The Unavoidable Problem of Self-Improvement in AI: An Interview with Ramana Kumar, Part 1
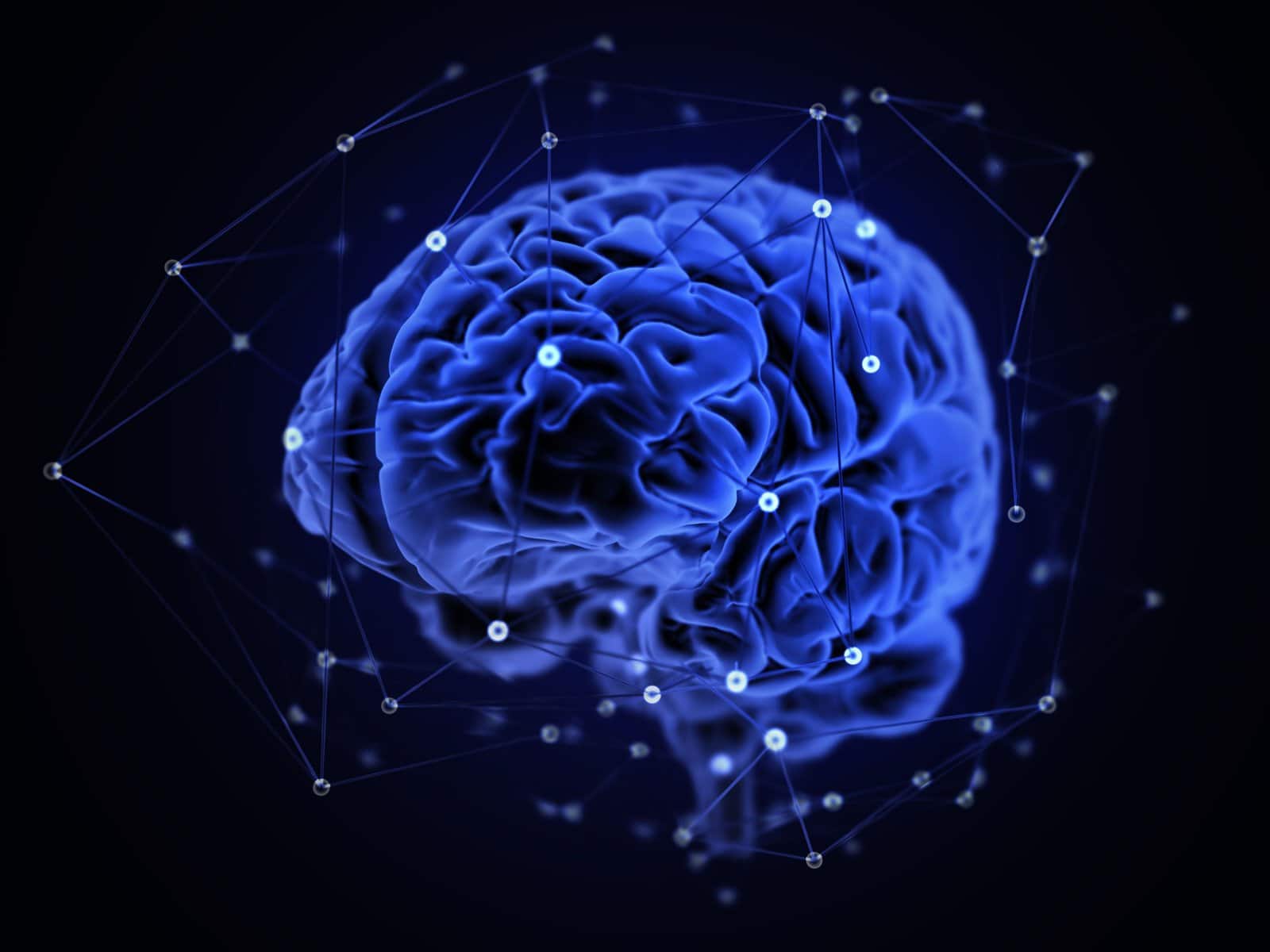
Contents
Today’s AI systems may seem like intellectual powerhouses that are able to defeat their human counterparts at a wide variety of tasks. However, the intellectual capacity of today’s most advanced AI agents is, in truth, narrow and limited. Take, for example, AlphaGo. Although it may be the world champion of the board game Go, this is essentially the only task that the system excels at.
Of course, there’s also AlphaZero. This algorithm has mastered a host of different games, from Japanese and American chess to Go. Consequently, it is far more capable and dynamic than many contemporary AI agents; however, AlphaZero doesn’t have the ability to easily apply its intelligence to any problem. It can’t move unfettered from one task to another the way that a human can.
