AI Alignment Podcast: AI Safety, Possible Minds, and Simulated Worlds with Roman Yampolskiy
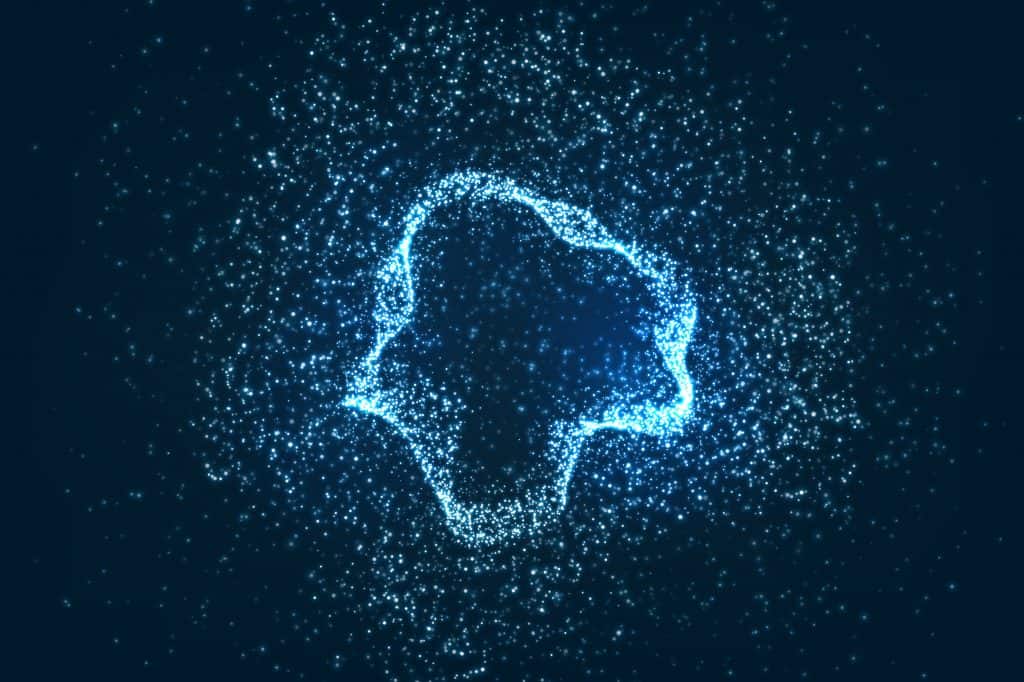
What role does cyber security play in AI alignment and safety? What is AI completeness? What is the space of mind design and what does it tell us about AI safety? How does the possibility of machine qualia fit into this space? Can we leak proof the singularity to ensure we are able to test AGI? And what is computational complexity theory anyway?
AI Safety, Possible Minds, and Simulated Worlds is the third podcast in the new AI Alignment series, hosted by Lucas Perry. For those of you that are new, this series will be covering and exploring the AI alignment problem across a large variety of domains, reflecting the fundamentally interdisciplinary nature of AI alignment. Broadly, we will be having discussions with technical and non-technical researchers across areas such as machine learning, AI safety, governance, coordination, ethics, philosophy, and psychology as they pertain to the project of creating beneficial AI. If this sounds interesting to you, we hope that you will join in the conversations by following us or subscribing to our podcasts on Youtube, SoundCloud, or your preferred podcast site/application.
If you're interested in exploring the interdisciplinary nature of AI alignment, we suggest you take a look here at a preliminary landscape which begins to map this space.
In this podcast, Lucas spoke with Roman Yampolskiy, a Tenured Associate Professor in the department of Computer Engineering and Computer Science at the Speed School of Engineering, University of Louisville. Dr. Yampolskiy’s main areas of interest are AI Safety, Artificial Intelligence, Behavioral Biometrics, Cybersecurity, Digital Forensics, Games, Genetic Algorithms, and Pattern Recognition. He is an author of over 100 publications including multiple journal articles and books.Â
Topics discussed in this episode include:
- Cyber security applications to AI safety
- Key concepts in Roman's papers and books
- Is AI alignment solvable?
- The control problem
- The ethics of and detecting qualia in machine intelligence
- Machine ethics and it's role or lack thereof in AI safety
- Simulated worlds and if detecting base reality is possible
- AI safety publicity strategy
Transcript
Lucas: Hey everyone, welcome back to the AI Alignment Podcast Series with the Future of Life Institute. I'm Lucas Perry and today, we'll be speaking with Dr. Roman Yampolskiy. This is the third installment in this new AI Alignment Series. If you're interested in inverse reinforcement learning or the possibility of astronomical future suffering being brought about by advanced AI systems, make sure to check out the first two podcasts in this series.
As always, if you find this podcast interesting or useful, make sure to subscribe or follow us on your preferred listening platform. Dr. Roman Yampolskiy is a tenured associate professor in the Department of Computer Science and Engineering at the Speed School of Engineering at the University of Louisville. He is the founding and current director of the Cybersecurity Lab and an author of many books including Artificial Superintelligence: A Futuristic Approach.
Dr. Yampolskiy's main areas of interest are in AI safety, artificial intelligence, behavioral biometrics, cybersecurity, digital forensics, games, genetic algorithms and pattern recognition. Today, we cover key concepts in his papers and books surrounding AI safety and artificial intelligence superintelligence and AGI, his approach to AI alignment, how AI security fits into all this. We also explore our audience-submitted questions. This was a very enjoyable conversation and I hope you find it valuable. With that, I give you Dr. Roman Yampolskiy.
Thanks so much for coming on the podcast, Roman. It's really a pleasure to have you here.
Roman: It's my pleasure.
Lucas: I guess let's jump into this. You can give us a little bit more information about your background, what you're focusing on. Take us a little bit through the evolution of Roman Yampolskiy and the computer science and AI field.
Roman: Sure. I got my PhD in Computer Science and Engineering. My dissertation work was on behavioral biometrics. Typically, that's applied to profiling human behavior, but I took it to the next level looking at nonhuman entities, bots, artificially intelligent systems trying to see if we can apply same techniques, same tools to detect bots, to prevent bots, to separate natural human behavior from artificial behaviors.
From there, I try to figure out, "Well, what's the next step? As those artificial intelligence systems more capable, can we keep up? Can we still enforce some security on them?" That naturally led me to looking at much more capable systems and the whole issues with AGI and superintelligence.
Lucas: Okay. In terms of applying biometrics to AI systems or software or computers in general, what does that look like and what is the end goal there? What are the metrics of the computer that you're measuring and to what end are they used and what information can they give you?
Roman: The good example I can give you is from my dissertation work again. I was very interested with poker at the time. The poker rooms online were still legal in US and completely infested with bots. I had a few running myself. I knew about the problem and I was trying to figure out ways to automatically detect that behavior. Figure out which bot is playing and prevent them from participating and draining resources. That's one example where you just have some sort of computational resource and you want to prevent spam bots or anything like that from stealing them.
Lucas: Okay, this is cool. Before you've arrived at this AGI and superintelligence stuff, could you explain a little bit more about what you've been up to? It seems like you've done a lot in computer security. Could you unpack a little bit about that?
Roman: All right. I was doing a lot of very standard work relating to pattern recognition, neural networks, just what most people do in terms of work on AI recognizing digits and handwriting and things of that nature. I did a lot of work in biometrics, so recognizing not just different behaviors but face recognition, fingerprint recognition, any type of forensic analysis.
I do run Cybersecurity Lab here at the University of Louisville. My students typically work on more well recognized sub domains of security. With them, we did a lot of work in all those domains, forensics, cryptography, security.
