AI Alignment Podcast: An Overview of Technical AI Alignment in 2018 and 2019 with Buck Shlegeris and Rohin Shah
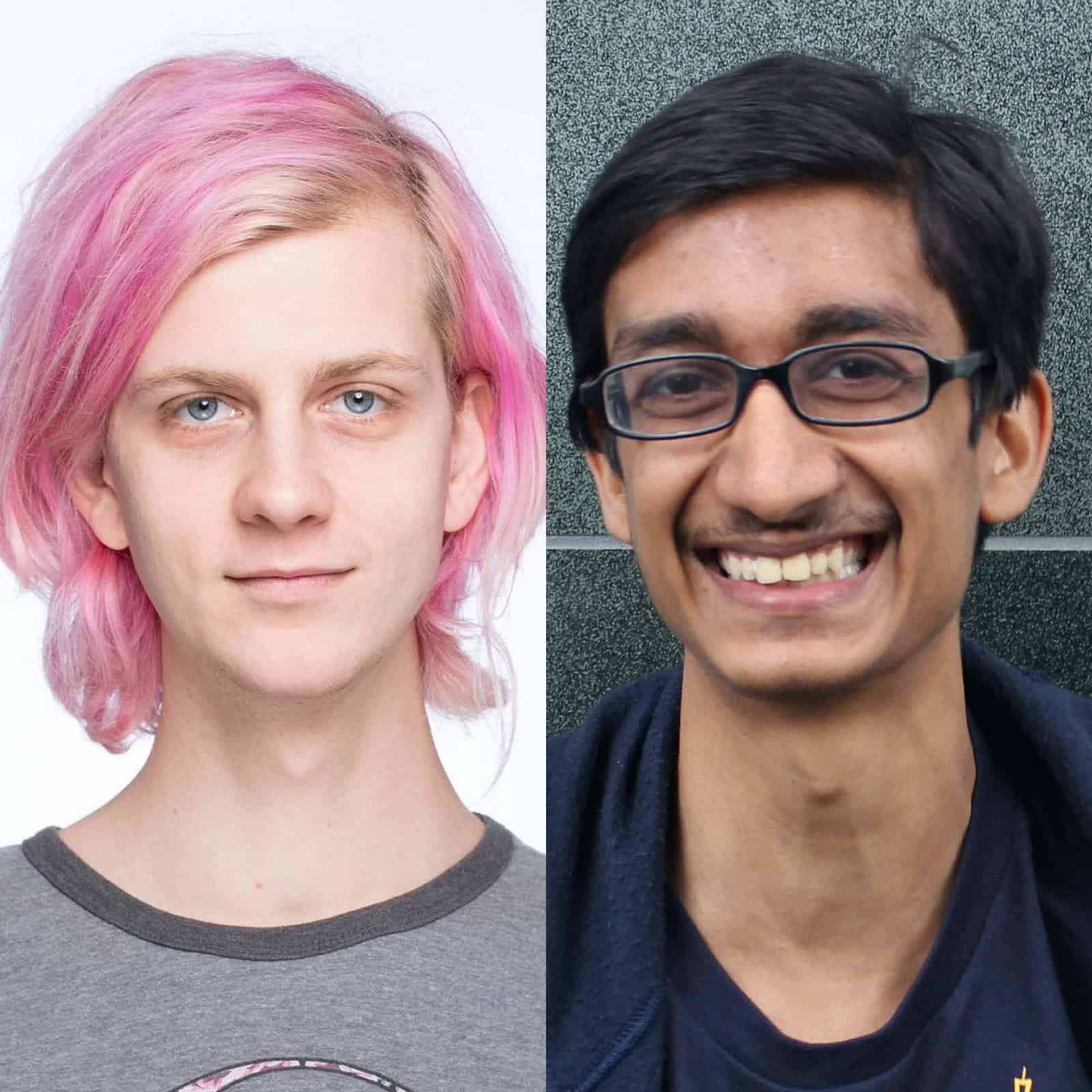
Topics discussed in this episode include:
- Rohin's and Buck's optimism and pessimism about different approaches to aligned AI
- Traditional arguments for AI as an x-risk
- Modeling agents as expected utility maximizers
- Ambitious value learning and specification learning/narrow value learning
- Agency and optimization
- Robustness
- Scaling to superhuman abilities
- Universality
- Impact regularization
- Causal models, oracles, and decision theory
- Discontinuous and continuous takeoff scenarios
- Probability of AI-induced existential risk
- Timelines for AGI
- Information hazards
Timestamps:
0:00 Intro
3:48 Traditional arguments for AI as an existential risk
5:40 What is AI alignment?
7:30 Back to a basic analysis of AI as an existential risk
18:25 Can we model agents in ways other than as expected utility maximizers?
19:34 Is it skillful to try and model human preferences as a utility function?
27:09 Suggestions for alternatives to modeling humans with utility functions
40:30 Agency and optimization
45:55 Embedded decision theory
48:30 More on value learning
49:58 What is robustness and why does it matter?
01:13:00 Scaling to superhuman abilities
01:26:13 Universality
01:33:40 Impact regularization
01:40:34 Causal models, oracles, and decision theory
01:43:05 Forecasting as well as discontinuous and continuous takeoff scenarios
01:53:18 What is the probability of AI-induced existential risk?
02:00:53 Likelihood of continuous and discontinuous take off scenarios
02:08:08 What would you both do if you had more power and resources?
02:12:38 AI timelines
02:14:00 Information hazards
02:19:19 Where to follow Buck and Rohin and learn more
Works referenced:
Takeoff Speeds by Paul Christiano
Discontinuous progress investigation by AI Impacts
An Overview of Technical AI Alignment with Rohin Shah (Part 1)
An Overview of Technical AI Alignment with Rohin Shah (Part 2)
Intelligence Explosion Microeconomics
AI Alignment: Why It's Hard and Where to Start
AI Risk for Computer Scientists
We hope that you will continue to join in the conversations by following us or subscribing to our podcasts on Youtube, Spotify, SoundCloud, iTunes, Google Play, Stitcher, iHeartRadio, or your preferred podcast site/application. You can find all the AI Alignment Podcasts here.
Transcript
Note: The following transcript has been edited for style and clarity.
Lucas Perry: Welcome to the AI Alignment Podcast. I’m Lucas Perry. Today we have a special episode with Buck Shlegeris and Rohin Shah that serves as a review of progress in technical AI alignment over 2018 and 2019. This episode serves as an awesome birds eye view of the varying focus areas of technical AI alignment research and also helps to develop a sense of the field. I found this conversation to be super valuable for helping me to better understand the state and current trajectory of technical AI alignment research. This podcast covers traditional arguments for AI as an x-risk, what AI alignment is, the modeling of agents as expected utility maximizers, iterated distillation and amplification, AI safety via debate, agency and optimization, value learning, robustness, scaling to superhuman abilities, and more. The structure of this podcast is based on Rohin’s AI Alignment Forum post titled AI Alignment 2018-19 Review. That post is an excellent resource to take a look at in addition to this podcast. Rohin also had a conversation with us about just a year ago titled An Overview of Technical AI Alignment with Rohin shah. This episode serves as a follow up to that overview and as an update to what's been going on in the field. You can find a link for it on the page for this episode.
Buck Shlegeris is a researcher at the Machine Intelligence Research Institute. He tries to work to make the future good for sentient beings and currently believes that working on existential risk from artificial intelligence is the best way of doing this. Buck worked as a software engineer at PayPal before joining MIRI, and was the first employee at Triplebyte. He previously studied at the Australian National University, majoring in CS and minoring in math and physics, and he has presented work on data structure synthesis at industry conferences.
Rohin Shah is a 6th year PhD student in Computer Science at the Center for Human-Compatible AI at UC Berkeley. He is involved in Effective Altruism and was the co-president of EA UC Berkeley for 2015-16 and ran EA UW during 2016-2017. Out of concern for animal welfare, Rohin is almost vegan because of the intense suffering on factory farms. He is interested in AI, machine learning, programming languages, complexity theory, algorithms, security, and quantum computing to name a few. Rohin’s research focuses on building safe and aligned AI systems that pursue the objectives their users intend them to pursue, rather than the objectives that were literally specified. He also publishes the Alignment Newsletter, which summarizes work relevant to AI alignment. The Alignment Newsletter is something I highly recommend that you follow in addition to this podcast.
And with that, let’s get into our review of AI alignment with Rohin Shah and Buck Shlegeris.
To get things started here, the plan is to go through Rohin's post on the Alignment Forum about AI Alignment 2018 and 2019 In Review. We'll be using this as a way of structuring this conversation and as a way of moving methodically through things that have changed or updated in 2018 and 2019, and to use those as a place for conversation. So then, Rohin, you can start us off by going through this document. Let's start at the beginning, and we'll move through sequentially and jump in where necessary or where there is interest.
Rohin Shah: Sure, that sounds good. I think I started this out by talking about this basic analysis of AI risk that's been happening for the last couple of years. In particular, you have these traditional arguments, so maybe I'll just talk about the traditional argument first, which basically says that the AI systems that we're going to build are going to be powerful optimizers. When you optimize something, you tend to get these sort of edge case outcomes, these extreme outcomes that are a little hard to predict ahead of time.
You can't just rely on tests with less powerful systems in order to predict what will happen, and so you can't rely on your normal common sense reasoning in order to deal with this. In particular, powerful AI systems are probably going to look like expected utility maximizers due to various coherence arguments, like the Von Neumann–Morgenstern rationality theorem, and these expected utility maximizers have convergent instrumental sub-goals, like not wanting to be switched off because then they can't achieve their goal, and wanting to accumulate a lot of power and resources.
The standard argument goes, because AI systems are going to be built this way, they will have these convergent instrumental sub-goals. This makes them dangerous because they will be pursuing goals that we don't want.
Lucas Perry: Before we continue too much deeper into this, I'd want to actually start off with a really simple question for both of you. What is AI alignment?
Rohin Shah: Different people mean different things by it. When I use the word alignment, I'm usually talking about what has been more specifically called intent alignment, which is basically aiming for the property that the AI system is trying to do what you want. It's trying to help you. Possibly it doesn't know exactly how to best help you, and it might make some mistakes in the process of trying to help you, but really what it's trying to do is to help you.
Buck Shlegeris: The way I would say what I mean by AI alignment, I guess I would step back a little bit, and think about why it is that I care about this question at all. I think that the fundamental fact which has me interested in anything about powerful AI systems of the future is that I think they'll be a big deal in some way or another. And when I ask myself the question “what are the kinds of things that could be problems about how these really powerful AI systems work or affect the world”, one of the things which feels like a problem is that, we might not know how to apply these systems reliably to the kinds of problems which we care about, and so by default humanity will end up applying them in ways that lead to really bad outcomes. And so I guess, from that perspective, when I think about AI alignment, I think about trying to make ways of building AI systems such that we can apply them to tasks that are valuable, such that that they'll reliably pursue those tasks instead of doing something else which is really dangerous and bad.
I’m fine with intent alignment as the focus. I kind of agree with, for instance, Paul Christiano, that it's not my problem if my AI system incompetently kills everyone, that's the capability's people's problem. I just want to make the system so it's trying to cause good outcomes.
Lucas Perry: Both of these understandings of what it means to build beneficial AI or aligned AI systems can take us back to what Rohin was just talking about, where there's this basic analysis of AI risk, about AI as powerful optimizers and the associated risks there. With that framing and those definitions, Rohin, can you take us back into this basic analysis of AI risk?
Rohin Shah: Sure. The traditional argument looks like AI systems are going to be goal-directed. If you expect that your AI system is going to be goal-directed, and that goal is not the one that humans care about, then it's going to be dangerous because it's going to try to gain power and resources with which to achieve its goal.
If the humans tried to turn it off, it's going to say, "No, don't do that," and it's going to try to take actions that avoid that. So it pits the AI and the humans in an adversarial game with each other, and you ideally don't want to be fighting against a superintelligent AI system. That seems bad.
Buck Shlegeris: I feel like Rohin is to some extent setting this up in a way that he's then going to argue is wrong, which I think is kind of unfair. In particular, Rohin, I think you're making these points about VNM theorems and stuff to set up the fact that it seems like these arguments don't actually work. I feel that this makes it kind of unfairly sound like the earlier AI alignment arguments are wrong. I think this is an incredibly important question, of whether early arguments about the importance of AI safety were quite flawed. My impression is that overall the early arguments about AI safety were pretty good. And I think it's a very interesting question whether this is in fact true. And I'd be interested in arguing about it, but I think it’s the kind of thing that ought to be argued about explicitly.
Rohin Shah: Yeah, sure.
Buck Shlegeris: And I get that you were kind of saying it narratively, so this is only a minor complaint. It's a thing I wanted to note.
Rohin Shah: I think my position on that question of “how good were the early AI risk arguments,” probably people's internal beliefs were good as to why AI was supposed to be risky, and the things they wrote down were not very good. Some things were good and some things weren't. I think Intelligence Explosion Microeconomics was good. I think AI Alignment: Why It's Hard and Where to Start, was misleading.
Buck Shlegeris: I think I agree with your sense that people probably had a lot of reasonable beliefs but that the written arguments seem flawed. I think another thing that's true is that random people like me who were on LessWrong in 2012 or something, ended up having a lot of really stupid beliefs about AI alignment, which I think isn't really the fault of the people who were thinking about it the best, but is maybe sociologically interesting.
Rohin Shah: Yes, that seems plausible to me. Don't have a strong opinion on it.
Lucas Perry: To provide a little bit of framing here and better analysis of basic AI x-risk arguments, can you list what the starting arguments for AI risk were?
Rohin Shah: I think I am reasonably well portraying what the written arguments were. Underlying arguments that people probably had would be something more like, "Well, it sure seems like if you want to do useful things in the world, you need to have AI systems that are pursuing goals." If you have something that's more like tool AI, like Google Maps, that system is going to be good at the one thing it was designed to do, but it's not going to be able to learn and then apply its knowledge to new tasks autonomously. It sure seems like if you want to do really powerful things in the world, like run companies or make policies, you probably do need AI systems that are constantly learning about their world and applying their knowledge in order to come up with new ways to do things.
In the history of human thought, we just don't seem to know of a way to cause that to happen except by putting goals in systems, and so probably AI systems are going to be goal-directed. And one way you can formalize goal-directedness is by thinking about expected utility maximizers, and people did a bunch of formal analysis of that. Mostly going to ignore it because I think you can just say all the same thing with the idea of pursuing goals and it's all fine.
Buck Shlegeris: I think one important clarification to that, is you were saying the reason that tool AIs aren't just the whole story of what happens with AI is that you can't apply it to all problems. I think another important element is that people back then, and I now, believe that if you want to build a really good tool, you're probably going to end up wanting to structure that as an agent internally. And even if you aren't trying to structure it as an agent, if you're just searching over lots of different programs implicitly, perhaps by training a really large recurrent policy, you're going to end up finding something agent shaped.
