FLI Podcast: AI Breakthroughs and Challenges in 2018 with David Krueger and Roman Yampolskiy
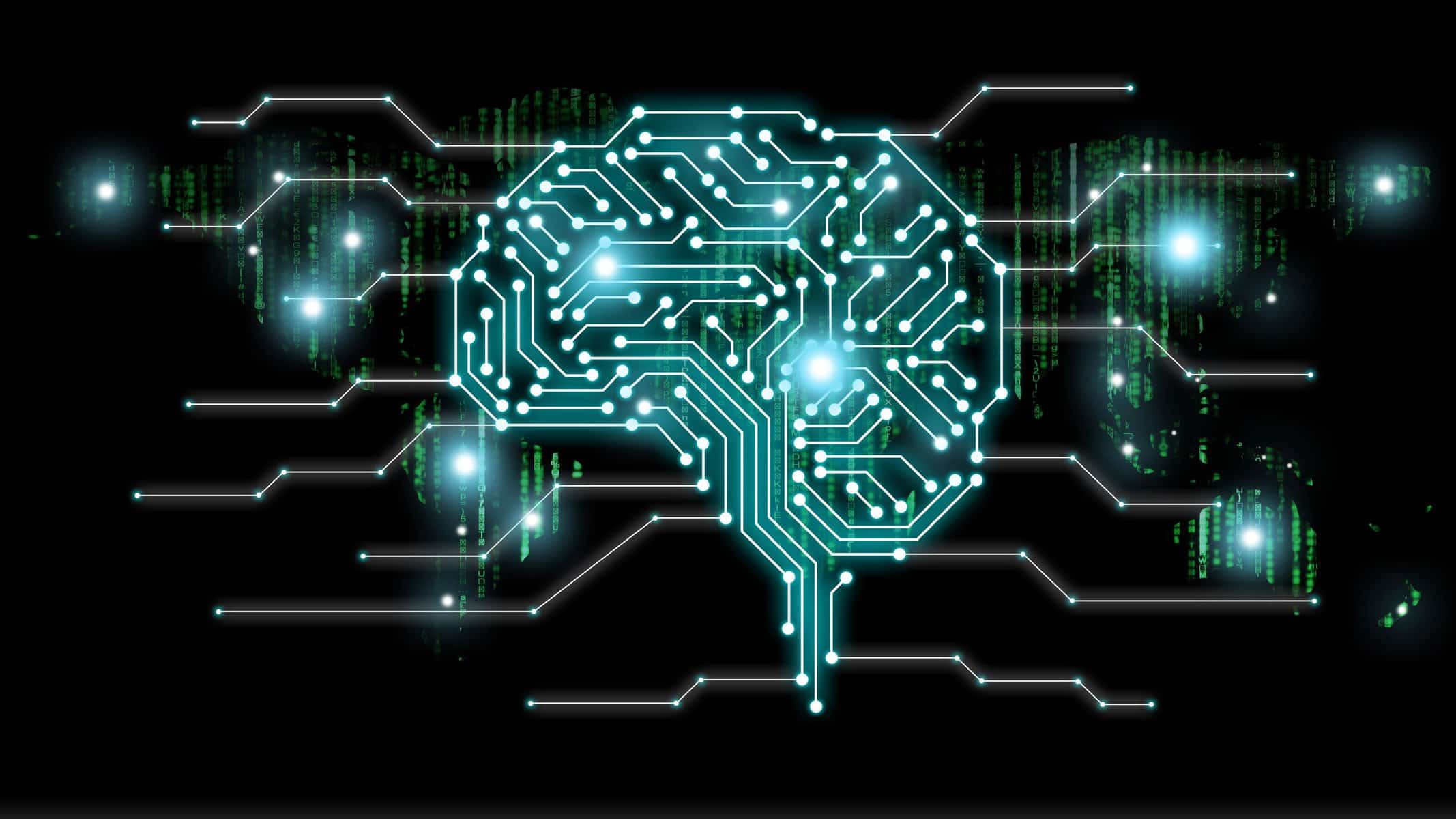
Every January, we like to look back over the past 12 months at the progress that’s been made in the world of artificial intelligence. Welcome to our annual “AI breakthroughs” podcast, 2018 edition.
Ariel was joined for this retrospective by researchers Roman Yampolskiy and David Krueger. Roman is an AI Safety researcher and professor at the University of Louisville. He also recently published the book Artificial Intelligence Safety & Security. David is a PhD candidate in the Mila lab at the University of Montreal, where he works on deep learning and AI safety. He's also worked with safety teams at the Future of Humanity Institute and DeepMind and has volunteered with 80,000 hours.
Roman and David shared their lists of 2018’s most promising AI advances, as well as their thoughts on some major ethical questions and safety concerns. They also discussed media coverage of AI research, why talking about “breakthroughs” can be misleading, and why there may have been more progress in the past year than it seems.
Topics discussed in this podcast include:
- DeepMind progress, as seen with AlphaStar and AlphaFold
- Manual dexterity in robots, especially QT Opt and Dactyl
- Advances in creativity, as with Generative Adversarial Networks (GANs)
- Feature-wise transformations
- Continuing concerns about DeepFakes
- Scaling up AI systems
- Neuroevolution
- Google Duplex, the AI assistant that sounds human on the phone
- The General Data Protection Regulation (GDPR) and AI policy more broadly
Publications discussed in this podcast include:
- Supervising strong learners by amplifying weak experts (OpenAI)
- AI safety via debate (OpenAI)
- Scalable agent alignment via reward modeling: a research direction (DeepMind)
- AI and Compute (OpenAI)
- A Style-Based Generator Architecture for Generative Adversarial Networks
- IMPALA: Scalable Distributed Deep-RL with Importance Weighted Actor-Learner Architectures
- ImageNet/ResNet-50 Training in 224 Seconds
- Attention Is All You Need
Transcript
Ariel: Hi everyone, welcome to the FLI podcast. I'm your host, Ariel Conn. For those of you who are new to the podcast, at the end of each month, I bring together two experts for an in-depth discussion on some topic related to the fields that we at the Future of Life Institute are concerned about, namely artificial intelligence, biotechnology, climate change, and nuclear weapons.
The last couple of years for our January podcast, I've brought on two AI researchers to talk about what the biggest AI breakthroughs were in the previous year, and this January is no different. To discuss the major developments we saw in AI in 2018, I'm pleased to have Roman Yampolskiy and David Krueger joining us today.
Roman is an AI safety researcher and professor at the University of Louisville, his new book Artificial Intelligence Safety and Security is now available on Amazon and we'll have links to it on the FLI page for this podcast. David is a PhD candidate in the Mila Lab at the University of Montreal, where he works on deep learning and AI safety. He's also worked with teams at the Future of Humanity Institute and DeepMind, and he's volunteered with 80,000 Hours to help people find ways to contribute to the reduction of existential risks from AI. So Roman and David, thank you so much for joining us.
David: Yeah, thanks for having me.
Roman: Thanks very much.
Ariel: So I think that one thing that stood out to me in 2018 was that the AI breakthroughs seemed less about surprising breakthroughs that really shook the AI community as we've seen in the last few years, and instead they were more about continuing progress. And we also didn't see quite as many major breakthroughs hitting the mainstream press. There were a couple of things that made big news splashes, like Google Duplex, which is a new AI assistant program that sounded incredibly human on phone calls it made during the demos. And there was also an uptick in government policy and ethics efforts, especially with the General Data Protection Regulation, also known as the GDPR, which went into effect in Europe earlier this year.
