AI Alignment Podcast: China’s AI Superpower Dream with Jeffrey Ding
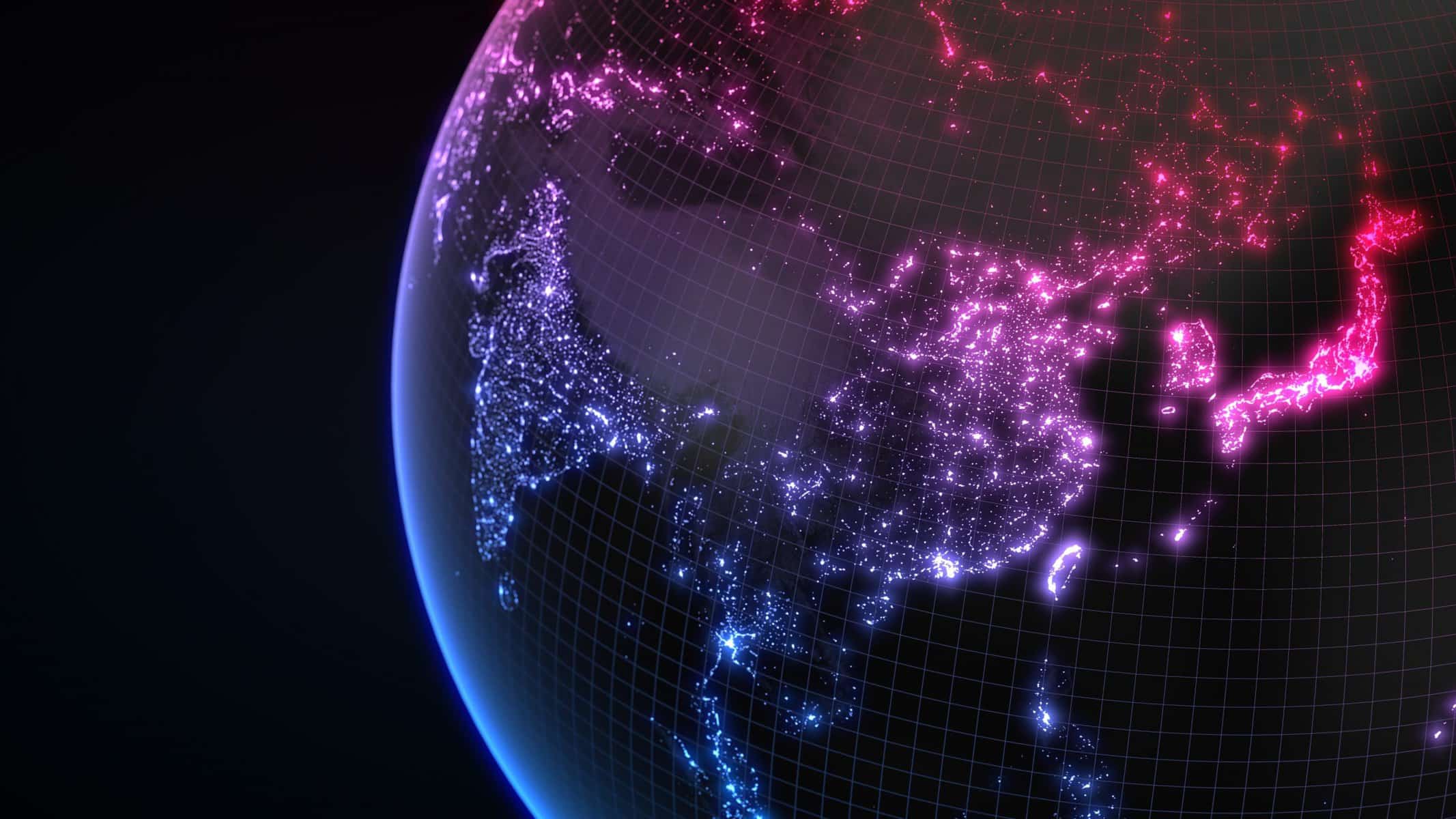
"In July 2017, The State Council of China released the New Generation Artificial Intelligence Development Plan. This policy outlines China’s strategy to build a domestic AI industry worth nearly US$150 billion in the next few years and to become the leading AI power by 2030. This officially marked the development of the AI sector as a national priority and it was included in President Xi Jinping’s grand vision for China." (FLI's AI Policy - China page) In the context of these developments and an increase in conversations regarding AI and China, Lucas spoke with Jeffrey Ding from the Center for the Governance of AI (GovAI). Jeffrey is the China lead for GovAI where he researches China's AI development and strategy, as well as China's approach to strategic technologies more generally.
Topics discussed in this episode include:
- China's historical relationships with technology development
- China's AI goals and some recently released principles
- Jeffrey Ding's work, Deciphering China's AI Dream
- The central drivers of AI and the resulting Chinese AI strategy
- Chinese AI capabilities
- AGI and superintelligence awareness and thinking in China
- Dispelling AI myths, promoting appropriate memes
- What healthy competition between the US and China might look like
You can take a short (3 minute) survey to share your feedback about the podcast here.
Key points from Jeffrey:
- "Even if you don't think Chinese AI capabilities are as strong as have been hyped up in the media and elsewhere, important actors will treat China as either a bogeyman figure or as a Sputnik type of wake-up call motivator... other key actors will leverage that as a narrative, as a Sputnik moment of sorts to justify whatever policies they want to do. So we want to understand what's happening and how the conversation around what's happening in China's AI development is unfolding."
- "There certainly are differences, but we don't want to exaggerate them. I think oftentimes analysis of China happens in a vacuum where it's like, 'Oh, this only happens in this mysterious far off land, we call China and it doesn't happen anywhere else.' Shoshana Zuboff has this great book on Surveillance Capitalism that shows how the violation of privacy is pretty extensive on the US side, not only from big companies but also from the national security apparatus. So I think a similar phenomenon is taking place with the social credit system. Jeremy Dom at Yale laws China Center has put it really nicely where he says that, 'We often project our worst fears about technology in AI onto what's happening in China, and we look through a glass darkly and we unleash all of our anxieties on what's happening on to China without reflecting on what's happening here in the US, what's happening here in the UK.'"
- "I think we have to be careful about which historical analogies and memes we choose. So 'arms race' is a very specific call back to cold war context, where there's almost these discrete types of missiles that we are racing Soviet Union on and discrete applications that we can count up; Or even going way back to what some scholars call the first industrial arms race in the military sphere over steam power boats between Britain and France in the late 19th century. And all of those instances you can count up. France has four iron clads, UK has four iron clads; They're racing to see who can build more. I don't think there's anything like that. There's not this discreet thing that we’re racing to see who can have more of. If anything, it's about a competition to see who can absorb AI advances from abroad better, who can diffuse them throughout the economy, who can adopt them in a more sustainable way without sacrificing core values. So that's sort of one meme that I really want to dispel. Related to that, assumptions that often influence a lot of our discourse on this is techno-nationalist assumption, which is this idea that technology is contained within national boundaries and that the nation state is the most important actor –– which is correct and a good one to have and a lot of instances. But there are also good reasons to adopt techno-globalist assumptions as well, especially in the area of how fast technologies diffuse nowadays and also how much underneath this national level competition, firms from different countries are working together and make standards alliances with each other. So there's this undercurrent of techno-globalism, where there are people flows, idea flows, company flows happening while the coverage and the sexy topic is always going to be about national level competition, zero sum competition, relative games rhetoric. So you're trying to find a balance between those two streams."