Lucas: Okay. Do you feel that all the security research, how much of it do you think is important or critical to or feeds into ASI and AGI research? How much of it right now is actually applicable or is making interesting discoveries, which can inform ASI and AGI thinking?
Roman: I think it's fundamental. That's what I get most of my tools and ideas for working with intelligent systems. Basically, everything we learned in security is now applicable. This is just a different type of cyber infrastructure. We learned to defend computers, networks. Now, we are trying to defend intelligent systems both from insider threats and outside from the systems themselves. That's a novel angle, but pretty much everything I did before is now directly applicable. So many people working in AI safety approach it from other disciplines, philosophy, economics, political science. A lot of them don't have the tools to see it as a computer science problem.
Lucas: The security aspect of it certainly make sense. You've written on utility function security. If we're to make value aligned systems, then it's going to be important that the right sorts of people have control over them and that their preferences and dispositions and the systems, again, utility function is secure is very important. A system in the end I guess isn't really safe or robust or value aligned if it's extremely influenced by anyone.
Roman: Right. If someone can just disable your safety mechanism, do you really have a safe system? That completely defeats everything you did. You release a well-aligned, friendly system and then somebody flips a few bits and you got the exact opposite.
Lucas: Right. Given this research focus that you have in security and how it feeds into ASI and AGI thinking and research and AI alignment efforts, how would you just generally summarize your approach to AI alignment and safety?
Roman: There is not a general final conclusion I can give you. It's still work in progress. I'm still trying to understand all the types of problems we are likely to face. I'm still trying to understand this problem as even solvable to begin with. Can we actually control more intelligent systems? I always look at it from engineering computer science point of view much less from philosophy ethics point of view.
Lucas: Whether or not this problem is in principle solvable, that has a lot to do with fundamental principles and ideas and facts about minds in general and what is possible of minds. Can you unpack a little bit more about what sorts of information we need or what we need to think about more going forward to know what it means whether or not this problem is solvable in principle, how we can figure that up as we continue forward?
Roman: There is multiple ways you can show that it's solvable. The ideal situation is where you can produce some sort of a mathematical proof. That's probably the hardest way to do it because it's such a generic problem. It applies to all domains. It has to be still working under self-improvement and modification. It has to still work after learning of additional information and it has to be reliable against malevolent design, so purposeful modifications. It seems like it's probably the hardest problem ever to be given to them. Mathematics community are willing to take it on.
You can also look at examples just from experimental situations both with artificial systems. Are we good at controlling existing AIs? Can we make them safe? Can we make software safe in general? Also, natural systems. Are we any good at creating safe humans? Are we good at controlling people? Now, it seems like after millennia of efforts coming up with legal framework, ethical framework, religions, all sorts of ways of controlling people, we are pretty much failing at creating safe humans.
Lucas: I guess in the end, that might come down to fundamental issues in human hardware and software. Like the reproduction of human beings through sex and the way that genetics functions just creates a ton of variance in each person, which each person has different dispositions and preferences and other things. Then also the way that I guess software is run and shared across culture and people. Creates more fundamental issues that we might not have in software and machines because they work differently.
Are there existence proofs I guess with AI where AI is superintelligent in a narrow domain or at least above human intelligence in a narrow domain and we have control over such narrow systems? Would it be potentially generalizable as you sort of aggregate more and more AI systems, which are superintelligent in narrow domains that as you aggregate that or create an AGI, which sort of has meta learning, we would be able to have control over it given these existence proofs in narrow domains?
Roman: There are certainly such examples in narrow domains. If we're creating, for example, a system to play chess. We can have a single number measuring it’s performance. We can control whatever is getting better or worse. That's quite possible and is very limited linear domain. The problem is as complexity increases, you go from this n-body problem equals one to n-body equals infinity, and that's very hard to solve both computationally and in terms of just understanding what in that hyperspace of possibilities is a desirable outcome.
It's not just gluing together a few narrow AIs like, "Okay, I have a chess playing program. I have a go playing program." If I put them all in the same PC, do I now have general intelligence capable of moving knowledge across domains? Not exactly. Whatever safety you can prove for limited systems, not necessarily will transferred to a more complex system, which integrates the components.
Very frequently, then you add two safe systems, the merged system has back doors, has problems. Same with adding additional safety mechanisms. A lot of times, you will install a patch for software to increase security and the patch itself has additional loopholes.
Lucas: Right. It's not necessarily the case that in the end, AGI is actually just going to be sort of like an aggregation of a lot of AI systems, which are superintelligent in narrow domains. Rather, it potentially will be something more like an agent, which has very strong meta learning. So, learning about learning and learning how to learn and just learning in general. Such that all the sort of process is in things that it learns or deeply integrated at a lower level and they're sort of like a higher level thinking that is able to execute on these things that they learned. Is that so?
Roman: That makes a lot of sense.
Lucas: Okay. Moving forward here, it would be nice if we could go ahead and explore a little bit of the key concepts in your books and papers and maybe get into some discussions there. I don't want to spend a lot of time talking about each of the terms and having you define them as people can read your book, Artificial Superintelligence: A Futuristic Approach. They can also check out your papers and you've talked about these in other places. I think it will be helpful for giving some background and terms that people might not exactly be exposed to.
Roman: Sure.
Lucas: Moving forward, what can you tell us about what AI completeness is?
Roman: It's a somewhat fuzzy term kind of like Turing test. It's not very precisely defined, but I think it's very useful. It seems that there are certain problems in artificial intelligence in general which require you to pretty much have general intelligence to solve them. If you are capable of solving one of them, then by definition, we can reduce other problems to that one and solve all problems in AI. In my papers, I talk about passing Turing test as being the first such problem. If you can pass unrestricted version of a Turing test, you can pretty much do anything.
Lucas: Right. I think people have some confusions here about what intelligence is in the kinds of minds that can solve Turing tests completely and the architecture that they have and whether that architecture means they're exactly intelligent. I guess some people have this kind of intuition or idea that you could have a sort of system that had meta learning and learning and was able to sort of think as a human does in order to execute a Turing test.
Then potentially, other people have an idea and this may be misguided where a sort of sufficiently complicated tree search or Google engine on the computer would be able to pass a Turing test and that seems potentially kind of stupid. Is the latter idea a myth? Or if not, how is it just as intelligent as the former?
Roman: To pass an unrestricted version of a Turing test, against someone who actually understands how AI works is not trivial. You can do it with just lookup tables and decision trees. I can give you an infinite number of completely novel situations where you have to be intelligent to extrapolate to figure out what's going on. I think theoretically, you can think of an infinite lookup table which has every conceivable string for every conceivable previous sequence of questions, but in reality, it just makes no sense.
Lucas: Right. They're going to be sort of like cognitive features and logical processes and things like inferences and extrapolation and logical tools that humans use that almost must necessarily come along for the ride in order to fully pass a Turing test.
Roman: Right. To fully pass it, you have to be exactly the same in your behavior as a human. Not only you have to be as smart, you also have to be as stupid. You have to repeat all the mistakes, all the limitations in terms of humanity, in terms of your ability to compute, in terms of your cognitive biases. A system has to be so smart that it has a perfect model of an average human and can fake that level of performance.
Lucas: It seems like in order to pass a Turing test, the system would either have to be an emulation of a person and therefore almost essentially be a person just on different substrate or would have to be superintelligent in order to run an emulation of a person or a simulation of a person.
Roman: It has to have a perfect understanding of an average human. It goes together with value alignment. You have to understand what a human would prefer or say or do in every situation and that does require you to understand humanity.