Now I'm going to want to come back to Google and policy and ethics later in this podcast, but I want to start by looking at this from the research and development side of things. So my very first question for both of you is: do you agree that 2018 was more about impressive progress, and less about major breakthroughs? Or were there breakthroughs that really were important to the AI community that just didn't make it into the mainstream press?
David: Broadly speaking I think I agree, although I have a few caveats for that. One is just that it's a little bit hard to recognize always what is a breakthrough, and a lot of the things in the past that have had really big impacts didn't really seem like some amazing new paradigm shift—it was sort of a small tweak that then made a lot of things work a lot better. And the other caveat is that there are a few works that I think are pretty interesting and worth mentioning, and the field is so large at this point that it's a little bit hard to know if there aren't things that are being overlooked.
Roman: So I'll agree with you, but I think the pattern is more important than any specific breakthrough. We kind of got used to getting something really impressive every month, so relatively it doesn't sound as good, all the AlphaStar, AlphaFold, AlphaZero happening almost every month. And it used to be it took 10 years to see something like that.
It's likely it will happen even more frequently. We'll conquer a new domain once a week or something. I think that's the main pattern we have to recognize and discuss. There are significant accomplishments in terms of teaching AI to work in completely novel domains. I mean now we can predict protein folding, now we can have multi-player games conquered. That never happened before so frequently. Chess was impressive because it took like 30 years to get there.
David: Yeah, so I think a lot of people were kind of expecting or at least hoping for StarCraft or Dota to be solved—to see, like we did with AlphaGo, AI systems that are beating the top players. And I would say that it's actually been a little bit of a let down for people who are optimistic about that, because so far the progress has been kind of unconvincing.
So the AlphaStar, which was a really recent result from last week, for instance: I've seen criticism of it that I think is valid that it was making more actions than a human could within a very short interval of time. So they carefully controlled the actions-per-minute that AlphaStar was allowed to take, but they didn't prevent it from doing really short bursts of actions that really helped its micro-game, and that means that it can win without really being strategically superior to its human opponents. And I think the Dota results that OpenAI has had was also criticized as being sort of not the hardest version of the problem, and still the AI sort of is relying on some crutches.
Ariel: So before we get too far into that debate, can we take a quick step back and explain what both of those are?
David: So these are both real-time strategy games that are, I think, actually the two most popular real-time strategy games in the world that people play professionally, and make money playing. I guess that's all to say about them.
Ariel: So a quick question that I had too about your description then, when you're talking about AlphaStar and you were saying it was just making more moves than a person can realistically make. Is that it—it wasn't doing anything else special?
David: I haven't watched the games, and I don't play StarCraft, so I can't say that it wasn't doing anything special. I'm basing this basically on reading articles and reading the opinions of people who are avid StarCraft players, and I think the general opinion seems to be that it is more sophisticated than what we've seen before, but the reason that it was able to win these games was not because it was out-thinking humans, it's because it was out-clicking, basically, in a way that just isn't humanly possible.
Roman: I would agree with this analysis, but I don't see it as a bug, I see it as a feature. That just shows another way machines can be superior to people. Even if they are not necessarily smarter, they can still produce superior performance, and that's what we really care about. Right? We found a different way, a non-human approach to solving this problem. That's impressive.
David: Well, I mean, I think if you have an agent that can just click as fast as it wants, then you can already win at StarCraft, before this work. There needs to be something that makes it sort of a fair fight in some sense.
Roman: Right, but think what you're suggesting: We have to handicap machines to make them even remotely within being comparative to people. We're talking about getting to superintelligent performance. You can get there by many ways. You can think faster, you can have better memory, you can have better reaction time—as long as you're winning in whatever domain we're interested in, you have superhuman performance.
David Krueger: So maybe another way of putting this would be if they actually made a robot play StarCraft and made it use the same interface that humans do, such as a screen and mouse, there's no way that it could have beat the human players. And so by giving it direct access to the game controls, it’s sort of not solving the same problem that a human is when they play this game.
Roman: I feel what you're saying, I just feel that it is solving it in a different way, and we have pro-human bias saying, well that's not how you play this game, you have an advantage. Human players usually rely on superior strategy, not just faster movements that may take advantage of it for a few nanoseconds, a couple of seconds. But it's not a long-term sustainable pattern.
One of the research projects I worked on was this idea of artificial stupidity, we called it—kind of limiting machines to human-level capacity. And I think that's what we're talking about it here. Nobody would suggest limiting a chess program to just human-level memory, or human memorization of opening moves. But we don't see it as a limitation. Machines have an option of beating us in ways humans can't. That's the whole point, and that's why it's interesting, that's why we have to anticipate such problems. That's where most of the safety and security issues will show up.
Ariel: So I guess, I think, Roman, your point earlier was sort of interesting that we've gotten so used to breakthroughs that stuff that maybe a couple of years ago would have seemed like a huge breakthrough is just run-of-the-mill progress. I guess you're saying that that's what this is sort of falling into. Relatively recently this would have been a huge deal, but because we've seen so much other progress and breakthroughs, that this is now interesting and we're excited about it—but it's not reaching that level of, oh my god, this is amazing! Is that fair to say?
Roman: Exactly! We get disappointed if the system loses one game. It used to be we were excited if it would match amateur players. Now it's, oh, we played a 100 games and you lost one? This is just not machine-level performance, you disappoint us.
Ariel: David, do you agree with that assessment?
David: I would say mostly no. I guess, I think what really impressed me with AlphaGo and AlphaZero was that it was solving something that had been established as a really grand challenge for AI. And then in the case of AlphaZero, I think the technique that they actually used to solve it was really novel and interesting from a research point of view, and they went on to show that this same technique can solve a bunch of other board games as well.
And my impression from what I've seen about how they did AlphaStar and AlphaFold is that there were some interesting improvements and the performance is impressive but I think it's neither, like, quite at the point where you can say we've solved it, we're better than everybody, or in the case of protein folding, there's not a bunch more room for improvement that has practical significance. And it's also—I don't see any really clear general algorithmic insights about AI coming out of these works yet. I think that's partially because they haven't been published yet, but from what I have heard about the details about how they work, I think it's less of a breakthrough on the algorithm side than AlphaZero was.
Ariel: So you've mentioned AlphaFold. Can you explain what that is real quick?
David: This is the protein folding project that DeepMind did, and I think there's a competition called C-A-S-P or CASP that happens every three years, and they sort of dominated that competition this last year doing what was described as two CASPs in one, so basically doubling the expected rate of improvement that people have seen historically at these tasks, or at least at the one that is the most significant benchmark.
Ariel: I find the idea of the protein folding thing interesting because that's something that's actually relevant to scientific advancement and health as opposed to just being able to play a game. Are we seeing actual applications for this yet?
David: I don't know about that, but I agree with you that that is a huge difference that makes it a lot more exciting than some of the previous examples. I guess one thing that I want to say about that, though, is that it does look a little bit more to me like continuation of progress that was already happening in the communities. It's definitely a big step up, but I think a lot of the things that they did there could have really happened over the next few years anyways, even without DeepMind being there. So, one of the articles I read put it this way: If this wasn't done by DeepMind, if this was just some academic group, would this have been reported in the media? I think the answer is sort of like a clear no, and that says something about the priorities of our reporting and media as well as the significance of the results, but I think that just gives some context.
