Ajeya Cotra on Forecasting Transformative Artificial Intelligence
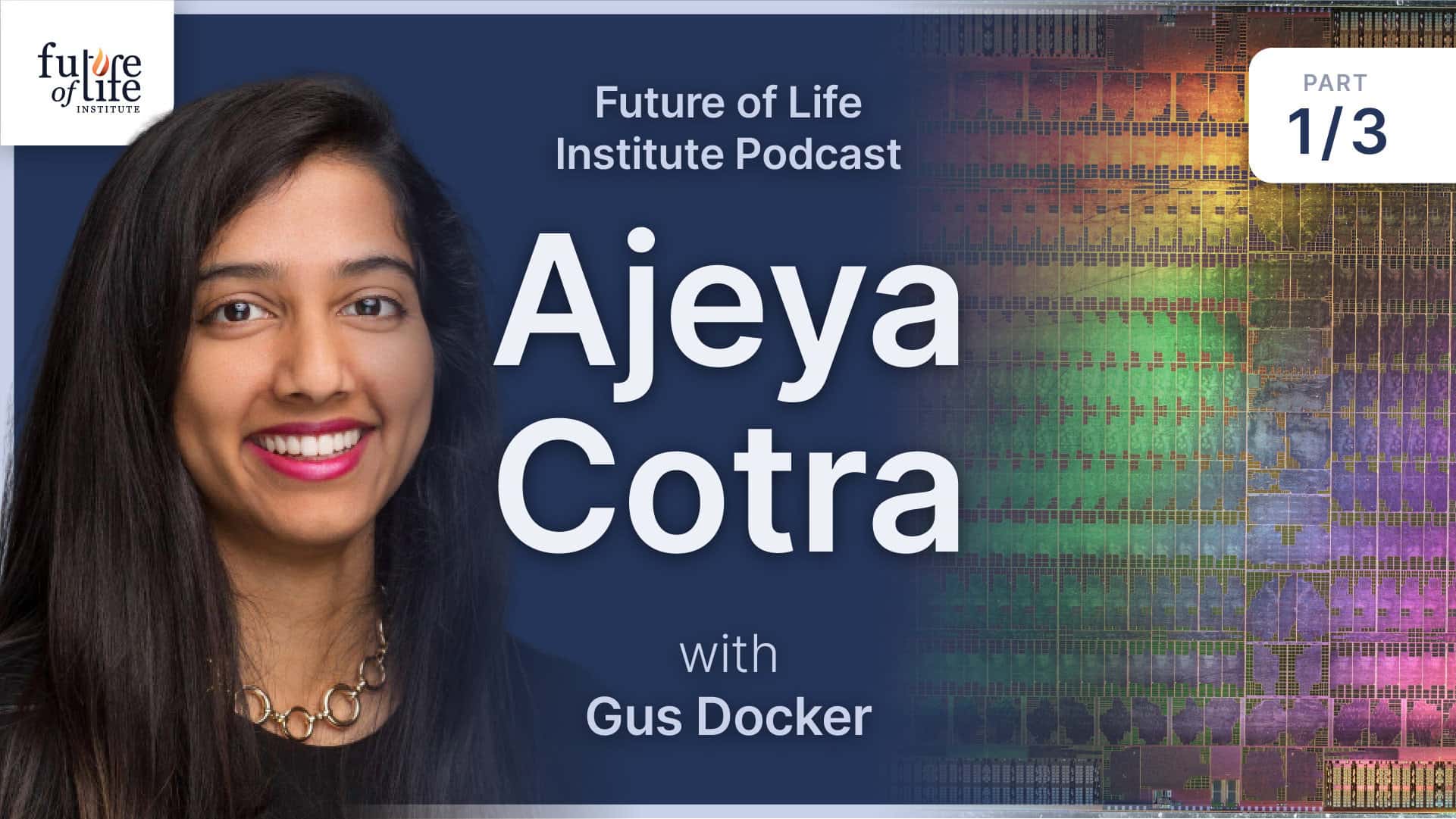
Ajeya Cotra joins us to discuss forecasting transformative artificial intelligence.
Follow the work of Ajeya and her colleagues
Timestamps:
00:00 Introduction
00:56 What is transformative AI?
03:09 Historical growth rates
05:32 Simpler forecasting methods
09:56 Biological anchors
18:55 Different paths to transformative AI
20:06 Which year will we get transformative AI?
29:13 Expert opinion on transformative AI
34:40 Are today's machine learning techniques enough?
38:00 Will AI be limited by the physical world and regulation?
44:10 Will AI be limited by training data?
48:05 Are there human abilities that AIs cannot learn?