- "I think currently a lot of people in the US are locked into this mindset that the only two players that exist in the world are the US and China. And if you look at our conversation, right, oftentimes I've displayed that bias as well. We should probably have talked a lot more about China-EU or China-Japan corporations in this space and networks in this space because there's a lot happening there too. So a lot of US policy makers see this as a two-player game between the US and China. And then in that sense, if there's some cancer research project about discovering proteins using AI that may benefit China by 10 points and benefit the US only by eight points, but it's going to save a lot of people from cancer –– if you only care about making everything about maintaining a lead over China, then you might not take that deal. But if you think about it from the broader landscape of it's not just a zero sum competition between US and China, then your kind of evaluation of those different point structures and what you think is rational will change."
Important timestamps:
0:00 intro
2:14 Motivations for the conversation
5:44 Historical background on China and AI
8:13 AI principles in China and the US
16:20 Jeffrey Ding’s work, Deciphering China’s AI Dream
21:55 Does China’s government play a central hand in setting regulations?
23:25 Can Chinese implementation of regulations and standards move faster than in the US? Is China buying shares in companies to have decision making power?
27:05 The components and drivers of AI in China and how they affect Chinese AI strategy
35:30 Chinese government guidance funds for AI development
37:30 Analyzing China’s AI capabilities
44:20 Implications for the future of AI and AI strategy given the current state of the world
49:30 How important are AGI and superintelligence concerns in China?
52:30 Are there explicit technical AI research programs in China for AGI?
53:40 Dispelling AI myths and promoting appropriate memes
56:10 Relative and absolute gains in international politics
59:11 On Peter Thiel’s recent comments on superintelligence, AI, and China
1:04:10 Major updates and changes since Jeffrey wrote Deciphering China’s AI Dream
1:05:50 What does healthy competition between China and the US look like?
1:11:05 Where to follow Jeffrey and read more of his work
Works referenced
We hope that you will continue to join in the conversations by following us or subscribing to our podcasts on Youtube, Spotify, SoundCloud, iTunes, Google Play, Stitcher, iHeartRadio, or your preferred podcast site/application. You can find all the AI Alignment Podcasts here.
Transcript
Lucas Perry: Hello everyone and welcome back to the AI Alignment Podcast at The Future of Life Institute. I'm Lucas Perry and today we'll be speaking with Jeffrey Ding from The Future of Humanity Institute on China and their efforts to be the leading AI Superpower by 2030. In this podcast, we provide a largely descriptive account of China's historical technological efforts, their current intentions and methods for pushing Chinese AI Success, some of the foundational AI principles being called for within China; We cover the drivers of AI progress, the components of success, China's strategies born of these variables; We also assess China's current and likely future AI capabilities, and the consequences of all this tied together. The FLI AI Policy China page, and Jeffrey Ding's publication Deciphering China's AI Dream are large drivers of this conversation, and I recommend you check them out.
If you find this podcast interesting or useful, consider sharing it with friends on social media platforms, forums, or anywhere you think it might be found valuable. As always, you can provide feedback for me by following the SurveyMonkey link found in the description of wherever you might find this podcast.
Jeffrey Ding specializes in AI strategy and China's approach to strategic technologies more generally. He is the China lead for the Center for the Governance of AI. There, Jeff researches China's development of AI and his work has been cited in the Washington Post, South China Morning Post, MIT Technological Review, Bloomberg News, Quartz, and other outlets. He is a fluent Mandarin speaker and has worked at the US Department of State and the Hong Kong Legislative Council. He is also reading for a PhD in international relations as a Rhodes scholar at the University of Oxford. And so without further ado, let's jump into our conversation with Jeffrey Ding.
Let's go ahead and start off by providing a bit of the motivations for this conversation today. So why is it that China is important for AI alignment? Why should we be having this conversation? Why are people worried about the US-China AI Dynamic?
Jeffrey Ding: Two main reasons, and I think they follow an “even if” structure. The first reason is China is probably second only to the US in terms of a comprehensive national AI capabilities measurement. That's a very hard and abstract thing to measure. But if you're taking which countries have the firms on the leading edge of the technology, the universities, the research labs, and then the scale to lead in industrial terms and also in potential investment in projects related to artificial general intelligence. I would put China second only to the US, at least in terms of my intuition and sort of my analysis that I've done on the subject.
The second reason is even if you don't think Chinese AI capabilities are as strong as have been hyped up in the media and elsewhere, important actors will treat China as either a bogeyman figure or as a Sputnik type of wake-up call motivator. And you can see this in the rhetoric coming from the US especially today, and even in areas that aren't necessarily connected. So Axios had a leaked memo from the US National Security Council that was talking about centralizing US telecommunication services to prepare for 5G. And in the memo, one of the justifications for this was because China is leading in AI advances. The memo doesn't really tie the two together. There are connections –– 5G may empower different AI technologies –– but that's a clear example of how even if Chinese capabilities in AI, especially in projects related to AGI, are not as substantial as has been reported, or we think, other key actors will leverage that as a narrative, as a Sputnik moment of sorts to justify whatever policies they want to do. So we want to understand what's happening and how the conversation around what's happening in China's AI development is unfolding.
Lucas Perry: So the first aspect being that they're basically the second most powerful AI developer. And we can get into later their relative strength to the US; I think that in your estimation, they have about half as much AI capability relative to the United States. And here, the second one is you're saying –– and there's this common meme in AI Alignment about how avoiding races is important because in races, actors have incentives to cut corners in order to gain decisive strategic advantage by being the first to deploy advanced forms of artificial intelligence –– so there's this important need, you're saying, for actually understanding the relationship and state of Chinese AI Development to dispel inflammatory race narratives?
Jeffrey Ding: Yeah, I would say China's probably at the center of most race narratives when we talk about AI arms races and the conversation in at least US policy-making circles –– which is what I follow most, US national security circles –– has not talked necessarily about AI as a decisive strategic advantage in terms of artificial general intelligence, but definitely in terms of decisive strategic advantage and who has more productive power, military power. So yeah, I would agree with that.
Lucas Perry: All right, so let's provide a little bit more historical background here, I think, to sort of contextualize why there's this rising conversation about the role of China in the AI space. So I'm taking this here from the FLI AI Policy China page: “In July of 2017, the State Council of China released the New Generation Artificial Intelligence Development Plan. And this was an AI research strategy policy to build a domestic AI industry worth nearly $150 billion in the next few years” –– again, this was in 2017 –– “and to become a leading AI power by 2030. This officially marked the development of the AI sector as a national priority, and it was included in President Xi Jinping's grand vision for China.” And just adding a little bit more color here: “given this, the government expects its companies and research facilities to be at the same level as leading countries like the United States by 2020.” So within a year from now –– maybe a bit ambitious, given your estimation that they have is about half as much capability as us.
But continuing this picture I'm painting: “five years later, it calls for breakthroughs in select disciplines within AI” –– so that would be by 2025. “That will become a key impetus for economic transformation. And then in the final stage, by 2030, China is intending to become the world's premier artificial intelligence innovation center, which will in turn foster a new national leadership and establish the key fundamentals for an economic great power,” in their words. So there's this very clear, intentional stance that China has been developing in the past few years.
Jeffrey Ding: Yeah, definitely. And I think it was Jess Newman who put together the AI policy in China page –– did a great job. It's a good summary of this New Generation AI Development Plan issued in July 2017 and I would say the plan was more reflective of momentum that was already happening at the local level with companies like Baidu, Tencent, Alibaba, making the shift to focus on AI as a core part of their business strategy. Shenzhen, other cities, had already set up their own local funds and plans, and this was an instance of the Chinese national government, in the words of I think Paul Triolo and some other folks at New America, “riding the wave,” and kind of joining this wave of AI development.
Lucas Perry: And so adding a bit more color here again: there's also been developments in principles that are being espoused in this context. I'd say probably the first major principles on AI were developed at the Asilomar Conference, at least those pertaining to AGI. In June 2019, the New Generation of AI Governance Expert Committee released principles for next-generation artificial intelligence governance, which included tenants like harmony and friendliness and fairness and justice, inclusiveness and sharing, open cooperation, shared responsibility, and agile governance.
