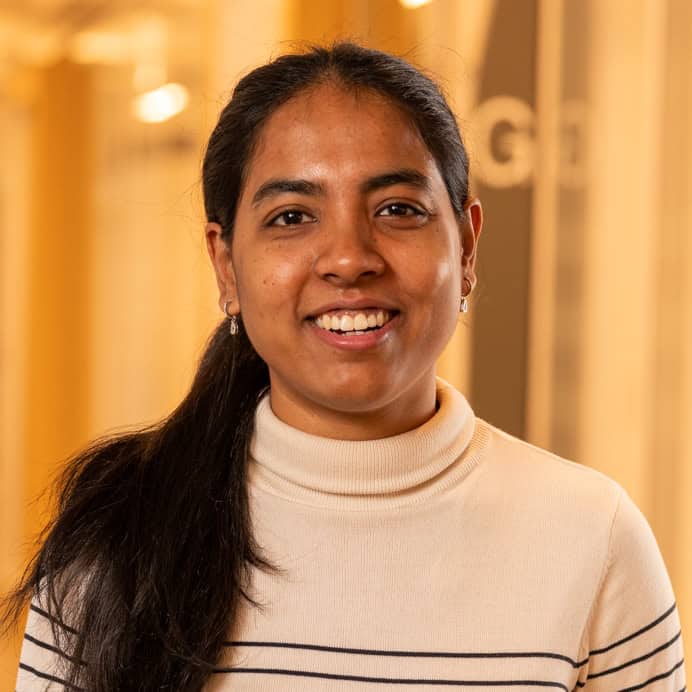
Aneri Muni
Why do you care about AI Existential Safety?
AI and Machine learning holds a great promise for advancing healthcare, agriculture, scientific discovery, and more. From developing autonomous robots for monitoring poultry houses to synthesizing antimicrobial peptides for combating antibiotic resistance, to designing verifiable safety certificates for industrial-processes, I have experienced first-hand the wide-ranging impacts of technology on society. While data-driven methods have proven effective in many online tasks, employing them to make safety-critical decisions in unknown environments is still a challenge. I am passionate about applied research that addresses the broader need for mathematical safety guarantees when deploying learning-based autonomous systems in the real-world. During my PhD, I plan on building tools that bridge the gap between academic research and real-world applications.
Please give at least one example of your research interests related to AI existential safety:
During my masters, I developed algorithms for ensuring safety guarantees during the sim2real transfer of control algorithms. Parameters for data-driven algorithms need to be tuned in order to maximize performance on the real system. Bayesian Optimization (BO) has been used to automate this process. However, in case of safety-critical systems, evaluation of unsafe parameters during the optimization process should be avoided. Recently, a safe BO algorithm, SAFEOPT, was proposed ; it employs Gaussian Processes to only evaluate parameters that satisfy safety constraints with high probability. Even so, it is known that BO does not scale to higher dimensions (d > 20). To overcome this limitation, we proposed the SAFEOPT-HD algorithm that identifies relevant domain regions that efficiently trade-off performance and safety, and restricts BO search to this pre-processed domain. By employing cheap (and potentially inaccurate) simulation models, offline computations are performed using Genetic Search Algorithms, to only consider domain subspaces that are likely to contain optimal policies for a given task, thus significantly reducing domain size. When combined with SAFEOPT, we obtain a safe BO algorithm applicable for problems with large input dimensions. To alleviate the issues due to sparsity of the non-uniform preprocessed domain, a method to systematically generate new controller parameters with desirable properties is implemented. To illustrate its effectiveness, we successfully deployed SAFEOPT-HD for optimizing a 48-dimensional control policy to execute full position control of a quadrotor, while guaranteeing safety.
Currently, my research focuses on risk-sensitive reinforcement learning (RL). I argue that the tradition RL objective to maximize the expected return is insufficient to handle decision making in uncertain scenarios. To overcome this limitations, I leverage tools from the financial literature and incorporate risk measures eg conditional value-at-risk and distortion risk metrics to develop safe RL strategies.