54:32 The next episode
Transcript
Gus Docker:
Welcome to The Future of Life Institute Podcast. I'm Gus Docker.
On this episode, I talk with Ajeya Cotra who's a Senior Research Analyst at Open Philanthropy.
When will the world be transformed by advanced AI? Ajeya has produced what is perhaps the most in-depth investigation of this question. We talk about how to define transformative AI, different methods of forecasting transformative AI, what we can learn from the predictions of AI experts, whether today's machine learning techniques are enough to create transformative AI, whether AI development will be limited by the need to interact with the physical world, regulation or lack of training data, and whether there are human abilities that AI cannot learn.
Ajeya's report on AI
Gus Docker: Ajeya, welcome to the podcast. Thank you for being here.
Ajeya Cotra: Thank you for having me. It's great to be here.
Gus Docker: You have this report that you published in 2020, where you attempt to forecast transformative AI with something called biological anchors. We should walk through that title and explain what is forecasting, what is transformative AI, what are these biological anchors?
What is transformative AI?
Ajeya Cotra: Transformative AI is this term that is meant to talk about AI systems either one system or a collection of systems that has an unprecedentedly large impact on the world while trying to be relatively agnostic about the form that takes.
And in my report, I operationalize that a little more as AI systems that are capable of causing a 10 X acceleration in economic growth, because that was roughly as much acceleration as happened during the industrial revolution.
So growth was roughly 0.1% per year before the industrial revolution and roughly 1% per year after. Right now, growth is 2 to 3% per year. So transformative AI would be systems that could cause the economy to grow 20 to 30% per year.
Forecasting transformative AI
Ajeya Cotra: And forecasting, that is just, when do we think that kind of event would occur?
When would AI systems be developed that would have that capability. And it may be the case that systems are developed that have that ability, but they're actually put to different uses. So in my mind, I'm thinking of it as when will we develop AI systems, such that if we were just selling them in a free market, using them wherever they were profitable, that would cause a 10 X acceleration in growth.
There may be reasons why the people who develop these systems decide not to use it that way. In which case we may not observe the full potential of that growth. And it might instead be directed toward e.g. having a military advantage or other things.
Historical growth rates
Gus Docker: This 10 X increase in economic growth, it's a very rare event in world history.
Ajeya Cotra: Yeah. I think this is actually a little bit complicated because there's a debate on how to view the world historical growth. There's kind of two perspectives. In one perspective, there have been one to two bursts of increased growth where we go from one mode to another, like going from hunting and gathering to farming, and then going from farming to industry.
In another perspective, there's actually been roughly smoothly accelerating growth rates. So a growth rate of 1% per year means that the amount of stuff we have is accelerating. So you have an exponential curve. But you can also have that growth rate itself be accelerating, in which case you have a super exponential curve that actually, if you extrapolated all the way out will apparently reach infinity in finite time.
But of course we would treat that as a model and then we would eventually model some limits to growth there. So there are kind of two perspectives. On one perspective with transformative AI, it's like we've changed growth modes a couple times before, that could happen again and transformative AI could be the thing that causes us to change into a new growth mode. Into a purely digital economy or an AI based economy, as opposed to an industrial economy or a farming economy or hunting economy.
From another perspective, though, transformative AI would be one more thing that continues a trend of growth rates, accelerating through history. And from that perspective, what we call the industrial revolution is like, a period we picked out where that process was above trend and unusually fast, but not massively special because before the industrial revolution and after the industrial revolution, there was still acceleration of growth. Just maybe like slightly less sharp than it was in that period.
Gus Docker: But under either of these models, we are talking about an enormous change to the everyday life of people on earth. How on earth do we go about forecasting this event? What are these biological anchors you're talking about in the report?
Simpler forecasting methods
Ajeya Cotra: So before I talk about the biological anchors, I kind of wanna back up and talk about simpler methods of potentially forecasting this event. So one thing I mentioned is that, you can potentially try and fit a curve to historical growth and just extrapolate forward that curve. And whether you frame it as there have been a few transitions and stocastically there might be a future transition, or there's some underlying trend in which growth rates are accelerating, and then that might continue.
In either way, either methodology would give you some number or range by which we might have much faster growth than we have now. And that's a very simple, not very AI-centric methodology. For example, it could be implicitly forecasting, something else that causes that much growth, such as, mind uploading where rather than developing AI systems, it's humans, whose minds are able to be accelerated a lot by being put on computers.
So that's one methodology that's broad and big picture and Open Philanthropy researchers, David Roodman and Tom Davidson have reports out about that methodology. You could have a survey of AI researchers or economists or whoever you think might have relevant expertise. And there, there have been a couple surveys done by Katja Grace at AI Impacts.