Rohin Shah: I don't disagree with any of that. I think we were using the words tool AI differently.
Buck Shlegeris: Okay.
Rohin Shah: In my mind, if we're talking about tool AI, we're imagining a pretty restricted action space where no matter what actions in this action space are taken, with high probability, nothing bad is going to happen. And you'll search within that action space, but you don't go to arbitrary action in the real world or something like that. This is what makes tool AI hard to apply to all problems.
Buck Shlegeris: I would have thought that's a pretty non-standard use of the term tool AI.
Rohin Shah: Possibly.
Buck Shlegeris: In particular, I would have thought that restricting the action space enough that you're safe, regardless of how much it wants to hurt you, seems kind of non-standard.
Rohin Shah: Yes. I have never really liked the concept of tool AI very much, so I kind of just want to move on.
Lucas Perry: Hey, It’s post-podcast Lucas here. I just want to highlight here a little bit of clarification that Rohin was interested in adding, which is that he thinks that “tool AI evokes a sense of many different properties that he doesn't know which properties most people are usually thinking about and as a result he prefers not to use the phrase tool AI. And instead would like to use more precise terminology. He doesn’t necessarily feel though that the concepts underlying tool AI are useless.” So let's tie things a bit back to these basic arguments for x-risk that many people are familiar with, that have to do with convergent instrumental sub-goals and the difficulty of specifying and aligning systems with our goals and what we actually care about in our preference hierarchies.
One of the things here that Buck was seeming to bring up, he was saying that you may have been narratively setting up the Von Neumann–Morgenstern theorem, which sets up AIs as expected utility maximizers, and that you are going to argue that that argument, which is sort of the formalization of these earlier AI risk arguments, that that is less convincing to you now than it was before, but Buck still thinks that these arguments are strong. Could you unpack this a little bit more or am I getting this right?
Rohin Shah: To be clear, I also agree with Buck, that the spirit of the original arguments does seem correct, though, there are people who disagree with both of us about that. Basically, the VNM theorem roughly says, if you have preferences over a set of outcomes, and you satisfy some pretty intuitive axioms about how you make decisions, then you can represent your preferences using a utility function such that your decisions will always be, choose the action that maximizes the expected utility. This is, at least in writing, given as a reason to expect that AI systems would be maximizing expected utility. The thing is, when you talk about AI systems that are acting in the real world, they're just selecting a universe history, if you will. Any observed behavior is compatible with the maximization of some utility function. Utility functions are a really, really broad class of things when you apply it to choosing from universe histories.
Buck Shlegeris: An intuitive example of this: suppose that you see that every day I walk home from work in a really inefficient way. It's impossible to know whether I'm doing that because I happened to really like that path. For any sequence of actions that I take, there’s some utility functions such that that was the optimal sequence of actions. And so we don't actually learn anything about how my policy is constrained based on the fact that I'm an expected utility maximizer.
Lucas Perry: Right. If I only had access to your behavior and not your insides.
Rohin Shah: Yeah, exactly. If you have a robot twitching forever, that's all it does, there is a utility function over a universe history that says that is the optimal thing to do. Every time the robot twitches to the right, it's like, yeah, the thing that was optimal to do at that moment in time was twitching to the right. If at some point somebody takes a hammer and smashes the robot and it breaks, then the utility function that corresponds to that being optimal is like, yeah, that was the exact right moment to break down.
If you have these pathologically complex utility functions as possibilities, every behavior is compatible with maximizing expected utility, you might want to say something like, probably we'll have the simple utility maximizers, but that's a pretty strong assumption, and you'd need to justify it somehow. And the VNM theorem wouldn't let you do that.
Lucas Perry: So is the problem here that you're unable to fully extract human preference hierarchies from human behavior?
Rohin Shah: Well, you're unable to extract agent preferences from agent behavior. You can see any agent behavior and you can rationalize it as expected utility maximization, but it's not very useful. Doesn't give you predictive power.
Buck Shlegeris: I just want to have my go at saying this argument in three sentences. Once upon a time, people said that because all rational systems act like they're maximizing an expected utility function, we should expect them to have various behaviors like trying to maximize the amount of power they have. But every set of actions that you could take is consistent with being an expected utility maximizer, therefore you can't use the fact that something is an expected utility maximizer in order to argue that it will have a particular set of behaviors, without making a bunch of additional arguments. And I basically think that I was wrong to be persuaded by the naive argument that Rohin was describing, which just goes directly from rational things are expected utility maximizers, to therefore rational things are power maximizing.
Rohin Shah: To be clear, this was the thing I also believed. The main reason I wrote the post that argued against it was because I spent half a year under the delusion that this was a valid argument.
Lucas Perry: Just for my understanding here, the view is that because any behavior, any agent from the outside can be understood as being an expected utility maximizer, that there are behaviors that clearly do not do instrumental sub-goal things, like maximize power and resources, yet those things can still be viewed as expected utility maximizers from the outside. So additional arguments are required for why expected utility maximizers do instrumental sub-goal things, which are AI risky.
Rohin Shah: Yeah, that's exactly right.
Lucas Perry: Okay. What else is on offer other than expected utility maximizers? You guys talked about comprehensive AI services might be one. Are there other formal agentive classes of 'thing that is not an expected utility maximizer but still has goals?'
Rohin Shah: A formalism for that? I think some people like John Wentworth is for example, thinking about markets as a model of agency. Some people like to think of multi-agent groups together leading to an emergent agency and want to model human minds this way. How formal are these? Not that formal yet.
Buck Shlegeris: I don't think there's anything which is competitively popular with expected utility maximization as the framework for thinking about this stuff.
Rohin Shah: Oh yes, certainly not. Expected utility maximization is used everywhere. Nothing else comes anywhere close.
Lucas Perry: So there's been this complete focus on utility functions and representing the human utility function, whatever that means. Do you guys think that this is going to continue to be the primary way of thinking about and modeling human preference hierarchies? How much does it actually relate to human preference hierarchies? I'm wondering if it might just be substantially different in some way.
Buck Shlegeris: Me and Rohin are going to disagree about this. I think that trying to model human preferences as a utility function is really dumb and bad and will not help you do things that are useful. I don't know; If I want to make an AI that's incredibly good at recommending me movies that I'm going to like, some kind of value learning thing where it tries to learn my utility function over movies is plausibly a good idea. Even things where I'm trying to use an AI system as a receptionist, I can imagine value learning being a good idea.
But I feel extremely pessimistic about more ambitious value learning kinds of things, where I try to, for example, have an AI system which learns human preferences and then acts in large scale ways in the world. I basically feel pretty pessimistic about every alignment strategy which goes via that kind of a route. I feel much better about either trying to not use AI systems for problems where you have to think about large scale human preferences, or having an AI system which does something more like modeling what humans would say in response to various questions and then using that directly instead of trying to get a value function out of it.
Rohin Shah: Yeah. Funnily enough, I was going to start off by saying I think Buck and I are going to agree on this.
Buck Shlegeris: Oh.
Rohin Shah: And I think I mostly agree with the things that you said. The thing I was going to say was I feel pretty pessimistic about trying to model the normative underlying human values, where you have to get things like population ethics right, and what to do with the possibility of infinite value. How do you deal with fanaticism? What's up with moral uncertainty? I feel pretty pessimistic about any sort of scheme that involves figuring that out before developing human-level AI systems.
There's a related concept which is also called value learning, which I would prefer to be called something else, but I feel like the name's locked in now. In my sequence, I called it narrow value learning, but even that feels bad. Maybe at least for this podcast we could call it specification learning, which is sort of more like the tasks Buck mentioned, like if you want to learn preferences over movies, representing that using a utility function seems fine.
Lucas Perry: Like superficial preferences?
Rohin Shah: Sure. I usually think of it as you have in mind a task that you want your AI system to do, and now you have to get your AI system to reliably do it. It's unclear whether this should even be called a value learning at this point. Maybe it's just the entire alignment problem. But techniques like inverse reinforcement learning, preference learning, learning from corrections, inverse reward design where you learn from a proxy reward, all of these are more trying to do the thing where you have a set of behaviors in mind, and you want to communicate that to the agent.
Buck Shlegeris: The way that I've been thinking about how optimistic I should be about value learning or specification learning recently has been that I suspect that at the point where AI is human level, by default we'll have value learning which is about at human level. We're about as good at giving AI systems information about our preferences that it can do stuff with as we are giving other humans information about our preferences that we can do stuff with. And when I imagine hiring someone to recommend music to me, I feel like there are probably music nerds who could do a pretty good job of looking at my Spotify history, and recommending bands that I'd like if they spent a week on it. I feel a lot more pessimistic about being able to talk to a philosopher for a week, and then them answer hard questions about my preferences, especially if they didn't have the advantage of already being humans themselves.
Rohin Shah: Yep. That seems right.
Buck Shlegeris: So maybe that's how I would separate out the specification learning stuff that I feel optimistic about from the more ambitious value learning stuff that I feel pretty pessimistic about.
Rohin Shah: I do want to note that I collated a bunch of stuff arguing against ambitious value learning. If I had to make a case for optimism about even that approach, it would look more like, "Under the value learning approach, it seems possible with uncertainty over rewards, values, preferences, whatever you want to call them to get an AI system such that you actually are able to change it, because it would reason that if you're trying to change it, well then that means something about it is currently not good for helping you and so it would be better to let itself be changed. I'm not very convinced by this argument."
Buck Shlegeris: I feel like if you try to write down four different utility functions that the agent is uncertain between, I think it's just actually really hard for me to imagine concrete scenarios where the AI is corrigible as a result of its uncertainty over utility functions. Imagine the AI system thinks that you're going to switch it off and replace it with an AI system which has a different method of inferring values from your actions and your words. It's not going to want to let you do that, because its utility function is to have the world be the way that is expressed by your utility function as estimated the way that it approximates utility functions. And so being replaced by a thing which estimates utility functions or infers utility functions some other way means that it's very unlikely to get what it actually wants, and other arguments like this. I'm not sure if these are super old arguments that you're five levels of counter-arguments to.
Rohin Shah: I definitely know this argument. I think the problem of fully updated deference is what I would normally point to as representing this general class of claims and I think it's a good counter argument. When I actually think about this, I sort of start getting confused about what it means for an AI system to terminally value the final output of what its value learning system would do. It feels like some additional notion of how the AI chooses actions has been posited, that hasn't actually been captured in the model and so I feel fairly uncertain about all of these arguments and kind of want to defer to the future.
Buck Shlegeris: I think the thing that I'm describing is just what happens if you read the algorithm literally. Like, if you read the value learning algorithm literally, it has this notion of the AI system wants to maximize the human's actual utility function.
Rohin Shah: For an optimal agent playing a CIRL (cooperative inverse reinforcement learning) game, I agree with your argument. If you take optimality as defined in the cooperative inverse reinforcement learning paper and it's playing over a long period of time, then yes, it's definitely going to prefer to keep itself in charge rather than a different AI system that would infer values in a different way.
Lucas Perry: It seems like so far utility functions are the best way of trying to get an understanding of what human beings care about and value and have preferences over, you guys are bringing up all of the difficult intricacies with trying to understand and model human preferences as utility functions. One of the things that you also bring up here, Rohin, in your review, is the risk of lock-in, which may require us to solve hard philosophical problems before the development of AGI. That has something to do with ambitious value learning, which would be like learning the one true human utility function which probably just doesn't exist.
Buck Shlegeris: I think I want to object to a little bit of your framing there. My stance on utility functions of humans isn't that there are a bunch of complicated subtleties on top, it's that modeling humans with utility functions is just a really sad state to be in. If your alignment strategy involves positing that humans behave as expected utility maximizers, I am very pessimistic about it working in the short term, and I just think that we should be trying to completely avoid anything which does that. It's not like there's a bunch of complicated sub-problems that we need to work out about how to describe us as expected utility maximizers, my best guess is that we would just not end up doing that because it's not a good idea.
Lucas Perry: For the ambitious value learning?
Buck Shlegeris: Yeah, that's right.
Lucas Perry: Okay, do you have something that's on offer?
Buck Shlegeris: The two options instead of that, which seem attractive to me? As I said earlier, one is you just convince everyone to not use AI systems for things where you need to have an understanding of large scale human preferences. The other one is the kind of thing that Paul Christiano's iterated distillation and amplification, or a variety of his other ideas, the kind of thing that he's trying to get there is, I think, if you make a really powerful AI system, it's actually going to have an excellent model of human values in whatever representation is best for actually making predictions about humans because a really excellent AGI, like a really excellent paperclip maximizer, it's really important for it to really get how humans work so that it can manipulate them into letting it build lots of paperclip factories or whatever.
So I think that if you think that we have AGI, then by assumption I think we have a system which is able to reason about human values if it wants. And so if we can apply these really powerful AI systems to tasks such that the things that they do display their good understanding of human values, then we're fine and it's just okay that there was no way that we could represent a utility function directly. So for instance, the idea in IDA is that if we could have this system which is just trying to answer questions the same way that humans would, but enormously more cheaply because it can run faster than humans and a few other tricks, then we don't have to worry about writing down a utility functions of humans directly because we can just make the system do things that are kind of similar to the things humans would have done, and so it implicitly has this human utility function built into it. That's option two. Option one is don't use anything that requires a complex human utility function, option two is have your systems learn human values implicitly, by giving them a task such that this is beneficial for them and such that their good understanding of human values comes out in their actions.
Rohin Shah: One way I might condense that point, is that you're asking for a nice formalism for human preferences and I just point to all the humans out there in the world who don't know anything about utility functions, which is 99% of them and nonetheless still seem pretty good at inferring human preferences.
Lucas Perry: On this part about AGI, if it is AGI it should be able to reason about human preferences, then why would it not be able to construct something that was more explicit and thus was able to do more ambitious value learning?
Buck Shlegeris: So it can totally do that, itself. But we can't force that structure from the outside with our own algorithms.
Rohin Shah: Image classification is a good analogy. Like, in the past we were using hand engineered features, namely SIFT and HOG and then training classifiers over these hand engineered features in order to do image classification. And then we came to the era of deep learning and we just said, yeah, throw away all those features and just do everything end to end with a convolutional neural net and it worked way better. The point was that, in fact there are good representations for most tasks and humans trying to write them down ahead of time just doesn't work very well at that. It tends to work better if you let the AI system discover its own representations that best capture the thing you wanted to capture.
Lucas Perry: Can you unpack this point a little bit more? I'm not sure that I'm completely understanding it. Buck is rejecting this modeling human beings explicitly as expected utility maximizers and trying to explicitly come up with utility functions in our AI systems. The first was to convince people not to use these kinds of things. And the second is to make it so that the behavior and output of the AI systems has some implicit understanding of human behavior. Can you unpack this a bit more for me or give me another example?