And then also in May of 2019 the Beijing AI Principles were released. That was by a multi-stakeholder coalition, including the Beijing Academy of Artificial Intelligence, a bunch of top universities in China, as well as industrial firms such as Baidu, Alibaba, and Tencent. And these 15 principles, among other things, called for "the construction of a human community with a shared future and the realization of beneficial AI for humankind in nature." So it seems like principles and intentions are also being developed similarly in China that sort of echo and reflect many of the principles and intentions that have been developing in the states.
Jeffrey Ding: Yeah, I think there's definitely a lot of similarities, and I think it's not just with this recent flurry of AI ethics documents that you've done a good job of summarizing. It dates back to even the plan that we were just talking about. If you read the July 2017 New Generation AI Plan carefully, there's a lot of sections devoted to AI ethics, including some sections that are worried about human robot alienation.
So, depending on how you read that, you could read that as already anticipating some of the issues that could occur if human goals and AI goals do not align. Even back in March, I believe, of 2018, a lot of government bodies came together with companies to put out a white paper on AI standardization, which I translated for New America. And in that, they talk about AI safety and security issues, how it's important to ensure that the design goals of AI are consistent with the interests, ethics, and morals of most humans. So a lot of these topics, I don't even know if they're western topics. These are just basic concepts: We want systems to be controllable and reliable. And yes, those have deeper meanings in the sense of AGI, but that doesn't mean that some of these initial core values can't be really easily applied to some of these deeper meanings that we talk about when we talk about AGI ethics.
Lucas Perry: So with all of the animosity and posturing and whatever that happens between the United States and China, these sort of principles and intentions which are being developed, at least in terms of AI –– both of them sort of have international intentions for the common good of humanity; At least that's what is being stated in these documents. How do you think about the reality of the day-to-day combativeness and competition between the US and China in relation to these principles which strive towards the deployment of AI for the common good of humanity more broadly, rather than just within the context of one country?
Jeffrey Ding: It's a really good question. I think the first point to clarify is these statements don't have teeth behind them unless they're enforced, unless there's resources dedicated to funding research on these issues, to track 1.5, track 2 diplomacy, technical meetings between researchers. These are just statements that people can put out and they don't have teeth unless they're actually enforced. Oftentimes, we know it's the case. Firms like Google and Microsoft, Amazon, will put out principles about facial recognition or what their ethical stances are, but behind the scenes they'll chase profit motives and maximize shareholder value. And I would say the same would take place for Tencent, Baidu, Alibaba. So I want to clarify that, first of all. The competitive dynamics are real: It's partly not just an AI story, it's a broader story of China's rise. I've come from international relations background, so I'm a PhD student at Oxford studying that, and there's a big debate in the literature about what happens when a rising power challenges an established power. And oftentimes frictions result, and it's about how to manage these frictions without leading to accidents, miscalculation, arms races. And that's the tough part of it.
Lucas Perry: So it seems –– at least for a baseline, thinking that we're still pretty early in the process of AI alignment or this long-term vision we have –– it seems like at least there is theoretically some shared foundational principles reflective across both the cultures. Again, these Beijing AI Principles also include focus on benefiting all of humanity and the environment; serving human values such as privacy, dignity, freedom, autonomy and rights; continuous focus on AI safety and security; inclusivity, openness; supporting international cooperation; and avoiding a malicious AI race. So the question now simply seems: implementation of these shared principles, ensuring that they manifest.
Jeffrey Ding: Yeah. I don't mean to be dismissive of these efforts to create principles that were at least expressing the rhetoric of planning for all of humanity. I think there's definitely a lot of areas of US-China cooperation in the past that have also echoed some of these principles: bi-lateral cooperation on climate change research; there's a good nuclear safety cooperation module; different centers that we've worked on. But at the same time, I also think that even with that list of terms you just mentioned, there are some differences in terms of how both sides understand different terms.
So with privacy in the Chinese context, it's not necessarily that Chinese people or political actors don't care about privacy. It's that privacy might mean more of privacy as an instrumental right, to ensure your financial data doesn't get leaked, you don't lose all your money; to ensure that your consumer data is protected from companies; but not necessarily in other contexts where privacy is seen as an intrinsic right, as a civil right of sorts, where it's also about an individual's protection from government surveillance. That type of protection is not caught up in conversations about privacy in China as much.
Lucas Perry: Right, so there are going to be implicitly different understandings about some of these principles that we’ll have to navigate. And again, you brought up privacy as something –– and this has been something people have been paying more attention to, as there has been kind of this hype and maybe a little bit of hysteria over the China social crediting system, and plenty of misunderstanding around that.
Jeffrey Ding: Yeah, and this ties into a lot of what I've been thinking about lately, which is there certainly are differences, but we don't want to exaggerate them. I think oftentimes analysis of China happens in a vacuum where it's like, "Oh, this only happens in this mysterious far off land we call China and it doesn't happen anywhere else." Shoshana Zuboff has this great book on surveillance capitalism that shows how the violation of privacy is pretty extensive on the US side, not only from big companies but also from the national security apparatus.
So I think a similar phenomenon is taking place with the social credit system. Jeremy Dom at Yale Law’s China Center has put it really nicely where he says that, "We often project our worst fears about technology in AI onto what's happening in China, and we look through a glass darkly and we unleash all of our anxieties on what's happening onto China without reflecting on what's happening here in the US, what's happening here in the UK."
Lucas Perry: Right. I would guess that generally in human psychology it seems easier to see the evil in the other rather than in the self.
Jeffrey Ding: Yeah, that's a little bit out of range for me, but I'm sure there's studies on that.
Lucas Perry: Yeah. All right, so let's get in here now to your work on deciphering China's AI dream. This is a work that you'd published in 2018 and in this work you divided up into these four different sections. First you work on context, then you discuss components, then you discuss capabilities, and then you discuss consequences all in relation to AI in China. Would you like to just sort of unpack the structuring?
Jeffrey Ding: Yeah, this was very much just a descriptive paper. I was just starting out researching this area and I just had a bunch of basic questions. So question number one for context: what is the background behind China's AI Strategy? How does it compare to other countries’ plans? How does it compare to its own past science and technology plans? The second question was, what are they doing in terms of pushing forward drivers of AI Development? So that's the component section. The third question is, how well are they doing? It's about assessing China's AI capabilities. And then the fourth is, so what's it all mean? Why does it matter? And that's where I talk about the consequences and the potential implications of China's AI ambitions for issues related to AI Safety, some of the AGI issues we've been talking about, national security, economic development, and social governance.
Lucas Perry: So let's go ahead and move sequentially through these. We've already here discussed a bit of context about what's going on in China in terms of at least the intentional stance and the development of some principles. Are there any other key facets or areas here that you'd like to add about China's AI strategy in terms of its past science and technology? Just to paint a picture for our listeners.
Jeffrey Ding: Yeah, definitely. I think two past critical technologies that you could look at are the plans to increase China's space industry, aerospace sector; and then also biotechnology. So in each of these other areas there was also a national level strategic plan; An agency or an office was set up to manage this national plan; Substantial funding was dedicated. With the New Generation AI Plan, there was also a sort of implementation office set up across a bunch of the different departments tasked with implementing the plan.
AI was also elevated to the level of a national strategic technology. And so what's different between these two phases? Because it's debatable how successful the space plan and the biotech plans have been. What's different with AI is you already had big tech giants who are pursuing AI capabilities and have the resources to shift a lot of their investments toward the AI space, independent of government funding mechanisms: companies like Baidu, Tencent, Alibaba, even startups that have really risen like SenseTime. And you see that reflected in the type of model.