Roman: I'll agree with David—the media is terrible in terms of what they report on, we can all agree on that. I think it was quite a breakthrough, I mean, to say that they not just beat the competition, but to actually kind of doubled performance improvement. That's incredible. And I think anyone who got to that point would not be denied publication in a top journal; It would be considered very important in that domain. I think it's one of the most important problems in medical research. If you can accurately predict this, possibilities are really endless in terms of synthetic biology, in terms of curing diseases.
So this is huge in terms of impact from being able to do it. As far as how applicable is it to other areas, is it a great game-changer for AI research? All those things can adapt between this ability to perform in real-life environments of those multiplayer games, and being able to do this. Look at how those things can be combined. Right? You can do things in the real world you couldn't do before, both in terms of strategy games, which are basically simulations for economic competition, for wars, for quite a few applications where impact would be huge.
So all of it is very interesting. It's easy to say that, "Well if they didn't do it, somebody else maybe would do it in a couple of years." But it's almost always true for all inventions. If you look at the history of inventions, things like, I don't know, telephone, have been invented at the same time by two or three people; radio, two or three people. It's just the point where science gets enough ingredient technology where yeah, somebody's going to do it, nice. But still, we give credit to whoever got there first.
Ariel: So I think that's actually a really interesting point, because I think for the last few years we have seen sort of these technological advances but I guess we also want to be considering the advances that are going to have a major impact on humanity even if it's not quite as technologically new.
David: Yeah, absolutely. I think the framing in terms of breakthroughs is a little bit unclear what we're talking about when we talk about AI breakthroughs, and I think a lot of people in the field of AI kind of don't like how much people talk about it in terms of breakthroughs because a lot of the progress is gradual and builds on previous work and it's not like there was some sudden insight that somebody had that just changed everything, although that does happen in some ways.
And I think you can think of the breakthroughs both in terms of like what is the impact—is this suddenly going to have a lot of potential to change the world? You can also think of it, though, from the perspective of researchers as like, is this really different from the kind of ideas and techniques we've seen or seen working before? I guess I'm more thinking about the second right now in terms of breakthroughs representing really radical new ideas in research.
Ariel: Okay, well I will take responsibility for being one of the media people who didn't do a good job with presenting AI breakthroughs. But I think both with this podcast and probably moving forward, I think that is actually a really important thing for us to be doing—is both looking at the technological progress and newness of something but also the impact it could have on either society or future research.
So with that in mind, you guys also have a good list of other things that did happen this year, so I want to start moving into some of that as well. So next on your list is manual dexterity in robots. What did you guys see happening there?
David: So this is something that's definitely not my area of expertise, so I can't really comment too much on it. But there are two papers that I think are significant and potentially representing something like a breakthrough in this application. In general robotics is really difficult, and machine learning for robotics is still, I think, sort of a niche thing, like most robotics is using more classical planning algorithms, and hasn't really taken advantage of the new wave of deep learning and everything.
So there's two works, one is QT-Opt, and the other one is Dactyl, and these are both by people from the Berkeley OpenAI crowd. And these both are showing kind of impressive results in terms of manual dexterity in robots. So there's one that does a really good job at grasping, which is one of the basic aspects of being able to act in the real world. And then there's another one that was sort of just manipulating something like a cube with different colored faces on it—that one's Dactyl; the grasping one is QT-Opt.
And I think this is something that was paid less attention to in the media, because it's been more of a story of kind of gradual progress I think. But my friend who follows this deep reinforcement learning stuff more told me that QT-Opt is the first convincing demonstration of deep reinforcement learning in the real world, as opposed to all these things we've seen in games. The real world is much more complicated and there's all sorts of challenges with the noise of the environment dynamics and contact forces and stuff like this that have been really a challenge for doing things in the real world. And then there's also the limited sample complexity where when you play a game you can sort of interact with the game as much as you want and play the game over and over again, whereas in the real world you can only move your robot so fast and you have to worry about breaking it, so that means in the end you can collect a lot less data, which makes it harder to learn things.
Roman: Just to kind of explain maybe what they did. So hardware's expensive, slow: It's very difficult to work with. Things don't go well in real life; It's a lot easier to create simulations in virtual worlds, train your robot in there, and then just transfer knowledge into a real robot in the physical world. And that's exactly what they did, training that virtual hand to manipulate objects, and they could run through thousands, millions of situations and then it's something you cannot do with an actual, physical robot at that scale. So, I think that's a very interesting approach for why lots of people try doing things in virtual environments. Some of the early AGI projects all concentrated on virtual worlds as domain of learning. So that makes a lot of sense.
David: Yeah, so this was for the Dactyl project, which was OpenAI. And that was really impressive I think, because people have been doing this sim-to-real thing—where you train in simulation and then try and transfer it to the real world—with some success for like a year or two, but this one I think was really kind of impressive in that sense, because they didn't actually train it in the real world at all, and what they had learned managed to transfer to the real world.
Ariel: Excellent. I'm going to keep going through your list. One thing that you both mentioned are GANs. So very quickly, if one of you, or both of you, could explain what a GAN is and what that stands for, and then we'll get into what happened last year with those.
Roman: Sure, so this is a somewhat new way of doing creative generational visuals and audio. You have two neural networks competing, one is kind of creating fakes, and the other one is judging them, and you get to a point where they're kind of 50/50. You can't tell if it's fake or real anymore. And it's a great way to produce artificial faces, cars, whatever. Any type of input you can provide to the networks, they quickly learn to extract the essence of that image or audio and generate artificial data sets full of such images.
And there's really exciting work on being able to extract properties from those, different styles. So if we talk about faces, for example: there could be a style for hair, a style for skin color, a style for age, and now it's possible to manipulate them. So I can tell you things like, "Okay, Photoshop, I need a picture of a female, 20 years old, blonde, with glasses," and it would generate a completely realistic face based on those properties. And we're starting to see it show up not just in images but transferred to video, to generating whole virtual worlds. It's probably the closest thing we ever had computers get to creativity: actually kind of daydreaming and coming up with novel outputs.
David: Yeah, I just want to say a little bit about the history of the research in GAN. So the first work on GANs was actually back four or five years ago in 2014, and I think it was actually kind of—didn't make a huge splash at the time, but maybe a year or two after that it really started to take off. And research in GANs over the last few years has just been incredibly fast-paced and there's been hundreds of papers submitted and published at the big conferences every year.
If you look just in terms of the quality of what is generated, this is, I think, just an amazing demonstration of the rate of progress in some areas of machine learning. The first paper had these sort of black and white pictures of really blurry faces, and now you can get giant—I think 256 by 256, or 512 by 512, or even bigger—really high resolution and totally indistinguishable from real photos, to the human eye anyway—images of faces. So it's really impressive, and we've seen really consistent progress on that, especially in the last couple years.
Ariel: And also, just real quick, what does it stand for?
David: Oh, generative adversarial network. So it's generative, because it's sort of generating things from scratch, or from its imagination or creativity. And it's adversarial because there are two networks: the one that generates the things, and then the one that tries to tell those fake images apart from real images that we actually collect by taking photos in the world.