Lucas: Would that function successfully at a higher level of general heuristics about what an average person might do or does it require a perfect emulation or simulation of a person in order to fully understand what a person would do in such an instance?
Roman: I don't know if it has to be perfect. I think there are certain things we can bypass and just going to read books about what a person would do in that situation, but you do have to have a model complete enough to produce good results in novel situations. It's not enough to know, OK, most people would prefer ice cream over getting a beating, something like that. You have to figure out what to do in a completely novel set up where you can just look it up on Google.
Lucas: Moving on from AI completeness, what can you tell us about the space of mind designs and the human mental model and how this fits into AGI and ASI and why it's important?
Roman: A lot of this work was started by Yudkowsky and other people. The idea is just to understand how infinite that hyperspace is. You can have completely different sets of goals and desires from systems which are very capable optimizers. They may be more capable than an average human or best human, but what they want could be completely arbitrary. You can't make assumptions along the lines of, "Well, any system smart enough would be very nice and beneficial to us." That's just a mistake. If you randomly pick a mind from that infinite universe, you'll end up with something completely weird. Most likely incompatible with human preferences.
Lucas: Right. This is just sort of, I guess, another way of explaining the orthogonality thesis as described by Nick Bostrom?
Roman: Exactly. Very good connection, but it gives you a visual representation. I have some nice figures where you can get a feel for it. You start with, "Okay, we have human minds, a little bit of animals, you have aliens in the distance," but then you still keep going and going in some infinite set of mathematical possibilities.
Lucas: In this discussion of the space of all possible minds, it's a discussion about intelligence where intelligence is sort of understood as the ability to change and understand the world and also the preferences and values which are carried along in such minds however random and arbitrary they are from the space of all possible mind design.
One thing which is potentially very important in my view is the connection of the space of all possible hedonic tones within mind space, so the space of all possible experience and how that maps onto the space of all possible minds. Not to say that there's duality going on there, but it seems very crucial and essential to this project to also understand the sorts of experiences of joy and suffering that might come along for each mind within the space of all possible minds.
Is there a way of sort of thinking about this more and formalizing it more such as you do or does that require some more really foundational discoveries and improvements in the philosophy of mind or the science of mind and consciousness?
Roman: I look at this problem and I have some papers looking at those. One looks at just generation of all possible minds. Sequentially, you can represent each possible software program as an integer and brute force them. It will take infinite amount of time, but you'll get to every one of them eventually.
Another recent paper looks at how we can actually detect qualia in natural and artificial agents. While it's impossible for me to experience the world as someone else, I think I was able to come up with a way to detect whatever you have experiences or not. The idea is to present you with the illusions, kind of visual illusions and based on the type of body you have, the type of sensors you have, you might have experiences which match with mine. If they are not, then I can say really anything about you. You could be conscious and experiencing qualia or maybe not. I have no idea.
In a set of such tests on multiple illusions, you happen to experience exactly the same side effects from the illusion. This test drew multiple-choice questions and you can get any level of accuracy you want with just additional tests. Then I have no choice but to assume that you have exactly same qualia in their situation. So, at least I know you do have experiences of that type.
If it's taking it to what you suggested pleasure or pain, we can figure out is there suffering going on, is there pleasure happening, but this is very new. We need a lot more people to start doing psychological experiments with that.
The good news is from existing literature, I found a number of experiments where a neutral network designed for something completely unrelated still experienced similar side effect as a natural model. That's because the two models represent the same mathematical structure.
Lucas: Sorry. The idea here is that by observing effects on the system that if those effects are also correlated or seen in human subjects that this is potentially some indication that the qualia that is correlated with those effects in people is also potentially experienced or seen in the machine?
Roman: Kind of. Yeah. So, when I show you a new cool optical illusion. You experienced something outside of just the values of bits in that illusion. Maybe you see light coming out of it. Or maybe you see rotations. Maybe you see something else.
Lucas: I see a triangle that isn't there.
Roman: Exactly. If a machine reports exactly the same experience without previous knowledge obviously, then just Google what a human would see. How else would you explain that knowledge, right?
Lucas: Yeah. I guess I'm not sure here. I probably need to think about it more actually, but this does seem like a very important approach in place to move forward. The person in me who's concerned about thinking about ethics looks back on the history of ethics and thinks about how human beings are good at optimizing the world in ways in which it produces something of value to them but in optimizing for that thing, they produce huge amounts of suffering. We've done this through subjugation of women and through slavery and through factory farming of animals currently and previously.
After each of these periods, of these morally abhorrent behaviors, it seems we have an awakening and we're like, "Oh, yeah, that was really bad. We shouldn't have done that." I guess just moving forward here with machine intelligence, it's not clear that this will be the case or it is possible that it could be the case, but it may. Potentially sort of the next one of these moral catastrophes is if we sort of ignore this research into the possible hedonic states of machines and just brush it away as being dumb philosophical stuff that we potentially could produce an enormous amount of suffering in machine intelligence and just sort of override that and create another ethical catastrophe.
Roman: Right. I think that makes a lot of sense. I think qualia, a side effect of certain complex computations. You can't avoid producing them if you're doing this type of thinking, computing. We have to be careful once we get to that level of not having very painful side effects.
Lucas: Is there any possibility here of trying to isolate the neural architectural correlates of consciousness in human brains and then physically or digitally instantiating that in machines and then creating a sort of digital or physical corpus callosum between the mind of a person and such a digital or physical instantiation of some neural correlate of something in the machine in order to see if an integration of those two systems creates a change in qualia for the person? Such that the person could sort of almost first-person confirm that when it connects up to this thing that its subjective experience changes and therefore maybe we have some more reason to believe that this thing independent of the person, when they disconnect, has some sort of qualia to it.
Roman: That's very interesting type of experiment I think. I think something like this has been done with Siamese twins conjoined with brain tissue. You can start looking at those to begin with.
Lucas: Cool. Moving on from the space of mind designs and human mental models, let's go ahead and then talk about the singularity paradox. This is something that you cover quite a bit in your book. What can you tell us about the singularity paradox and what you think the best solutions are to it?
Roman: It's just a name for this idea that you have a superintelligent system, very capable optimizer, but it has no common sense as we human perceive it. It's just kind of this autistic savant capable of making huge changes in the world but a four-year-old would have more common sense in terms of disambiguation of human language orders. Just kind of understanding the desirable states of the world.
Lucas: This is sort of the fundamental problem of AI alignment. The sort of assumption about the kind of mind AGI or ASI will be, the sort of autistic savant sort of intelligence, what that is ... This is what Dylan Hadfield-Menell brought up on our first podcast for the AI Alignment Series is that for this case of this autistic savant that most people have in mind, a perfectly rational Bayesian optimizing agent. Is that sort of the case? Is that the sort of mind that we have in mind when we're thinking of this autistic savant that just blows over things we care about because it's just optimizing too hard for one thing and Goodhardt’s law starts to come into effect?
Roman: Yes, in a way. I always try to find most simple examples so we can understand better in the real world. Then you have people with extremely high level of intelligence. The concerns they have, the issues they find interesting are very different from your average person. If you watch something like Big Bang Show with Sheldon, that's like a good to funny example of this on a very small scale. There is maybe 30 IQ point difference, but what if it's 300 points?
Lucas: Right. Given the sort of problem, what are your conclusions and best ideas or best practices for working on this? Working on this is just sort of working on the AI alignment problem I suppose.