It's no longer the traditional national champion model where the government almost builds a company from the ground up, maybe with the help of like international financers and investors. Now it's a national team model where they ask for the support of these leading tech giants, but it's not like these tech giants are reliant on the government for subsidies or funding to survive. They are already flourishing firms that have international presence.
The other bit of context I would just add is that if you look at the New Generation Plan, there's a lot of terms that are related to manufacturing. And I mentioned in Deciphering China's AI Dream, how there's a lot of connections and callbacks to manufacturing plans. And I think this is key because it's one aspect of China's strive for AI as they want to escape the middle income trap and kind of get to those higher levels of value-add in the manufacturing chain. So I want to stress that as a key point of context.
Lucas Perry: So the framing here is the Chinese government is trying to enable companies which already exist and already are successful. And this stands in contrast to the US and the UK where it seems like the government isn't even part of a teamwork effort.
Jeffrey Ding: Yeah. So maybe a good comparison would be how technical standards develop, which is an emphasis of not only this deciphering China dream paper but a lot of later work. So I'm talking about technical standards, like how do you measure the accuracy of facial recognition systems and who gets to set those measures, or product safety standards for different AI applications. And in many other countries, including the US, the process for that is much more decentralized. It's largely done through industry alliances. There is the NIST, which is a body under the Department of Commerce in the US that helps coordinate that to some extent, but not nearly as much as what happens in China with the Standards Administration Commission (SAC), I believe. There, it's much more of a centralized effort to create technical standards. And there are pros and cons to both.
With the more decentralized approach, you minimize the risks of technological lock-in by setting standards too early, and you let firms have a little bit more freedom, competition as well. Whereas having a more centralized top-down effort might lead to earlier harmonization on standards and let you leverage economies of scale when you just have more interoperable protocols. That could help with data sharing, help with creating stable test bed for different firms to compete and measure stuff I was talking about earlier, like algorithmic accuracy. So there are pros and cons of the two different approaches. But I think yeah, that does flush out how the relationship between firms and the government differs a little bit, at least in the context of standards setting.
Lucas Perry: So on top of standards setting, would you say China's government plays more of a central hand in the regulation as well?
Jeffrey Ding: That's a good question. It probably differs in terms of what area of regulation. So I think in some cases there's a willingness to let companies experiment and then put down regulations afterward. So this is the classic example with mobile payments: There was definitely a gray space as to how these platforms like Alipay, WeChat Pay were essentially pushing into a gray area of law in terms of who could handle this much money that's traditionally in the hands of the banks. Instead of clamping down on it right away, the Chinese government kind of let that play itself out, and then once these mobile pay platforms got big enough that they're holding so much capital and have so much influence on the monetary stock, they then started drafting regulations for them to be almost treated as banks. So that's an example of where it's more of a hands-off approach.
In AI, folks have said that the US and China are probably closer in terms of their approach to regulation, which is much more hands-off than the EU. And I think that's just a product partly of the structural differences in the AI ecosystem. The EU has very few big internet giants and AI algorithm firms, so they have more of an incentive to regulate other countries’ big tech giants and AI firms.
Lucas Perry: So two questions are coming up. One is, is there sufficiently more unity and coordination in the Chinese government such that when standards and regulations, or decisions surrounding AI, need to be implemented that they're able to move, say, much quicker than the United States government? And the second thing was, I believe you mentioned also that the Chinese government is also trying to find ways of using potential government money for buying up shares in these companies and try to gain decision making power.
Jeffrey Ding: Yeah, I'll start with the latter. The reference is to the establishment of special management shares: so these would be almost symbolic, less than 1% shares in a company so that they could maybe get a seat on the board –– or another vehicle is through the establishment of party committees within companies, so there's always a tie to party leadership. I don't have that much more insight into how these work. I think probably it's fair to say that the day-to-day and long-term planning decisions of a lot of these companies are mostly just driven by what their leadership wants, not necessarily what the party leaders want, because it's just very hard to micromanage these billion dollar giants.
And that was part of a lot of what was happening with the reform of the state-owned enterprise sector, where, I think it was the SAC –– there are a lot of acronyms –– but this was the body in control of state-owned enterprises and they significantly cut down the number of enterprises that they directly oversee and sort of focused on the big ones, like the big banks or the big oil companies.
To your first point on how smooth policy enforcement is, this is not something I've studied that carefully. I think to some extent there's more variability in terms of what the government does. So I read somewhere that if you look at the government relations departments of Chinese big tech companies versus US big tech companies, there's just a lot more on the Chinese side –– although that might be changing with recent developments in the US. Two cases I'm thinking of right now are the Chinese government worrying about addictive games and then issuing the ban against some games including Tencent’s PUBG, which has wrecked Tencent’s game revenues and was really hurtful for their stock value.
So that's something that would be very hard for the US government to be like, "Hey, this game is banned." At the same time, there's a lot of messiness with this, which is why I'm pontificating and equivocating and not really giving you a stable answer, because local governments don't implement things that well. There's a lot of local center attention. And especially with technical stuff –– this is the case of the US as well –– there's just not as much technical talent in the government. So with a lot of these technical privacy issues, it's very hard to develop good regulations if you don't actually understand the tech. So what they've been trying to do is audit privacy policies of different social media tech companies and they started with 10 of the biggest and have tried to audit them. So I think it's very much a developing process in both China and the US.
Lucas Perry: So you're saying that the Chinese government, like the US, lacks much scientific or technical expertise? I had some sort of idea in my head that many of the Chinese mayors or other political figures actually have engineering degrees or degrees in science.
Jeffrey Ding: That's definitely true. But I mean, by technical expertise I mean something like what the US government did with the digital service corps, where they're getting people who have worked in the leading edge tech firms to then work for the government. That type of stuff would be useful in China.
Lucas Perry: So let's move on to the second part, discussing components. And here you relate the key features of China's AI strategy to the drivers of AI development, and here the drivers of AI development you say are hardware in the form of chips for training and executing AI algorithms, data as an input for AI Algorithms, research and algorithm development –– so actual AI researchers working on the architectures and systems through which the data will be put, and then the commercial AI ecosystems, which I suppose support and feed these first three things. What can you say about the state of these components in China and how it affects China's AI strategy?
Jeffrey Ding: I think the main thing that I want to emphasize here that a lot of this is the Chinese government is trying to fill in some of the gaps, a lot of this is about enabling people, firms that are already doing the work. One of the gaps is private firms tend to under-invest in basic research or will under-invest in broader education because they don't get a capture all those gains. So the government tries to support not only AI as a national level discipline but also to construct AI institutes, help fund talent programs to bring back the leading researchers from overseas. So that's one part of it.
The second part of it, which I did not talk about that much in the report in this section but I've recently researched more and more about, is that where the government is more actively driving things is when they are the final end client. So this is definitely the case in the surveillance industry space: provincial-level public security bureaus are working with companies in both hardware, data, research and development and the whole security systems integration process to develop more advanced high tech surveillance systems.
Lucas Perry: Expanding here, there's also this way of understanding Chinese AI strategy as it relates to previous technologies and how it's similar or different. Ways in which it's similar involve strong degree of state support and intervention, transfer of both technology and talent, and investment in long-term whole-of-society measures; I'm quoting you here.
Jeffrey Ding: Yeah.
Lucas Perry: Furthermore, you state that China is adopting a catch-up approach in the hardware necessary to train and execute AI algorithms. This points towards an asymmetry, that most of the chip manufacturers are not in China and they have to buy them from Nvidia. And then you go on to mention about how access to large quantities of data is an important driver for AI systems and that China's data protectionism favors Chinese AI companies and accessing data from China's large domestic market, but it also detracts from cross-border pooling of data.