Ariel: This is an interesting one because it can sort of transition into some ethics stuff that came up this past year, but I'm not sure if we want to get there yet, or if you guys want to talk a little bit more about some of the other things that happened on the research and development side.
David: I guess I want to talk about a few other things that have been making, I would say, sort of steady progress, like GANs. With a lot of interest in, I guess I would say, their ideas that are coming to fruition, even though some of these are not exactly from the last year, they sort of really started to improve themselves and become widely used in the last year.
Ariel: Okay.
David: I think this is actually used in maybe the latest, greatest GAN paper, is something that's called feature-wise transformations. So this is an idea that actually goes back up to 40 years, depending on how you measure it, but has sort of been catching on in specific applications in machine learning in the last couple of years—starting with, I would say, style-transfer, which is sort of like what Roman mentioned earlier.
So the idea here is that in a neural network, you have what are called features, which basically correspond to the activations of different neurons in the network. Like how much that neuron likes what it's seeing, let's say. And those can also be interpreted as representing different kinds of visual patterns, like different kinds of textures, or colors. And these feature-wise transformations basically just take each of those different aspects of the image, like the color or texture in a certain location, and then allow you to manipulate that specific feature, as we call it, by making it stronger or amplifying whatever was already there.
And so you can sort of view this as a way of specifying what sort of things are important in the image, and that's why it allows you to manipulate the style of images very easily, because you can sort of look at a certain painting style for instance, and say, oh this person uses a lot of wide brush strokes, or a lot of narrow brush strokes, and then you can say, I'm just going to modulate the neurons that correspond to wide or narrow brush strokes, and change the style of the painting that way. And of course you don't do this by hand, by looking in and seeing what the different neurons represent. This all ends up being learned end-to-end. And so you sort of have an artificial intelligence model that predicts how to modulate the features within another network, and that allows you to change what that network does in a really powerful way.
So, I mentioned that it has been applied in the most recent GAN papers, and I think they're just using those kinds of transformations to help them generate images. But other examples where you can explain what's happening more intuitively, or why it makes sense to try and do this, would be something like visual question answering. So there you can have the modulation of the vision network being done by another network that looks at a question and is trying to help answer that question. And so it can sort of read the question and see what features of images might be relevant to answering that question. So for instance, if the question was, "Is it a sunny day outside?" then it could have the vision network try and pay more attention to things that correspond to signs of sun. Or if it was asked something like, "Is this person's hair combed?" then you could look for the patterns of smooth, combed hair and look for the patterns of rough, tangled hair, and have those features be sort of emphasized in the vision network. That allows the vision network to pay attention to the parts of the image that are most relevant to answering the question.
Ariel: Okay. So, Roman, I want to go back to something on your list quickly in a moment, but first I was wondering if you have anything that you wanted to add to the feature-wise transformations?
Roman: All of it, you can ask, "Well why is this interesting, what are the applications for it?" So you are able to generate inputs, inputs for computers, inputs for people, images, sounds, videos. A lot of times they can be adversarial in nature as well—what we call deep fakes. Right? You can make, let's say, a video of a famous politician say something, or do something.
Ariel: Yeah.
Roman: And this has very interesting implications for elections, for forensic science, for evidence. As those systems get better and better, it becomes harder and harder to tell if something is real or not. And maybe it's still possible to do some statistical analysis, but it takes time, and we talked about media being not exactly always on top of it. So it may take 24 hours before we realize if this video was real or not, but the election is tonight.
Ariel: So I am definitely coming back to that. I want to finish going through the list of the technology stuff, but yeah I want to talk about deep fakes and in general, a lot of the issues that we've seen cropping up more and more with this idea of using AI to fake images and audio and video, because I think that is something that's really important.
David: Yeah, it's hard for me to estimate these things, but I would say this is probably, in terms of the impact that this is going to have societally, this is sort of the biggest story maybe of the last year. And it's not like something that happened all of the sudden. Again, it's something that has been building on a lot of progress in generative models and GANs and things like this. And it's just going to continue, we're going to see more and more progress like that, and probably some sort of arms' race here where—I shouldn't use that word.
Ariel: A competition.
David: A competition between people who are trying to use that kind of technology to fake things and people who are sort of doing forensics to try and figure out what is real and what is fake. And that also means that people are going to have to trust the people who have the expertise to do that, and believe that they're actually doing that and not part of some sort of conspiracy or something.
Ariel: Alright, well are you guys ready to jump into some of those ethical questions?
David: Well, there are like two other broad things I wanted to mention, which I think are sort of interesting trends in the research community. One is just the way that people have been continuing to scale up AI systems. So a lot of the progress I think has arguably just been coming from more and more computation and more and more data. And there was a pretty great blog post by OpenAI about this last year that argued that the amount of computation that's being used to train the most advanced AI systems is increasing by a factor of 10 times every year for the last several years, which is just astounding. But it also suggests that this might not be sustainable for a long time, so to the extent that you think that using more computation is a big driver of progress, we might start to see that slow down within a decade or so.
Roman: I'll add another—what I think also is kind of building-on technology, not so much a breakthrough, we had it for a long time—but neural evolution is something I'm starting to pay a lot more attention to and that's kind of borrowing from biology, trying to evolve ways for neural networks, optimized neural networks. And it's producing very impressive results. It's possible to run it in parallel really well, and it's competitive with some of the leading alternative approaches.
So, the idea basically is you have this very large neural network, brain-like structure, but instead of trying to train it back, propagate errors, teach it in a standard neural networks way, you just kind of have a population of those brains competing for who's doing best in a particular problem, and they share weights between good parents, and after a while you just evolve really well performing solutions to some of the most interesting problems.
Additionally you can kind of go meta-level on it and evolve architectures for the neural network itself—how many layers, how many inputs. This is nice because it doesn't require much human intervention. You're essentially letting the system figure out what the solutions are. We had some very successful results with genetic algorithms for optimization. We didn't have much success with genetic programming, and now neural evolution kind of brings it back where you're optimizing intelligence systems, and that's very exciting.
Ariel: So you're saying that you'll have—to make sure I understand this correctly—there's two or more neural nets trying to solve a problem, and they sort of play off of each other?
Roman: So you create a population of neural networks, and you give it a problem, and you see this one is doing really well, and that one. The others, maybe not so great. So you take weights from those two and combine them—like mom and dad, parent situation that produces offspring. And so you have this simulation of evolution where unsuccessful individuals are taken out of a population. Successful ones get to reproduce and procreate, and provide their high fitness weights to the next generation.
Ariel: Okay. Was there anything else that you guys saw this year that you want to talk about, that you were excited about?
David: Well I wanted to give a few examples of the kind of massive improvements in scale that we've seen. One of the most significant models and benchmarks in the community is ImageNet and training image classifiers that can tell you what a picture is a picture of on this dataset.So the whole sort of deep learning revolution was arguably started, or at least really came into the eyes of the rest of the machine learning community, because of huge success on this ImageNet competition. And training the model there took something like two weeks, and this last year there was a paper where you can train a more powerful model in less than four minutes, and they do this by using like 3000 graphics cards in parallel.
And then DeepMind also had some progress on parallelism with this model called IMPALA, which basically was in the context of reinforcement learning as opposed to classification, and there they sort of came up with a way that allowed them to do updates in parallels, like learn on different machines and combine everything that was learned in a way that's asynchronous. So in the past the sort of methods that they would use for these reinforcement learning problems, you'd have to wait for all of the different machines to finish their learning on the current problem or instance that they're learning about, and then combine all of that centrally—whereas the new method allows you to just as soon as you're done computing or learning something, you can communicate it to the rest of the system, the other computers that are learning in parallel. And that was really important for allowing them to scale to hundreds of machines working on their problem at the same time.