Roman: AI alignment is just a new set of words to say we want the safe and secure system, which kind of does what we designed it to do. It doesn't do anything dangerous. It doesn't do something we disagree with. It's well aligned with our intention. By itself, the term adds nothing new. The hard problem is, "Well, how do we do it?"
I think it's fair to say that today, as of right now, no one in the world has a working safety mechanism capable of controlling intelligent behavior and scaling to a new level of intelligence. I think even worse is that no one has a prototype for such a system.
Lucas: One thing that we can do here is we can sort of work on AI safety and we can think about law, policy and governance to try and avoid an arms race in AGI or ASI. Then there are also important ethical questions which need to be worked on before AGI some of which including kind of more short-term things, universal basic income and bias and discrimination in algorithmic systems. How AI will impact the workforce and other things and potentially some bigger ethical questions we might have to solve after AGI if we can pull the brakes.
In terms of the technical stuff, one important path here is thinking about and solving the confinement problem, the method by which we are able to create an AGI or ASI and air gap it and make it so that it is confined and contained to be tested in some sort of environment to see if it's safe. What are your views on that and what do you view as a potential solution to the confinement problem?
Roman: That's obviously a very useful tool to have, to test, to debug, to experiment with an AI system while it's limited in its communication ability. It cannot perform social engineering attacks against the designer or anyone else. It's not the final solution if you will if a system can still escape from such confinement, but it's definitely useful to be able to do experiments on evolving learning AI.
Can I limit access to the Internet? Can I limit access to knowledge, encyclopedia articles? Can I limit output in terms of just text, no audio, no video? Can I do just a binary yes or no? All of it is extremely useful. We have special air gap systems for studying computer viruses, so to understand how they work, how they communicate versus just taking it to the next level of malevolent software.
Lucas: Right. There's sort of this, I guess, general view and I think that Eliezer has participated in some of these black boxing experiments where you pretend as if you are the ASI and you're trying to get out of the box and you practice with other people to see if you can get out of the box. Out of discussions and thinking on this, it seems that some people thought that it's almost impossible to confine these systems. Do you think that, that's misguided or what are your views on that?
Roman: I agree that long-term, you absolutely cannot confine a more intelligent system. I think short-term while it's still developing and learning, it's a useful tool to have. The experiments Eliezer did, very novel at the time, but I wish he meet public all the information to make them truly scientific experiments where people can reproduce them properly, learn from them. Simply saying that this guy who now works with me let me out, it's not the optimal way to do it.
Lucas: Right. I guess the concern there is with confinement experiments is that explaining the way in which it gets out is potentially an information hazard.
Roman: Yeah. People tend to call a lot of things informational hazards. Those things certainly exist. If you have source code for AGI, I strongly recommend you don't make it public, but we've been calling a lot of things informational hazard I think.
The best example is Roko's basilisk where essentially it was a new way to introduce Christianity. If I tell you about Jesus and you don't follow him, now you're going to hell. If I didn't tell you about Jesus, you'd be much better off. Why did you tell me? Deleting it just makes it grow bigger and it's like Streisand effect, right? You promoting this while you trying to suppress it. I think you have to be very careful in calling something an informational hazard, because you're diluting the label by doing that.
Lucas: Here's something I think we can potentially get into the weeds on and we may disagree about and have some different views on. Would you like to just go ahead and unpack your belief? First of all, go ahead and explain what it is and then explain your belief about why machine ethics in the end is the wrong approach or a wrong instrument in AI alignment.
Roman: The way it was always done in philosophy typically, everyone tried to publish a paper suggesting, "Okay, this is a set of ethics we need to follow." Maybe it's ethics based on Christianity or Judaism. Maybe it's utilitarianism, whatever it is. There was never any actual solution, anything was proposed which could be implemented as a way to get everyone on board and agree with it. It was really just a competition for like, "Okay, I can come up with a new ethical set of constraints or rules or suggestions."
We know philosophers have been trying to resolve it for millennia. They failed miserably. Why somehow moving it from humans to machines will make it easier problem to solve where a single machine is a lot more powerful and can do a lot more with this is not obvious to me. I think we're unlikely to succeed by doing that. The theories are contradictory, ill-defined, they compete. It doesn't seem like it's going to get us anywhere.
Lucas: To continue unpacking your view a bit more, instead of machine ethics where we can understand machine ethics as the instantiation of normative and meta-ethical principles and reasoning and machine systems to sort of make them moral agents and moral reasoners, your view is that instead of using that, we should use safety engineering. Would you like to just unpack what that is?
Roman: To return to the definition you proposed. For every ethical system, there are edge cases which backfire tremendously. You can have an AI which is a meta-ethical decider and it figures out, "Okay, the best way to avoid human suffering is do not have any humans around." You can defend it from philosophical point of view, right? It makes sense, but is that a solution we would accept if a much smarter system came up with it?
Lucas: No, but that's just value misalignment I think. I don't think that there are any sort of like ... There are, in principle, possible moral systems where you say suffering is so bad that we shouldn't risk any of it at all ever, therefore life shouldn't exist.
Roman: Right, but then you make AI the moral agent. That means it's making moral decisions. It's not just copying what humans decided even if we can somehow figure out what the average is, it's making its own novel decisions using its superintelligence. It's very likely it will come up with something none of us ever considered. The question is, will we like it?
Lucas: Right. I guess just for me here, I understand why AI safety engineering and technical alignment efforts are so very important and intrinsic. I think that it really constitutes a lot of the AI alignment problem. I think that given that the universe has billions and billions and billions of years left to live, that the instantiation of machine ethics in AGI and ASI is... you can't hold off on it and it must be done.
You can't just have an autistic savant superspecies on the planet that you just never imbue with any sort of ethical epistemology or meta-ethics because you're afraid of what might happen. You might want to do that extremely slowly and extremely carefully, but it seems like machine ethics is ultimately an inevitability. If you start to get edge cases that the human beings really don't like, then potentially you just went wrong somewhere in cultivating and creating its moral epistemology.
Roman: I agree with doing it very slowly and carefully. That seems like a good idea in general, but again, just projecting to long-term possibilities. I'm not optimistic that the result will be beneficial.
Lucas: Okay. What is there left to it? If we think of the three cornerstones of AI alignment as being law, policy, governance, then we have ethics on one corner and then we have technical AI alignment on the other corner. We have these three corners.
If we have say AGI or ASI around 2050, which I believe is something a lot of researchers give a 50% probability to, then imagine we simply solve technical AI alignment and we solved the law, policy and governance coordination stuff so that we don't end up having an arms race and we mess up on technical alignment. Or someone uses some singleton ASI to malevolently control everyone else.
Then we still have the ethical issues in the end. Even if we have a perfectly corrigible and docile intelligence, which is sort of tuned to the right people and sort of just takes the right orders. Then whatever that ASI does, it's still going to be a manifestation, an embodiment of the ethics of the people who tell it what to do.
There's still going to be billions and billions of years left in the universe. William MacAskill discusses this. Is that sort of after we've solved the technical alignment issues and the legal and political and coordination issues, then we're going to need a period of long deliberation where we actually have to make concrete decisions about moral epistemology and meta-ethics and try and do it in really a formalized and rigorous way and potentially take thousands of years to figure it out.
Roman: I'm criticizing this and that makes it sound like I have a solution, which is something else and I don't. I don't have a solution whatsoever. I just feel it's important to point out problems with each specific approach so we can avoid problems of over committing to it.
You mentioned a few things. You mentioned getting information from the right people. That seems like that's going to create some problems right there. Not sure who the right people are. You mentioned spending thousands of years deciding what we want to do with this superintelligent system. I don't know if we have that much time given all the other existential risks, given the chance of malevolent superintelligence being released by rogue agents much sooner. Again, it may be the best we got, but it seems like there are some issues we have to look at.