Jeffrey Ding: Yeah, and just to expand on that point, there's been good research out of folks at DigiChina, which is a New America Institute, that looks at the cybersecurity law –– and we're still figuring out how that's going to be implemented completely, but the original draft would have prevented companies from taking data that was collected inside of China and taking it outside of China.
And actually these folks at DigiChina point out how some of the major backlash to this law didn't just come from US multinational incorporations but also Chinese multinationals. That aspect of data protectionism illustrates a key trade-off: on one sense, countries and national security players are valuing personal data almost as a national security asset for the risk of blackmail or something. So this is the whole Grindr case in the US where I think Grindr was encouraged or strongly encouraged by the US government to find a non-Chinese owner. So that's on one aspect you want to protect personal information, but on the other hand, free data flows are critical to spurring gains and innovation as well for some of these larger companies.
Lucas Perry: Is there an interest here to be able to sell their data to other companies abroad? Is that why they're against this data protectionism in China?
Jeffrey Ding: I don't know that much about this particular case, but I think Alibaba and Tencent have labs all around the world. So they might want to collate their data together, so they were worried that the cybersecurity law would affect that.
Lucas Perry: And just highlighting here for the listeners that access to large amounts of high quality data is extremely important for efficaciously training models and machine learning systems. Data is a new, very valuable resource. And so you go on here to say, I'm quoting you again, "China's also actively recruiting and cultivating talented researchers to develop AI algorithms. The state council’s AI plan outlines a two pronged gathering and training approach." This seems to be very important, but it also seems like from your report that China's losing AI talent to America largely. What can you say about this?
Jeffrey Ding: Often the biggest bottleneck cited to AI development is lack of technical talent. That gap will eventually be filled just based on pure operations in the market, but in the meantime there has been a focus on AI talent, whether that's through some of these national talent programs, or it also happens through things like local governments offering tax breaks for companies who may have headquarters around the world.
For example, Jingchi which is an autonomous driving startup, they had I think their main base in California or one of their main bases in California; But then Shenzhen or Guangzhou, I'm not sure which local government it was, they gave them basically free office space to move one of their bases back to China and that brings a lot of talented people back. And you're right, a lot of the best and brightest do go to US companies as well, and one of the key channels for recruiting Chinese students are big firms setting up offshore research and development labs like Microsoft Research Asia in Beijing.
And then the third thing I'll point out, and this is something I've noticed recently when I was doing translations from science and tech media platforms that are looking at the talent space in particular: They've pointed out that there's sometimes a tension between the gathering and the training planks. So there've been complaints from domestic Chinese researchers, so maybe you have two super talented PhD students. One decides to stay in China, the other decides to go abroad for their post-doc. And oftentimes the talent plans –– the recruiting, gathering plank of this talent policy –– will then favor the person who went abroad for the post-doc experience over the person who stayed in China, and they might be just as good. So then that actually creates an incentive for more people to go abroad. There's been good research that a lot of the best and brightest ended up staying abroad; The stay rates, especially in the US for Chinese PhD students in computer science fields, are shockingly high.
Lucas Perry: What can you say about Chinese PhD student anxieties with regards to leaving the United States to go visit family in China and come back? I've heard that there may be anxieties about not being let back in given that their research has focused on AI and that there's been increasing US suspicions of spying or whatever.
Jeffrey Ding: I don't know how much of it is a recent development but I think it's just when applying for different stages of the path to permanent residency –– whether it's applying for the H-1B visa or if you're in the green card pipeline –– I've heard just secondhand that they avoid traveling abroad or going back to visit family just to kind of show commitment that they're residing here in the US. So I don't know how much of that is recent. My dad actually, he started out as a PhD student in math at University of Iowa before switching to computer science and I remember we had a death in the family and he couldn't go back because it was so early on in his stay. So I'm sure it's a conflicted situation for a lot of Chinese international students in the US.
Lucas Perry: So moving along here and ending this component section, you also say here –– and this kind of goes back to what we were discussing earlier about government guidance funds –– Chinese government is also starting to take a more active role in funding AI ventures, helping to grow the fourth driver of AI development, which again is the commercial AI ecosystems, which support and are the context for hardware data and research on algorithm development. And so the Chinese government is disbursing funds through what are called Government Guidance Funds or GGFs, set up by local governments and state owned companies. And the government has invested more than a billion US dollars on domestic startups. This seems to be in clear contrast with how America functions on this, with much of the investments shifting towards healthcare and AI as the priority areas in the last two years.
Jeffrey Ding: Right, yeah. So the GGFs are an interesting funding vehicle. The China Money Network, which has I think the best English language coverage of these vehicles, say that they may be history's greatest experiment in using state capitol to reshape a nation's economy. These essentially are Public Private Partnerships, PPPs, which do exist across the world, in the US. And the idea is basically the state seeds and anchors these investment vehicles and then they partner with private capital to also invest in startups, companies that the government thinks either are supporting a particular policy initiative or are good for overall development.
A lot of this is hard to decipher in terms of what the impact has been so far, because publicly available information is relatively scarce. I mentioned in my report that these funds haven't had a successful exit yet, which means that maybe just they need more time. I think there's also been some complaints that the big VCs –– whether it's Chinese VCs or even international VCs that have a Chinese arm –– they much prefer to just to go it on their own rather than be tied to all the strings and potential regulations that come with working with the government. So I think it's definitely a case of time will tell, and also this is a very fertile research area that I know some people are looking into. So be on the lookout for more conclusive findings about these GGFs, especially how they relate to the emerging technologies.
Lucas Perry: All right. So we're getting to your capabilities section, which assesses the current state of China's AI capabilities across the four drivers of AI development. Here you're constructing an AI Potential Index, which is an index for the potentiality of, say, a country, based off these four variables, to be able to create successful AI products. So based on your research, you give China an AI Potential Index score of 17, which is about half of the US's AI Potential Index score of 33. And so you state here that what is sort of essential to draw from this finding is the relative scale, or at least the proportionality, between China and the US. So the conclusion which we can try to draw from this is that China trails the US in every driver except for access to data, and that on all of these dimensions China is about half as capable as the US.
Jeffrey Ding: Yes, so the AIPI, the AI Potential Index, was definitely just meant as a first cut at developing a measure for which we can make comparative claims. I think at the time, and even now, I think we just throw around things like, “who is ahead in AI?” I was reading this recent Defense One article that was like, “China's the world leader in GANs,” G-A-Ns, Generative Adversarial Networks. That's just not even a claim that is coherent. Are you the leader at developing the talent who is going to make advancement to GANs? Are you the leader at applying and deploying GANs in the military field? Are you the leader in producing the most publications related to GANs?
I think that's what was frustrating me about the conversation and net assessment of different countries’ AI capabilities, so that's why I tried to develop a more systematic framework which looked at the different drivers, and it was basically looking at what is the potential of country's AI capabilities based on their marks across these drivers.
Since then, probably the main thing that I've done update this was in my written testimony before the US China Economic and Security Review Commission, where I kind of switch up a little bit how I evaluate the current AI capabilities of China and the US. Basically there's this very fuzzy concept of national AI capabilities that we throw around and I slice it up into three cross-sections. The first is, let's look at what the scientific and technological inputs and outputs different countries are putting into AI. So that's: how many publications are coming out of this country in Europe versus China versus US? How many outputs also in the sense of publications or inputs in the sense of R&D investments? So let's take a look at that.
The second slice is, let's not just say AI. I think every time you say AI it's always better to specify subtypes, or at least in the second slice I look at different layers of the AI value chain: foundational layers, technological layers, and the application layer. So, for example, foundation layers may be who is leading in developing the AI open source software that serves as the technological backbone for a lot of these AI applications and technologies?