Ariel: Okay, and so that, just to clarify as well, that goes back to this idea that right now we're seeing a lot of success just scaling up the computing, but at some point that could slow things down essentially, if we had a limit for how much computing is possible.
David: Yeah, and I guess one of my points is also doing this kinds of scaling of computing requires some amount of algorithmic insight or breakthrough if you want to be dramatic as well. So this DeepMind paper I talked about, they had to devise new reinforcement learning algorithms that would still be stable when they had this real-time asynchronous updating. And so, in a way, yeah, a lot of the research that's interesting right now is on finding ways to make the algorithm scale so that you can keep taking advantage of more and more hardware. And the evolution stuff also fits into that picture to some extent.
Ariel: Okay. I want to start making that transition into some of the concerns that we have for misuse around AI and how easy it is for people to be deceived by things that have been created by AI. But I want to start with something that's hopefully a little bit more neutral, and talk about Google Duplex, which is the program that Google came out with, I think last May. I don't know the extent to which it's in use now, but they presented it, and it's an AI assistant that can essentially make calls and set up appointments for you. So their examples were it could make a reservation at a restaurant for you, or it could make a reservation for you to get a haircut somewhere. And it got sort of mixed reviews, because on the one hand people were really excited about this, and on the other hand it was kind of creepy because it sounded human, and the people on the other end of the call did not know that they were talking to a machine.
So I was hoping you guys could talk a little bit I guess maybe about the extent to which that was an actual technological breakthrough versus just something—this one being more one of those breakthroughs that will impact society more directly. And then also I guess if you agree that this seems like a good place to transition into some of the safety issues.
David: Yeah, no, I would be surprised if they really told us about the details of how that worked. So it's hard to know how much of an algorithmic breakthrough or algorithmic breakthroughs were involved. It's very impressive, I think, just in terms of what it was able to do, and of course these demos that we saw were maybe selected for their impressiveness. But I was really, really impressed personally, just to see a system that's able to do that.
Roman: It's probably built on a lot of existing technology, but it is more about impact than what you can do with this. And my background is cybersecurity, so I see it as a great tool for like automating spear-phishing attacks on a scale of millions. You’re getting a real human calling you, talking to you, with access to your online data; Pretty much everyone’s gonna agree and do whatever the system is asking of you, if it's credit card numbers, or social security numbers. So, in many ways it's going to be a game changer.
Ariel: So I'm going to take that as a definite transition into safety issues. So, yeah, let's start talking about, I guess, sort of human manipulation that's happening here. First, the phrase “deep fake” shows up a lot. Can you explain what those are?
David: So “deep fakes” is basically just: you can make a fake video of somebody doing something or saying something that they did not actually do or say. People have used this to create fake videos of politicians, they've used it to create porn using celebrities. That was one of the things that got it on the front page of the internet, basically. And Reddit actually shut down the subreddit where people were doing that. But, I mean, there's all sorts of possibilities.
Ariel: Okay, so I think the Reddit example was technically the very end of 2017. But all of this sort became more of an issue in 2018. So we're seeing this increase in capability to both create images that seem real, create audio that seems real, create video that seems real, and to modify existing images and video and audio in ways that aren't immediately obvious to a human. What did we see in terms of research to try to protect us from that, or catch that, or defend against that?
Roman: So here's an interesting observation, I guess. You can develop some sort of a forensic tool to analyze it, and give you a percentage likelihood that it's real or that it's fake. But does it really impact people? If you see it with your own eyes, are you going to believe your lying eyes, or some expert statistician on CNN?
So the problem is it will still have tremendous impact on most people. We're not very successful at convincing people about multiple scientific facts. They simply go outside, or it's cold right now, so global warming is false. I suspect we'll see exactly that with, let's say, fake videos of politicians, where a majority of people easily believe anything they hear once or see once versus any number of peer reviewed publications disproving it.
David: I kind of agree. I mean, I think, when I try to think about how we would actually solve this kind of problem, I don't think a technical solution that just allows somebody who has technical expertise to distinguish real from fake is going to be enough. We really need to figure out how to build a better trust infrastructure in our whole society which is kind of a massive project. I'm not even sure exactly where to begin with that.
Roman: I guess the good news is it gives you plausible deniability. If a video of me comes out doing horrible things I can play it straight.
Ariel: That's good for someone. Alright, so, I mean, you guys are two researchers, I don't know how into policy you are, but I don't know if we saw as many strong policies being developed. We did see the implementation of the GDPR, and for people who aren't familiar with the GDPR, it's essentially European rules about what data companies can collect from your interactions online, and the ways in which you need to give approval for companies to collect your data, and there's a lot more to it than that. One of the things that I found most interesting about the GDPR is that it's entirely European based, but it had a very global impact because it's so difficult for companies to apply something only in Europe and not in other countries. And so earlier this year when you were getting all of those emails about privacy policies, that was all triggered by the GDPR. That was something very specific that happened and it did make a lot of news, but in general I felt that we saw a lot of countries and a lot of national and international efforts for governments to start trying to understand how AI is going to be impacting their citizens, and then also trying to apply ethics and things like that.
I'm sort of curious, before we get too far into anything: just as researchers, what is your reaction to that?
Roman: So I never got as much spam as I did that week when they released this new policy, so that kind of gives you a pretty good summary of what to expect. If you look at history, we have regulations against spam, for example. Computer viruses are illegal. So that's a very expected result. It's not gonna solve technical problems. Right?
David: I guess I like that they're paying attention and they're trying to tackle these issues. I think the way GDPR was actually worded, it has been criticized a lot for being either much too broad or demanding, or vague. I'm not sure—there are some aspects of the details of that regulation that I'm not convinced about, or not super happy about. I guess overall it seems like people who are making these kinds of decisions, especially when we're talking about cutting edge machine learning, it's just really hard. I mean, even people in the fields don't really know how you would begin to effectively regulate machine learning systems, and I think there's a lot of disagreement about what a reasonable level of regulation would be or how regulations should work.
People are starting to have that sort of conversation in the research community a little bit more, and maybe we'll have some better ideas about that in a few years. But I think right now it seems premature to me to even start trying to regulate machine learning in particular, because we just don't really know where to begin. I think it's obvious that we do need to think about how we control the use of the technology, because it's just so powerful and has so much potential for harm and misuse and accidents and so on. But I think how you actually go about doing that is a really unclear and difficult problem.
Ariel: So for me it's sort of interesting, we've been debating a bit today about technological breakthroughs versus societal impacts, and whether 2018 actually had as many breakthroughs and all of that. But I would guess that all of us agree that AI is progressing a lot faster than government does.
David: Yeah.
Roman: That's almost a tautology.
Ariel: So I guess as researchers, what concerns do you have regarding that? Like do you worry about the speed at which AI is advancing?
David: Yeah, I would say I definitely do. I mean, we were just talking about this issue with fakes and how that's going to contribute to things like fake news and erosion of trust in media and authority and polarization of society. I mean, if AI wasn't going so fast in that direction, then we wouldn't have that problem. And I think the rate that it's going, I don't see us catching up—or I should say, I don't see the government catching up on its own anytime soon—to actually control the use of AI technology, and do our best anyways to make sure that it's used in a safe way, and a fair way, and so on.