And you could also kind of take a like broad outside view picture of the development of the AI field, where you could say something like: AI as a field hasn't existed for too long, it's existed since say 1950 or 1940, depending on how you count. The whole field hasn't been working for too much and it's been growing, so more of the work has kind of concentrated in the recent years.
So you could have Laplace's law of succession type methodology where you say: we've been working for 70 years, or maybe the last 50 years are the most important. So we probably won't get AI in the next 20 years, but we plausibly will get it in the next 70 years just because we haven't really ruled out that AI can be developed in 140 years of effort, because we've only gone through 70 years of that.
And then you can refine this a little more by observing that, investment has been accelerating, the field has never been this funded and this large before. And so you might wanna make simple boosts for that sort of observation.
Gus Docker: Does this rely on an estimate of how much progress we've made towards transformative AI then? Is that the methodology?
Ajeya Cotra: That's not the methodology. The methodology is simpler than that. It's based on this mathematician Pierre-Simone Laplace had this very simple formula for determining the probability of an event that has never happened before. So if you flip a bunch of coins and they all come up heads, you don't think the probability of tails is zero on the next flip. And the way he suggested estimating that probability, the bias of the coin, is as assuming that you had two virtual coins, one which was heads and one, which was tails, that you saw before you started making your observations. So basically you would determine the probability of tails in that situation. If you had 10 heads in a row, as, 10 plus one over 10 plus two, by adding in the virtual head and the virtual tail.
So if we're applying this methodology to forecasting technologies, the only observation we're really thinking of is, it hasn't happened yet. So this is the methodology that someone living in a cave that every year just gets the information, they haven't developed TAI yet would place on the probability of developing TAI in the next N years.
Biological anchors
Gus Docker: Okay, makes sense. It's a very simple method. But you went with none of these methods, you went with this method of biological anchors. So, why is that method better in your opinion?
Ajeya Cotra: There's a spectrum of how inside view methodologies are versus how outside view they are. And the methodologies that I described are more outside view than the one I'm using.
Gus Docker: We should mention what you mean by inside view and outside view.
Ajeya Cotra: Yeah, so inside view is having a relatively detailed model of some phenomenon that you use to make predictions about it or think about it. If you were trying to predict who would win an election, say an inside view methodology might involve more thinking about, the relative merits of the candidates and which ones seem to have better positions and which one has more charisma, et cetera.
Whereas an outside view methodology just uses reference class forecasting, which means you regress to a prior of saying, roughly half the time one of the candidates wins and roughly half the time the other wins. So that's gonna be my estimate. In the case of transformative AI, you can't really have truly outside view estimates in the sense that I described, because, we haven't seen many observations of transformative AI in other worlds that we can just do a reference class forecast over.
But the methodologies I've talked about are closer in spirit to that. So the Laplace's law of succession, trying not to use very much information about AI and the economic forecasting is also trying not to use very much information about AI. So the method that I used in my report uses more information about AI systems and is more detailed in general, but I think it's important to have these other simpler methods in mind because if the estimates were kind of wildly off between what I concluded in this report and what these simpler methods would've said, I think that would be a tension worth exploring.
Holden Karnofsky on his blog, Cold Takes, has a post called "AI forecasting - where the arguments and the experts stand" in which he basically has a table that goes through all these methods that I just talked about. And they definitely have different estimates. But it's not the case that the outside view estimates say, AI is impossible this century and my estimates say 50% in 30 years, it's not that big of a gap. And so it's important to have these outside view estimates as background as the prior that you need to overcome, the like baseline from which you start updating.
Gus Docker: Makes perfect sense. Let's try to explain what biological anchors are.
Ajeya Cotra: Yeah. So biological anchors are this idea that you might try to forecast when human-level AI systems might be developed by supposing that the amount of computation that you need to run a human level AI system is roughly as much computation as the human brain itself runs on, if you were to view it as a computer. Open Philanthropy researcher Joseph Carlsmith has a report about how much computation is the human brain running on, if we imagine it as a computer. And there are, a lot of philosophical subtleties there, as well as a lot of neuroscience details that could move your estimate.
But broadly his conclusion, which is similar to the conclusions of other people, historically, who went through this exercise, is that the human brain runs on 10 to the 15 floating point operations per second, where floating point operations per second, is this measure of how powerful a computer is. Roughly, it's asking how many simple calculations, like adding two numbers or multiplying two numbers, can the system perform per second?
So that would be saying, the human brain performs roughly one quadrillion simple calculations like that per second, in the course of seeing things and talking and all this stuff. If you start from the premise that the human brain is this artifact, this computer built by evolution and it's, so efficient, you know, it has 10 to the 15 floating point operations that it does per second.