And then the third slice that I take is different sub domains of AI –– so computer vision, predictive intelligence, natural language processing, et cetera. And basically my conclusion: I throw a bunch of statistics in this written testimony out there –– some of it draws from this AI potential index that I put out last year –– and my conclusion is that China is not poised to overtake the US in the technology domain of AI; Rather the US maintains structural advantages in the quality of S and T inputs and outputs, the fundamental layers of the AI value chain, and key sub domains of AI.
So yeah, this stuff changes really fast too. I think a lot of people are trying to put together more systemic ways of measuring these things. So Jack Clark at openAI; projects like the AI index out of Stanford University; Matt Sheehan recently put out a really good piece for MacroPolo on developing sort of a five-dimensional framework for understanding data. So in this AIPI first cut, my data indicator is just a very raw who has more mobile phone users, but that obviously doesn't matter for who's going to lead in autonomous vehicles. So having finer grained understanding of how to measure different drivers will definitely help this field going forward.
Lucas Perry: What can you say about symmetries or asymmetries in terms of sub-fields in AI research like GANs or computer vision or any number of different sub-fields? Can we expect very strong specialties to develop in one country rather than another, or there to be lasting asymmetries in this space, or does research publication subvert this to some extent?
Jeffrey Ding: I think natural language processing is probably the best example because everyone says NLP, but then you just have that abstract word and you never dive into, "Oh wait, China might have a comparative advantage in Chinese language data processing, speech recognition, knowledge mapping,” which makes sense. There is just more of an incentive for Chinese companies to put out huge open source repositories to train automatic speech recognition.
So there might be some advantage in Chinese language data processing, although Microsoft Research Asia has very strong NOP capabilities as well. Facial recognition, maybe another area of comparative advantage: I think in my testimony I cite that China has published 900 patents in this sub domain in 2017; In that same year less than 150 patents related to facial recognition were filed in the US. So that could be partly just because there's so much more of a fervor for surveillance applications, but in other domains such as the larger scale business applications the US probably possesses a decisive advantage. So autonomous vehicles are the best example of that: In my opinion, Google's Waymo, GM’s Cruise are lapping the field.
And then finally in my written testimony I also try to look at military applications, and I find one metric that puts the US as having more than seven times as many military patents filed with the terms “autonomous” or “unmanned” in the patent abstract in the years 2003 to 2015. So yeah, that's one of the research streams I'm really interested in, is how can we have more fine grain metrics that actually put into context China's AI development, and that way we can have a more measured understanding of it.
Lucas Perry: All right, so we've gone into length now providing a descriptive account of China and the United States and key descriptive insights of your research. Moving into consequences now, I'll just state some of these insights which you bring to light in your paper and then maybe you can expand on them a bit.
Jeffrey Ding: Sure.
Lucas Perry: You discuss the potential implications of China's AI dream for issues of AI safety and ethics, national security, economic development, and social governance. The thinking here is becoming more diversified and substantive, though you claim it's also too early to form firm conclusions about the long-term trajectory of China's AI development; This is probably also true of any other country, really. You go on to conclude that a group of Chinese actors is increasingly engaged with issues of AI safety and ethics.
A new book has been authored by Tencent's Research Institute, and it includes a chapter in which the authors discuss the Asilomar Principles in detail and call for strong regulations and controlling spells for AI. There's also this conclusion that military applications of AI could provide a decisive strategic advantage in international security. The degree to which China's approach to military AI represents a revolution in military affairs is an important question to study, to see how strategic advantages between the United States and China continue to change. You continue by elucidating how the economic benefit is the primary and immediate driving force behind China's development of AI –– and again, I think you highlighted this sort of manufacturing perspective on this.
And finally, China's adoption of AI Technologies could also have implications for its mode of social governance. For the state council’s AI plan, you state, “AI will play an irreplaceable role in maintaining social stability, an aim reflected in local level integrations of AI across a broad range of public services, including judicial services, medical care, and public security.” So given these sort of insights that you've come to and consequences of this descriptive picture we've painted about China and AI, is there anything else you'd like to add here?
Jeffrey Ding: Yeah, I think as you are laying out those four categories of consequences, I was just thinking this is what makes this area so exciting to study because if you think about it, each four of those consequences map out onto four research fields: AI ethics and safety, which with benevolent AI efforts, stuff that FLI is doing, the broader technology studies, critical technologies studies, technology ethics field; then in the social governance space, AI as a tool of social control: what are the social aftershocks of AI's economic implications? You have this entire field of democracy studies or studies of technology and authoritarianism; and the economic benefits, you have this entire field of innovation studies: how do we understand the productivity benefits of general purpose technologies? And of course with AI as a revolution in military affairs, you have this whole field of security studies that is trying to understand what are the implications of new emerging technologies for national security?
So it's easy to start delineating these into their separate containers. I think what's hard, especially for those of us are really concerned about that first field, AI ethics and safety, and the risks of AGI arms races, is a lot of other people are really, really concerned about those other three fields. And how do we tie in concepts from those fields? How do we take from those fields, learn from those fields, shape the language that we're using to also be in conversation with those fields –– and then also see how those fields may actually be in conflict with some of what our goals are? And then how do we navigate those conflicts? How do we prioritize different things over others? It's an exciting but daunting prospect ahead.
Lucas Perry: If you're listening to this and are interested in becoming an AI researcher in terms of the China landscape, we need you. There's a lot of great and open research questions here to work on.
Jeffrey Ding: For sure. For sure.
Lucas Perry: So I've extracted some insights from previous podcasts you did –– I can leave a link for that in the page for this podcast –– so I just want to kind of rapid fire these as points that I thought were interesting that we may or may not have covered here. You point out a language asymmetry: The best Chinese AI researchers read English and Chinese, whereas the western researchers generally cannot do this. You have a newsletter called China AI with 1A; Your newsletter attempts to correct for this as you translate important Chinese tech-related things into English. I suggest everyone follow that if you're interested in continuing to track China and AI. There is more international cooperation on research at international conferences –– this is a general trend that you point out: Some top Chinese AI conferences are English only. Furthermore, I believe that you claim that the top 10% of AI research is still happening in America and the UK.
Another point which I think that you've brought up is that China is behind on military AI uses. I'm also interested here just to see if you can expand a little bit more on it, but that China and AI safety and superintelligence is also something interesting to hear a little bit more about because on this podcast we often take the lens of long-term AI issues and AGI and super intelligence. So I think you mentioned that the Nick Bostrom of China is Professor, correct me if I get this wrong, Jao ting Wang. And also I'm curious here if you might be able to expand on how large or serious this China superintelligence FLI/FHI vibe is and what the implications of this are, and if there are any orgs in China that are explicitly focused on this. I'm sorry if this is a silly question, but are there like nonprofits in China in the same way that there are in the US? How does that function? Is China on the brink of having an FHI or FLI or MIRI or anything like this?
Jeffrey Ding: So a lot to untangle there and all really good questions. First, just to clarify, yeah, there are definitely nonprofits, non-governmental organizations. In recent years there has been some pressure on international nongovernmental organizations, nonprofit organizations, but there's definitely nonprofits. One of the open source NLP initiatives I mentioned earlier, the Chinese language Corpus, was put together by a nonprofit online organization called AIShell Foundation, and they put together AIShell-1, AIShell-2, which are the largest open source speech Corpus available for Mandarin speech recognition.
I haven't really followed up on Jao ting Wang. He's a philosopher at the Chinese Academy of Social Sciences. The sort of “Nick Bostrom of China” label was more of a newsletter headline to get people to read, but he does devote a lot of time and thinking to the long-term risks of AI. Another professor at Nanjing University by the name of Zhi-Hua Zhou, he's published articles about the need to not even touch some of what he calls strong AI. These were published in a pretty influential publication outlet by the Chinese Computer Federation, which brings together a lot of the big name computer scientists. So there's definitely conversations about this happening. Whether there is an FHI, FLI equivalent, let's say probably not, at least not yet.