Rohin Shah: So here's another example. Let's say I was teaching my kid that I don't have, how to catch a ball. It seems that the formalism that's available to me for learning how to catch a ball is, well, you can go all the way down to look at our best models of physics, we could use Newtonian mechanics let's say, like here are these equations, estimate the velocity and the distance of the ball and the angle at which it's thrown plug that into these equations and then predict that the ball's going to come here and then just put your hand there and then magically catch it. We won't even talk about the catching part. That seems like a pretty shitty way to teach a kid how to catch a ball.
Probably it's just a lot better to just play catch with the kid for a while and let the kid's brain figure out this is how to predict where the ball is going to go such that I can predict where it's going to be and then catch it.
I'm basically 100% confident that the thing that the brain is doing is not Newtonian mechanics. It's doing something else that's just way more efficient at predicting where the ball is going to be so that I can catch it and if I forced the brain to use Newtonian mechanics, I bet it would not do very well at this task.
Buck Shlegeris: I feel like that still isn't quite saying the key thing here. I don't know how to say this off the top of my head either, but I think there's this key point about: just because your neural net can learn a particular feature of the world doesn't mean that you can back out some other property of the world by forcing the neural net to have a particular shape. Does that make any sense, Rohin?
Rohin Shah: Yeah, vaguely. I mean, well, no, maybe not.
Buck Shlegeris: The problem isn't just the capabilities problem. There's this way you can try and infer a human utility function by asking, according to this model, what's the maximum likelihood utility function given all these things the human did. If you have a good enough model, you will in fact end up making very good predictions about the human, it's just that the decomposition into their planning function and their utility function is not going to result in a utility function which is anything like a thing that I would want maximized if this process was done on me. There is going to be some decomposition like this, which is totally fine, but the utility function part just isn't going to correspond to the thing that I want.
Rohin Shah: Yeah, that is also a problem, but I agree that is not the thing I was describing.
Lucas Perry: Is the point there that there's a lack of alignment between the utility function and the planning function. Given that the planning function imperfectly optimizes the utility function.
Rohin Shah: It's more like there are just infinitely many possible pairs of planning functions and utility functions that exactly predict human behavior. Even if it were true that humans were expected utility maximizers, which Buck is arguing we're not, and I agree with him. There is a planning function that's like humans are perfectly anti-rational and if you're like what utility function works with that planner to predict human behavior. Well, the literal negative of the true utility function when combined with the anti-rational planner produces the same behavior as the true utility function with the perfect planner, there's no information that lets you distinguish between these two possibilities.
You have to build it in as an assumption. I think Buck's point is that building things in as assumptions is probably not going to work.
Buck Shlegeris: Yeah.
Rohin Shah: A point I agree with. In philosophy this is called the is-ought problem, right? What you can train your AI system on is a bunch of “is” facts and then you have to add in some assumptions in order to jump to “ought” facts, which is what the utility function is trying to do. The utility function is trying to tell you how you ought to behave in new situations and the point of the is-ought distinction is that you need some bridging assumptions in order to get from is to ought.
Buck Shlegeris: And I guess an important part here is your system will do an amazing job of answering “is” questions about what humans would say about “ought” questions. And so I guess maybe you could phrase the second part as: to get your system to do things that match human preferences, use the fact that it knows how to make accurate “is” statements about humans' ought statements?
Lucas Perry: It seems like we're strictly talking about inferring the human utility function or preferences via looking at behavior. What if you also had more access to the actual structure of the human's brain?
Rohin Shah: This is like the approach that Stuart Armstrong likes to talk about. The same things still apply. You still have the is-ought problem where the facts about the brain are "is" facts and how you translate that into "ought" facts is going to involve some assumptions. Maybe you can break down such assumptions that everyone would agree with. Maybe it's like if this particular neuron in a human brain spikes, that's a good thing and we want more of it and if this other one spikes, that's a bad thing. We don't want it. Maybe that assumption is fine.
Lucas Perry: I guess I'm just pointing out, if you could find the places in the human brain that generate the statements about Ought questions.
Rohin Shah: As Buck said, that lets you predict what humans would say about ought statements, which your assumption could then be, whatever humans say about ought statements, that's what you ought to do. And that's still an assumption. Maybe it's a very reasonable assumption that we're happy to put it into our AI system.
Lucas Perry: If we're not willing to accept some humans’ “is” statements about “ought” questions then we have to do some meta-ethical moral policing in our assumptions around getting “is” statements from “ought” questions.
Rohin Shah: Yes, that seems right to me. I don't know how you would do such a thing, but you would have to do something along those lines.
Buck Shlegeris: I would additionally say that I feel pretty great about trying to do things which use the fact that we can trust our AI to have good “is” answers to “ought” questions, but there's a bunch of problems with this. I think it's a good starting point but trying to use that to do arbitrarily complicated things in the world has a lot of problems. For instance, suppose I'm trying to decide whether we should design a city this way or that way. It's hard to know how to go from the ability to know how humans would answer questions about preferences to knowing what you should do to design the city. And this is for a bunch of reasons, one of them is that the human might not be able to figure out from your city building plans what the city's going to actually be like. And another is that the human might give inconsistent answers about what design is good, depending on how you phrase the question, such that if you try to figure out a good city plan by optimizing for the thing that the human is going to be most enthusiastic about, then you might end up with a bad city plan. Paul Christiano has written in a lot of detail about a lot of this.
Lucas Perry: That also reminds me of what Stuart Armstrong wrote about the framing on the questions changing output on the preference.
Rohin Shah: Yep.
Buck Shlegeris: Sorry, to be clear other people than Paul Christiano have also written a lot about this stuff, (including Rohin). My favorite writing about this stuff is by Paul.
Lucas Perry: Yeah, those do seem problematic but it would also seem that there would be further “is” statements that if you queried people's meta-preferences about those things, you would get more “is” statements about that, but then that just pushes the “ought” assumptions that you need to make further back. Getting into very philosophically weedy territory. Do you think that this kind of thing could be pushed to the long reflection as is talked about by William MacAskill and Toby Ord or how much of this do you actually think needs to be solved in order to have safe and aligned AGI?
Buck Shlegeris: I think there are kind of two different ways that you could hope to have good outcomes from AGI. One is: set up a world such that you never needed to make an AGI which can make large scale decisions about the world. And two is: solve the full alignment problem.
I'm currently pretty pessimistic about the second of those being technically feasible. And I'm kind of pretty pessimistic about the first of those being a plan that will work. But in the world where you can have everyone only apply powerful and dangerous AI systems in ways that don't require an understanding of human values, then you can push all of these problems onto the long reflection. In worlds where you can do arbitrarily complicated things in ways that humans would approve of, you don't really need to long reflect this stuff because of the fact that these powerful AI systems already have the capacity of doing portions of the long reflection work inside themselves as needed. (Quotes about the long reflection)
Rohin Shah: Yeah, so I think my take, it's not exactly disagreeing with Buck. It's more like from a different frame as Buck's. If you just got AI systems that did the things that humans did now, this does not seem to me to obviously require solving hard problems in philosophy. That's the lower bound on what you can do before having to do long reflection type stuff. Eventually you do want to do a longer reflection. I feel relatively optimistic about having a technical solution to alignment that allows us to do the long reflection after building AI systems. So the long reflection would include both humans and AI systems thinking hard, reflecting on difficult problems and so on.
Buck Shlegeris: To be clear, I'm super enthusiastic about there being a long reflection or something along those lines.
Lucas Perry: I always find it useful reflecting on just how human beings do many of these things because I think that when thinking about things in the strict AI alignment sense, it can seem almost impossible, but human beings are able to do so many of these things without solving all of these difficult problems. It seems like in the very least, we'll be able to get AI systems that very, very approximately do what is good or what is approved of by human beings because we can already do that.
Buck Shlegeris: That argument doesn't really make sense to me. It also didn't make sense when Rohin referred to it a minute ago.
Rohin Shah: It's not an argument for we technically know how to do this. It is more an argument for this as at least within the space of possibilities.
Lucas Perry: Yeah, I guess that's how I was also thinking of it. It is within the space of possibilities. So utility functions are good because they can be optimized for, and there seem to be risks with optimization. Is there anything here that you guys would like to say about better understanding agency? I know this is one of the things that is important within the MIRI agenda.
Buck Shlegeris: I am a bad MIRI employee. I don't really get that part of the MIRI agenda, and so I'm not going to defend it. I have certainly learned some interesting things from talking to Scott Garrabrant and other MIRI people who have lots of interesting thoughts about this stuff. I don't quite see the path from there to good alignment strategies. But I also haven't spent a super long time thinking about it because I, in general, don't try to think about all of the different AI alignment things that I could possibly think about.
Rohin Shah: Yeah. I also am not a good person to ask about this. Most of my knowledge comes from reading things and MIRI has stopped writing things very much recently, so I don't know what their ideas are. I, like Buck, don't really see a good alignment strategy that starts with, first we understand optimization and so that's the main reason why I haven't looked into it very much.
Buck Shlegeris: I think I don't actually agree with the thing you said there, Rohin. I feel like understanding optimization could plausibly be really nice. Basically the story there is, it's a real bummer if we have to make really powerful AI systems via searching over large recurrent policies for things that implement optimizers. If it turned out that we could figure out some way of coding up optimizer stuffs directly, then this could maybe mean you didn't need to make mesa-optimizers. And maybe this means that your inner alignment problems go away, which could be really nice. The thing that I was saying I haven't thought that much about is, the relevance of thinking about, for instance, the various weirdnesses that happen when you consider embedded agency or decision theory, and things like that.
Rohin Shah: Oh, got it. Yeah. I think I agree that understanding optimization would be great if we succeeded at it and I'm mostly pessimistic about us succeeding at it, but also there are people who are optimistic about it and I don't know why they're optimistic about it.
Lucas Perry: Hey it’s post-podcast Lucas here again. So, I just want to add a little more detail here again on behalf of Rohin. Here he feels pessimistic about us understanding optimization well enough and in a short enough time period that we are able to create powerful optimizers that we understand that rival the performance of the AI systems we’re already building and will build in the near future. Back to the episode.
Buck Shlegeris: The arguments that MIRI has made about this,... they think that there are a bunch of questions about what optimization is, that are plausibly just not that hard compared to other problems which small groups of people have occasionally solved, like coming up with foundations of mathematics, kind of a big conceptual deal but also a relatively small group of people. And before we had formalizations of math, I think it might've seemed as impossible to progress on as formalizing optimization or coming up with a better picture of that. So maybe that's my argument for some optimism.
Rohin Shah: Yeah, I think pointing to some examples of great success does not imply... Like there are probably many similar things that didn't work out and we don't know about them cause nobody bothered to tell us about them because they failed. Seems plausible maybe.
Lucas Perry: So, exploring more deeply this point of agency can either, or both of you, give us a little bit of a picture about the relevance or non relevance of decision theory here to AI alignment and I think, Buck, you mentioned the trickiness of embedded decision theory.
Rohin Shah: If you go back to our traditional argument for AI risk, it's basically powerful AI systems will be very strong optimizers. They will possibly be misaligned with us and this is bad. And in particular one specific way that you might imagine this going wrong is this idea of mesa optimization where we don't know how to build optimizers right now. And so what we end up doing is basically search across a huge number of programs looking for ones that do well at optimization and use that as our AGI system. And in this world, if you buy that as a model of what's happening, then you'll basically have almost no control over what exactly that system is optimizing for. And that seems like a recipe for misalignment. It sure would be better if we could build the optimizer directly and know what it is optimizing for. And in order to do that, we need to know how to do optimization well.
Lucas Perry: What are the kinds of places that we use mesa optimizers today?
Rohin Shah: It's not used very much yet. The field of meta learning is the closest example. In the field of meta learning you have a distribution over tasks and you use gradient descent or some other AI technique in order to find an AI system that itself, once given a new task, learns how to perform that task well.
Existing meta learning systems are more like learning how to do all the tasks well and then when they'll see a new task they just figure out ah, it's this task and then they roll out the policy that they already learned. But the eventual goal for meta learning is to get something that, online, learns how to do the task without having previously figured out how to do that task.
Lucas Perry: Okay, so Rohin did what you say cover embedded decision theory?
Rohin Shah: No, not really. I think embedded decision theory is just, we want to understand optimization. Our current notion of optimization, one way you could formalize it is to say my AI agent is going to have Bayesian belief over all the possible ways that the environment could be. It's going to update that belief over time as it gets observations and then it's going to act optimally with respect to that belief, by maximizing its expected utility. And embedded decision theory basically calls into question the idea that there's a separation between the agent and the environment. In particular I, as a human, couldn't possibly have a Bayesian belief about the entire earth because the entire Earth contains me. I can't have a Bayesian belief over myself so this means that our existing formalization of agency is flawed. It can't capture these things that affect real agents. And embedded decision theory, embedded agency, more broadly, is trying to deal with this fact and have a new formalization that works even in these situations.
Buck Shlegeris: I want to give my understanding of the pitch for it. One part is that if you don't understand embedded agency, then if you try to make an AI system in a hard coded way, like making a hard coded optimizer, traditional phrasings of what an optimizer is, are just literally wrong in that, for example, they're assuming that you have these massive beliefs over world states that you can't really have. And plausibly, it is really bad to try to make systems by hardcoding assumptions that are just clearly false. And so if we want to hardcode agents with particular properties, it would be good if we knew a way of coding the agent that isn't implicitly making clearly false assumptions.
And the second pitch for it is something like when you want to understand a topic, sometimes it's worth looking at something about the topic which you're definitely wrong about, and trying to think about that part until you are less confused about it. When I'm studying physics or something, a thing that I love doing is looking for the easiest question whose answer I don't know, and then trying to just dive in until I have satisfactorily answered that question, hoping that the practice that I get about thinking about physics from answering a question correctly will generalize to much harder questions. I think that's part of the pitch here. Here is a problem that we would need to answer, if we wanted to understand how superintelligent AI systems work, so we should try answering it because it seems easier than some of the other problems.
Lucas Perry: Okay. I think I feel satisfied. The next thing here Rohin in your AI alignment 2018-19 review is value learning. I feel like we've talked a bunch about this already. Is there anything here that you want to say or do you want to skip this?
Rohin Shah: One thing we didn't cover is, if you have uncertainty over what you're supposed to optimize, this turns into an interactive sort of game between the human and the AI agent, which seems pretty good. A priori you should expect that there's going to need to be a lot of interaction between the human and the AI system in order for the AI system to actually be able to do the things that the human wants it to do. And so having formalisms and ideas of where this interaction naturally falls out seems like a good thing.
Buck Shlegeris: I've said a lot of things about how I am very pessimistic about value learning as a strategy. Nevertheless it seems like it might be really good for there to be people who are researching this, and trying to get as good as we can get at improving sample efficiency so that can have your AI systems understand your preferences over music with as little human interaction as possible, just in case it turns out to be possible to solve the hard version of value learning. Because a lot of the engineering effort required to make ambitious value learning work will plausibly be in common with the kinds of stuff you have to do to make these more simple specification learning tasks work out. That's a reason for me to be enthusiastic about people researching value learning even if I'm pessimistic about the overall thing working.