You start from the premise that evolution performed this large search over many millions, billions of years on brain designs. It was optimizing for organisms to have higher genetic fitness, to have more descendants in expectation and, other things being equal, consuming more energy or resources to do the same thing, likely reduces your genetic fitness.
So in that sense, we say that evolution as a search process or an optimization process is providing some pressure to increase this number in floating point operations per second, to make it so that a given brain can be more powerful with a similar amount of energy usage.
So we're saying this is kind of a variable that evolution was quote unquote, trying to optimize. And so we might expect it to be difficult to design an artifact that is way, way more efficient than the human brain while still having its same abilities. So we might guess that whenever we develop transformative AI or human level, AI, it might need to run on as much computation as we believe the human brain runs on.
That's sort of broadly the amount of resources it takes to create this level of intelligence or ability if you're trying to use resources economically in a very broad sense. So that's the starting premise and this starting premise has been used by various people in the eighties and nineties to try to forecast AI progress and forecast human level AI.
So notably, Hans Moravec is a computer scientist that has a forecast that starts from this premise. Ray Kurzweil is a futurist that also uses this kind of premise and methodology to try to forecast AI progress. So that kind of frame isn't novel. The thing that the report does that is more novel is asking: okay, you know, what if we say that we're trying to build a system that has this much computation, but rather than building it directly with humans, working with a computer that big and trying to write a software program that's as powerful as the brain, which is kind of implicitly what Moravec and Kurzweil were imagining.
Let's imagine that we use modern machine learning techniques to train a system that runs on that much computation. So, how much computation would it take to use modern, deep learning techniques to train a model that is as big as we believe the human brain is in terms of computation? So that was the kind of additional premise that my report brought in, because it seems unlikely at this point that we will kind of hand code an AI system and that it seems likely to be some sort of machine learning process.
How much data and how much computation would it take to train a model that's the size of the brain. That's the question that the report is asking. And the report is saying that might be like a proxy to the question of when we might train transformative AI systems.
Different paths to transformative AI
Ajeya Cotra: So it's kind of a different question in that we're asking about, when we might be able to afford to train one kind of unified system, that's like more of a classical AGI type system that's the size of a human brain that can do everything humans can do, or, a large subset of the most important things humans can do. We might get transformative AI in other ways, for example, we might get transformative AI by having, you know, many smaller systems that each do more specialized things.
But the insight here is that if you can name one specific way of getting transformative AI, that in some sense seems kind of brute force, just training a giant machine learning model, it seems plausible that we would've gotten transformative AI either through that path or through something even more efficient that, you know, we discovered through human ingenuity.
In that sense, you can think of it as an upper bound for timelines, but I don't believe it to be like too loose of an upper bound, I think for various reasons, just because things are difficult and they take time, I'm more inclined to treat this as something like a median. So conceptually it might be an upper bound, but to just account for how often these kinds of forecasts are oversimplified and how this kind of forecast in the past has tended to overestimate how quickly AI progress would go, I'm treating that as a median.
Which year will we get transformative AI?
Gus Docker: Okay, so we should mention the conclusion of the report and your further thinking about when we might have transformative AI. You mentioned that we could see it as a median or as a conceptual upper bround. What are the numbers here? What are we talking?
Ajeya Cotra: Yeah, so the conclusion of the report at the time was that there was roughly a 50% chance that the amount of computation required to train a brain-sized AI model would be available by 2050. So that was the median of the report, which is 30 years from the time the report was written. There was significant probabilities on sooner than that, but the median was 2050.
Gus Docker: 2050. That seems very, very close to the present.
Ajeya Cotra: Yes.
Gus Docker: But you've you've moved your AI or TAI timelines even closer to the present in the meantime.
Ajeya Cotra: Yeah. So I recently wrote an update post in which I said that over the last two years, I've updated toward it being more like 2040 as a median. So there are a few reasons for that. One is that in the report, I was imagining that in order to train AI systems to be as useful as humans, the AI system would itself have to learn as quickly as humans can learn.
And as efficiently as humans can learn. So this is not the like learning process that trains the AI system, which might be analogous to evolution. This is the AI system itself, like the computer program while it's running, should be able to learn as efficiently as humans can in order to be as useful as them.
And so the process that produces the AI system seems likely to be some sort of meta learning. So the task that it's teaching the AI is the task of learning new things efficiently, and that seemed potentially harder to train than many of the tasks that we had done up to that point, like Image recognition and language modeling were all these pretty short horizon tasks where you get really dense feedback.
But if the task you're trying to learn is learning itself where you kind of encounter a new system, interact with it for a few hours, discover something about it and use what you discover to do something useful. That whole process takes longer to pay off as either "you did well" or "you didn't do well".