Peking University may be developing something in this space. Berggruen Institute is also I think looking at some related issues. There's probably a lot of stuff happening in Hong Kong as well; Maybe we just haven't looked hard enough. I think the biggest difference is there's definitely not something on the level of a DeepMind or OpenAI, because even the firms with the best general AI capabilities –– DeepMind and OpenAI almost like these unique entities where profits and stocks don't matter.
So yeah, definitely some differences, but honestly I updated significantly once I started reading more, and nobody had really looked at this Zhi-Hua Zhou essay before we went looking and found it. So maybe there are a lot of these organizations and institutions out there but we just need to look harder.
Lucas Perry: So on this point of there not being OpenAI or DeepMind equivalents, are there any research organizations or departments explicitly focused on the mission of creating artificial general intelligence or superintelligence safely scalable machine learning systems that could go from now until infinity? Or is this just more like scattered researchers?
Jeffrey Ding: I think it’s how you define an AGI project. Like what you just said is probably a good tight definition. I know Seth Baum, he's done some research tracking AGI projects and he says that there are six in China. I would say probably the only ones that come close are, I guess Tencent says it's one of their missions streams to develop artificial general intelligence; horizon robotics, which is actually like a chip company, they also state it as one of their objectives. It depends also on how much you think work on neuroscience related pathways into AGI count or not. So there's probably some Chinese Academy of Science labs working on whole brain emulation or kind of more brain inspired approaches to AGI, but definitely not anywhere to the level of DeepMind, OpenAI.
Lucas Perry: All right. So there are some myths in table one of your paper which you demystify. Three of these are: China's approach to AI is defined by its top-down and monolithic nature; China is winning the AI arms race; And there is little to no discussion of issues of AI ethics and safety in China. And then maybe lastly I might add, if you might be able to add to it, that there is just to begin with an AI arms race between the US and China.
Jeffrey Ding: Yeah, I think that's a good addition. I think we have to be careful about which historical analogies and memes we choose. So “arms race” is a very specific call back to cold war context, where there's almost these discrete types of missiles that we are racing Soviet Union on and discrete applications that we can count up; Or even going way back to what some scholars call the first industrial arms race in the military sphere over steam power boats between Britain and France in the late 19th century. And all of those instances you can count up. France has four iron clads, UK has four iron clads; They're racing to see who can build more. I don't think there's anything like that. There's not this discreet thing that we’re racing to see who can have more of. If anything, it's about a competition to see who can absorb AI advances from abroad better, who can diffuse them throughout the economy, who can adopt them in a more sustainable way without sacrificing core values.
So that's sort of one meme that I really want to dispel. Related to that, assumptions that often influence a lot of our discourse on this is techno-nationalist assumption, which is this idea that technology is contained within national boundaries and that the nation state is the most important actor –– which is correct and a good one to have and a lot of instances. But there are also good reasons to adopt techno-globalist assumptions as well, especially in the area of how fast technologies diffuse nowadays and also how much underneath this national level competition, firms from different countries are working together and make standards alliances with each other. So there's this undercurrent of techno-globalism, where there are people flows, idea flows, company flows happening while the coverage and the sexy topic is always going to be about national level competition, zero sum competition, relative games rhetoric. So you're trying to find a balance between those two streams.
Lucas Perry: What can you say about this sort of reflection on zero sum games versus healthy competition and the properties of AI and AI research? I'm seeking clarification on this secondary framing that we can take on a more international perspective about deployment and implementation of AI research and systems rather than, as you said, this sort of techno-nationalist one.
Jeffrey Ding: Actually, this idea comes from my supervisor: Relative gains make sense if there's only two players involved, just from a pure self-interest maximizing standpoint. But once you introduce three or more players, relative gains doesn't make as much sense as optimizing for absolute gains. So maybe one way to explain this is to take the perspective of a European country –– let's say Germany –– and you are working on an AI project with China or some other country that maybe the US is pressuring you not to work with; You’re working with Saudi Arabia or China on some project and it's going to benefit China 10 arbitrary points and it's going to benefit Germany eight arbitrary points versus if you didn't choose to cooperate at all.
So in that sense, Germany, the rational actor, would take that deal. You're not just caring about being better than China; From a German perspective, you care about maintaining leadership in the European Union, providing health benefits to your citizens, continuing to power your economy. So in that sense you would take the deal even though China benefits a little bit more, relatively speaking.
I think currently a lot of people in the US are locked into this mindset that the only two players that exist in the world are the US and China. And if you look at our conversation, right, oftentimes I've displayed that bias as well. We should probably have talked a lot more about China-EU or China-Japan cooperation in this space and networks in this space because there's a lot happening there too. So a lot of US policy makers see this as a two-player game between the US and China. And then in that sense, if there's some cancer research project about discovering proteins using AI that may benefit China by 10 points and benefit the US only by eight points, but it's going to save a lot of people from cancer –– if you only care about making everything about maintaining a lead over China, then you might not take that deal. But if you think about it from the broader landscape of it's not just a zero sum competition between US and China, then your kind of evaluation of those different point structures and what you think is rational will change.
Lucas Perry: So as there's more actors, is the idea here that you care more about absolute gains in the sense that these utility points or whatever can be translated into decisive strategic advantages like military advantages?
Jeffrey Ding: Yeah, I think that's part of it. What I was thinking along that example is basically
if you as Germany don't choose to cooperate with Saudi Arabia or work on this joint research project with China then the UK or some other countries just going to swoop in. And that possibility doesn't exist in the world where you're just thinking about two players. There's a lot of different ways to fit these sort of formal models, but that's probably the most simplistic way of explaining it.
Lucas Perry: Okay, cool. So you've spoken a bit here on important myths that we need to dispel or memes that we need to combat. And recently Peter Thiel has been on a bunch of conservative platforms, and he also wrote an op-ed, basically fanning the flames of AGI as a military weapon, AI as a path to superintelligence and, “Google campuses have lots of Chinese people on them who may be spies,” and that Google is actively helping China with AI military technology. In terms of bad memes and myths to combat, what are your thoughts here?
Jeffrey Ding: There's just a lot of things that Thiel gets wrong. I’m mostly kind of just confused because he is one of the original founders of OpenAI, he’s funded other institutions, really concerned about AGI safety, really concerned about race dynamics –– and then in the middle of this piece, he first says AI is a military technology, then he goes back to saying AI is dual use in the middle, and then he says this ambiguity is "strangely missing from the narrative that pits a monolithic AI against all of humanity." He out of anyone should know that these conversations about the risks of AGI, why are you attacking this straw man in the form of a terminator AI meme? Especially, you're funding a lot of the organizations that are worried about the risks of AGI for all of humanity.
The other main thing that's really problematic is if you're concerned about the US military advantage, that more than ever is rooted on our innovation advantage. It's not about spinoff from military innovation to civilian innovation, which was the case in the days of US tech competition against Japan. It's more the case of spin on, where innovations are happening in the commercial sector that are undergirding the US military advantage.
And this idea of painting Google as anti-American for setting up labs in China is so counterproductive. There are independent Google developer conferences all across China just because so many Chinese programmers want to use Google tools like TensorFlow. It goes back to the fundamental AI open source software I was talking about earlier that lets Google expand its talent pool: People want to work on Google products; They’re more used to the framework of Google tools to build all these products. Google's not doing this out of charity to help the Chinese military. They’re doing this because the US has a flawed high-skilled immigration system, so they need to go to other countries to get talent.