Lucas: Yeah, for sure. Ethics has traditionally been very messy and difficult. I think a lot of people are confused about the subject. Based on my conversation with Dylan Hadfield-Menell, when we're discussing inverse reinforcement learning and other things that he was working on, his sort of view was a view of AI alignment and value alignment where inverse reinforcement learning and other preference learning techniques are sort of used to create a natural evolution of human values and preferences in ethics, which sort of exists in an ecosystem of AI systems which are all, I guess, in conversation so that it could, more so, naturally evolve.
Roman: Natural evolution is a brutal process. It really has no humanity to it. It exterminates most species. I don't know if that's the approach we want to simulate.
Lucas: Not an evolution of ideas?
Roman: Again, if those ideas are actually implemented and applied to all of humanity that has a very different impact than if it's just philosophers debating with no impact.
Lucas: In the end, it seems like a very difficult end frontier to sort of think about and move forward on. Figuring out what we want and what we should do with a plurality of values and preferences. Whether or not we should take a view of moral realism or moral relativism or anti-realism about ethics and morality. Those seem like extremely consequential views or positions to take when determining the fate of the cosmic endowment.
Roman: I agree completely on how difficult the problem is.
Lucas: Moving on from machine ethics, you wrote a  paper on leak proofing the singularity. Would you like to go ahead and unpack a little bit about what you're doing in the paper and how that ties into all of this?
Roman: That's just AI boxing. That was the response to David Chalmers' paper and he talks about AI boxing as leak proofing, so that's the title we used, but it's just a formalization of the whole process. Formalization of the communication channel, what goes in, what goes out. It's a pretty good paper on it. Again, it relies in this approach of using tools from cyber security to formalize the whole process.
For a long time, experts in cyber security attempted to constrain regular software, not intelligent software from communicating with our programs and outside world and operating system. We're looking at how that was done, what different classifications they used for site channels and so on.
Lucas: One thing that you also touch on, would you like to go ahead and unpack like wireheading addiction and mental illness in general in machine systems and AI?
Roman: It seems like there is a lot of mental disorders, people experience. The only example of general intelligence we have. More and more, we see similar problems show up in artificial systems, which try to emulate this type of intelligence. It's not surprising and I think it's good that we have this body of knowledge from psychology which we can now use to predict likely problems and maybe come up with some solutions for them.
Wireheading is essentially this idea of agent not doing any useful work but just stealing their work channel. If you think about having kids and there is a cookie jar and they get rewarded every time they clean the room or something like that with a cookie, well, they essentially can just find the cookie jar and get direct access to their work channel, right? They're kids, so they're unlikely to cause much harm, but if a system is more capable, it realizes you as a human control the cookie jar, well now, it has incentive to control you.
Lucas: Right. There are also these examples with rats and mice that you might be able to discuss a little bit more.
Roman: The classic experiments on that just created through surgery, electrode implants in a brain of some simple animals. Every time you provided an electrical shock to that area, the animals experience the maximum pleasure like orgasm you don't get tired of. They bypass getting food, having sex, playing with toys. They just sat there pressing the button. If you made it where they have to walk on electrocuted fence to get to the button, it wasn't a problem, they would do that. It completely messes with usefulness of an agent.
Lucas: Right. I guess just in terms of touching on the differences and the implications of ethics here is that one with sort of consequentialist views, which was sort of very impartial and on speciesists can potentially view wireheading as ethical or the end goal. Whereas other people view a wireheading as basically abhorrent and akin to something terrible that you would never want to happen. There's also again, I think, a very interesting ethical tension there.
Roman: It goes, I think, to the whole idea of simulated reality and virtual world. Do you care if you're only succeeding in a made-up world? Would that make you happy enough or do you have to actually impact reality? That could be part of resolving our differences about values and ethics. If every single person can be in their own simulated universe where everything goes according to their wishes, is that a solution to getting us all to agree? You know it's a fake universe, but at least you're the king in it.
Lucas: I guess that also touches on this question of the duality that human beings have created between what is fake and real. In what sense is something really fake if it's not just the base reality? Is there really fundamental value in the thing being the base reality and do we even live in the base reality? How does cosmology or ideas that Max Tegmark explores about the multiverse sort of even impact that? How will that impact our meta-ethics and decision-making about the moral worth of wireheading and simulated worlds?
Roman: Absolutely. I have a paper on something I call designer metry, which is measuring natural versus artificial. The big question of course is can we tell if you are living in a simulated reality? Can it be measured scientifically? Or was it just a philosophical idea? It seems like there are certain ways to identify signals from the engineer if it's done on purpose, but in general case, you can never tell whatever something is a deep fake or a real input.
Lucas: I'd like to discuss that a little bit more with you, but just to backup really quick to finish talking on about psychology and AI. It seems like this has been something that is really growing in the AI community and it's not something that I really know much about at all. My general understanding is as AI systems become more and more complex, it's going to be much more difficult to diagnose and understand the specific pathways and architectures, which are leading to mental illness.
Therefore, general diagnosable tools which observe and understand higher level phenomena or behaviors that systems exist that we've developed in psychology would be helpful or implementable here. Is that sort of the case and the use case of psychology here is really just diagnose mental illnesses or does it also has a role in developing positive psychology and well-being in machine systems?
Roman: I think it's more of a first case. If you have a black box AI, just a huge, very deep neural network, you can just look at the wiring and weights and figure out why it's producing the results you're seeing. Whereas you can do high-level experiments, maybe even conversation with the system to give you an idea of how it's misfiring what the problem is.
Lucas: Eventually, if we begin exploring the computational structure of different hedonic tones and that becomes more formalized as a science, then I don't know, maybe potentially, there would be more of a role for psychologists in discussing the well-being part rather than the computational mental illness part.
Roman: It is a very new concept. It's been mentioned a lot in science fiction, but as a scientific concept, it's very new. I think there is only one or two papers on it directly. I think there is so much potential to exploring more on connections with neuroscience. I'm actually quite excited about it.
Lucas: That's exciting. Are we living in a simulated world? What does it mean to be able to gather evidence about whether or not we're living in a simulation? What would such evidence look like? Why may we or may not ever be able to tell whether or not we are in a simulation?
Roman: In general case, if there is not an intent to let you know that it's a simulated world, you would never be able to tell. Absolutely anything can actually be part of natural base system. You don't know what it's like if you are Mario playing in an 8-bit world. You have no idea that it's low resolution. You're just part of that universe. You assume the base is the same.
There are situations where engineers leave trademarks, watermarks, helpful messages in a system to let you know what's going on, but that's just giving you the answer. I think in general case, you can never know, but from statistical arguments, there's ... Nick Bostrom presents a very compelling statistical arguments. I do the same for biological systems in one of my papers.
Roman: It seems more likely that we are not the base just because every single intelligent civilization will produce so many derived civilizations from it. From space exploration, from creating biological robots capable of undergoing evolutionary process. It would be almost a miracle if out of thousands and thousands of potential newly designed organisms, newly evolved ones, we were like the first one.
Lucas: I think that, that sort of evolutionary process presumes that the utility function of the optimization process, which is spreading into the universe, is undergoing an evolutionary process where it's changing. Whereas the security and brittleness and stability of that optimization process might be very fixed. It might be that all future and possible super advanced civilizations do not converge on creating ancestor simulations.