So you kind of have a sparser feedback signal here and that might make training take longer. So a naive guess would be that it takes linearly longer. If you have a feedback signal, every, one second of a certain level of quality in one training run and in a different training run, you have a similar level of quality of feedback signal every one hour, then you might expect the second one to take 3,600 times as much as the first one because you kind of need just as many data points if you're training a model of a similar size, but each data point is more expensive for you to produce, involves the model running for longer, involves collecting more data, more like environment frames or whatever.
I had that as a premise that I put significant weight on in the report in 2020. I did have a bunch of stuff that I said then of how, it might be shorter than that are easier than that. Systems that are trained on like pretty short horizon tasks, we might be able to put them together and compose them in order to do these higher level, more useful tasks without having to train in them the ability to learn themselves.
I think over the course of the last couple years, I updated toward it being more likely that systems could have transformative impacts while not really having this meta-learning ability. So in some sense, I lowered my bar for what transformative even means because programming and software engineering tasks are tasks where you have unusually much data lying out there in the world and data that can be produced by employees at tech companies just doing their normal jobs.
And it seems unusually rich in feedback based on, you can see whether your code worked, you can see whether it passed tests and so on. And it seems like a system that was able to do all these software engineering tasks, not through the human methodology of learning things quickly and adapting quickly, but through a different methodology of just having memorized a lot of stuff and just already knowing a lot of things on the basis of its training.
It seems like that in itself might be enough to kick off a transformative change of the kind that we're talking about because that kind of set of activities of programming could be used to make AI systems themselves improve in various ways. And it would essentially accelerate AI research.
And a previous premise of our work is that AI research will eventually produce this thing that accelerates the entire economy or has the potential to accelerate the entire economy. As a very simplistic case, if I thought that in 2040, it would take 10 years of research from the human field to be able to produce transformative AI from that point, then any technology that sped up AI progress itself would bring that date forward. So if they had a system that speeds up AI progress by 10 X, then the research that I thought had to be done would instead be done in one year.
Lowering the bar for transformative AI and believing that narrowly software engineering might be a lot of the first task that we need to clear. And also that in order to get that software engineering task, we maybe don't need meta-learning. We don't need systems that are good at handling totally novel situations because we can instead have systems that rely on having memorized and seen a lot more than any human programmer has seen caused me to kind of like shift more toward the estimates that would be produced if you assume that the task you're training transformative AI on is highly dense in its feedback signals.
So that was one thing. I think that was the biggest thing that caused the update. A couple other things. Another one is that I had sort of very simple extrapolations for hardware progress, software progress, and money spent training AI systems in my report.
So I spent most time kind of thinking about how much computation would it take to train an AI system that had this transformative impact if we had to do it today. And then I combined that with forecasts of, how are our architectures becoming more efficient? How is our spending increasing, how is hardware getting cheaper? To extrapolate that forward into a probability that the amount of computation would be affordable.
So I had these like very simple trend line based forecasts for those three things, but actually there's a potentially important feedback loop in those things, where if early AI systems have, exciting applications, even if they're narrow, that's likely to attract more investment into the field.
So investment speeds up and then the greater investment into the field is probably gonna cause more people to go into research, which causes accelerations in software progress and hardware progress. So it's a demand driven acceleration of all of those things that I had been plotting as static trend lines also contributes to expecting progress to be sooner.
So this is dependent on you don't get transformative AI all at once. You get some earlier applications that make money, attract investment and kind of speed up that whole track toward getting transformative AI.
Expert opinion on transformative AI
Gus Docker: To many listeners these timelines, or these predictions with a median of 2040, will seem incredibly fast or this is right around the corner. It will sound unbelievable. But of course, as I think it's obvious to everyone listening to this, a lot of work has gone into, to the report you're talking about a lot of thinking has gone into this. How can we try to test the conclusions of this report? Are there sanity checks we can run to see whether we have theorized ourself into a corner with this report?
Specifically, I'm talking about this survey, you mentioned earlier by Katja Grace, where she surveys 700 machine learning researchers. And they conclude that with a 50% probability, we will have human level AI by 2059. And by human level AI, they mean that machines can do every task better than human workers. How would you value the information you got from working on the report versus these surveys of machine learning experts?
Ajeya Cotra: Yeah. So I think that a thing that was very noteworthy about that survey is that there was some clear evidence that people weren't thinking about the question very hard. So you reported the kind of median probability of human level AI, but the question also asked a number of different questions to pin down the logical structure of their beliefs here and for a lot of individual tasks that were pulled out and named, such as when would it be the case that AI can automate machine learning research? Or when would it be the case that AIs can automate math research? Those experts actually gave longer answers.