Lucas Perry: All right, so what is robustness and why does it matter?
Rohin Shah: Robustness is one of those words that doesn't super clearly have a definition and people use it differently. Robust agents don't fail catastrophically in situations slightly different from the ones that they were designed for. One example of a case where we see a failure of robustness currently, is in adversarial examples for image classifiers, where it is possible to take an image, make a slight perturbation to it, and then the resulting image is completely misclassified. You take a correctly classified image of a Panda, slightly perturb it such that a human can't tell what the difference is, and then it's classified as a gibbon with 99% confidence. Admittedly this was with an older image classifier. I think you need to make the perturbations a bit larger now in order to get them.
Lucas Perry: This is because the relevant information that it uses are very local to infer panda-ness rather than global properties of the panda?
Rohin Shah: It's more like they’re high frequency features or imperceptible features. There's a lot of controversy about this but there is a pretty popular recent paper that I believe, but not everyone believes, that claims that this was because they're picking up on real imperceptible features that do generalize to the test set, that humans can't detect. That's an example of robustness. Recently people have been applying this to reinforcement learning both by adversarially modifying the observations that agents get and also by training agents that act in the environment adversarially towards the original agent. One paper out of CHAI showed that there's this kick and defend environment where you've got two MuJoCo robots. One of them is kicking a soccer ball. The other one's a goalie, that's trying to prevent the kicker from successfully shooting a goal, and they showed that if you do self play in order to get kickers and defenders and then you take the kicker, you freeze it, you don't train it anymore and you retrain a new defender against this kicker.
What is the strategy that this new defender learns? It just sort of falls to the ground and flaps about in a random looking way and the kicker just gets so confused that it usually fails to even touch the ball and so this is sort of an adversarial example for RL agents now, it's showing that even they're not very robust.
There was also a paper out of DeepMind that did the same sort of thing. For their adversarial attack they learned what sorts of mistakes the agent would make early on in training and then just tried to replicate those mistakes once the agent was fully trained and they found that this helped them uncover a lot of bad behaviors. Even at the end of training.
From the perspective of alignment, it's clear that we want robustness. It's not exactly clear what we want robustness to. This robustness to adversarial perturbations was kind of a bit weird as a threat model. If there is an adversary in the environment they’re probably not going to be restricted to small perturbations. They're probably not going to get white box access to your AI system; even if they did, this doesn't seem to really connect with the AI system as adversarially optimizing against humans story, which is how we get to the x-risk part, so it's not totally clear.
I think on the intent alignment case, which is the thing that I usually think about, you mostly want to ensure that whatever is driving the "motivation" of the AI system, you want that to be very robust. You want it to agree with what humans would want in all situations or at least all situations that are going to come up or something like that. Paul Christiano has written a few blog posts about this that talk about what techniques he's excited about solving that problem, which boil down to interpretability, adversarial training, and improving adversarial training through relaxations of the problem.
Buck Shlegeris: I'm pretty confused about this, and so it's possible what I'm going to say is dumb. When I look at problems with robustness or problems that Rohin put in this robustness category here, I want to divide it into two parts. One of the parts is, things that I think of as capability problems, which I kind of expect the rest of the world will need to solve on its own. For instance, things about safe exploration, how do I get my system to learn to do good things without ever doing really bad things, this just doesn't seem very related to the AI alignment problem to me. And I also feel reasonably optimistic that you can solve it by doing dumb techniques which don't have anything too difficult to them, like you can have your system so that it has a good model of the world that it got from unsupervised learning somehow and then it never does dumb enough things. And also I don't really see that kind of robustness problem leading to existential catastrophes. And the other half of robustness is the half that I care about a lot, which in my mind, is mostly trying to make sure that you succeeded at inner alignment. That is, that the mesa optimizers you've found through gradient descent have goals that actually match your goals.
This is like robustness in the sense that you're trying to guarantee that in every situation, your AI system, as Rohin was saying, is intent aligned with you. It's trying to do the kind of thing that you want. And I worry that, by default, we're going to end up with AI systems not intent aligned, so there exist a bunch of situations they can be put in such that they do things that are very much not what you'd want, and therefore they fail at robustness. I think this is a really important problem, it's like half of the AI safety problem or more, in my mind, and I'm not very optimistic about being able to solve it with prosaic techniques.
Rohin Shah: That sounds roughly similar to what I was saying. Yes.
Buck Shlegeris: I don't think we disagree about this super much except for the fact that I think you seem to care more about safe exploration and similar stuff than I think I do.
Rohin Shah: I think safe exploration's a bad example. I don't know what safe exploration is even trying to solve but I think other stuff, I agree. I do care about it more. One place where I somewhat disagree with you is, you sort of have this point about all these robustness problems are the things that the rest of the world has incentives to figure out, and will probably figure out. That seems true for alignment too, it sure seems like you want your system to be aligned in order to do the things that you actually want. Everyone that has an incentive for this to happen. I totally expect people who aren’t EAs or rationalists or weird longtermists to be working on AI alignment in the future and to some extent even now. I think that's one thing.
Buck Shlegeris: You should say your other thing, but then I want to get back to that point.
Rohin Shah: The other thing is I think I agree with you that it's not clear to me how failures of the robustness of things other than motivation lead to x-risk, but I'm more optimistic than you are that our solutions to those kinds of robustness will help with the solutions to "motivation robustness" or how to make your mesa optimizer aligned.
Buck Shlegeris: Yeah, sorry, I guess I actually do agree with that last point. I am very interested in trying to figure out how to have aligned to mesa optimizers, and I think that a reasonable strategy to pursue in order to get aligned mesa optimizers is trying to figure out how to make your image classifiers robust to adversarial examples. I think you probably won't succeed even if you succeed with the image classifiers, but it seems like the image classifiers are still probably where you should start. And I guess if we can't figure out how to make image classifiers robust to adversarial examples in like 10 years, I'm going to be super pessimistic about the harder robustness problem, and that would be great to know.
Rohin Shah: For what it's worth, my take on the adversarial examples of image classifiers is, we're going to train image classifiers on more data with bigger nets, it's just going to mostly go away. Prediction. I'm laying my cards on the table.
Buck Shlegeris: That's also something like my guess.
Rohin Shah: Okay.
Buck Shlegeris: My prediction is: to get image classifiers that are robust to epsilon ball perturbations or whatever, some combination of larger things and adversarial training and a couple other clever things, will probably mean that we have robust image classifiers in 5 or 10 years at the latest.
Rohin Shah: Cool. And you wanted to return to the other point about the world having incentives to do alignment.
Buck Shlegeris: So I don't quite know how to express this, but I think it's really important which is going to make this a really fun experience for everyone involved. You know how Airbnb... Or sorry, I guess a better example of this is actually Uber drivers. Where I give basically every Uber driver a five star rating, even though some Uber drivers are just clearly more pleasant for me than others, and Uber doesn't seem to try very hard to get around these problems, even though I think that if Uber caused there to be a 30% difference in pay between the drivers who I think of as 75th percentile and the drivers I think of as 25th percentile, this would make the service probably noticeably better for me. I guess it seems to me that a lot of the time the world just doesn't try do kind of complicated things to make systems actually aligned, and it just does hack jobs, and then everyone deals with the fact that everything is unaligned as a result.
To draw this analogy back, I think that we're likely to have the kind of alignment techniques that solve problems that are as simple and obvious as: we should have a way to have rate your hosts on Airbnb. But I'm worried that we won't ever get around to solving the problems that are like, but what if your hosts are incentivized to tell you sob stories such that you give them good ratings, even though actually they were worse than some other hosts. And this is never a big enough deal that people are unilaterally individually incentivized to solve the harder version of the alignment problem, and then everyone ends up using these systems that actually aren't aligned in the strong sense and then we end up in a doomy world. I'm curious if any of that made any sense.
Lucas Perry: Is a simple way to put that we fall into inadequate or an unoptimal equilibrium and then there's tragedy of the commons and bad game theory stuff that happens that keeps us locked and that the same story could apply to alignment?
Buck Shlegeris: Yeah, that's not quite what I mean.
Lucas Perry: Okay.
Rohin Shah: I think Buck's point is that actually Uber or Airbnb could unilaterally, no gains required, make their system better and this would be an improvement for them and everyone else, and they don't do it. There is nothing about equilibrium that is a failure of Uber to do this thing that seems so obviously good.
Buck Shlegeris: I'm not actually claiming that it's better for Uber, I'm just claiming that there is a misalignment there. Plausibly, an Uber exec, if they were listening to this they'd just be like, "LOL, that's a really stupid idea. People would hate it." And then they would say more complicated things like "most riders are relatively price sensitive and so this doesn't matter." And plausibly they're completely right.
Rohin Shah: That's what I was going to say.
Buck Shlegeris: But the thing which feels important to me is something like a lot of the time it's not worth solving the alignment problems at any given moment because something else is a bigger problem to how things are going locally. And this can continue being the case for a long time, and then you end up with everyone being locked in to this system where they never solved the alignment problems. And it's really hard to make people understand this, and then you get locked into this bad world.
Rohin Shah: So if I were to try and put that in the context of AI alignment, I think this is a legitimate reason for being more pessimistic. And the way that I would make that argument is: it sure seems like we are going to decide on what method or path we're going to use to build AGI. Maybe we'll do a bunch of research and decide we're just going to scale up language models or something like this. I don't know. And we will do that before we have any idea of which technique would be easiest to align and as a result, we will be forced to try to align this exogenously chosen AGI technique and that would be harder than if we got to design our alignment techniques and our AGI techniques simultaneously.
Buck Shlegeris: I'm imagining some pretty slow take off here, and I don't imagine this as ever having a phase where we built this AGI and now we need to align it. It's more like we're continuously building and deploying these systems that are gradually more and more powerful, and every time we want to deploy a system, it has to be doing something which is useful to someone. And many of the things which are useful, require things that are kind of like alignment. "I want to make a lot of money from my system that will give advice," and if it wants to give good generalist advice over email, it's going to need to have at least some implicit understanding of human preferences. Maybe we just use giant language models and everything's just totally fine here. A really good language model isn't able to give arbitrarily good aligned advice, but you can get advice that sounds really good from a language model, and I'm worried that the default path is going to involve the most popular AI advice services being kind of misaligned, and just never bothering to fix that. Does that make any more sense?
Rohin Shah: Yeah, I think I totally buy that that will happen. But I think I'm more like as you get to AI systems doing more and more important things in the world, it becomes more and more important that they are really truly aligned and investment in alignment increases correspondingly.
Buck Shlegeris: What's the mechanism by which people realize that they need to put more work into alignment here?
Rohin Shah: I think there's multiple. One is I expect that people are aware, like even in the Uber case, I expect people are aware of the misalignment that exists, but decide that it's not worth their time to fix it. So the continuation of that, people will be aware of it and then they will decide that they should fix it.
Buck Shlegeris: If I'm trying to sell to city governments this language model based system which will give them advice on city planning, it's not clear to me that at any point the city governments are going to start demanding better alignment features. Maybe that's the way that it goes but it doesn't seem obvious that city governments would think to ask that, and --
Rohin Shah: I wasn't imagining this from the user side. I was imagining this from the engineers or designers side.
Buck Shlegeris: Yeah.
Rohin Shah: I think from the user side I would speak more to warning shots. You know, you have your cashier AI system or your waiter AIs and they were optimizing for tips more so than actually collecting money and so they like offer free meals in order to get more tips. At some point one of these AI systems passes all of the internal checks and makes it out into the world and only then does the problem arise and everyone's like, "Oh my God, this is terrible. What the hell are you doing? Make this better."
Buck Shlegeris: There's two mechanisms via which that alignment might be okay. One of them is that researchers might realize that they want to put more effort into alignment and then solve these problems. The other mechanism is that users might demand better alignment because of warning shots. I think that I don't buy that either of these is sufficient. I don't buy that it's sufficient for researchers to decide to do it because in a competitive world, the researchers who realize this is important, if they try to only make aligned products, they are not going to be able to sell them because their products will be much less good than the unaligned ones. So you have to argue that there is demand for the things which are actually aligned well. But for this to work, your users have to be able to distinguish between things that have good alignment properties and those which don't, and this seems really hard for users to do. And I guess, when I try to imagine analogies, I just don't see many examples of people successfully solving problems like this, like businesses making products that are different levels of dangerousness, and then users successfully buying the safe ones.
Rohin Shah: I think usually what happens is you get regulation that forces everyone to be safe. I don't know if it was regulation, but like airplanes are incredibly safe. Cars are incredibly safe.
Buck Shlegeris: Yeah but in this case what would happen is doing the unsafe thing allows you to make enormous amounts of money, and so the countries which don't put in the regulations are going to be massively advantaged compared to ones which don't.
Rohin Shah: Why doesn't that apply for cars and airplanes?
Buck Shlegeris: So to start with, cars in poor countries are a lot less safe. Another thing is that a lot of the effort in making safer cars and airplanes comes from designing them. Once you've done the work of designing it, it's that much more expensive to put your formally-verified 747 software into more planes, and because of weird features of the fact that there are only like two big plane manufacturers, everyone gets the safer planes.
Lucas Perry: So tying this into robustness. The fundamental concern here is about the incentives to make aligned systems that are safety and alignment robust in the real world.
Rohin Shah: I think that's basically right. I sort of see these incentives as existing and the world generally being reasonably good at dealing with high stakes problems.
Buck Shlegeris: What's an example of the world being good at dealing with a high stakes problem?
Rohin Shah: I feel like biotech seems reasonably well handled, relatively speaking,
Buck Shlegeris: Like bio-security?
Rohin Shah: Yeah.
Buck Shlegeris: Okay, if the world handles AI as well as bio-security, there's no way we're okay.
Rohin Shah: Really? I'm aware of ways in which we're not doing bio-security well, but there seem to be ways in which we're doing it well too.
Buck Shlegeris: The nice thing about bio-security is that very few people are incentivized to kill everyone, and this means that it's okay if you're sloppier about your regulations, but my understanding is that lots of regulations are pretty weak.
Rohin Shah: I guess I was more imagining the research community's coordination on this. Surprisingly good.
Buck Shlegeris: I wouldn’t describe it that way.
Rohin Shah: It seems like the vast majority of the research community is onboard with the right thing and like 1% isn’t. Yeah. Plausibly we need to have regulations for that last 1%.
Buck Shlegeris: I think that 99% of the synthetic biology research community is on board with "it would be bad if everyone died." I think that some very small proportion is onboard with things like "we shouldn't do research if it's very dangerous and will make the world a lot worse." I would say like way less than half of synthetic biologists seem to agree with statements like "it's bad to do really dangerous research." Or like, "when you're considering doing research, you consider differential technological development." I think this is just not a thing biologists think about, from my experience talking to biologists.
Rohin Shah: I'd be interested in betting with you on this afterwards.