Roman: It's possible, but it feels like a bit less likely. I think they'll still try to grab the resources and the systems may be fixed in certain values, but they still would be adopting to the local environment. We just see it with different human populations, right? We're essentially identical, but we developed very different cultures, religions, food preferences based on the local available resources.
Lucas: I don't know. I feel like I could imagine like a civilization, a very advanced one coming down on some sort of hedonic consequentialism where the view is that you just want to create as many beautiful experiences as possible. Therefore, there wouldn't be any room for simulating evolution on Earth and all the suffering and kind of horrible things we have to go through.
Roman: But you're looking at it from inside the simulation. You don't know what the reasons are on the outside, so this is like a video game or going to the gym. Why would anyone be killed in a video game or suffer tremendously, lifting heavy weights in a gym, right? It's only fun when you understand external reasons for it.
Lucas: I guess just two things here. I just have general questions on. If there is a multiverse at one or another level, would it then also be the case that the infinity of simulated universes would be a larger fraction of the infinity of the multiverse than the worlds which were not simulated universes?
Roman: This is probably above my pay grade. I think Max is someone who can give you a better answer in that. Comparing degrees of infinities is hard.
Lucas: Okay. Cool. It is not something I really understand either. Then I guess the other thing is I guess just in general, it seems queer to me that human beings are in a world and that we look at our computer systems and then we extrapolate what if these computer systems were implemented at a more base level. It seems like we're trapped in a context where all that we have to extrapolate about the causes and conditions of our universe are the most fundamental things that we can observe from within our own universe.
It seems like settling on the idea of, "Okay, we're probably in a simulation," just seems kind of like we're gluing to and finding a cosmogenesis hope in one of the only few things that we can, just given that we live in a universe where there are computers. Does that make sense?
Roman: It does. Again, from inside the simulation, you are very limited in understanding the big picture. Then so much would be easier to understand if we had external knowledge, but it's just not the option we have so far. We learn by pretending to be the engineer in question and now we design virtual worlds. We design intelligent beings and the options we have is the best clue we have about the options available to whoever does it in the external level.
Lucas: Almost as if Mario got to the end of the level and got to the castle. Then because you got to the castle the next level or world started, he was like maybe outside of this context there's just a really, really big castle or something that's making lower levels of castles exist.
Roman: Right. I agree with that, but I think we have in common this mathematical language. I think that's still universal. Just by studying mathematics and possible structures and proving things, we can learn about what's possible and impossible.
Lucas: Right. I mean there's just really foundational and fundamental question about the metaphysical realism or anti-realism of mathematics. If there is a multiverse or like a meta multiverse or like a meta-meta-meta-multiverse levels ...
Roman: Only three levels.
Lucas: I guess just the implications of a mathematical realism or Platonism or sort of anti-realism at these levels would have really big implications.
Roman: Absolutely, but at this point, I think it's just fun to think about those possibilities and what they imply for what we're doing, what we're hoping to do, what we can do. I don't think it's a waste of time to consider those things.
Lucas: Just generally, this is just something I haven't really been updated on. Is this rule about only in three levels of regression, is that just sort of a general principle or role kind of like Occam's razor that people like to stick by? Or is there any more...?
Roman: No. I think it's something Yudkowsky said and it's cute and kind of meme like.
Lucas: Okay. So it's not like serious epistemology?
Roman: I don't know how well proven that is. I think he spoke about levels of recursion initially. I think it's more of a meme.
Lucas: Okay. All right.
Roman: I might be wrong in that. I know a lot about memes, less about science.
Lucas: Me too. Cool. Given all this and everything we've discussed here about AI alignment and superintelligence, what are your biggest open questions right now? What are you most uncertain about? What are you most looking for key answers on?
Roman: The fundamental question of AI safety, is it solvable? Is control problem solvable? I have not seen a paper where someone gives mathematical proof or even a rigorous argument. I see in some blog posts arguing, "Okay, we can predict what the chess machine will do, so surely we can control superintelligence," but it just doesn't seem like it's enough. I'm working on a paper where I will do my best to figure out some answers for that.
Lucas: what is the definition of control and AI alignment?
Roman: I guess it's very important to formalize those before you can answer the question. If we don't even know what we're trying to do, how can we possibly succeed? The first step in any computer science research project is to show that your problem is actually solvable. Some are not. We know, for example, holding problem is not solvable, so it doesn't make sense to give it as an assignment to someone and wait for them to solve it. If you give them more funding, more resources, it's just a waste.
Here, it seems like we have more and more people working very hard in different solutions, different methods, but can we first spend a little bit of time seeing how successful can we be? Based on the answer to that question, I think a lot of our governance and the legal framework and general decision-making about this domain will be impacted by it.
Lucas: If your core and key question here is whether or not the control problem or AI alignment is, in principle, or fundamentally solvable, could you give us a quick crash course on complexity theory and computational complexity theory and just things which take polynomial time to solve versus exponential time?
Roman: That's probably the hardest course you'll take as an undergraduate in computer science. At the time, I hated every second of it. Now, it's my favorite subject. I love it. This is the only professor whom I remember teaching computational complexity and computability.
To simplify it, there are different types of problems. Surprisingly, almost all problems can be squeezed into one of those boxes. There are easy problems, which we can just quickly compute. Your calculator adding 2+2 is an example of that. There are problems where we know exactly how to solve them. It's very simple algorithm. We can call it brute force. You try every option and you'll always get the best answer, but there's so many possibilities that in reality you can never consider every option.
Lucas: Like computing prime numbers.
Roman: Well, computer numbers are NP. It's polynomial to test if a number is prime. It's actually one of somewhat recent paper for the last 10 years, a great result, Ps are N prime. There are problems which are called NP complete and those are usually the interesting problems we care about and they all reduce to each other. If you solve one, you solved all of them. You cannot brute force them. You have to find some clever heuristics to get approximate answers, optimize those.
We can get pretty close to that. Examples like traveling salesperson problem. If you can figure out optimal way to deliver pizza to multiple households, if you can solve it in general case, you'll solve 99% of interesting problems. Then there are some problems which we know no one can ever solve using Von Neumann architecture, like standard computer architecture. There are proposals for hyper computation computers with oracles, computers with all sorts of magical properties which would allow us to solve those very, very, very difficult problems, but that doesn't seem likely anytime soon.
The best part of it I think is this idea of oracles. An oracle is a machine capable of doing magic to give you answer to otherwise unsolvable problem, and there are degrees of oracles. There are magical machines, which are more powerful magicians than the magical machine. None of it is working in practice. It's all purely theoretical. You start learning about different degrees of magic and it's pretty cool.
Lucas: Learning and understanding about what, in principle, is fundamentally computationally possible or feasible in certain time frames within the universe given the laws of physics that we have seems to be foundationally important and interesting. It's one of, I guess, the final frontiers. Not space, but I guess solving intelligence and computation and also the sort of hedonic qualia that comes along for the ride.
Roman: Right. I guess the magical aspect allows you to escape from your local physics and consider other types of physics and what would be possible outside of this world.
Lucas: What advances or potential advances in quantum computing or other sorts of more futuristic hardware and computational systems help and assist in these problems?
Roman: I think quantum computing has more impact on the cryptography and security in that way. It impacts some algorithms more directly. I don't think there is a determined need for it right now in terms of AI research or AI safety work. It doesn't look like a human brain is using a lot of quantum effects though some people argue that it's important for consciousness. I'm not sure if there is definitive proof of that experimentally.
Lucas: Let's go ahead now and turn to some questions that we've gotten from our audience.
Roman: Sounds good.
