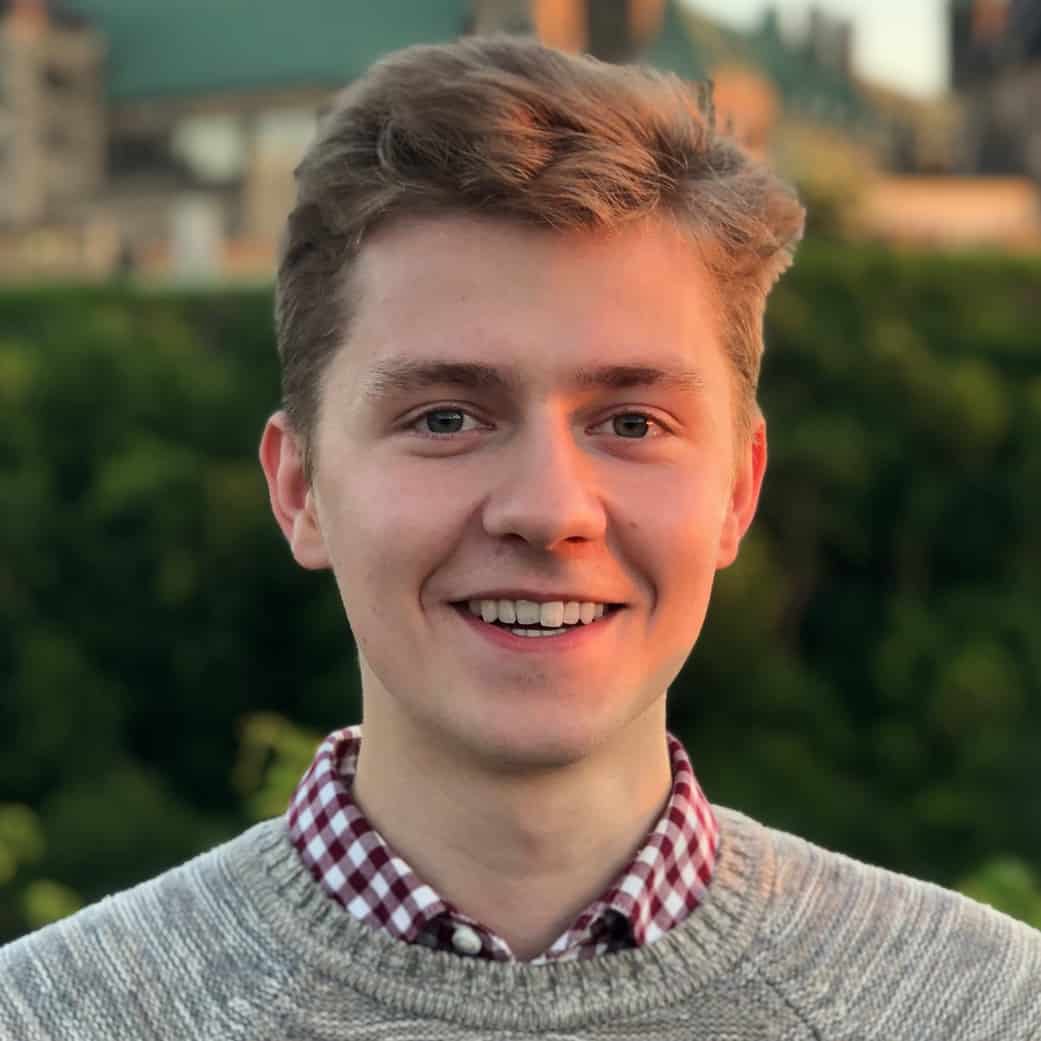
Mykyta Baliesnyi
Why do you care about AI Existential Safety?
Like most people, I want humanity to keep existing and live better with time. AIX risk is one of the key threats to that desire, both on its own and as an amplifier of other threats. On the flipside, resolving it is hence a unique opportunity to do great good for the world.
Please give one or more examples of research interests relevant to AI existential safety:
Here are two examples of research directions I am interested in:
(a) More efficient reward learning. A key approach for learning the reward function in RL is inverse reinforcement learning (IRL) [4]. Recent work on combining learning from demonstrations with active preference elicitation [2] has improved upon IRL sample efficiency by using only a few demonstrations as a prior, and then ”honing in” on the true reward function by asking preference queries. It would be interesting to frame the setup as a meta-learning problem, where the final reward recovered with costly preference learning would be used to improve the prior during training, reducing the number of preference queries necessary at test time.
(b) Task specification in Language models. With language models ever-growing in size and capabilities, aligning them with our intentions efficiently is an important urgent problem. Large LMs can often be steered to be helpful more effectively by the use of in-context prompts rather than through fine-tuning [1]; but the prompt itself occupies useful space in the transformer memory, putting a restriction on how much we can influence its behavior. There has been great progress in this direction, e.g. by using distillation of the prompts back into the model [3], but there is still a lot of space for exploration. For example, it would be interesting to distill larger prompts iteratively in small parts that fit in memory, to leverage smaller but very high-quality demonstrations.
[1] Amanda Askell et al. A General Language Assistant as a Laboratory for Alignment. 2021. arXiv: 2112.00861 [cs.CL].
[2] Erdem Bıyık et al. Learning Reward Functions from Diverse Sources of Human Feedback: Optimally Integrating Demonstrations and Preferences. 2021. arXiv: 2006.14091 [cs.RO].
[3] Yanda Chen et al. Meta-learning via Language Model In-context Tuning. 2021. arXiv: 2110.07814 [cs.CL].
[4] Andrew Y. Ng and Stuart Russell. Algorithms for Inverse Reinforcement Learning. 2000.