It's hard to be like consistent and it was an overlapping but different set of people that got the different questions. I think that even if all of these machine learning researchers have intuition from their jobs that is informative for timelines, which I think is true, the way that was elicited and expressed in this survey where they just thought about the question and gave an answer is clearly lacking in some ways.
Another thing I wanna say is that at least recently it feels like many machine learning researchers have underestimated progress over the last few years. So, Jacob Steinhardt, who's a professor at Berkeley ran a forecasting contest. I think it began in 2021, early 2021, in which he asked super forecasters, like professional forecasters, that forecast things like political events and also machine learning researchers, when AI systems would reach 50% on a couple of benchmarks. A sort of general knowledge benchmark and a math benchmark. And both groups of people actually substantially underestimated progress in that those benchmarks were cleared in 2022. And the distribution generated by the forecasts placed very low probability on that. And the median of the distribution was something more like 2026. So it does seem like it's the case that at least recently machine learning researchers underestimate progress in the field.
Gus Docker: Maybe there aren't really any experts at forecasting transformative AI? So if you're a professor of machine learning you specialize in some very specific aspect of maybe the training process. Your day job isn't thinking about predicting when we will have transformative AI. Maybe there are actually far fewer experts in this area than we might believe.
Ajeya Cotra: I think that's right. I think they're definitely different jobs. And I don't think there's really anybody. Who's an expert in AI forecasting. It's a very immature field. Forecasting in general is a very immature field and AI timelines forecasting is especially so. Holden once joke that asking machine learning researchers to forecast when we'll get transformative AI is sort of like asking the CEOs of oil companies to forecast climate change. Or even the CEOs of renewable energy companies to forecast climate change outcomes. Those are just different things like working on a technology is different from having the kind of background and thought process to forecast where that technology will go.
Are today's machine learning techniques enough?
Gus Docker: When you're thinking about transformative AI, are you assuming that we won't need radically new insights and that we can get to transformative AI by the machine learning techniques that we already have today?
Ajeya Cotra: When I described my previous forecast as being a sort of soft upper bound, this was based on having a high probability, in that report it was 80%, of something in the spectrum of training a large machine learning model being sufficient for transformative AI. Some of those values in the spectrum are extremely expensive, very close to replicating all the computation done by evolution.
And if in fact that's what it would take to get transformative AI through this, train a big model brute force way, I expect actually people would find something more clever that did involve insights to avoid paying such a large cost. So in that sense, it's a soft upper bound of some of these like more expensive ways of getting transformative AI from using machine learning techniques are really very brute force. And I think they would plausibly succeed if we had that astronomical level of resources.
But in fact if that's what it would take to do things with deep learning, probably people would invent something more efficient than that. On the lower end, if you imagine all you need to do is train a large machine learning model with fairly dense feedback signals similar to the training regimes we have now there, I think that suggests that this technique is pretty simple, but it might get us to transformative AI before big breakthrough insights have time to happen because those are pretty stochastic and relatively rare by their nature. So because in the report, and especially now, I have relatively short timelines, I expect a relatively high probability that we kind of just, turns out it's pretty straightforward to build these systems.
And I definitely think that there's gonna be algorithmic progress. There's gonna be better architectures, better optimization algorithms, better loss functions. A lot of things will improve in the way things tend to, and that will play a role in making the systems, making it affordable to train a transformative model.
But ultimately I don't expect something that we would in retrospect, call a big game changing paradigm shift.
Gus Docker: It's basically a game of scale, then. Getting lots of computing power, getting lots of training data and training these models in very expensive ways.
Ajeya Cotra: Yeah, that's my mainline picture. It's definitely very uncertain. And I think if you told me that we don't develop transformative AI in the next 20 to 30 years, my probability that when we actually do develop it, it involves a paradigm shift and like kind of larger insights goes up a lot.
Will AI be limited by the physical world and regulation?
Gus Docker: Let's talk about something that might slow down this picture of when we could get to transformative AI. If you're running a scientific research program, you have to collect lots of data from the real world. And that takes a number of years. Maybe you also have to get regulatory approval to run some experiments, and already there you could be talking about five or seven years before you even could get some result. If we imagine AI doing science for us, wouldn't it run into these same limits of collecting empirical data or regulation?
Ajeya Cotra: You're asking about, suppose after we have AI systems that could automate science going from there to having an impact in terms of accelerated growth.
Gus Docker: Yes. How would this very advanced system have this impact? If it needs to engage with the physical world in a way where the feedback is very slow over a number of years. So how could this happen in the next 20 years, if it takes, say two years or five years to get regulatory approval, to run an experiment?