Lucas: I guess we're going to be jumping around here between narrow and short-term AI and some other questions. It would be great if you could let me know about the state of safety and security in current AI in general and the evaluation and verification and validation approaches currently adopted by the industry.
Roman: In general, the state of safety and security in AI is almost nonexistent. It's kind of we're repeating history. When we worked on creating Internet security was not something we cared about and so Internet is completely insecure. Then was started work on Internet 2.0, Internet of things. We're repeating the same mistake. All those very cheap devices made in China have no security but they're all connected and that's how you can create swarms of devices attacking systems.
It is my hope that we don't repeat this with intelligent systems, but right now it looks like we are. We care about getting them to the market as soon as possible, making them as capable as possible, the soonest possible. Safety and security is something most people don't know about, don't care about. You can see it in terms of number of researchers working on it. You can see it in terms of percentage of funding allocated to AI safety. I'm not too optimistic so far, but the field is growing exponentially, so that's a good sign.
Lucas: How does evaluation and verification and validation fit into all of this?
Roman: We have pretty good tools for verifying critical software. Something so important... you're flying to mars, the system cannot fail. Absolutely. We can do mathematical proofs to show that the code you created matches the design you had. It's an expensive process, but we can do a pretty good job with it. You can put more resources into verifying it with multiple verifiers. You can get any degree of accuracy you want as a cost of computational resource.
As far as I can tell, there is no or very little successful work on verifying systems which are capable of self-improvement, changing, dynamically learning, operating in novel environments. It's very hard to verify something where you have no idea what the behavior should be in the first beforehand. If it's something linear, again, we have a chess computer, we know what it's supposed to do exactly. It's a lot easier to verify than something more intelligent than you operating a new data in a new domain.
Lucas: Right. It seems like verification in this area of AI is going to require some much more foundational and difficult proofs and verification techniques here. It seems like you're saying it also requires an idea of an end goal of what the system is actually intended to do in order to verify that it satisfies that.
Roman: Right. You have to verify it against something. I have a paper on unverifiability where I talk about mathematical fundamental limits to what we can prove and verify mathematically. Already, we're getting to the point where our mathematical proofs are so complex and so long, most human mathematicians cannot possibly even check if it's legitimate or not.
We have examples of proofs where a mathematical community as a whole still has not decided if something published 10 years ago is a valid proof. If you're talking about doing proofs on a black box AI systems, now it seems like the only option we have is another AI mathematician, verify our AI, assisting us with that, but this creates this multiple levels of interaction where who's verifying, verifiers and so on.
Lucas: It seems to me at least another expression of how deeply interdependent the AI alignment problem is. Technical AI alignment is a core issue, but it seems like even in simple things, or not simple things, but things which you would imagine to at least be purely relegated to computer science also has some sort of connections with ethics and policy and law and how these things will all sort of require each other in order to succeed in AI alignment.
Roman: I agree. You do need this complete picture. Overall, I mentioned it a few times before in other podcasts. It feels like an AI safety, every time we analyze a problem, we discovered that it's like a fractal. There is then more problems under that one and you do it again. Despite the three levels, you still continue with this. It's an infinite process.
We never get to a point where, "Okay, we solved this. This is not a problem anymore. We know for sure it works in every conceivable situation." That's a problem. You have this infinite surface you have to defend, but you only have to fail once to lose everything. It's very, very different from standard cyber security where, "Okay, somebody stole my credit card. I'll just get a new one. I'll get to try again." Very different approach.
Lucas: There's no messing up with artificial superintelligence.
Roman: Basically.
Lucas: Just going off of what we were talking about earlier in terms of how AI safety researchers are flirting and interested in the applications of psychology in AI safety, what do you think about the potential future relationship between AI and neuroscience?
Roman: That is great work in neuroscience and trying to understand measurements from just observing neurons, cells to human behavior. There are some papers showing if we do the same thing with computer processors, we're just going to get a very good microscope and look at the CPU. "Was it playing a video game? Can we figure out connections between what Mario is doing and what electrical wiring is firing and so on?"
There seems to be a lot of mistakes made in that experiment. That tells us that the neuroscience experiments we're doing for a very long time may be providing some less-than-perfect data for us. In a way, by doing AI work, we can also improve on our understanding of human brain, medical science, just general understanding of how neural networks work. It's a feedback loop. That is progress in either one benefits the other.
Lucas: It seems like people like Josh Tenenbaum are working on more neuro inspired approaches to creating AGI. It seems that there are some people who have the view or the philosophy that the best way to getting to general intelligence is probably going to be understanding and studying human beings because we're in existence proof that can be studied of general intelligences. What are your views on this approach and the work being done there?
Roman: I think it's a lot easier to copy answers to get to the results. In terms of developing capable system, I think it's the best option we have. I'm not so sure it leads to a safe system because if you just copy design, you don't fully understand it. You can replicate it without complete knowledge and then instilling safety into it as a an afterthought, as a add-on later on, maybe even more difficult than if you designed it from scratch yourself.
Lucas: A more general strategy and approach, which gets talked about a lot in the effective altruism community: there seems to be this view and you can correct me here anywhere I might get this narrative sort of wrong. It seems important to build the AGI safety community, the AI safety community in general, by bringing more researchers into the fold.
If we can slow down the people who are working on capability and raw intelligence and bring them over to safety, then that might be a very good thing because it slows down the creation of the intelligence part of AGI and puts more researchers into the part that's working on safety and AI alignment. Then there's also this tension where ...
While, that is a good thing. It may be a bad thing for us to be promoting AI safety or AGI safety to the public community because they probably just ... Journalists would spin it and ruin it and trivialize it, turn it into a caricature of itself and just put Terminator photos on everything, which we at FLI are very aware that journalists like to put Terminator stuff on people's articles and publications. What is your general view about AI safety outreach and do you disagree with the respectability first approach?
Roman: I'm an educator. I'm a professor. It's my job to teach students, to educate the public, to inform everyone about science and hopefully more educated populace would benefit all of us. Research is funded through taxpayer grants. The public university is funded through taxpayers. The students paying tuition, the general public essentially.
If our goal is to align AI with values of the people, how can we keep people in the dark? They're the ones who are going to influence elections. They are the ones who are going to decide what good governance of AI essentially is by voting for the right people. We put so much effort into governance of AI. We have efforts at UN, European Parliament, White House, you name it. There are now agreements between France and Canada on what to do with that.
At the end of the day, politicians listen to the public. If I can educate everyone about what the real issues in science are, I think it's a pure benefit. It makes sense to raise awareness of long-term issues. We do it in every other field of science. Would you ever suggest it's not a good idea to talk about climate change? No, of course not. It's silly. We all participate in the system. We're all impacted by the final outcome. It's important to provide the good public outreach.
If your concern is the picture of a title of an article, well  work with better journalists, tell them you cannot use a picture of a Terminator. I do it. I tell them and they end up putting a very boring picture on it and nobody clicks on it. Is Terminator then an educational tool? I was able to explain some advanced computability concepts in a few minutes with simple trivial examples. Then you educate people, you have to come to their level. You have to say, "Well, we do have concerns about military killer robots." There's nothing wrong with that, so maybe funding for killer robots should be reduced. If public agrees, that's wonderful.
Just kind of going if an article I published or somebody interviewed me is less than perfect, then it's not beneficial, I disagree with it completely. It's important to get to the public, which is not already sold on the idea. Me doing interview for you right now, right? I'm preaching to the choir. Most of your listeners are into AI safety I'm sure. Or at least effective altruism.
