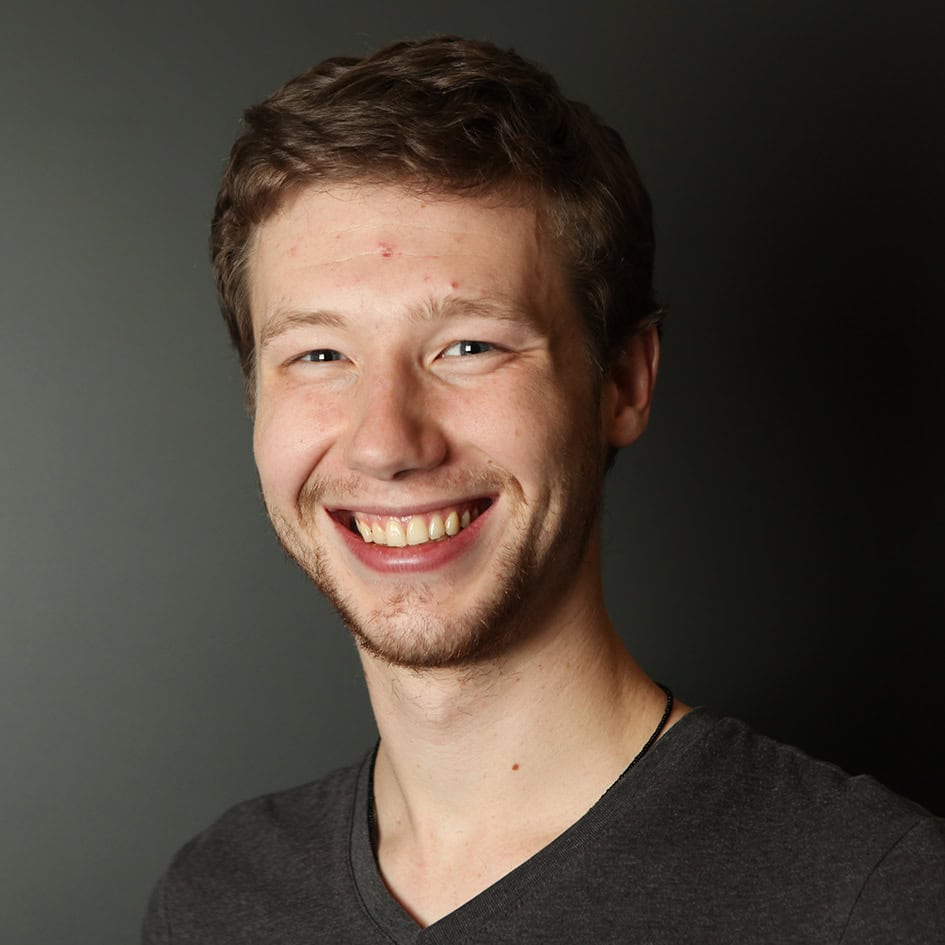
Kai Sandbrink
Why do you care about AI Existential Safety?
The rapid scale-up of AI capabilities and deployment carries large risks for human society. These stem from both unanticipated consequences of powerful AI systems and from their potential misuse. I believe that it is our responsibility as AI researchers and practitioners to take these risks seriously. Through proactive research into the dangers associated with AI and how to counter them, we can mitigate these risks and ensure that AI can work for the benefit of all. A complete strategy for dealing with AI risks will inevitably go beyond the purely technical to take into account the social and political factors that create conditions in which AI technology can be misused and safety neglected. It will also consider social ramifications of deploying AI systems that have the potential for social upheaval. As a PhD student in computational cognitive neuroscience at the University of Oxford and affiliate at Concordia AI, I am particularly invested in how AI systems interact with humans and affect decision-making, and in improving East-West cooperation on AI safety and governance.
Please give at least one example of your research interests related to AI existential safety:
My AI-safety-relevant research interests include improving deep learning’s understanding of uncertainty, and designing safer and more interpretable reward functions for RL algorithms.
Deep learning algorithms struggle to estimate uncertainty effectively, and can make highly-certain but inaccurate judgments in areas such as computer vision, RL, and language processing. As artificial intelligence becomes more agentic, however, a robust understanding of uncertainty becomes increasingly important, since we want the systems to be able to realize when they don’t have information needed to make a particular decision so that they can seek input from humans or delay taking actions as necessary. My first large PhD project working with Christopher Summerfield used RL to model the ability of humans to adapt to changes in environmental controllability. As part of this project, I designed an RL algorithm that estimates uncertainty more effectively by predicting how likely a chosen action is to succeed, mimicking cognitive control structures in humans. We show that this allows the agent to adapt its policy to changes in environmental controllability in situations where traditional meta-RL fails. We show that the algorithm that makes predictions about the environmental controllability also recapitulates human behavior better in decision-making tasks. This paper is currently under review at Nature Neuroscience, but is available as a preprint. I am currently working on expanding this algorithm to other kinds of uncertainty, as I believe it can provide a more general framework, and am interested in developing safety applications of this kind of research more directly.
Another important problem in RL is determining effective reward functions to guide agent behavior. Since the purely task-driven rewards are usually sparse, intrinsic rewards (which are supplied to the agent by itself rather than the environment) are frequently used to supplement the extrinsic reward signal. Handcrafting these intrinsic motivation factors is notoriously difficult, however, as RL agents will frequently find exploits or hacks to maximize its rewards in ways the researcher didn’t consider, resulting in unpredictable behavior. Prior work has looked at using meta-learning in an outer loop to learn an intrinsic reward function that can then be used to guide agent behavior in an inner loop. My project at the Principles of Intelligent Behavior in Biological and Social Systems (PIBBSS) summer research fellowship considered how meta-learning could be used instead to learn an intrinsic motivation function to encourage safe exploration specifically, by guiding agent choices before the agent has taken an action. A variant of this work focusing on how it can also model learning across human development has been published in Proceedings of the Meeting of the Cognitive Society. I am currently supervising a student at EPFL who are working on extensions of this project.