Ajeya Cotra: Yeah. So, broadly my answer is that I imagine that AI will be disproportionately applied to areas where there aren't a lot of regulation. And I think there are many of those areas. Programming and computer science in general, computer hacking and things like that, trading.
There are kind of like large swathes of the economy that are maybe not consumer facing and not labeled as explicit "this is science and it's in the domain of science" that could produce a lot of value that people just kind of can do what they want in. Anything that's the domain of a Silicon Valley startup, I think could be radically transformed by such a system.
You need to have regulatory approval to do phase two trials on some drug on humans. But just inventing, you know, insanely good virtual reality technology, or just making a lot of money on the stock market, inventing very compelling and persuasive kinds of art. There's just a lot of things that are sort of unregulated and people just do them now. And AI systems could just do them then.
And this goes for things like, Alpha Fold was developed by Deepmind. This is a model that, based on a protein's molecular structure predicts its three dimensional form, like how it will fold up into a three-dimensional object. And this had been a very difficult and important question for a long time. The way they trained this AI system didn't really involve interacting with anything physical.
They trained it on a number of proteins and their three-dimensional forms and it picked up on these patterns. So I do agree that when you have AI systems that are able to think a lot faster than humans and are a lot more numerous than humans, relatively speaking, interacting with the physical world and getting some result back from the physical world becomes very painful.
And, from the perspective of those AI systems, they might be waiting a lifetime or multiple lifetimes to get some result back from the real world. But I think that will result in systems shifting a lot of effort to having really accurate simulations and having like really efficient, experimental design so when they do interact with the real world, they try a lot of experiments in parallel, the experiments have been like winnowed down to testing like some pretty specific hypotheses that couldn't be ruled out in simulation and so on.
Things like drug discovery and so on, I think will still be accelerated even if the part of the process where you make a physical drug and you test it on animals and you're tested on humans, isn't particularly accelerated just because the ideation part of the process, which narrows that down, you know, a whole universe of possibilities into a few drugs, you actually try to develop, is greatly accelerated and there's greater search power to pick out the things in that space that are likely to actually work and have major impacts.
Gus Docker: It's an interesting picture of the future in which everything that's digital might be moving much faster than everything that's physical.
Ajeya Cotra: Yeah.
Gus Docker: Could we see, for example, housing staying relatively the same while we get incredibly advanced virtual reality or drug discovery or anything that's purely digital?
Ajeya Cotra: I do think that the digital world will be moving faster than the physical world. But one of the things that I think is not particularly regulated, where you don't need IRB approval right now is building robots. And you have a lot of robotics startups. And I think one of the tasks that we would put these AI scientists and engineers toward is designing better, smaller, cheaper robots.
And robots that like physically move faster than humans can, like skidder around like bugs or whatever. So I think that kind of advance would speed up physical manufacturing of all kinds as well, less so and less immediately than the digital world is sped up. But I don't think the gap is 10 plus years between those two things, given that you can pour a lot of purely digital effort into producing really efficient robots.
Gus Docker: You might even be able to train the robots in a simulated environment and then transfer what they've learned in that environment into the physical world.
Will AI be limited by training data?
Gus Docker: Let's talk about training data, because this is very important. If we are thinking about training machine learning models. Could it be the case that we are running out of data? As I understand it, many of the most recent language models and image models are trained on large parts of the data on the internet. Is it the case that data is a limiting factor in how good these models can be?
Ajeya Cotra: You know, If you asked me how I might be wrong about my aggressive timelines forecasts, I do think data is one of the few things I would maybe point at as potentially causing a big swing.
I haven't thought about this as much as I'd like to, but here are kind of a few reasons why I at least don't think it's appropriate to be confident that this would stall progress. One thing is that as you get to AI systems that are very large and very expensive, single training runs, the compute could cost, a billion dollars or something.
When you're in that regime, it becomes affordable and sensible to pay human beings a lot of money to generate data or provide RL-supervision where that isn't really the case now. So if you spend a million dollars on a training run, you kind of want to just scrape up passive data because you don't wanna pay many humans by the hour to produce demonstrations for your model. Or to judge, which of the models' completions are better. So when you're kind of in this regime where things are much more expensive, I think, yes, you might run out of passive data, but it might be much more worthwhile to have literally human scientists, human software engineers think about what kind of data they could produce that would cause this model to learn something more efficiently and produce that data.
And you could also use smaller models to produce that kind of data, to train larger models. And if we move more toward a reinforcement learning regime, then we move more to a regime where the model is generating its own data. And the learning is in saying which of two answers it gave to a question was better.