Also, the other thing about the piece is he cites no empirical research on any of these fronts, when there's this whole globalization of innovation literature that backs up empirically a lot of what I'm saying. And then I've done my own empirical research on Microsoft Research Asia, which as we've mentioned is their second biggest lab overall, it’s based in Beijing. I've tracked their PhD Fellowship Program: This basically gives people at Chinese PhD programs, you get a full scholarship and you just do an internship at Microsoft Research Asia for one of the summers. And then we track their career trajectories, and a lot of them end up coming to the US or working for Microsoft Research Asia in Beijing. And the ones that come to the US don't just go to Microsoft: They go to Snapchat or Facebook or other companies. And it's not just about the people: As I mentioned earlier, we have this innovation centrism about who produces the technology first, but oftentimes it's about who diffuses and adopts the technology first. And we're not always going to be the first on the scene, so we have to be able to adopt and diffuse technologies that are invented first in other areas. And these overseas labs are some of our best portals into understanding what's happening in these other areas. If we lose them, it's another form of asymmetry because Chinese AI companies are going abroad and expanding.
I honestly, I'm just really confused about what the point of this piece was and to be honest, it's kind of sad because this is not what Thiel researches every day. So he's obviously picking up bits and pieces from the narrative frames that are dominating our conversation. And it's actually probably a structural stain on how we've allowed the discourse to have so many of these bad problematic memes, and we need more people calling them out actively, doing the heart to heart conversations behind the scenes to get people to change their minds or have productive constructive conversations about these.
And the last thing I'll point out here is there's this zombie Cold War mentality that still lingers today, and I think the historian Walter McDougall was really great in calling this out, where he talks about we paint this other, this enemy, and we use it to justify sacrifices in human values to drive society to its fullest technological potential. And that often comes with sacrificing human values like privacy, equality, freedom of speech. And I don't want us to compete with China over who can build better tools to sensor, repress, and surveil dissidents and minority groups, right? Let's see who can build the better, I don't know, industrial internet of things or build better privacy preserving algorithms that are going to sustain a more trustworthy AI ecosystem.
Lucas Perry: Awesome. So just moving along here as we're making it to the end of our conversation: What are updates you've had or major changes since you've written Deciphering China's AI Dreams, since it has been a year?
Jeffrey Ding: Yeah, I mentioned some of the updates in the capability section. The consequences, I mean I think those are still the four main big issues, all of them tied to four different literature bases. The biggest change would probably be in the component section. I think when I started out, I was pretty new in this field, I was reading a lot of literature from the China watching community and also a lot from Chinese comparative politics or articles about China, and so I focused a lot on government policies. And while I think the party and the government are definitely major players, I think I probably overemphasized the importance of government policies versus what is happening at the local level.
So if I were to go back and rewrite it, I would’ve looked a lot more at what is happening at the local level, given more examples of AI firms, like iFlytek I think is a very interesting under-covered firm, and how they are setting up research institutes with a university in Chung Cheng very similar to the industry- academia style collaborations in the US, basically just ensuring that they're able to train the next generation of talent. They have relatively close ties to the state as well, I think controlling shares or a large percentage of shares owned by state-owned vehicles. So I probably would have gone back and looked at some of these more under-covered firms and localities and looked at what they were doing rather than just looking at the rhetoric coming from the central government.
Lucas Perry: Okay. What does it mean for there to be healthy competition between the United States and China? What is an ideal AI research and political situation? What are the ideal properties of the relations the US and China can have on the path to superintelligence?
Jeffrey Ding: Yeah.
Lucas Perry: Solve AI Governance for me, Jeff!
Jeffrey Ding: If I could answer that question, I think I could probably retire or something. I don't know.
Lucas Perry: Well, we'd still have to figure out how to implement the ideal governance solutions.
Jeffrey Ding: Yeah. I think one starting point is on the way to more advanced AI systems, we have to stop looking at AI as if it's like this completely special area with no analogs, because even though there are unique aspects of AI –– like their autonomous intelligence systems, a possibility of the product surpassing human level intelligence, or the process surpassing human level intelligence –– we can learn a lot from past general purpose technologies like steam, electricity, the diesel engine. And we can learn about a lot of competition in past strategic industries like chips, steel.
So I think probably one thing that we can distill from some of this literature is there are some aspects of AI development that are going to be more likely to lead to race dynamics than others. So one cut that you could take are industries where it's likely that there are only going to be two or three, four or five major players –– so it might be the case that capital costs, the upstart costs, the infrastructure costs of autonomous vehicles requires that there are going to be only one or two players across the world. And that is like, hey, if you're a national government who's thinking strategically, you might really want to have a player in that space, so that might incentivize more competition. Whereas in other fields, maybe there's just going to be a lot more competition or less need for relative gain, zero sum thinking. So like neural machine translation, that could be a case of something that just almost becomes like a commodity.
So then there are things we can think about in those fields where there's only going to be four or five players or three or four players. Can we maybe balance it out so that at least one is from the two major powers or is the better approach to, I don't know, enact global competition, global antitrust policy to kind of ensure that there's always going to be a bunch of different players from a bunch of different countries? So those are some of the things that come to mind that I'm thinking about, but yeah, this is definitely something where I claim zero credibility relative to others who are thinking about it.
Lucas Perry: Right. Well unclear anyone has very good answers here. I think my perspective, to add at least one frame on it, is that given the dual use nature of many of the technologies like computer vision and like embedded robot systems and developing autonomy and image classification –– all of these different AI specialty subsystems can be sort of put together in arbitrary ways. So in terms of autonomous weapons, FLI's position is, it's important to establish international standards around the appropriate and beneficial uses of these technologies.
Image classification, as people already know, can be used for discrimination or beneficial things. And the technologies can be aggregated to make anything from literal terminator swarm robots to lifesaving medical treatments. So the relation between the United States and China can be made more productive if clear standards based on the expression of the principles we enumerated earlier could be created. And given that, then we might be taking some paths towards a beneficial beautiful future of advanced AI systems.
Jeffrey Ding: Yeah, no, I like that a lot. And some of the technical standards documents I've been translating: I definitely think in the short-term, technical standards are a good way forward, sort of solve the starter pack type of problems before AGI. Even some Chinese white papers on AI standardization have put out the idea of ranking the intelligence level of different autonomous systems –– like an autonomous car might be more than a smart speaker or something: Even that is a nice way to kind of keep track of the progress, is continuities in terms of intelligence explosions and trajectories in the space. So yeah, I definitely second that idea. Standardization efforts, autonomous weapons regulation efforts, as serving as the building blocks for larger AGI safety issues.
Lucas Perry: I would definitely like to echo this starter pack point of view. There's a lot of open questions about the architectures or ways in which we're going to get to AGI, about how the political landscape and research landscape is going to change in time. But I think that we already have enough capabilities and questions that we should really be considering where we can be practicing and implementing the regulations and standards and principles and intentions today in 2019 that are going to lead to robustly good futures for AGI and superintelligence.
Jeffrey Ding: Yeah. Cool.
Lucas Perry: So Jeff, if people want to follow you, what is the best way to do that?
Jeffrey Ding: You can hit me up on Twitter, I'm @JJDing99; Or I put out a weekly newsletter featuring translations on AI related issues from Chinese media, Chinese scholars and that's China AI Newsletter, C-H-I-N-A-I. if you just search that, it should pop up.
Lucas Perry: Links to those will be provided in the description of wherever you might find this podcast. Jeff, thank you so much for coming on and thank you for all of your work and research and efforts in this space, for helping to create a robust and beneficial future with AI.
Jeffrey Ding: All right, Lucas. Thanks. Thanks for the opportunity. This was fun.
Lucas Perry: If you enjoyed this podcast, please subscribe, give it a like or share it on your preferred social media platform. We'll be back again soon with another episode in the AI Alignment series.