I think in and of itself it's maybe not bad that the technology is progressing fast. I mean, it's really amazing; Scientifically there's gonna be all sorts of amazing applications for it. But there's going to be more and more problems as well, and I don't think we're really well equipped to solve them right now.
Roman: I'll agree with David, I'm very concerned at its relative rate of progress. AI development progresses a lot faster than anything we see in AI safety. AI safety is just trying to identify problem areas, propose some general directions, but we have very little to show in terms of solved problems.
If you look at our work in adversarial fields, maybe a little bit cryptography, the good guys have always been a step ahead of the bad guys, whereas here you barely have any good guys as a percentage. You have like less than 1% of researchers working directly on safety full-time. Same situation with funding. So it's not a very optimistic picture at this point.
David: I think it's worth definitely distinguishing the kind of security risks that we're talking about, in terms of fake news and stuff like that, from long-term AI safety, which is what I'm most interested in, and think is actually even more important, even though I think there's going to be tons of important impacts we have to worry about already, and in the coming years.
And the long-term safety stuff is really more about artificial intelligence that becomes broadly capable and as smart or smarter than humans across the board. And there, there's maybe a little bit more signs of hope if I look at how the fields might progress in the future, and that's because there's a lot of problems that are going to be relevant for controlling or aligning or understanding these kind of generally intelligent systems that are probably going to be necessary anyways in terms of making systems that are more capable in the near future.
So I think we're starting to see issues with trying to get AIs to do what we want, and failing to, because we just don't know how to specify what we want. And that's, I think, basically the core of the AI safety problem—is that we don't have a good way of specifying what we want. An example of that is what are called adversarial examples, which sort of demonstrate that computer vision systems that are able to do a really amazing job at classifying images and seeing what's in an image and labeling images still make mistakes that humans just would never make. Images that look indistinguishable to humans can look completely different to the AI system, and that means that we haven't really successfully communicated to the AI system what our visual concepts are. And so even though we think we have done a good job of telling it what to do, it's like, "tell us what this picture is of"—the way that it found to do that really isn't the way that we would do it and actually there's some very problematic and unsettling differences there. And that's another field that, along with the ones that I mentioned, like generative models and GANs, has been receiving a lot more attention in the last couple of years, which is really exciting from the point of view of safety and specification.
Ariel: So, would it be fair to say that you think we've had progress or at least seen progress in addressing long-term safety issues, but some of the near-term safety issues, maybe we need faster work?
David: I mean I think to be clear, we have such a long way to go to address the kind of issues we're going to see with generally intelligent and super intelligent AIs, that I still think that's an even more pressing problem, and that's what I'm personally focused on. I just think that you can see that there are going to be a lot of really big problems in the near term as well. And we're not even well equipped to deal with those problems right now.
Roman: I'll generally agree with David. I'm more concerned about long-term impacts. There are both more challenging and more impactful. It seems like short-term things may be problematic right now, but the main difficulty is that we didn't start working on them in time. So problems like algorithmic fairness, bias, technological unemployment, are social issues which are quite solvable; They are not really that difficult from engineering or technical points of view. Whereas long-term control of systems which are more intelligent than you are—very much unsolved at this point in any even toy model. So I would agree with the part about bigger concerns but I think current problems we have today, they are already impacting people, but the good news is we know how to do better.
David: I'm not sure that we know how to do better exactly. Like I think a lot of these problems, it's more of a problem of willpower and developing political solutions, so the ones that you mentioned. But with the deep fakes, this is something that I think requires a little bit more of a technical solution in the sense of how we organize our society so that people are either educated enough to understand this stuff, or so that people actually have someone they trust and have a reason to trust, who they can take their word for it on that.
Roman: That sounds like a great job, I'll take it.
Ariel: It almost sounds like something we need to have someone doing in person, though.
So going back to this past year: were there, say, groups that formed, or research teams that came together, or just general efforts that, while maybe they didn't produce something yet, you think could produce something good, either in safety or AI in general?
David: I think something interesting is happening in terms of the way AI safety is perceived and talked about in the broader AI and machine learning community. It's a little bit like this phenomenon where once we solve something people don't consider it AI anymore. So I think machine learning researchers, once they actually recognize the problem that the safety community has been sort of harping on and talking about and saying like, "Oh, this is a big problem"—once they say, "Oh yeah, I'm working on this kind of problem, and that seems relevant to me," then they don't really think that it's AI safety, and they're like, “This is just part of what I'm doing, making something that actually generalizes well and learns the right concept, or making something that is actually robust, or being able to interpret the model that I'm building, and actually know how it works.”
These are all things that people are doing a lot of work on these days in machine learning that I consider really relevant for AI safety. So I think that's like a really encouraging sign, in a way, that the community is sort of starting to recognize a lot of the problems, or at least instances of a lot of the problems that are going to be really critical for aligning generally intelligent AIs.
Ariel: And Roman, what about you? Did you see anything sort of forming in the last year that maybe doesn't have some specific result, but that seemed hopeful to you?
Roman: Absolutely. So I've mentioned that there is very few actual AI safety researchers as compared to the number of AI developers, researchers directly creating more capable machines. But the growth rate is much better I think. The number of organizations, the number of people who show interest in it, the number of papers I think is growing at a much faster rate, and it's encouraging because as David said, it's kind of like this convergence if you will, where more and more people realize, “I cannot say I built an intelligent system if it kills everyone.” That's just not what an intelligent system is.
So safety and security become integral parts of it. I think Stuart Russell has a great example where he talks about bridge engineering. We don't talk about safe bridges and secure bridges—there's just bridges. If it falls down, it's not a bridge. Exactly the same is starting to happen here: People realize, “My system cannot fail and embarrass the company, I have to make sure it will not cause an accident.”
David: I think that a lot of people are thinking about that way more and more, which is great, but there is a sort of research mindset, where people just want to understand intelligence, and solve intelligence. And I think that's kind of a different pursuit. Solving intelligence doesn't mean that you make something that is safe and secure, it just means you make something that's really intelligent, and I would like it if people who had that mindset were still, I guess, interested in or respectful of or recognized that this research is potentially dangerous. I mean, not right now necessarily, but going forward I think we're going to need to have people sort of agree on having that attitude to some extent of being careful.
Ariel: Would you agree though that you're seeing more of that happening?
David: Yeah, absolutely, yeah. But I mean it might just happen naturally on its own, which would be great.
Ariel: Alright, so before I get to my very last question, is there anything else you guys wanted to bring up about 2018 that we didn't get to yet?
David: So we were talking about AI safety and there's kind of a few big developments in the last year. I mean, there's actually too many I think for me to go over all of them, but I wanted to talk about something which I think is relevant to the specification problem that I was talking about earlier.
Ariel: Okay.
David: So, there are three papers in the last year, actually, on what I call superhuman feedback. The idea motivating these works is that even specifying what we want on a particular instance in some particular scenario can be difficult. So typically the way that we would think about training an AI that understands our intentions is to give it a bunch of examples, and say, "In this situation, I prefer if you do this. This is the kind of behavior I want," and then the AI is supposed to pick up on the patterns there and sort of infer what our intentions are more generally.