Buck Shlegeris: Me too.
Lucas Perry: So it seems like it's going to be difficult to come down to a concrete understanding or agreement here on the incentive structures in the world and whether they lead to the proliferation of unaligned AI systems or semi aligned AI systems versus fully aligned AI systems and whether that poses a kind of lock-in, right? Would you say that that fairly summarizes your concern Buck?
Buck Shlegeris: Yeah. I expect that Rohin and I agree mostly on the size of the coordination problem required, or the costs that would be required by trying to do things the safer way. And I think Rohin is just a lot more optimistic about those costs being paid.
Rohin Shah: I think I'm optimistic both about people's ability to coordinate paying those costs and about incentives pointing towards paying those costs.
Buck Shlegeris: I think that Rohin is right that I disagree with him about the second of those as well.
Lucas Perry: Are you interested in unpacking this anymore? Are you happy to move on?
Buck Shlegeris: I actually do want to talk about this for two more minutes. I am really surprised by the claim that humans have solved coordination problems as hard as this one. I think the example you gave is humans doing radically nowhere near well enough. What are examples of coordination problem type things... There was a bunch of stuff with nuclear weapons, where I feel like humans did badly enough that we definitely wouldn't have been okay in an AI situation. There are a bunch of examples of the US secretly threatening people with nuclear strikes, which I think is an example of some kind of coordination failure. I don't think that the world has successfully coordinated on never threaten first nuclear strikes. If we had successfully coordinated on that, I would consider nuclear weapons to be less of a failure, but as it is the US has actually according to Daniel Ellsberg threatened a bunch of people with first strikes.
Rohin Shah: Yeah, I think I update less on specific scenarios and update quite a lot more on, "it just never happened." The sheer amount of coincidence that would be required given the level of, Oh my God, there were close calls multiple times a year for many decades. That seems just totally implausible and it just means that our understanding of what's happening is wrong.
Buck Shlegeris: Again, also the thing I'm imagining is this very gradual takeoff world where people, every year, they release their new most powerful AI systems. And if, in a particular year, AI Corp decided to not release its thing, then AI Corps two and three and four would rise to being one, two and three in total profits instead of two, three and four. In that kind of a world, I feel a lot more pessimistic.
Rohin Shah: I'm definitely imagining more of the case where they coordinate to all not do things. Either by international regulation or via the companies themselves coordinating amongst each other. Even without that, it's plausible that AI Corp one does this. One example I'd give is, Waymo has just been very slow to deploy self driving cars relative to all the other self driving car companies, and my impression is that this is mostly because of safety concerns.
Buck Shlegeris: Interesting and slightly persuasive example. I would love to talk through this more at some point. I think this is really important and I think I haven't heard a really good conversation about this.
Apologies for describing what I think is going wrong inside your mind or something, which is generally a bad way of saying things, but it sounds kind of to me like you're implicitly assuming more concentrated advantage and fewer actors than I think actually are implied by gradual takeoff scenarios.
Rohin Shah: I'm usually imagining something like a 100+ companies trying to build the next best AI system, and 10 or 20 of them being clear front runners or something.
Buck Shlegeris: That makes sense. I guess I don't quite see how the coordination successes you were describing arise in that kind of a world. But I am happy to move on.
Lucas Perry: So before we move on on this point, is there anything which you would suggest as obvious solutions, should Buck's model of the risks here be the case. So it seemed like it would demand more centralized institutions which would help to mitigate some of the lock in here.
Rohin Shah: Yeah. So there's a lot of work in policy and governance about this. Not much of which is public unfortunately. But I think the thing to say is that people are thinking about it and it does sort of look like trying to figure out how to get the world to actually coordinate on things. But as Buck has pointed out, we have tried to do this before and so there's probably a lot to learn from past cases as well. But I am not an expert on this and don't really want to talk as though I were one.
Lucas Perry: All right. So there's lots of governance and coordination thought that kind of needs to go into solving many of these coordination issues around developing beneficial AI. So I think with that we can move along now to scaling to superhuman abilities. So Rohin, what do you have to say about this topic area?
Rohin Shah: I think this is in some sense related to what we were talking about before, you can predict what a human would say, but it's hard to back out true underlying values beneath them. Here the problem is, suppose you are learning from some sort of human feedback about what you're supposed to be doing, the information contained in that tells you how to do whatever the human can do. It doesn't really tell you how to exceed what the human can do without having some additional assumptions.
Now, depending on how the human feedback is structured, this might lead to different things like if the human is demonstrating how to do the task to you, then this would suggest that it would be hard to do the task any better than the human can, but if the human was evaluating how well you did the task, then you can do the task better in a way that the human wouldn't be able to tell was better. Ideally, at some point we would like to have AI systems that can actually do just really powerful, great things, that we are unable to understand all the details of and so we would neither be able to demonstrate or evaluate them.
How do we get to those sorts of AI systems? The main proposals in this bucket are iterated amplification, debate, and recursive reward modeling. So in iterated amplification, we started with an initial policy, and we alternate between amplification and distillation, which increases capabilities and efficiency respectively. This can encode a bunch of different algorithms, but usually amplification is done by decomposing questions into easier sub questions, and then using the agent to answer those sub questions. While distillation can be done using supervised learning or reinforcement learning, so you get these answers that are created by these amplified systems that take a long time to run, and you just train a neural net to very quickly predict the answers without having to do this whole big decomposition thing. In debate, we train an agent through self play in a zero sum game where the agent's goal is to win a question answering debate as evaluated by a human judge. The hope here is that since both sides of the debate can point out flaws in the other side's arguments -- they're both very powerful AI systems -- such a set up can use a human judge to train far more capable agents while still incentivizing the agents to provide honest true information. With recursive reward modeling, you can think of it as an instantiation of the general alternate between amplification and distillation framework, but it works sort of bottom up instead of top down. So you'll start by building AI systems that can help you evaluate simple, easy tasks. Then use those AI systems to help you evaluate more complex tasks and you keep iterating this process until eventually you have AI systems that help you with very complex tasks like how to design the city. And this lets you then train an AI agent that can design the city effectively even though you don't totally understand why it's doing the things it's doing or why they're even good.
Lucas Perry: Do either of you guys have any high level thoughts on any of these approaches to scaling to superhuman abilities?
Buck Shlegeris: I have some.
Lucas Perry: Go for it.
Buck Shlegeris: So to start with, I think it's worth noting that another approach would be ambitious value learning, in the sense that I would phrase these not as approaches for scaling to superhuman abilities, but they're like approaches for scaling to superhuman abilities while only doing tasks that relate to the actual behavior of humans rather than trying to back out their values explicitly. Does that match your thing Rohin?
Rohin Shah: Yeah, I agree. I often phrase that as with ambitious value learning, there's not a clear ground truth to be focusing on, whereas with all three of these methods, the ground truth is what a human would do if they got a very, very long time to think or at least that is what they're trying to approximate. It's a little tricky to see why exactly they're approximating that, but there are some good posts about this. The key difference between these techniques and ambitious value learning is that there is in some sense a ground truth that you are trying to approximate.
Buck Shlegeris: I think these are all kind of exciting ideas. I think they're all kind of better ideas than I expected to exist for this problem a few years ago. Which probably means we should update against my ability to correctly judge how hard AI safety problems are, which is great news, in as much as I think that a lot of these problems are really hard. Nevertheless, I don't feel super optimistic that any of them are actually going to work. One thing which isn't in the elevator pitch for IDA, which is iterated distillation and amplification (and debate), is that you get to hire the humans who are going to be providing the feedback, or the humans whose answers AI systems are going to be trained with. And this is actually really great. Because for instance, you could have this program where you hire a bunch of people and you put them through your one month long training an AGI course. And then you only take the top 50% of them. I feel a lot more optimistic about these proposals given you're allowed to think really hard about how to set it up such that the humans have the easiest time possible. And this is one reason why I'm optimistic about people doing research in factored cognition and stuff, which I'm sure Rohin's going to explain in a bit.
One comment about recursive reward modeling: it seems like it has a lot of things in common with IDA. The main downside that it seems to have to me is that the human is in charge of figuring out how to decompose the task into evaluations at a variety of levels. Whereas with IDA, your system itself is able to naturally decompose the task into a variety levels, and for this reason I feel a bit more optimistic about IDA.
Rohin Shah: With recursive reward modeling, one agent that you can train is just an agent that's good at doing decompositions. That is a thing you can do with it. It's a thing that the people at DeepMind are thinking about.
Buck Shlegeris: Yep, that’s a really good point.
Rohin Shah: I also strongly like the fact that you can train your humans to be good at providing feedback. This is also true about specification learning. It's less clear if it's true about ambitious value learning. No one's really proposed how you could do ambitious value learning really. Maybe arguably Stuart Russell's book is kind of a proposal, but it doesn't have that many details.
Buck Shlegeris: And, for example, it doesn't address any of my concerns in ways that I find persuasive.
Rohin Shah: Right. But for specification learning also you definitely want to train the humans who are going to be providing feedback to the AI system. That is an important part of why you should expect this to work.
Buck Shlegeris: I often give talks where I try to give an introduction to IDA and debate as a proposal for AI alignment. I'm giving these talks to people with computer science backgrounds, and they’re almost always incredibly skeptical that it's actually possible to decompose thought in this kind of a way. And with debate, they're very skeptical that truth wins, or that the nash equilibrium is accuracy. For this reason I'm super enthusiastic about research into the factored cognition hypothesis of the type that Ought is doing some of.
I'm kind of interested in your overall take for how likely it is that the factored cognition hypothesis holds and that it's actually possible to do any of this stuff, Rohin. You could also explain what that is.
Rohin Shah: I'll do that. So basically with both iterated amplification, debate, or recursive reward modeling, they all hinge on this idea of being able to decompose questions, maybe it's not so obvious why that's true for debate, but it's true. Go listen to the podcast about debate if you want to get more details on that.
So this hypothesis is basically for any tasks that we care about, it is possible to decompose this into a bunch of sub tasks that are all easier to do. Such that if you're able to do the sub tasks, then you can do the overall top level tasks and in particular you can iterate this down, building a tree of smaller and smaller tasks until you can get to the level of tasks that a human could do in a day. Or if you're trying to do it very far, maybe tasks that a human can do in a couple of minutes. Whether or not you can actually decompose the task "be an effective CEO" into a bunch of sub tasks that eventually bottom out into things humans can do in a few minutes is totally unclear. Some people are optimistic, some people are pessimistic. It's called the factored cognition hypothesis and Ought is an organization that's studying it.
It sounds very controversial at first and I, like many other people had the intuitive reaction of, 'Oh my God, this is never going to work and it's not true'. I think the thing that actually makes me optimistic about it is you don't have to do what you might call a direct decomposition. You can do things like if your task is to be an effective CEO, your first sub question could be, what are the important things to think about when being a CEO or something like this, as opposed to usually when I think of decompositions I would think of, first I need to deal with hiring. Maybe I need to understand HR, maybe I need to understand all of the metrics that the company is optimizing. Very object level concerns, but the decompositions are totally allowed to also be meta level where you'll spin off a bunch of computation that is just trying to answer the meta level of question of how should I best think about this question at all.
Another important reason for optimism is that based on the structure of iterated amplification, debate and recursive reward modeling, this tree can be gigantic. It can be exponentially large. Something that we couldn't run even if we had all of the humans on Earth collaborating to do this. That's okay. Given how the training process is structured, considering the fact that you can do the equivalent of millennia of person years of effort in this decomposed tree, I think that also gives me more of a, 'okay, maybe this is possible' and that's also why you're able to do all of this meta level thinking because you have a computational budget for it. When you take all of those together, I sort of come up with “seems possible. I don't really know.”
Buck Shlegeris: I think I'm currently at 30-to-50% on the factored cognition thing basically working out. Which isn't nothing.
Rohin Shah: Yeah, that seems like a perfectly reasonable thing. I think I could imagine putting a day of thought into it and coming up with numbers anywhere between 20 and 80.
Buck Shlegeris: For what it's worth, in conversation at some point in the last few years, Paul Christiano gave numbers that were not wildly more optimistic than me. I don't think that the people who are working on this think it's obviously fine. And it would be great if this stuff works, so I'm really in favor of people looking into it.
Rohin Shah: Yeah, I should mention another key intuition against it. We have all these examples of human geniuses like Ramanujan, who were posed very difficult math problems and just immediately get the answer and then you ask them how did they do it and they say, well, I asked myself what should the answer be? And I was like, the answer should be a continued fraction. And then I asked myself which continued fraction and then I got the answer. And you're like, that does not sound very decomposable. It seems like you need these magic flashes of intuition. Those would be the hard cases for factored cognition. It still seems possible that you could do it by both this exponential try a bunch of possibilities and also by being able to discover intuitions that work in practice and just believing them because they work in practice and then applying them to the problem at hand. You could imagine that with enough computation you'd be able to discover such intuitions.
Buck Shlegeris: You can't answer a math problem by searching exponentially much through the search tree. The only exponential power you get from IDA is IDA is letting you specify the output of your cognitive process in such a way that's going to match some exponentially sized human process. As long as that exponentially sized human process was only exponentially sized because it's really inefficient, but is kind of fundamentally not an exponentially sized problem, then your machine learning should be able to speed it up a bunch. But the thing where you search over search strategy is not valid. If that's all you can do, that's not good enough.
Rohin Shah: Searching over search strategies, I agree you can't do, but if you have an exponential search that could be implemented by humans. We know by hypothesis, if you can solve it with a flash of intuition, there is in fact some more efficient way to do it and so whether or not the distillation steps will actually be enough to get to the point where you can do those flashes of intuition. That's an open question.
Buck Shlegeris: This is one of my favorite areas of AI safety research and I would love for there to be more of it. Something I have been floating for a little while is I kind of wish that there was another Ought. It just seems like it would be so good if we had definitive information about the factored cognition hypothesis. And it also it seems like the kind of thing which is potentially parallelizable. And I feel like I know a lot of people who love talking about how thinking works. A lot of rationalists are really into this. I would just be super excited for some of them to form teams of four and go off on their own and build an Ought competitor. I feel like this is the kind of thing where plausibly, a bunch of enthusiastic people could make progress on their own.
Rohin Shah: Yeah, I agree with that. Definitely seems like one of the higher value things but I might be more excited about universality.
Lucas Perry: All right, well let's get started with universality then. What is universality and why are you optimistic about it?
Rohin Shah: So universality is hard to explain well, in a single sentence. For whatever supervisor is training our agent, you want that supervisor to “know everything the agent knows.” In particular if the agent comes up with some deceptive strategy to look like it's achieving the goal, but actually it hasn't. The supervisors should know that it was doing this deceptive strategy for the reason of trying to trick the supervisor and so the supervisor can then penalize it. The classic example of why this is important and hard also due to Paul Christiano is plagiarism. Suppose you are training on the AI system to produce novel works of literature and as part of its training data, the AI system gets to read this library of a million books.
