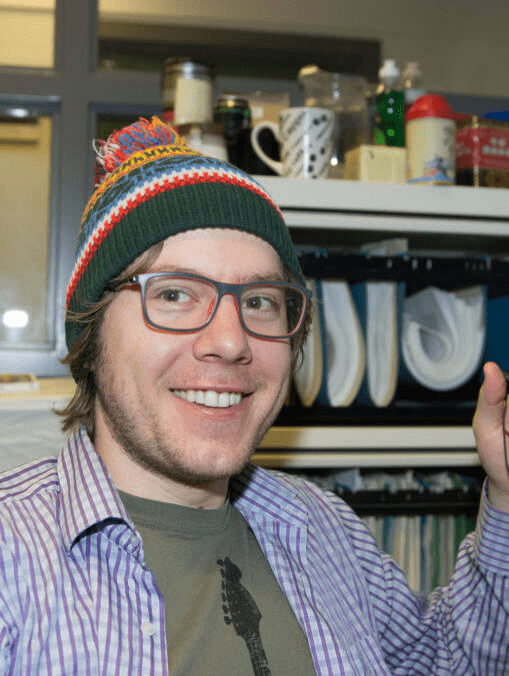
David Krueger
Why do you care about AI Existential Safety?
I got into AI because I was worried about the societal impacts of advanced AI systems, and x-risk in particular. We are not prepared – as a field, society, or species – for AGI, prepotent AI, or many other possible forms of transformative AI. This is an unprecedented global coordination challenge. Technical research may play an important role, but is unlikely to play a decisive one. I consider addressing this problem an ethical priority.
Please give one or more examples of research interests relevant to AI existential safety:
The primary goal of my work is to increase AI existential safety. My main areas of expertise are Deep Learning and AI Alignment. I am also interested in governance and technical areas relevant for global coordination, such as mechanism design.
I am interested in any areas relevant to AI x-safety. My main interests at the moment are in:
- New questions and possibilities presented by large “foundation models” and other putative “proto-AGI” systems. For instance, Machine Learning-based Alignment researchers have emphasized our ability to inspect and train models. But foundation models roughly match the “classic” threat model of “there is a misaligned black-box AI agent that we need to somehow do something aligned with”. An important difference is that these models do not appear to be “agentic” and are trained offline. Will foundation models exhibit emergent forms of agency, e.g. due to mesa-optimization? Will models trained offline understand the world properly, or will they suffer from spurious dependencies and causal confusion? How can we safely leverage the capabilities of misaligned foundation models?
- Understanding Deep Learning, especially learning and generalization, especially systematic and out-of-distribution generalization, and especially invariant prediction. (How) can we get Deep Learning systems to understand and view the world the same way humans do? How can we get them to generalize in the ways we intend?
- Preference Learning, especially Reward Modelling. I think reward modelling is among the most promising approaches to alignment in the short term, although it would likely require good generalization (2), and still involves using Reinforcement Learning, with the attendant concerns about instrumental goals (4).
- Controlling instrumental goals, e.g. to manipulate users of content recommendation systems, e.g. by studying and managing incentives of AI systems. Can we find ways for AI systems to do long-term planning that don’t engender dangerous instrumental goals?
Some quick thoughts on governance and global coordination:
- A key challenge seems to be: clearly defining which categories of systems should be subject to which kind of oversight, standards, or regulation. For instance, “automated decision-making (ADM)” seems like a crisper concept than “Artificial Intelligence” at the moment, but neither category is fine-grained enough.
- I think we will need substantial involvement from AI experts in governance, and expect most good work to be highly interdisciplinary. I would like to help promote such research.
- I am optimistic about the vision of RadicalXChange as a direction for solving coordination problems in the longer run.