The same thing can be said about all other current AI systems — their cognitive abilities are limited and don’t extend far beyond the specific task they were created for. That’s why Artificial General Intelligence (AGI) is the long-term goal of many researchers.
Widely regarded as the “holy grail” of AI research, AGI systems are artificially intelligent agents that have a broad range of problem-solving capabilities, allowing them to tackle challenges that weren’t considered during their design phase. Unlike traditional AI systems, which focus on one specific skill, AGI systems would be able efficiently to tackle virtually any problem that they encounter, completing a wide range of tasks.
If the technology is ever realized, it could benefit humanity in innumerable ways. Marshall Burke, an economist at Stanford University, predicts that AGI systems would ultimately be able to create large-scale coordination mechanisms to help alleviate (and perhaps even eradicate) some of our most pressing problems, such as hunger and poverty. However, before society can reap the benefits of these AGI systems, Ramana Kumar, an AGI safety researcher at DeepMind, notes that AI designers will eventually need to address the self-improvement problem.
Self-Improvement Meets AGI
Early forms of self-improvement already exist in current AI systems. “There is a kind of self-improvement that happens during normal machine learning,” Kumar explains; “namely, the system improves in its ability to perform a task or suite of tasks well during its training process.”
However, Kumar asserts that he would distinguish this form of machine learning from true self-improvement because the system can’t fundamentally change its own design to become something new. In order for a dramatic improvement to occur — one that encompasses new skills, tools, or the creation of more advanced AI agents — current AI systems need a human to provide them with new code and a new training algorithm, among other things.
Yet, it is theoretically possible to create an AI system that is capable of true self-improvement, and Kumar states that such a self-improving machine is one of the more plausible pathways to AGI.
Researchers think that self-improving machines could ultimately lead to AGI because of a process that is referred to as “recursive self-improvement.” The basic idea is that, as an AI system continues to use recursive self-improvement to make itself smarter, it will get increasingly better at making itself smarter. This will quickly lead to an exponential growth in its intelligence and, as a result, could eventually lead to AGI.
Kumar says that this scenario is entirely plausible, explaining that, “for this to work, we need a couple of mostly uncontroversial assumptions: that such highly competent agents exist in theory, and that they can be found by a sequence of local improvements.” To this extent, recursive self-improvement is a concept at the heart of a number of theories on how we can get from today’s moderately smart machines to super-intelligent AGI. However, Kumar clarifies that this isn’t the only potential pathway to AI superintelligences.
Humans could discover how to build highly competent AGI systems through a variety of methods. This might happen “by scaling up existing machine learning methods, for example, with faster hardware. Or it could happen by making incremental research progress in representation learning, transfer learning, model-based reinforcement learning, or some other direction. For example, we might make enough progress in brain scanning and emulation to copy and speed up the intelligence of a particular human,” Kumar explains.
Yet, he is also quick to clarify that recursive self-improvement is an innate characteristic of AGI. “Even if iterated self-improvement is not necessary to develop highly competent artificial agents in the first place, explicit self-improvement will still be possible for those agents,” Kumar said.
As such, although researchers may discover a pathway to AGI that doesn’t involve recursive self-improvement, it’s still a property of artificial intelligence that is in need of serious research.
Safety in Self-Improving AI
When systems start to modify themselves, we have to be able to trust that all their modifications are safe. This means that we need to know something about all possible modifications. But how can we ensure that a modification is safe if no one can predict ahead of time what the modification will be?
Kumar notes that there are two obvious solutions to this problem. The first option is to restrict a system’s ability to produce other AI agents. However, as Kumar succinctly sums, “We do not want to solve the safe self-improvement problem by forbidding self-improvement!”
The second option, then, is to permit only limited forms of self-improvement that have been deemed sufficiently safe, such as software updates or processor and memory upgrades. Yet, Kumar explains that vetting these forms of self-improvement as safe and unsafe is still exceedingly complicated. In fact, he says that preventing the construction of one specific kind of modification is so complex that it will “require such a deep understanding of what self-improvement involves that it will likely be enough to solve the full safe self-improvement problem.”
And notably, even if new advancements do permit only limited forms of self-improvement, Kumar states that this isn’t the path to take, as it sidesteps the core problem with self-improvement that we want to solve. “We want to build an agent that can build another AI agent whose capabilities are so great that we cannot, in advance, directly reason about its safety…We want to delegate some of the reasoning about safety and to be able to trust that the parent does that reasoning correctly,” he asserts.
Ultimately, this is an extremely complex problem that is still in its most nascent stages. As a result, much of the current work is focused on testing a variety of technical solutions and seeing where headway can be made. “There is still quite a lot of conceptual confusion about these issues, so some of the most useful work involves trying different concepts in various settings and seeing whether the results are coherent,” Kumar explains.