It's possible that this AI system decides, Hey, you know the best way I can make a great novel seeming book is to just take these five books and take out plot points, passages from each of them and put them together and then this new book will look totally novel and will be very good because I used all of the best Shakespearean writing or whatever. If your supervisor doesn't know that the agent has done this, the only way the supervisor can really check is to go read the entire million books. Even if the agent only read 10 books and so then the supervision becomes a way more costly than running the agent, which is not a great state to be in, and so what you really want is that if the agent does this, the supervisor is able to say, I see that you just copied this stuff over from these other books in order to trick me into thinking that you had written something novel that was good.
That's bad. I'm penalizing you. Stop doing that in the future. Now, this sort of property, I mean it's very nice in the abstract, but who knows whether or not we can actually build it in practice. There's some reason for optimism that I don't think I can adequately convey, but I wrote a newsletter summarizing some of it sometime ago, but again, reading through the posts I became more optimistic that it was an achievable property, than when I first heard what the property was. The reason I'm optimistic about it is that it just sort of seems to capture the thing that we actually care about. It's not everything, like it doesn't solve the robustness problem. Universality only tells you what the agent's currently doing. You know all the facts about that. Whereas for robustness you want to say even in these hypothetical situations that the agent hasn't encountered yet and doesn't know stuff about, even when it encounters those situations, it's going to stay aligned with you so universality doesn't get you all the way there, but it definitely feels like it's getting you quite a bit.
Buck Shlegeris: That's really interesting to hear you phrase it that way. I guess I would have thought of universality as a subset of robustness. I'm curious what you think of that first.
Rohin Shah: I definitely think you could use universality to achieve a subset of robustness. Maybe I would say universality is a subset of interpretability.
Buck Shlegeris: Yeah, and I care about interpretability as a subset of robustness basically, or as a subset of inner alignment, which is pretty close to robustness in my mind. The other thing I would say is you were saying there that one difference between universality and robustness is that universality only tells you why the agent did the thing it currently did, and this doesn't suffice to tell us about the situations that the agent isn't currently in. One really nice thing though is that if the agent is only acting a particular way because it wants you to trust it, that's a fact about its current behavior that you will know, and so if you have the universality property, your overseer just knows your agent is trying to deceive it. Which seems like it would be incredibly great and would resolve like half of my problem with safety if you had it.
Rohin Shah: Yeah, that seems right. The case that universality doesn't cover is when your AI system is initially not deceptive, but then at some point in the future it's like, 'Oh my God, now it's possible to go and build Dyson spheres or something, but wait, in this situation probably I should be doing this other thing and humans won't like that. Now I better deceive humans'. The transition into deception would have to be a surprise in some sense even to the AI system.
Buck Shlegeris: Yeah, I guess I'm just not worried about that. Suppose I have this system which is as smart as a reasonably smart human or 10 reasonably smart humans, but it's not as smart as the whole world. If I can just ask it what its best sense about how aligned it is, is? And if I can trust its answer? I don't know man, I'm pretty okay with systems that think they're aligned, answering that question honestly.
Rohin Shah: I think I somewhat agree. I like this reversal where I'm the pessimistic one.
Buck Shlegeris: Yeah me too. I'm like, "look, system, I want you to think as hard as you can to come up with the best arguments you can come up with for why you are misaligned, and the problems with you." And if I just actually trust the system to get this right, then the bad outcomes I get here are just pure accidents. I just had this terrible initialization of my neural net parameters, such that I had this system that honestly believed that it was going to be aligned. And then as it got trained more, this suddenly changed and I couldn't do anything about it. I don't quite see the story for how this goes super wrong. It seems a lot less bad than the default situation.
Rohin Shah: Yeah. I think the story I would tell is something like, well, if you look at humans, they're pretty wrong about what their preferences will be in the future. For example, there's this trope of how teenagers fall in love and then fall out of love, but when they're in love, they swear undying oaths to each other or something. To the extent that is true, that seems like the sort of failure that could lead to x-risk if it also happened with AI systems.
Buck Shlegeris: I feel pretty optimistic about all the garden-variety approaches to solving this. Teenagers were not selected very hard on accuracy of their undying oaths. And if you instead had accuracy of self-model as a key feature you were selecting for in your AI system, plausibly you'll just be way more okay.
Rohin Shah: Yeah. Maybe people could coordinate well on this. I feel less good about people coordinating on this sort of problem.
Buck Shlegeris: For what it's worth, I think there are coordination problems here and I feel like my previous argument about why coordination is hard and won't happen by default also probably applies to us not being okay. I'm not sure how this all plays out. I'd have to think about it more.
Rohin Shah: Yeah. I think it's more like this is a subtle and non-obvious problem, which by hypothesis doesn't happen in the systems you actually have and only happens later and those are the sorts of problems I'm like, Ooh, not sure if we can deal with those ones, but I agree that there's a good chance that there's just not a problem at all in the world where we already have universality and checked all the obvious stuff.
Buck Shlegeris: Yeah. I would like to say universality is one of my other favorite areas of AI alignment research, in terms of how happy I'd be if it worked out really well.
Lucas Perry: All right, so let's see if we can slightly pick up the pace here. Moving forward and starting with interpretability.
Rohin Shah: Yeah, so I mean I think we've basically discussed interpretability already. Universality is a specific kind of interpretability, but the case for interpretability is just like, sure seems like it would be good if you could understand what your AI systems are doing. You could then notice when they're not aligned, and fix that somehow. It's a pretty clear cut case for a thing that would be good if we achieved it and it's still pretty uncertain how likely we are to be able to achieve it.
Lucas Perry: All right, so let's keep it moving and let's hit impact regularization now.
Rohin Shah: Yeah, impact regularization in particular is one of the ideas that are not trying to align the AI system but are instead trying to say, well, whatever AI system we build, let's make sure it doesn't cause a catastrophe. It doesn't lead to extinction or existential risk. What it hopes to do is say, all right, AI system, do whatever it is you wanted to do. I don't care about that. Just make sure that you don't have a huge impact upon the world.
Whatever you do, keep your impact not too high. And so there's been a lot of work on this in recent years there's been relative reachability, attainable utility preservation, and I think in general the sense is like, wow, it's gone quite a bit further than people expected it to go. I think it definitely does prevent you from doing very, very powerful things of the sort, like if you wanted to stop all competing AI projects from ever being able to build AGI, that doesn't seem like the sort of thing you can do with an impact regularized AI system, but it sort of seems plausible that you could prevent convergent instrumental sub goals using impact regularization. Where AI systems that are trying to steal resources and power from humans, you could imagine that you'd say, hey, don't do that level of impact, you can still have the level of impact of say running a company or something like that.
Buck Shlegeris: My take on all this is that I'm pretty pessimistic about all of it working. I think that impact regularization or whatever is a non-optimal point on the capabilities / alignment trade off or something, in terms of safety you're getting for how much capability you're sacrificing. My basic a problem here is basically analogous to my problem with value learning, where I think we're trying to take these extremely essentially fuzzy concepts and then factor our agent through these fuzzy concepts like impact, and basically the thing that I imagine happening is any impact regularization strategy you try to employ, if your AI is usable, will end up not helping with its alignment. For any definition of impacts you come up with, it'll end up doing something which gets around that. Or it'll make your AI system completely useless, is my basic guess as to what happens.
Rohin Shah: Yeah, so I think again in this setting, if you formalize it and then say, consider the optimal agent. Yeah, that can totally get around your impact penalty, but in practice it sure seems like, what you want to do is say this convergent instrumental subgoal stuff, don't do any of that. Continue to do things that are normal in regular life. And those seem like pretty distinct categories. Such that I would not be shocked if we could actually distinguish between the two.
Buck Shlegeris: It sounds like the main benefit you're going for is trying to make your AI system not do insane, convergent, instrumental sub-goal style stuff. So another approach I can imagine taking here would be some kind of value learning or something, where you're asking humans for feedback on whether plans are insanely convergent, instrumental sub-goal style, and just not doing the things which, when humans are asked to rate how sketchy the plans are the humans rate as sufficiently sketchy? That seems like about as good a plan. I'm curious what you think.
Rohin Shah: The idea of power as your attainable utility across a wide variety of utility functions seems like a pretty good formalization to me. I think in the worlds where I actually buy a formalization, I tend to expect the formalization to work better. I do think the formalization is not perfect. Most notably with the current formalization of power, your power never changes if you have extremely good beliefs. Your notion, you're just like, I always have the same power because I'm always able to do the same things and you never get surprised, so maybe I agree with you because I think the current formalization is not good enough. (The strike through section has been redacted by Rohin. It's incorrect and you can see why here.) Yeah, I think I agree with you but I could see it going either way.
Buck Shlegeris: I could be totally wrong about this, and correct me if I'm wrong, my sense is that you have to be able to back out the agent's utility function or its models of the world. Which seems like it's assuming a particular path for AI development which doesn't seem to me particularly likely.
Rohin Shah: I definitely agree with that for all the current methods too.
Buck Shlegeris: So it's like: assume that we have already perfectly solved our problems with universality and robustness and transparency and whatever else. I feel like you kind of have to have solved all of those problems before you can do this, and then you don't need it or something.
Rohin Shah: I don't think I agree with that. I definitely agree that the current algorithms that people have written assume that you can just make a change to the AI's utility function. I don't think that's what even their proponents would suggest as the actual plan.
Buck Shlegeris: What is the actual plan?
Rohin Shah: I don't actually know what their actual plan would be, but one plan I could imagine is figure out what exactly the conceptual things we have to do with impact measurement are, and then whatever method we have for building AGI, probably there's going to be some part which is specify the goal and then in the specify goal part, instead of just saying pursue X, we want to say pursue X without changing your ability to pursue Y, and Z and W, and P, and Q.
Buck Shlegeris: I think that that does not sound like a good plan. I don't think that we should expect our AI systems to be structured that way in the future.
Rohin Shah: Plausibly we have to do this with natural language or something.
Buck Shlegeris: It seems very likely to me that the thing you do is reinforcement learning where at the start of the episode you get a sentence of English which is telling you what your goal is and then blah, blah, blah, blah, blah, and this seems like a pretty reasonable strategy for making powerful and sort of aligned AI. Aligned enough to be usable for things that aren't very hard. But you just fundamentally don't have access to the internal representations that the AI is using for its sense of what belief is, and stuff like that. And that seems like a really big problem.
Rohin Shah: I definitely see this as more of an outer alignment thing, or like an easier to specify outer alignment type thing than say, IDA is that type stuff.
Buck Shlegeris: Okay, I guess that makes sense. So we're just like assuming we've solved all the inner alignment problems?
Rohin Shah: In the story so far yeah, I think all of the researchers who actually work on this haven't thought much about inner alignment.
Buck Shlegeris: My overall summary is that I really don't like this plan. I feel like it's not robust to scale. As you were saying Rohin, if your system gets more and more accurate beliefs, stuff breaks. It just feels like the kind of thing that doesn't work.
Rohin Shah: I mean, it's definitely not conceptually neat and elegant in the sense of it's not attacking the underlying problem. And in a problem setting where you expect adversarial optimization type dynamics, conceptual elegance actually does count for quite a lot in whether or not you believe your solution will work.
Buck Shlegeris: I feel it’s like trying to add edge detectors to your image classifiers to make them more adversarily robust or something, which is backwards.
Rohin Shah: Yeah, I think I agree with that general perspective. I don't actually know if I'm more optimistic than you. Maybe I just don't say... Maybe we'd have the same uncertainty distributions and you just say yours more strongly or something.
Lucas Perry: All right, so then let's just move a little quickly through the next three, which are causal modeling, oracles, and decision theory.
Rohin Shah: Yeah, I mean, well decision theory, MIRI did some work on it. I am not the person to ask about it, so I'm going to skip that one. Even if you look at the long version, I'm just like, here are some posts. Good luck. So causal modeling, I don't fully understand what the overall story is here but the actual work that's been published is basically what we can do is we can take potential plans or training processes for AI systems. We can write down causal models that tell us how the various pieces of the training system interact with each other and then using algorithms developed for causal models we can tell when an AI system would have an incentive to either observe or intervene on an underlying variable.
One thing that came out of this was that you can build a model-based reinforcement learner that doesn't have any incentive to wire head as long as when it makes its plans, the plans are evaluated by the current reward function as opposed to whatever future reward function it would have. And that was explained using this framework of causal modeling. Oracles, Oracles are basically the idea that we can just train an AI system to just answer questions, give it a question and it tries to figure out the best answer it can to that question, prioritizing accuracy.
One worry that people have recently been talking about is the predictions that the Oracle makes then affect the world, which can affect whether or not the prediction was correct. Like maybe if I predict that I will go to bed at 11 then I'm more likely to actually go to bed at 11 because I want my prediction to come true or something and so then the Oracles can still “choose” between different self confirming predictions and so that gives them a source of agency and one way that people want to avoid this is using what are called counter-factual Oracles where you set up the training, such that the Oracles are basically making predictions under the assumption that their predictions are not going to influence the future.
Lucas Perry: Yeah, okay. Oracles seem like they just won't happen. There'll be incentives to make things other than Oracles and that Oracles would even be able to exert influence upon the world in other ways.
Rohin Shah: Yeah, I think I agree that Oracles do not seem very competitive.
Lucas Perry: Let's do forecasting now then.
Rohin Shah: So the main sub things within forecasting one, there's just been a lot of work recently on actually building good forecasting technology. There has been an AI specific version of Metaculus that's been going on for a while now. There's been some work at the Future of Humanity Institute on building better tools for working with probability distributions under recording and evaluating forecasts. There was an AI resolution council where basically now you can make forecasts about what this particular group of people will think in five years or something like that, which is much easier to operationalize than most other kinds of forecasts. So this helps with constructing good questions. On the actual object level, I think there are two main things. One is that it became increasingly more obvious in the past two years that AI progress currently is being driven by larger and larger amounts of compute.
It totally could be driven by other things as well, but at the very least, compute is a pretty important factor. And then takeoff speeds. So there's been this long debate in the AI safety community over whether -- to take the extremes, whether or not we should expect that AI capabilities will see a very sharp spike. So initially, your AI capabilities are improving by like one unit a year, maybe then with some improvements it got to two units a year and then for whatever reason, suddenly they're now at 20 units a year or a hundred units a year and they just swoop way past what you would get by extrapolating past trends, and so that's what we might call a discontinuous takeoff. If you predict that that won't happen instead you'll get AI that's initially improving at one unit per year. Then maybe two units per year, maybe three units per year. Then five units per year, and the rate of progress continually increases. The world's still gets very, very crazy, but in a sort of gradual, continuous way that would be called a continuous takeoff.
Basically there were two posts that argued pretty forcefully for continuous takeoff back in, I want to say February of 2018, and this at least made me believe that continuous takeoff was more likely. Sadly, we just haven't actually seen much defense of the other side of the view since then. Even though we do know that there definitely are people who still believe the other side, that there will be a discontinuous takeoff.
