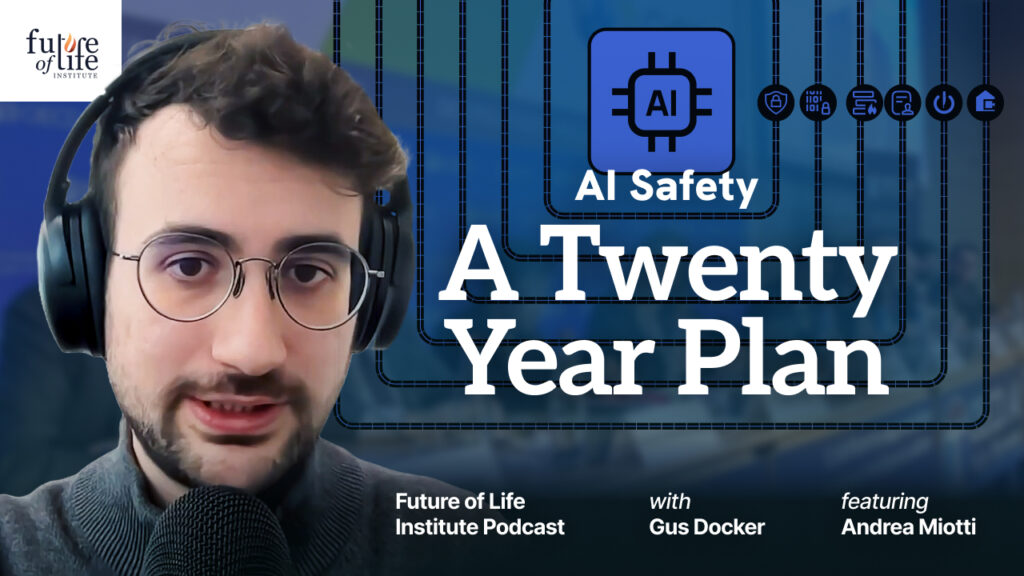
Andrea Miotti on a Narrow Path to Safe, Transformative AI
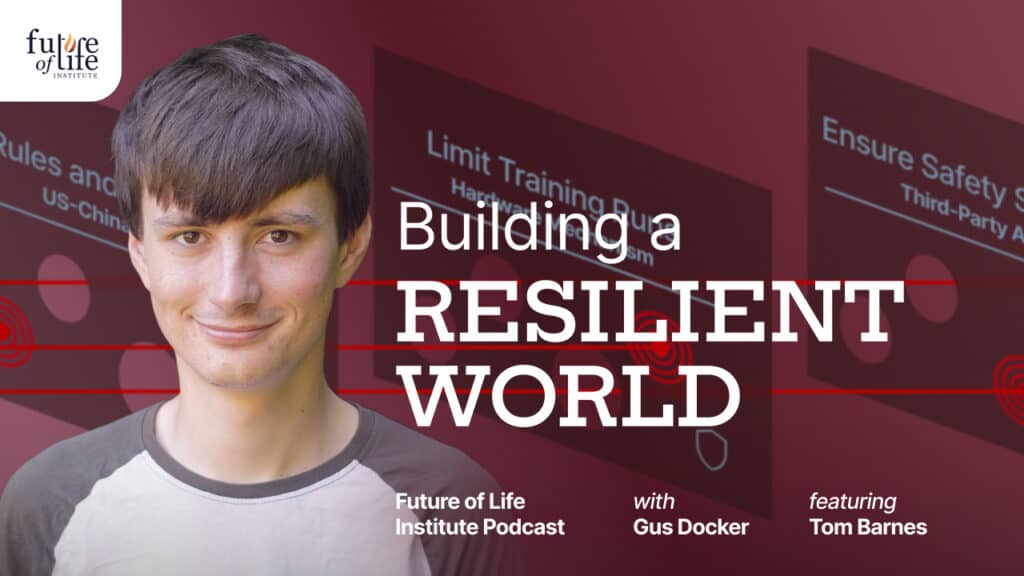