And so then you kind of produce quote unquote data just by running the model. In particular, some things that might enhance general intelligence or reasoning ability that are functionally infinite data. So if you're training a model to play chess or any kind of zero sum board game, or to prove theorems in mathematics, you can procedurally generate infinite data in those domains.
You can ask the model to prove any number of theorems that you generate. You can ask the model to play itself in chess, you know, as many times as you want, because you have this kind of compact description of what the game is and you just run it every time to generate a new data point for the model.
So yeah, the combination of it seems worthwhile to pay humans, to generate data or provide RL-supervision, the model can generate its own data in an RL-context, and there are at least some environments that we haven't fully tapped out, which have functionally infinite data, suggest to me that at least if the kind of level of training we need for transformative AI, the size of the model is not too enormous, we might be able to, find creative ways to get it the data that it needs.
Are there human abilities that AIs cannot learn?
Gus Docker: So my question is whether there is some kind of human secret source, maybe it's creativity, maybe it's um, some ability we have that cannot be learned by these machine learning models? This is a bit of a risky question in the sense that it is a question that, when asked in the past, people have been embarrassed by how things actually turned out. If we're interested in whether AI can do science, then we are also interested in the kind of the biggest scientific breakthroughs we can imagine. So developing a new theory in physics, for example. Is it possible to learn how to develop a new theory in physics only by reading or processing whatever data already exists? Or is there some kind of leap of creativity involved in that process?
Ajeya Cotra: I have a few different responses to different parts of that. One is, I don't imagine that we'll end up training systems purely by consuming and reading. I think systems will end up doing a lot of learning by doing. So in the case of a physics model, a lot of its training would involve reading papers on the internet, but a lot of its training would also involve proposing experiments and being told how good its experiment proposal was, writing papers and getting feedback on the papers it writes, and generally doing things and getting reinforcement learning feedback from those things.
And if you really cared about getting a system that was very good at producing insights in physics, you would also try to be really careful about its curriculum. You'd have it start out doing short things that had dense feedback that were relatively easy, and then you'd iteratively move it toward harder things to give it a good learning curve.
I would share some of your skepticism if you were imagining getting transformative AI purely through reading and predicting what you'll see next in the text that you're reading. And then the other thing I wanna say is that I don't think growth is mainly driven by these like big, sexy, cool insights that come along rarely. I think Einstein definitely punched above his weight in terms of generating economic insight and the stuff he thought about ended up being used in GPS systems and stuff.
But if you think about the total amount of economic value that has been produced by like cool, sexy insights versus like more plotting work, I think that the more plotting work as a whole, as a block, has contributed far more to economic value. Little R&D iteration things, that you wouldn't have, you know, legends written about later, I think are gonna be easier to train AI systems to do and are actually collectively more important than the really cool insights.
And this was part of what moved me to lowering the bar in my mind, that I was imagining for transformative AI systems, because having a kind of somewhat closer interaction with how machine learning research is done.
You know, obviously, machine learning researchers are very intelligent and they have creative ideas all the time, but often you try a tweak on something that was tried before people had that idea before, maybe something got a little bit easier and it's a little bit more practical to try that thing now so you try it and it's like, you know, one incremental improvement on your system and you have many of those. And that seems to be what drives progress more. Although there are big insights occasionally.
And then finally addressing the first thing you were talking about, is there a human special sauce that we can't train these systems to learn? I do think that on a very abstract level, there are gonna be some cognitive skills where humans just happen by chance to be much better suited to those skills than AI systems. And I think that doesn't necessarily mean that no amount of training and no size of model could be taught those skills. But it might mean that the first transformative systems lack some abilities that humans have.
And so it's not the case that the first transformative systems are going to be able to do exactly what humans do or exactly a super set of what humans do, but that there's going to be some Venn diagram where they're able to do a lot of the things that humans can do, sometimes better than us, sometimes worse than us. And they'll have some skills that we don't have at all. And we'll have some skills that they don't really have at all.
And if I had to guess at what those skills might be, I think it would go back to efficient learning. I think that probably the first transformative systems will know what to do in a lot of situations by virtue of having consumed much more information than humans could consume in their lifetime and will be relatively weaker at quickly picking up on something that's totally novel.
I think we will eventually develop AI systems that are better than humans that everything humans do, but I think that's a higher bar than is necessary for transformative AI, and that higher bar might be achieved through first transformative AI systems doing AI research, eventually leading to a different paradigm. And that kind of like smarter systems that are superhuman in every domain might be built in a very different way from plain deep learning even if the first transformative AI systems are built through plain deep learning.
The next episode
That's it for this episode. On the next episode, I talk with Ayeja about how AI development might result in a catastrophe. Ajeya lays out a concrete scenario for how the incentives of today's AI development could cause humanity to lose control over our AI systems.