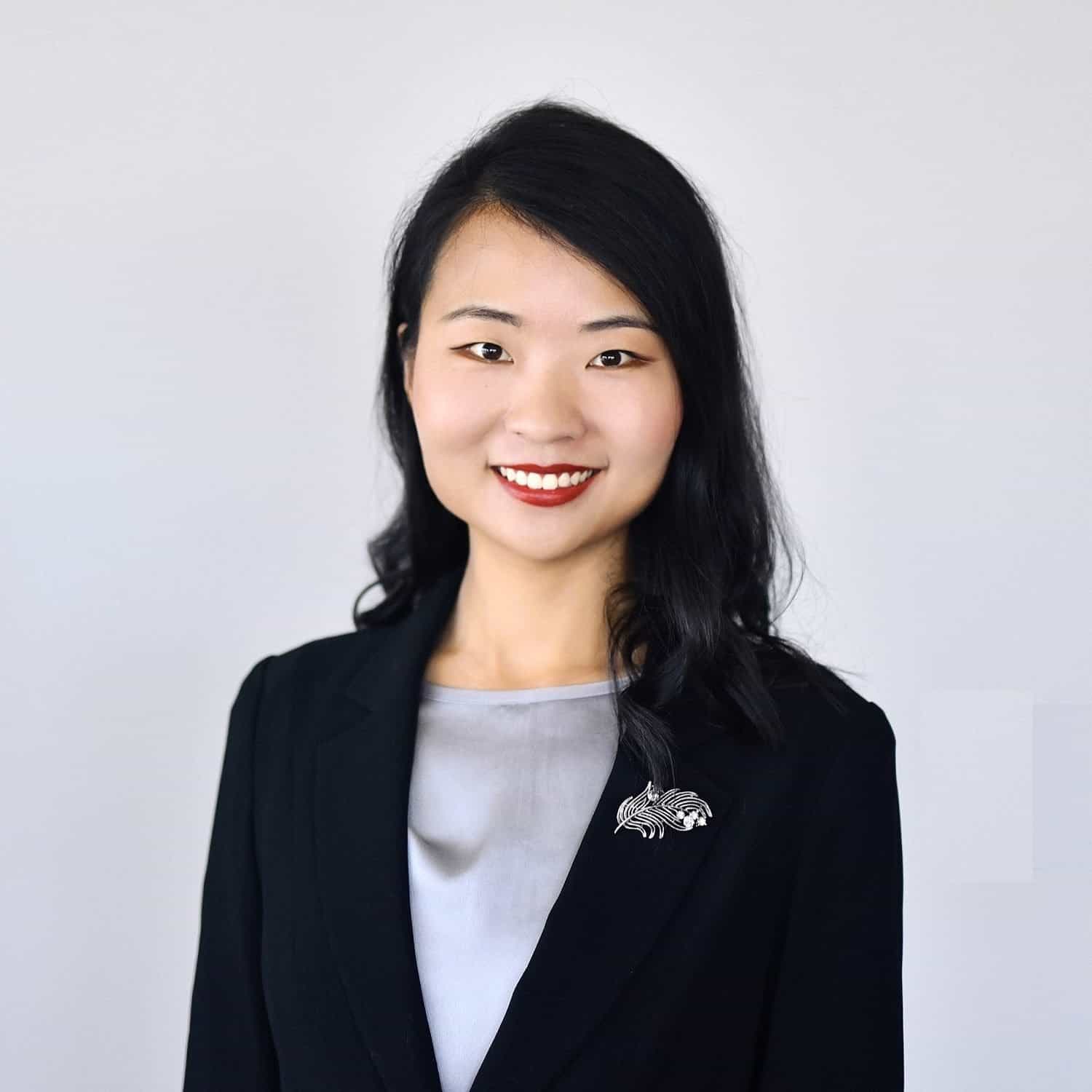
Sharon Li
Why do you care about AI Existential Safety?
As artificial intelligence reaches society at large, the need for safe and reliable decision-making is increasingly critical. This requires intelligent systems to have an awareness of uncertainty and a mandate to confront unknown situations with caution. Yet for many decades, machine learning methods commonly have made the closed-world assumption—the test data is drawn from the same distribution as the training data (i.e., in-distribution data). Such an idealistic assumption rarely holds true in the open world, where test inputs can naturally arise from unseen categories that were not in the training data. When such a discrepancy occurs, algorithms that classify OOD samples as one of the in-distribution (ID) classes can be catastrophic. For example, a medical AI system trained on a certain set of diseases (ID) may encounter a different disease (OOD) and can cause mistreatment if not handled cautiously. Unfortunately, modern deep neural networks can produce overconfident predictions on OOD data, which raises significant reliability concerns. In my research, I deeply care about improving the safety and reliability of modern machine learning models in deployment.
Please give one or more examples of research interests relevant to AI existential safety:
My broad research interests are in deep learning and machine learning. My time in both academia and industry has shaped my view and approach in research. The goal of my research is to enable transformative algorithms and practices towards safe and reliable open-world learning, which can function safely and adaptively in the presence of evolving and unpredictable data streams. My works explore, understand, and mitigate the many challenges where failure modes can naturally occur in deploying machine learning models in the open world. Research topics that I am currently focusing on include: (1) Out-of-distribution uncertainty estimation for reliable decision-making; (2) Uncertainty-aware deep learning in healthcare and computer vision; (3) Open-world deep learning.
My research stands to benefit a wide range of societal activities and systems that range from AI services (e.g., content understanding) to transportation (e.g., autonomous vehicles), finance (e.g., risk management), and healthcare (e.g., medical diagnosis).