But there can be some things that we would like AI systems to be competent at doing, ideally, that are really difficult to even assess individual instances of. Two examples that I like to use are designing a transit system for a large city, or maybe for a whole country, or the world or something. That's something that right now is done by a massive team of people. Using that whole team to sort of assess a proposed design that the AI might make would be one example of superhuman feedback, because it's not just a single human. But you might want to be able to do this with just a single human and a team of AIs helping them, instead of a team of humans. And there's a few proposals for how you could do that that have come out of the safety community recently, which I think are pretty interesting.
Ariel: Why is it called superhuman feedback?
David: Actually, this is just my term for it. I don't think anyone else is using this term.
Ariel: Okay.
David: Sorry if that wasn't clear. The reason I use it is because there are three different, like, lines of work here. So there's these two papers from OpenAI on what's called amplification and debate, and then another paper from DeepMind on reward learning and recursive reward learning. And I like to view these as all kind of trying to solve the same problem. How can we assist humans and enable them to make good judgements and informed judgements that actually reflect what their preferences are when they're not capable of doing that by themselves unaided. So it's superhuman in the sense that it's better than a single human can do. And these proposals are also aspiring to do things I think that even teams of humans couldn't do by having AI helpers that sort of help you do the evaluation.
An example that Yan—who's the lead author on the DeepMind paper, which I also worked on—gives is assessing an academic paper. So if you yourself aren't familiar with the field and don't have the expertise to assess this paper, you might not be able to say whether or not it should be published. But if you can decompose that task into things like: is the paper valid? Are the proofs valid? Are the experiments following a reasonable protocol? Is it novel? Is it formatted correctly for the venue where it's submitted? And you got answers to all of those from helpers, then you could make the judgment. You'd just be like okay, it meets all of the criteria, so it should be published. The idea would be to get AI helpers to do those sorts of evaluations for you across a broad range of tasks, and allow us to explain to AIs, or teach AIs what we want across a broad range of tasks in that way.
Ariel: So, okay, and so then were there other things that you wanted to mention as well?
David: I do feel like I should talk about another thing that was, again, not developed last year, but really sort of took off last year—is this new kind of neural network architecture called the transformer, which is basically being used in a lot of places where convolutional neural networks and recurrent neural networks were being used before. And those were kind of the two main driving factors behind the deep learning revolution in terms of vision, where you use convolutional networks and things that have a sequential structure, like speech, or text, where people were using recurrent neural networks. And this architecture is actually motivated originally by the same sort of scaling consideration because it allowed them to remove some of the most computationally heavy parts of running these kind of models in the context of translation, and basically make it a hundred times cheaper to train a translation model. But since then it's also been used in a lot of other contexts and has shown to be a really good replacement for these other kinds of models for a lot of applications.
And I guess the way to describe what it's doing is it's based on what's called an attention mechanism, which is basically a way of giving a neural network the ability to pay more attention to different parts of an input than other parts. So like to look at one word that is most relevant to the current translation task. So if you're imagining outputting words one at a time, then because different languages have words in different order, it doesn't make sense to sort of try and translate the next word. You want to look through the whole input sentence, like a sentence in English, and find the word that corresponds to whatever word should come next in your output sentence.
And that was sort of the original inspiration for this attention mechanism, but since then it's been applied in a bunch of different ways, including paying attention to different parts of the model's own computation, paying attention to different parts of images. And basically just using this attention mechanism in the place of the other sort of neural architectures that people thought were really important to give you temporal dependencies across something sequential like a sentence that you're trying to translate, turned out to work really well.
Ariel: So I want to actually pass this to Roman real quick. Did you have any comments that you wanted to add to either the superhuman feedback or the transformer architecture?
Roman: Sure, so superhuman feedback: I like the idea and I think people should be exploring that, but we can kind of look at similar examples previously. So, for a while we had situation where teams of human chess players and machines did better than just unaided machines or unaided humans. That lasted about ten years. And then machines became so much better, humans didn't really contribute anything, it was kind of just like an additional bottleneck to consult with them. I wonder if long term this solution will face similar problems. It's very useful right now, but it seems like, I don't know if it will scale.
David: Well I want to respond to that, because I think it's—the idea here is, in my mind, to have something that actually scales in the way that you're describing, where it can sort of out-compete pure AI systems. Although I guess some people might be hoping that that's the case, because that would make the strategic picture better in terms of people's willingness to use safer systems. But this is more about just how can we even train systems—if we have the willpower, if people want to build a system that has the human in charge, and ends up doing what the human wants—how can we actually do that for something that's really complicated?
Roman: Right. And as I said, I think it's a great way to get there. So this part I'm not concerned about. It's a long-term game with that.
David: Yeah, no, I mean I agree that that is something to be worried about as well.
Roman: There is a possibility of manipulation if you have a human in the loop, and that itself makes it not safer but more dangerous in certain ways.
David: Yeah, one of the biggest concerns I have for this whole line of work is that the human needs to really trust the AI systems that are assisting it, and I just don't see that we have good enough mechanisms for establishing trust and building trustworthy systems right now, to really make this scale well without introducing a lot of risk for things like manipulation, or even just compounding of errors.
Roman: But those approaches, like the debate approach, it just feels like they're setting up humans for manipulation from both sides, and who's better at breaking the human psychological model.
David: Yep, I think it's interesting, and I think it's a good line of work. But I think we haven't seen anything that looks like a convincing solution to me yet.
Roman: Agreed.
Ariel: So, Roman, was there anything else that you wanted to add about things that happened in the last year that we didn't get to?
Roman: Well, as a professor, I can tell you that students stop learning after about 40 minutes. So I think at this point we’re just being counterproductive.
Ariel: So for what it's worth, our most popular podcasts have all exceeded two hours. So, what are you looking forward to in 2019?
Roman: Are you asking about safety or development?
Ariel: Whatever you want to answer. Just sort of in general, as you look toward 2019, what relative to AI are you most excited and hopeful to see, or what do you predict we'll see?
David: So I'm super excited for people to hopefully pick up on this reward learning agenda that I mentioned that Jan and me and people at DeepMind worked on. I was actually pretty surprised how little work has been done on this. So the idea of this agenda at a high level is just: we want to learn a reward function—which is like a score, that tells an agent how well it's doing—learn reward functions that encode what we want the AI to do, and that's the way that we're going to specify tasks to an AI. And I think from a machine learning researcher point of view this is kind of the most obvious solution to specification problems and to safety—is just learner reward function. But very few people are really trying to do that, and I'm hoping that we'll see more people trying to do that, and encountering and addressing some of the challenges that come up.
Roman: So I think by definition we cannot predict short-term breakthroughs. So what we'll see is a lot of continuation of 2018 work, and previous work scaling up. So, if you have, let’s say, Texas hold 'em poker: so for two players, we'll take it to six players, ten players, something like that. And you can make similar projections for other fields, so the strategy games will be taken to new maps, involve more players, maybe additional handicaps will be introduced for the bots. But that's all we can really predict, kind of gradual improvement.
Protein folding will be even more efficient in terms of predicting actual structures: Any type of accuracy rates, if they were climbing from 80% to 90%, will hit 95, 96. And this is a very useful way of predicting what we can anticipate, and I'm trying to do something similar with accidents. So if we can see historically what was going wrong with systems, we can project those trends forward. And I'm happy to say that there is now at least two or three different teams working and collecting those examples and trying to analyze them and create taxonomies for them. So that's very encouraging.