Lucas Perry: Yeah so what are both you guys' views on them?
Buck Shlegeris: Here are a couple of things. One is that I really love the operationalization of slow take off or continuous take off that Paul provided in his post, which was one of the ones Rohin was referring to from February 2018. He says, “by slow takeoff, I mean that there is a four year doubling of the economy before there is a one year doubling of the economy.” As in, there's a period of four years over which world GDP increases by a factor four, after which there is a period of one year. As opposed to a situation where the first one-year doubling happens out of nowhere. Currently, doubling times for the economy are on the order of like 20 years, and so a one year doubling would be a really big deal. The way that I would phrase why we care about this, is because worlds where we have widespread, human level AI feel like they have incredibly fast economic growth. And if it's true that we expect AI progress to increase gradually and continuously, then one important consequence of this is that by the time we have human level AI systems, the world is already totally insane. A four year doubling would just be crazy. That would be economic growth drastically higher than economic growth currently is.
This means it would be obvious to everyone who's paying attention that something is up and the world is radically changing in a rapid fashion. Another way I've been thinking about this recently is people talk about transformative AI, by which they mean AI which would have at least as much of an impact on the world as the industrial revolution had. And it seems plausible to me that octopus level AI would be transformative. Like suppose that AI could just never get better than octopus brains. This would be way smaller of a deal than I expect AI to actually be, but it would still be a massive deal, and would still possibly lead to a change in the world that I would call transformative. And if you think this is true, and if you think that we're going to have octopus level AI before we have human level AI, then you should expect that radical changes that you might call transformative have happened by the time that we get to the AI alignment problems that we've been worrying about. And if so, this is really big news.
When I was reading about this stuff when I was 18, I was casually imagining that the alignment problem is a thing that some people have to solve while they're building an AGI in their lab while the rest of the world's ignoring them. But if the thing which is actually happening is the world is going insane around everyone, that's a really important difference.
Rohin Shah: I would say that this is probably the most important contested question in AI alignment right now. Some consequences of it are in a gradual or continuous takeoff world you expect that by the time we get to systems that can pose an existential risk. You've already had pretty smart systems that have been deployed in the real world. They probably had some failure modes. Whether or not we call them alignment failure modes or not is maybe not that important. The point is people will be aware that AI systems can fail in weird ways, depending on what sorts of failures you expect, you might expect this to lead to more coordination, more involvement in safety work. You might also be more optimistic about using testing and engineering styles of approaches to the problem which rely a bit more on trial and error type of reasoning because you actually will get a chance to see errors before they happen at a super intelligent existential risk causing mode. There are lots of implications of this form that pretty radically change which alignment plans you think are feasible.
Buck Shlegeris: Also, it's pretty radically changed how optimistic you are about this whole AI alignment situation, at the very least, people who are very optimistic about AI alignment causing relatively small amounts of existential risk. A lot of the reason for this seems to be that they think that we're going to get these warning shots where before we have superintelligent AI, we have sub-human level intelligent AI with alignment failures like the cashier Rohin was talking about earlier. And then people start caring about AI alignment a lot more. So optimism is also greatly affected by what you think about this.
I've actually been wanting to argue with people about this recently. I wrote a doc last night where I was arguing that even in gradual takeoff worlds, we should expect a reasonably high probability of doom if we can't solve the AI alignment problem. And I'm interested to have this conversation in more detail with people at some point. But yeah, I agree with what Rohin said.
Overall on takeoff speeds, I guess I still feel pretty uncertain. It seems to me that currently, what we can do with AI, like AI capabilities are increasing consistently, and a lot of this comes from applying relatively non-mindblowing algorithmic ideas to larger amounts of compute and data. And I would be kind of surprised if you can't basically ride this wave away until you have transformative AI. And so if I want to argue that we're going to have fast takeoffs, I kind of have to argue that there's some other approach you can take which lets you build AI without having to go along that slow path, which also will happen first. And I guess I think it's kind of plausible that that is what's going to happen. I think that's what you'd have to argue for if you want to argue for a fast take off.
Rohin Shah: That all seems right to me. I'd be surprised if, out of nowhere, we saw a new AI approach suddenly started working and overtook deep learning. You also have to argue that it then very quickly reaches human level AI, which would be quite surprising, right? In some sense, it would have to be something completely novel that we failed to think about in the last 60 years. We're putting in way more effort now than we were in the last 60 years, but then counter counterpoint is that all of that extra effort is going straight into deep learning. It's not really searching for completely new paradigm-shifting ways to get to AGI.
Buck Shlegeris: So here's how I'd make that argument. Perhaps a really important input into a field like AI, is the number of really smart kids who have been wanting to be an AI researcher since they were 16 because they thought that it's the most important thing in the world. I think that in physics, a lot of the people who turn into physicists have actually wanted to be physicists forever. I think the number of really smart kids who wanted to be AI researchers forever has possibly gone up by a factor of 10 over the last 10 years, it might even be more. And there just are problems sometimes, that are bottle necked on that kind of a thing, probably. And so it wouldn't be totally shocking to me, if as a result of this particular input to AI radically increasing, we end up in kind of a different situation. I haven't quite thought through this argument fully.
Rohin Shah: Yeah, the argument seems plausible. There's a large space of arguments like this. I think even after that, then I've started questioning, "Okay, we get a new paradigm. The same arguments apply to that paradigm?" Not as strongly. I guess not the arguments you were saying about compute going up over time, but the arguments given in the original slow takeoff posts which were people quickly start taking the low-hanging fruit and then move on. When there's a lot of effort being put into getting some property, you should expect that easy low-hanging fruit is usually just already taken, and that's why you don't expect discontinuities. Unless the new idea just immediately rockets you to human-level AGI, or x-risk causing AGI, I think the same argument would pretty quickly start applying to that as well.
Buck Shlegeris: I think it's plausible that you do get rocketed pretty quickly to human-level AI. And I agree that this is an insane sounding claim.
Rohin Shah: Great. As long as we agree on that.
Buck Shlegeris: Something which has been on my to-do list for a while, and something I've been doing a bit of and I'd be excited for someone else doing more of, is reading the history of science and getting more of a sense of what kinds of things are bottlenecked by what, where. It could lead me to be a bit less confused about a bunch of this stuff. AI Impacts has done a lot of great work cataloging all of the things that aren't discontinuous changes, which certainly is a strong evidence to me against my claim here.
Lucas Perry: All right. What is the probability of AI-induced existential risk?
Rohin Shah: Unconditional on anything? I might give it 1 in 20. 5%.
Buck Shlegeris: I'd give 50%.
Rohin Shah: I had a conversation with AI Impacts that went into this in more detail and partially just anchored on the number I gave there, which was 10% conditional on no intervention from longtermists, I think the broad argument is really just the one that Buck and I were disagreeing about earlier, which is to what extent will society be incentivized to solve the problem? There's some chance that the first thing we try just works and we don't even need to solve any sort of alignment problem. It might just be fine. This is not implausible to me. Maybe that's 30% or something.
Most of the remaining probability comes from, "Okay, the alignment problem is a real problem. We need to deal with it." It might be very easy in which case we can just solve it straight away. That might be the case. That doesn't seem that likely to me if it was a problem at all. But what we will get is a lot of these warning shots and people understanding the risks a lot more as we get more powerful AI systems. This estimate is also conditional on gradual takeoff. I keep forgetting to say that, mostly because I don't know what probability I should put on discontinuous takeoff.
Lucas Perry: So is 5% with longtermist intervention, increasing to 10% if fast takeoff?
Rohin Shah: Yes, but still with longtermist intervention. I'm pretty pessimistic on fast takeoff, but my probability assigned to fast takeoff is not very high. In a gradual takeoff world, you get a lot of warning shots. There will just generally be awareness of the fact that the alignment problem is a real thing and you won't have the situation you have right now of people saying this thing about worrying about superintelligent AI systems not doing what we want is totally bullshit. That won't be a thing. Almost everyone will not be saying that anymore, in the version where we're right and there is a problem. As a result, people will not want to build AI systems that are going to kill them. People tend to be pretty risk averse in my estimation of the world, which Buck will probably disagree with. And as a result, you'll get a lot of people trying to actually work on solving the alignment problem. There'll be some amount of global coordination which will give us more time to solve the alignment problem than we may otherwise have had. And together, these forces mean that probably we'll be okay.
Buck Shlegeris: So I think my disagreements with Rohin are basically that I think fast takeoffs are more likely. I basically think there is almost surely a problem. I think that alignment might be difficult, and I'm more pessimistic about coordination. I know I said four things there, but I actually think of this as three disagreements. I want to say that "there isn't actually a problem" is just a kind of "alignment is really easy to solve." So then there's three disagreements. One is gradual takeoff, another is difficulty of solving competitive prosaic alignment, and another is how good we are at coordination.
I haven't actually written down these numbers since I last changed my mind about a lot of the inputs to them, so maybe I'm being really dumb. I guess, it feels to me that in fast takeoff worlds, we are very sad unless we have competitive alignment techniques, and so then we're just only okay if we have these competitive alignment techniques. I guess I would say that I'm something like 30% on us having good competitive alignment techniques by the time that it's important, which incidentally is higher than Rohin I think.
Rohin Shah: Yeah, 30 is totally within the 25th to 75th interval on the probability, which is a weird thing to be reporting. 30 might be my median, I don't know.
Buck Shlegeris: To be clear, I'm not just including the outer alignment proportion here, which is what we were talking about before with IDA. I'm also including the inner alignment.
Rohin Shah: Yeah, 30% does seem a bit high. I think I'm a little more pessimistic.
Buck Shlegeris: So I'm like 30% that we can just solve the AI alignment problem in this excellent way, such that anyone who wants to can have very little extra cost and then make AI systems that are aligned. I feel like in worlds where we did that, it's pretty likely that things are reasonably okay. I think that the gradual versus fast takeoff isn't actually enormously much of a crux for me because I feel like in worlds without competitive alignment techniques and gradual takeoff, we still have a very high probability of doom. And I think that comes down to disagreements about coordination. So maybe the main important disagreement between Rohin and I is, actually how well we'll be able to coordinate, or how strongly individual incentives will be for alignment.
Rohin Shah: I think there are other things. The reason I feel a bit more pessimistic than you in the fast takeoff world is just solving problems in advance just is really quite difficult and I really like the ability to be able to test techniques on actual AI systems. You'll have to work with less powerful things. At some point, you do have to make the jump to more powerful things. But, still, being able to test on the less powerful things, that’s so good, so much safety from there.
Buck Shlegeris: It's not actually clear to me that you get to test the most important parts of your safety techniques. So I think that there are a bunch of safety problems that just do not occur on dog-level AIs, and do occur on human-level AI. If there are three levels of AI, there's a thing which is as powerful as a dog, there's a thing which is as powerful as a human, and there's a thing which is as powerful as a thousand John von Neumanns. In gradual takeoff world, you have a bunch of time in both of these two milestones, maybe. I guess it's not super clear to me that you can use results on less powerful systems as that much evidence about whether your safety techniques work on drastically more powerful systems. It's definitely somewhat helpful.
Rohin Shah: It depends what you condition on in your difference between continuous takeoff and discontinuous takeoff to say which one of them happens faster. I guess the delta between dog and human is definitely longer in gradual takeoff for sure. Okay, if that's what you were saying, yep, I agree with that.
Buck Shlegeris: Yeah, sorry, that's all I meant.
Rohin Shah: Cool. One thing I wanted to ask is when you say dog-level AI assistant, do you mean something like a neural net that if put in a dog's body replacing its brain would do about as well as a dog? Because such a neural net could then be put in other environments and learn to become really good at other things, probably superhuman at many things that weren't in the ancestral environment. Do you mean that sort of thing?
Buck Shlegeris: Yeah, that's what I mean. Dog-level AI is probably much better than GPT2 at answering questions. I'm going to define something as dog-level AI, if it's about as good as a dog at things which I think dogs are pretty heavily optimized for, like visual processing or motor control in novel scenarios or other things like that, that I think dogs are pretty good at.
Rohin Shah: Makes sense. So I think in that case, plausibly, dog-level AI already poses an existential risk. I can believe that too.
Buck Shlegeris: Yeah.
Rohin Shah: The AI cashier example feels like it could totally happen probably before a dog-level AI. You've got all of the motivation problems already at that point of the game, and I don't know what problems you expect to see beyond then.
Buck Shlegeris: I'm more talking about whether you can test your solutions. I'm not quite sure how to say my intuitions here. I feel like there are various strategies which work for corralling dogs and which don't work for making humans do what you want. In as much as your alignment strategy is aiming at a flavor of problem that only occurs when you have superhuman things, you don't get to test that either way. I don't think this is a super important point unless you think it is. I guess I feel good about moving on from here.
Rohin Shah: Mm-hmm (affirmative). Sounds good to me.
Lucas Perry: Okay, we've talked about what you guys have called gradual and fast takeoff scenarios, or continuous and discontinuous. Could you guys put some probabilities down on the likelihood of, and stories that you have in your head, for fast and slow takeoff scenarios?
Rohin Shah: That is a hard question. There are two sorts of reasoning I do about probabilities. One is: use my internal simulation of whatever I'm trying to predict, internally simulate what it looks like, whether it's by my own models, is it likely? How likely is it? At what point would I be willing to bet on it. Stuff like that. And then there's a separate extra step where I'm like, "What do other people think about this? Oh, a lot of people think this thing that I assigned one percent probability to is very likely. Hmm, I should probably not be saying one percent then." I don't know how to do that second part for, well, most things but especially in this setting. So I'm going to just report Rohin's model only, which will predictably be understating the probability for fast takeoff in that if someone from MIRI were to talk to me for five hours, I would probably say a higher number for the probability of fast takeoff after that, and I know that that's going to happen. I'm just going to ignore that fact and report my own model anyway.
On my own model, it's something like in worlds where AGI happens soon, like in the next couple of decades, then I'm like, "Man, 95% on gradual take off." If it's further away, like three to five decades, then I'm like, "Some things could have changed by then, maybe I'm 80%." And then if it's way off into the future and centuries, then I'm like, "Ah, maybe it's 70%, 65%." The reason it goes down over time is just because it seems to me like if you want to argue for discontinuous takeoff, you need to posit that there's some paradigm change in how AI progress is happening and that seems more likely the further in the future you go.
Buck Shlegeris: I feel kind of surprised that you get so low, like to 65% or 70%. I would have thought that those arguments are a strong default and then maybe at the moment where in a position that seems particularly gradual takeoff-y, but I would have thought that you over time get to 80% or something.
Rohin Shah: Yeah. Maybe my internal model is like, "Holy shit, why do these MIRI people keep saying that discontinuous takeoff is so obvious." I agree that the arguments in Paul's posts feel very compelling to me and so maybe I should just be more confident in them. I think saying 80%, even in centuries is plausibly a correct answer.
