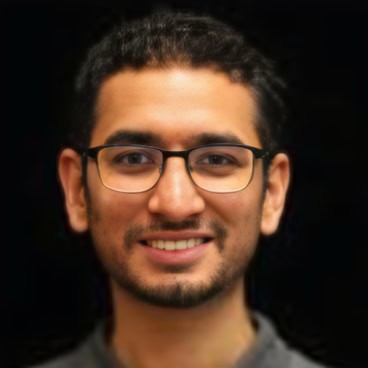
Aashiq Muhamed
Why do you care about AI Existential Safety?
I care deeply about AI existential safety because I believe safeguarding humanity’s future is a profound moral responsibility. The existential risk posed by misaligned superintelligent AI is the threat of permanent foreclosure of humanity’s vast future—trillions of lives and boundless possibilities spanning astronomical timescales. This danger arises from the likely pursuit of instrumentally convergent subgoals such as resource acquisition and self-preservation by superintelligent systems irrespective of their ultimate objectives, which creates the potential for rapid, irreversible changes that could culminate in human extinction. Beyond sudden, catastrophic failures, I am equally concerned by the less-discussed, but insidious, gradual, accumulative erosion of societal resilience culminating in an irreversible collapse. This dual threat—immediate and long-term—demands significant advances in aligning AI with human values and mitigating the dangers of concentrated power. My research in mechanistic interpretability and the democratization of AI is a direct response to what I view as the most critical challenge to humanity’s continued flourishing.
Please give at least one example of your research interests related to AI existential safety:
My research directly addresses existential risks from advanced AI through two interconnected directions: interpretability and democratizing AI.
Interpretability for Alignment
The core challenge with increasingly powerful AIs lies in the mismatch between their external behavior and internal mechanisms. While these systems may demonstrate strong capabilities on established benchmarks and appear aligned, we cannot fully verify their internal decision-making processes or guarantee consistent behavior beyond those limited test cases. This constitutes a significant risk: we are deploying systems whose internal workings remain fundamentally opaque, particularly concerning given the potential for misaligned AGI to be exploited in domains such as automated warfare, bioterrorism, or autonomous rogue agents.
My interpretability research directly addresses this risk by developing techniques to reveal the internal representations and reasoning processes of LLMs. A concrete example is my work on Specialized Sparse Autoencoders (SSAEs). Standard Sparse Autoencoders (SAEs) offer a promising path toward disentangling LLM activations into monosemantic, interpretable features. However, they do not capture rare safety-relevant concepts without impractically large model widths. SSAEs overcome this limitation by illuminating rare features in specific subdomains. By finetuning with Tilted Empirical Risk Minimization on subdomain-specific data selected via dense retrieval from the pretraining corpora, SSAEs achieve a Pareto improvement over existing SAEs in the spectrum of concepts captured.
Democratizing AI: Mitigating the Risks of Concentrated Power
Even with perfect technical alignment, concentrated control of superintelligent AI presents a separate existential risk. Open-source development serves as a critical countermeasure by enabling early detection of alignment failures and democratic oversight of AI behavior. My research contributes to this democratization through improved efficiency across training, deployment, and communication.
My work on training efficiency includes GRASS, an optimizer employing sparse projections to drastically reduce the memory requirements for training LLMs. GRASS made it possible to pretrain 13B parameter LLMs on a single 40GB GPU, lowering the barrier to entry for large-model training. My research on deployment efficiency led to the development of ReAugKD, a technique that augments student models with a non-parametric memory derived from teacher representations. This improves test-time performance with minimal additional computational overhead.
By making the development and deployment of powerful AI systems more accessible and collaborative, we can mitigate the risks associated with concentrated power and increase the probability that these technologies are developed and utilized responsibly, for the benefit of all humanity rather than a privileged few.