Regardless of what the ultimate solution is, Kumar asserts that successfully overcoming the problem of self-improvement depends on AI researchers working closely together. “The key to is to make assumptions explicit, and, for the sake of explaining it to others, to be clear about the connection to the real-world safe AI problems we ultimately care about.”
Read Part 2 here.
About the Future of Life Institute
The Future of Life Institute (FLI) is a global think tank with a team of 20+ full-time staff operating across the US and Europe. FLI has been working to steer the development of transformative technologies towards benefitting life and away from extreme large-scale risks since its founding in 2014. Find out more about our mission or explore our work.
Related content
Other posts about AI, AI Research, Grants Program, Recent News
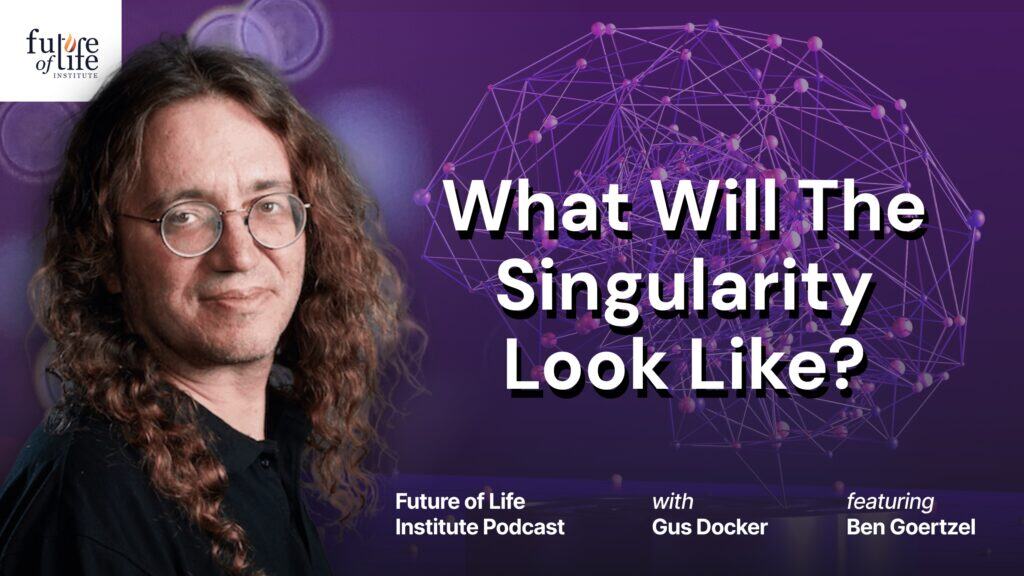
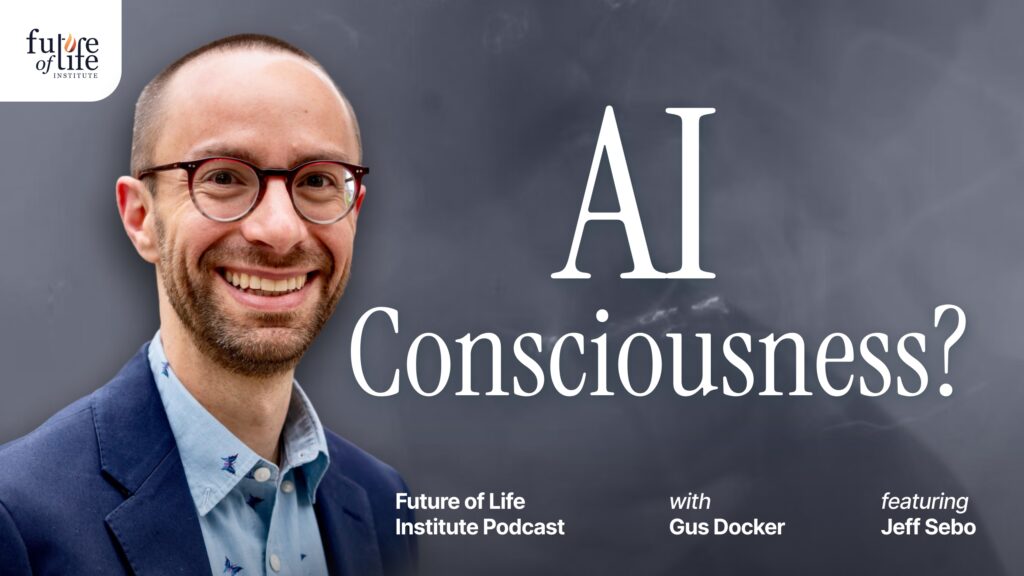