David: Another thing that comes to mind is—I mentioned adversarial examples earlier, which are these imperceptible differences to a human that change how the AI system perceives something like an image. And so far, for the most part, the field has been focused on really imperceptible changes. But I think now people are starting to move towards a broader idea of what counts as an adversarial example. So basically anything that a human thinks clearly should belong to this class and the AI system thinks clearly should belong to this other class that has sort have been constructed deliberately to create that kind of a difference.
And I think this going to be really interesting and exciting to see how the field tries to move in that direction, because as I mentioned, I think it's hard to define how humans decide whether or not something is a picture of a cat or something. And the way that we've done it so far is just by giving lots of examples of things that we say are cats. But it turns out that that isn't sufficient, and so I think this is really going to push a lot of people closer towards thinking about some of the really core safety challenges within the mainstream machine learning community. So I think that's super exciting.
Roman: It is a very interesting topic, and I am in particular looking at a side subject in that, which is adversarial inputs for humans, and machines developing which I guess is kind of like optical illusions, and audio illusions, where a human is mislabeling inputs in a predictable way, which is allowing for manipulation.
Ariel: Along very similar lines, I think I want to modify my questions slightly, and also ask: coming up in 2019, what are you both working on that you're excited about, if you can tell us?
Roman: Sure, so there has been a number of publications looking at particular limitations, either through mathematical proofs or through well known economic models, and what is possible in fact, from computational, complexity points of view. And I'm trying to kind of integrate those into a single model showing—in principle, not in practice, but even in principle—what can we do with the AI control problem? How solvable is it? Is it solvable? Is it not solvable? Because I don't think there is a mathematically rigorous proof, or even a rigorous argument either way. So I think that will be helpful, especially with kind of arguing about importance of a problem and resource allocation.
David: I'm trying to think what I can talk about. I guess right now I have some ideas for projects that are not super well thought out, so I won't talk about those. And I have a project that I'm trying to finish off which is a little bit hard to describe in detail, but I'll give the really high level motivation for it. And it's about something that people in the safety community like to call capability control. I think Nick Bostrom has these terms, capability control and motivation control. And so what I've been talking about most of the time in terms of safety during this podcast was more like motivation control, like getting the AI to want to do the right thing, and to understand what we want. But that might end up being too hard, or sort of limited in some respect. And the alternative is just to make AIs that aren't capable of doing things that are dangerous or catastrophic.
A lot of people in the safety community sort of worry about capability control approaches failing because if you have a very intelligent agent, it will view these attempts to control it as undesirable, and try and free itself from any constraints that we give it. And I think a way of sort of trying to get around that problem is to sort of look at capability control from the lens of motivation control. So to basically make an AI that doesn't want to influence certain things, and maybe doesn't have some of these drives to influence the world, or to influence the future. And so in particular I'm trying to see how can we design agents that really don't try to influence the future, and really only care about doing the right thing, right now. And if we try and do that in a sort of naïve way, or there ways that can fail, and we can get some sort of emergent drive to still try and optimize over the long term, or try and have some influence in the future. And I think to the extent we see things like that, that's problematic from this perspective of let's just make AIs that aren't capable or motivated to influence the future.
Ariel: Alright! I think I've kept you both on for quite a while now. So, David and Roman, thank you so much for joining us today.
David: Yeah, thank you both as well.
Roman: Thank you so much.
Related episodes
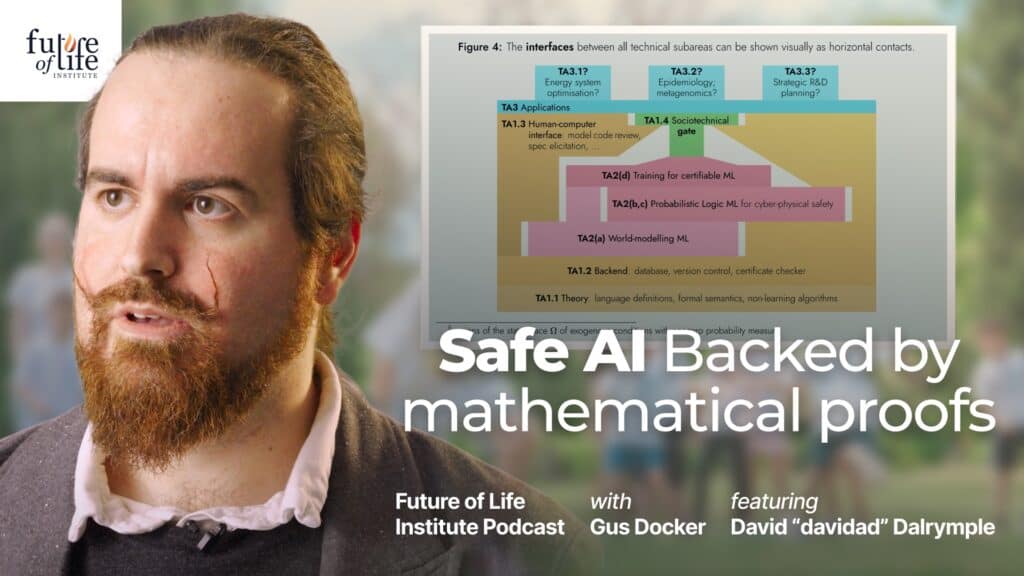
David Dalrymple on Safeguarded, Transformative AI
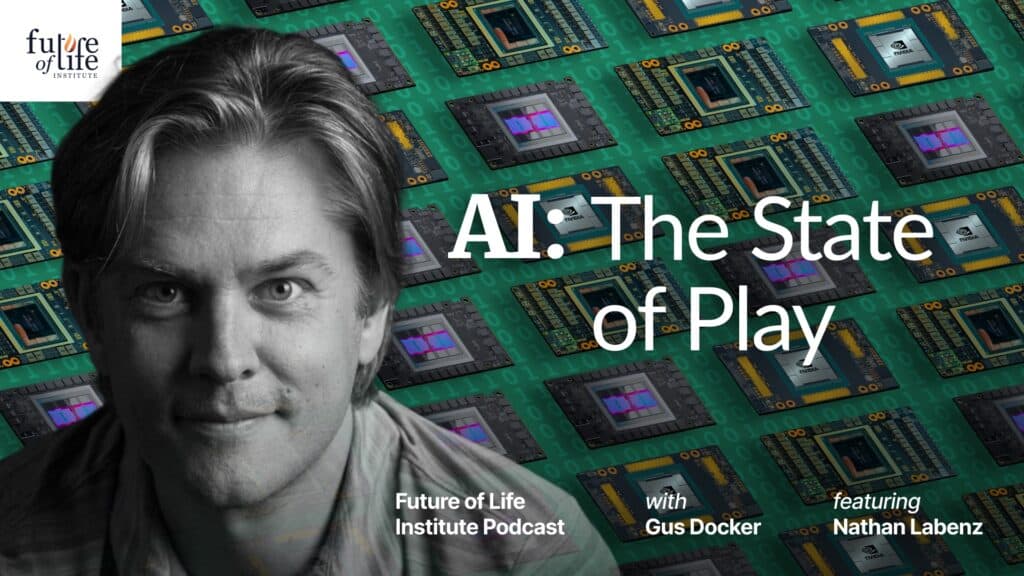