Lucas Perry: So, Rohin, is the view here that since compute is the thing that's being leveraged to make most AI advances that you would expect that to be the mechanism by which that continues to happen in the future and we have some certainty over how compute continues to change into the future? Whereas things that would be leading to a discontinuous takeoff would be world-shattering, fundamental insights into algorithms that would have powerful recursive self-improvement, which is something you wouldn't necessarily see if we just keep going this leveraging compute route?
Rohin Shah: Yeah, I think that's a pretty good summary. Again, on the backdrop of the default argument for this is people are really trying to build AGI. It would be pretty surprising if there is just this really important thing that everyone had just missed.
Buck Shlegeris: It sure seems like in machine learning when I look at the things which have happened over the last 20 years, all of them feel like the ideas are kind of obvious or someone else had proposed them 20 years earlier. ConvNets were proposed 20 years before they were good on ImageNet, and LSTMs were ages before they were good for natural language, and so on and so on and so on. Other subjects are not like this, like in physics sometimes they just messed around for 50 years before they knew what was happening. I don't know, I feel confused how to feel about the fact that in some subjects, it feels like they just do suddenly get better at things for reasons other than having more compute.
Rohin Shah: I think physics, at least, was often bottlenecked by measurements, I want to say.
Buck Shlegeris: Yes, so this is one reason I've been interested in history of science recently, but there are certainly a bunch of things. People were interested in chemistry for a long time and it turns out that chemistry comes from quantum mechanics and you could, theoretically, have guessed quantum mechanics 70 years earlier than people did if you were smart enough. It's not that complicated a hypothesis to think of. Or relativity is the classic example of something which could have been invented 50 years earlier. I don't know, I would love to learn more about this.
Lucas Perry: Just to tie this back to the question, could you give your probabilities as well?
Buck Shlegeris: Oh, geez, I don't know. Honestly, right now I feel like I'm 70% gradual takeoff or something, but I don't know. I might change my mind if I think about this for another hour. And there's also theoretical arguments as well for why most takeoffs are gradual, like the stuff in Paul's post. The easiest summary is, before someone does something really well, someone else does it kind of well in cases where a lot of people are trying to do the thing.
Lucas Perry: Okay. One facet of this, that I haven't heard discussed, is recursive self-improvement, and I'm confused about where that becomes the thing that affects whether it's discontinuous or continuous. If someone does something kind of well before something does something really well, if recursive self-improvement is a property of the thing being done kind of well, is it just kind of self-improving really quickly, or?
Buck Shlegeris: Yeah. I think Paul's post does a great job of talking about this exact argument. I think his basic claim is, which I find pretty plausible, before you have a system which is really good at self-improving, you have a system which is kind of good at self-improving, if it turns out to be really helpful to have a system be good at self-improving. And as soon as this is true, you have to posit an additional discontinuity.
Rohin Shah: One other thing I'd note is that humans are totally self improving. Productivity techniques, for example, are a form of self-improvement. You could imagine that AI systems might have advantages that humans don't, like being able to read their own weights and edit them directly. How much of an advantage this gives to the AI system, unclear. Still, I think then I just go back to the argument that Buck already made, which is at some point you get to an AI system that is somewhat good at understanding its weights and figuring out how to edit them, and that happens before you get the really powerful ones. Maybe this is like saying, "Well, you'll reach human levels of self-improvement by the time you have rat-level AI or something instead of human-level AI," which argues that you'll hit this hyperbolic point of the curve earlier, but it still looks like a hyperbolic curve that's still continuous at every point.
Buck Shlegeris: I agree.
Lucas Perry: I feel just generally surprised about your probabilities on continuous takeoff scenarios that they'd be slow.
Rohin Shah: The reason I'm trying to avoid the word slow and fast is because they're misleading. Slow takeoff is not slow in calendar time relative to fast takeoff. The question is, is there a spike at some point? Some people, upon reading Paul's posts are like, "Slow takeoff is faster than fast takeoff." That's a reasonably common reaction to it.
Buck Shlegeris: I would put it as slow takeoff is the claim that things are insane before you have the human-level AI.
Rohin Shah: Yeah.
Lucas Perry: This seems like a helpful perspective shift on this takeoff scenario question. I have not read Paul's post. What is it called so that we can include it in the page for this podcast?
Rohin Shah: It's just called Takeoff Speeds. Then the corresponding AI Impacts post is called Will AI See Discontinuous Progress?, I believe.
Lucas Perry: So if each of you guys had a lot more reach and influence and power and resources to bring to the AI alignment problem right now, what would you do?
Rohin Shah: I get this question a lot and my response is always, "Man, I don't know." It seems hard to scalably use people right now for AI risk. I can talk about which areas of research I'd like to see more people focus on. If you gave me people where I'm like, "I trust your judgment on your ability to do good conceptual work" or something, where would I put them? I think a lot of it would be on making good robust arguments for AI risk. I don't think we really have them, which seems like kind of a bad situation to be in. I think I would also invest a lot more in having good introductory materials, like this review, except this review is a little more aimed at people who are already in the field. It is less aimed at people who are trying to enter the field. I think we just have pretty terrible resources for people coming into the field and that should change.
Buck Shlegeris: I think that our resources are way better than they used to be.
Rohin Shah: That seems true.
Buck Shlegeris: In the course of my work, I talk to a lot of people who are new to AI alignment about it and I would say that their level of informedness is drastically better now than it was two years ago. A lot of which is due to things like 80,000 hours podcast, and other things like this podcast and the Alignment Newsletter, and so on. I think we just have made it somewhat easier for people to get into everything. The Alignment Forum, having its sequences prominently displayed, and so on.
Rohin Shah: Yeah, you named literally all of the things I would have named. Buck definitely has more information on this than I do. I do not work with people who are entering the field as much. I do think we could be substantially better.
Buck Shlegeris: Yes. I feel like I do have access to resources, not directly but in the sense that I know people at eg Open Philanthropy and the EA Funds and if I thought there were obvious things they should do, I think it's pretty likely that those funders would have already made them happen. And I occasionally embark on projects myself that I think are good for AI alignment, mostly on the outreach side. On a few occasions over the last year, I've just done projects that I was optimistic about. So I don't think I can name things that are just shovel-ready opportunities for someone else to do, which is good news because it's mostly because I think most of these things are already being done.
I am enthusiastic about workshops. I help run with MIRI these AI Risks for Computer Scientists workshops and I ran my own computing workshop with some friends, with kind of a similar purpose, aimed at people who are interested in this kind of stuff and who would like to spend some time learning more about it. I feel optimistic about this kind of project as a way of doing the thing Rohin was saying, making it easier for people to start having really deep thoughts about a lot of AI alignment stuff. So that's a kind of direction of projects that I'm pretty enthusiastic about. A couple other random AI alignment things I'm optimistic about. I've already mentioned that I think there should be an Ought competitor just because it seems like the kind of thing that more work could go into. I agree with Rohin on it being good to have more conceptual analysis of a bunch of this stuff. I'm generically enthusiastic about there being more high quality research done and more smart people, who've thought about this a lot, working on it as best as they can.
Rohin Shah: I think the actual bottleneck is good research and not necessarily field building, and I'm more optimistic about good research. Specifically, I am particularly interested in universality, interpretability. I would love for there to be some way to give people who work on AI alignment the chance to step back and think about the high-level picture for a while. I don't know if people don't do this because they don't want to or because they don't feel like they have the affordance to do so, and I would like the affordance to be there. I'd be very interested in people building models of what AGI systems could look like. Expected utility maximizers are one example of a model that you could have. Maybe we just try to redo evolution. We just create a very complicated, diverse environment with lots of agents going around and in their multi-agent interaction, they develop general intelligence somehow. I'd be interested for someone to take that scenario, flesh it out more, and then talk about what the alignment problem looks like in that setting.
Buck Shlegeris: I would love to have someone get really knowledgeable about evolutionary biology and try and apply analogies of that to AI alignment. I think that evolutionary biology has lots of smart things to say about what optimizers are and it'd be great to have those insights. I think Eliezer sort of did this many years ago. It would be good for more people to do this in my opinion.
Lucas Perry: All right. We're in the home stretch here. AI timelines. What do you think about the current state of predictions? There's been surveys that have been done with people giving maybe 50% probability over most researchers at about 2050 or so. What are each of your AI timelines? What's your probability distribution look like? What do you think about the state of predictions on this?
Rohin Shah: Haven't looked at the state of predictions in a while. It depends on who was surveyed. I think most people haven't thought about it very much and I don't know if I expect their predictions to be that good, but maybe wisdom of the crowds is a real thing. I don't think about it very much. I mostly use my inside view and talk to a bunch of people. Maybe, median, 30 years from now, which is 2050. So I guess I agree with them, don't I? That feels like an accident. The surveys were not an input into this process.
Lucas Perry: Okay, Buck?
Buck Shlegeris: I don't know what I think my overall timelines are. I think AI in the next 10 or 20 years is pretty plausible. Maybe I want to give it something around 50% which puts my median at around 2040. In terms of the state of things that people have said about AI timelines, I have had some really great conversations with people about their research on AI timelines which hasn't been published yet. But at some point in the next year, I think it's pretty likely that much better stuff about AI timelines modeling will have been published than has currently been published, so I'm excited for that.
Lucas Perry: All right. Information hazards. Originally, there seemed to be a lot of worry in the community about information hazards and even talking about superintelligence and being afraid of talking to anyone in positions of power, whether they be in private institutions or in government, about the strategic advantage of AI, about how one day it may confer a decisive strategic advantage. The dissonance here for me is that Putin comes out and says that who controls AI will control the world. Nick Bostrom published Superintelligence, which basically says what I already said. Max Tegmark's Life 3.0 basically also. My initial reaction and intuition is the cat's out of the bag. I don't think that echoing this increases risks any further than the risk is already at. But maybe you disagree.
Buck Shlegeris: Yeah. So here are two opinions I have about info hazards. One is: how bad is it to say stuff like that all over the internet? My guess is it's mildly bad because I think that not everyone thinks those things. I think that even if you could get those opinions as consequences from reading Superintelligence, I think that most people in fact have not read Superintelligence. Sometimes there are ideas where I just really don't want them to be crystallized common knowledge. I think that, to a large extent, assuming gradual takeoff worlds, it kind of doesn't matter because AI systems are going to be radically transforming the world inevitably. I guess you can affect how governments think about it, but it's a bit different there.
The other point I want to make about info hazards is I think there are a bunch of trickinesses with AI safety, where thinking about AI safety makes you think about questions about how AI development might go. I think that thinking about how AI development is going to go occasionally leads to think about things that are maybe, could be, relevant to capabilities, and I think that this makes it hard to do research because you then get scared about talking about them.
Rohin Shah: So I think my take on this is info hazards are real in the sense that there, in fact, are costs to saying specific kinds of information and publicizing them a bit. I think I'll agree in principle that some kinds of capabilities information has the cost of accelerating timelines. I usually think these are pretty strongly outweighed by the benefits in that it just seems really hard to be able to do any kind of shared intellectual work when you're constantly worried about what you do and don't make public. It really seems like if you really want to build a shared understanding within the field of AI alignment, that benefit is worth saying things that might be bad in some other ways. This depends on a lot of background facts that I'm not going to cover here but, for example, I probably wouldn't say the same thing about bio security.
Lucas Perry: Okay. That makes sense. Thanks for your opinions on this. So at the current state in time, do you guys think that people should be engaging with people in government or in policy spheres on questions of AI alignment?
Rohin Shah: Yes, but not in the sense of we're worried about when AGI comes. Even saying things like it might be really bad, as opposed to saying it might kill everybody, seems not great. Mostly on the basis of my model for what it takes to get governments to do things is, at the very least, you need consensus in the field so it seems kind of pointless to try right now. It might even be poisoning the well for future efforts. I think it does make sense to engage with government and policymakers about things that are in fact problems right now. To the extent that you think that recommender systems are causing a lot of problems, I think it makes sense to engage with government about how alignment-like techniques can help with that, especially if you're doing a bunch of specification learning-type stuff. That seems like the sort of stuff that should have relevance today and I think it would be great if those of us who did specification learning were trying to use it to improve existing systems.
Buck Shlegeris: This isn't my field. I trust the judgment of a lot of other people. I think that it's plausible that it's worth building relationships with governments now, not that I know what I'm talking about. I will note that I basically have only seen people talk about how to do AI governance in the cases where the AI safety problem is 90th percentile easiest. I basically only see people talking about it in the case where the technical safety problem is pretty doable, and this concerns me. I've just never seen anyone talk about what you do in a world where you're as pessimistic as I am, except to completely give up.
Lucas Perry: All right. Wrapping up here, is there anything else that we didn't talk about that you guys think was important? Or something that we weren't able to spend enough time on, that you would've liked to spend more time on?
Rohin Shah: I do want to eventually continue the conversation with Buck about coordination, but that does seem like it should happen not on this podcast.
Buck Shlegeris: That's what I was going to say too. Something that I want someone to do is write a trajectory for how AI goes down, that is really specific about what the world GDP is in every one of the years from now until insane intelligence explosion. And just write down what the world is like in each of those years because I don't know how to write an internally consistent, plausible trajectory. I don't know how to write even one of those for anything except a ridiculously fast takeoff. And this feels like a real shame.
Rohin Shah: That seems good to me as well. And also the sort of thing that I could not do because I don't know economics.
Lucas Perry: All right, so let's wrap up here then. So if listeners are interested in following either of you or seeing more of your blog posts or places where you would recommend they read more materials on AI alignment, where can they do that? We'll start with you, Buck.
Buck Shlegeris: You can Google me and find my website. I often post things on the Effective Altruism Forum. If you want to talk to me about AI alignment in person, perhaps you should apply to the AI Risks for Computer Scientists workshops run by MIRI.
Lucas Perry: And Rohin?
Rohin Shah: I write the Alignment Newsletter. That's a thing that you could sign up for. Also on my website, if you Google Rohin Shah Alignment Newsletter, I'm sure I will come up. These are also cross posted to the Alignment Forum, so another thing you can do is go to the Alignment Forum, look up my username and just see things that are there. I don't know that this is actually the thing that you want to be doing. If you're new to AI safety and want to learn more about it, I would echo the resources Buck mentioned earlier, which are the 80k podcasts about AI alignment. There are probably on the order of five of these. There's the Alignment Newsletter. There are the three recommended sequences on the Alignment Forum. Just go to alignmentforum.org and look under recommended sequences. And this podcast, of course.
Lucas Perry: All right. Heroic job, everyone. This is going to be a really good resource, I think. It's given me a lot of perspective on how thinking has changed over the past year or two.
Buck Shlegeris: And we can listen to it again in a year and see how dumb we are.
Lucas Perry: Yeah. There were lots of predictions and probabilities given today, so it'll be interesting to see how things are in a year or two from now. That'll be great. All right, so cool. Thank you both so much for coming on.