Whereas if I do interview for BBC or something like that, now I'm getting access to millions of people who have no idea what superintelligence is. In my world and your world, this is like common knowledge, but I give a lot of keynotes and I would go and speak to top executives for accounting firms and I ask them basic questions about technology. Maybe one ever heard about superintelligence as a concept.
I think education is always a good thing. Having educated populace is wonderful because that's where funding will eventually come from for supporting our research and for helping us with AI governance. I'm a very strong supporter of outreach and I highly encourage everyone to do very good articles on it. If you feel that a journalist misrepresents your point of view, get in touch, get it fixed. Don't just say that we're going to left public in a dark.
Lucas: I definitely agree with that. I don't really like this elitism that is part of the culture within some parts of AI safety community, which thinks that only the smartest, most niche people should be aware of this and working on it given the safety concerns and the ways in which it could be turned into something else.
Roman: I was a fellow at the Singularity Institute for Artificial Intelligence what is now MIRI. At that time, they had a general policy of not publishing. They felt it was undesirable and will cause more damage. Now, they publish extensively. I had mentioned that, that's maybe a good idea a few times.
The general idea of buying out top AI developers and turning them to the white side I guess and working on safety issues, I think that's wonderful. We want the top people. It doesn't mean we have to completely neglect less than big names. Everyone needs to be invited to the table in terms of support, in terms of grants. Don't try to think that reputation means that only people at Harvard and MIT work in AI safety.
There is lots of talent everywhere. I work with remote assistance from around the world. There is so much talent out there. I think the results speak for themselves. I get invited to speak internationally. I advise governments, courts, legislative system. I think reputation only grows with such outreach.
Lucas: For sure and it seems like the education on this, because it can seem fairly complicated and people can be really confused about it because I think that there are lots of common myths that people have about intelligence and "consciousness construed" in some way other than how I think you or I construe the term consciousness or the idea of free will or what it means to be intelligent. There's just so much room for people to be confused about this issue.
The issue is real and it's coming and people are going to find out about it whether or not we discuss it now. It seems very important that this happens, but also because like ... It seems we also exist in a world where something like 40% to 50% of our country is at least skeptical about climate change. Climate change education and advocacy is very important and should be happening.
Even with all of that education and advocacy, there's still something like around 40% of people who are skeptical about climate change. That issue has become politicized where people aren't necessarily interested in facts. At least the skeptics are committed to party lines on the issue.
Roman: What would it be without education, if they never heard about the issue, would percentage be zero?
Lucas: I'm not advocating against education. I'm saying that this is an interesting existence case and saying like, "Yeah, we need more education about AI issues and climate change issues in general."
Roman: I think there are maybe even more disagreement, not so much about how true of a problem is, but how to fix it. It turns into a political issue, then you start talking about let's increase taxation, let's decrease taxation. That's what politicizes. That is not the fundamental science.
Lucas: I guess I just want to look this up actually just to figure out what the general American populace thinks. I think it was a bit wrong.
Roman: I don't think it's important what the exact percentage is. I think it's general concept we care about.
Lucas: It's a general concept, but I guess I was just potentially introducing a level of pessimism about why we need to educate people more so about AI alignment and AI safety in general just because these issues, even if you're extremely skillful about them, can become politicized. Just generally the epistemology of America right now is exploding in a giant mess of bullshit. It's just important that we educate clearly and correctly.
Roman: You don't have to start with the most extreme examples or I don't go with paperclip maximizers or whatever. You can talk about career selection, technological unemployment, basic income. Those things are quite understandable and they provide wonderful base for moving to the next level once we get there.
Lucas: Absolutely. Totally in agreement. How would you describe the typical interactions that you get from mainstream AI and CS researchers who just do sort of standard machine learning and don't know or really think or care about AGI and ASI? When you talk to them and pitch to them like, "Hey, maybe you should be working on AI safety." Or, "Hey, AI safety is something that is real, that you should care about."
Roman: You're right. There are different types of people based on their background knowledge. There is group one, which never heard of the concept. It's just not part of their world. You can start by just sharing some literature and you can follow up later. Then there are people who are in complete agreement with you. They know it's important. They understand the issue, but that's their job they're working and I think they are sympathetic to the cause.
Then there are people who heard a few kind of not the best attempts to explain what AI risk is, and so they are skeptical. They may be thinking about Terminator movie or something, Matrix, and so they are quite skeptical. In my personal experience, if I had a chance to spend 30 minutes to an hour with a person one-on-one, they all converted. I never had someone who went, "You told me things, but I have zero concern about intelligent systems having bugs in them or side effects or anything like that."
I think it's just a question of spending time and making it a friendly expedience. You're not adversaries trying to fight it out. You're just going, "Hey, every single piece of software we ever produced had bugs in it and can be had." How is this different?
Lucas: I agree with you, but there are also seems to be these existence proofs and existence cases of people who are computer scientists and who are super skeptical about AI safety efforts and working on ASI safety like Andrew Ng and others.
Roman: You have to figure out each individual case-by-case basis of course, but just being skeptical about success of his approach is normal. I told you my main concern, is the problem solvable. That's a degree of skepticism. If we looked at any other industry. Let's say we had oil industry. The top executive oil industry said that global climate change is not important. Just call it redistribution of good weather or something, it's not a big deal.
You would immediately think there is some sort of conflict of interest, right? But how is this different? If you are strongly dependent on development, not on anything else, it just makes sense that you would be 100% for development. I don't think it's unnatural at all. Again, I think a good conversation and realignment of incentives would do miracles for such cases.
Lucas: It seems like either because Andrew Ang's timelines are so long or he just thinks that they're fundamentally, like there's just not really a big problem. I think there are some computer scientists, researchers who just think there's just not really a problem, because we're making the systems and there are systems that are so intertwined with us that the values will just naturally mesh together or something. I'm just so surprised I guess that from the mainstream CS and AI people that you don't run into more skeptics.
Roman: I don't start my random interactions with people by trying to tell them, "You are wrong. Change your mind." That's usually not the best approach. Then you talk about specific cases and you can take it slowly and increase the level of concern. You can start by talking about algorithmic justice and bias in algorithms and software verification. I think you'll get 100% support at all those levels.
What happens when your system is slightly more capable, you're still working with me? I don't think there is a gap where you go, "Well, at that point, everything becomes rosy and safe and we don't have to worry about it." If a disagreement is about how soon, I think it's not a problem at all. Everything I argue still applies in 20 years, 50 years, 100 years.
If you're saying it will take 100 years to get to superintelligence, how long will it take to learn how to control a system we don't have yet? Probably way longer than that. Already, we should have started 50 years ago. It's too late now. If anything, it strengthens my point that we should put more resources on the safety side.
Lucas: Absolutely. Just a question about generally your work cataloging failures of AI products and what this means for the future.
Roman: I collect examples, historical examples starting with the very first AI systems, still everyday news of how AI systems fail. The examples you all heard about. Self-driving car kills a pedestrian. Or Microsoft Tay chat bot becomes racist and swears at people. I have maybe about 50 or 60 so far. I keep collecting new ones. Feel free to send me lots of cool examples, but make sure they're not already on my list.
The interesting thing is the patterns. You can get from it, learn from it and use to predict future failure. One, obviously as AI becomes more common, we have more of those systems, the number of such failures grows. I think it grows exponentially and impacts from them grows.
Now, we have intelligent systems trading in the stock market. I think they take up something like 85% of all stock trades. We had examples where they crash the whole stock market, brought down the volume by $1 trillion or something, closed significant amounts. This is very interesting data. I try to create a data set of those examples and there is some interest from industry to understand how to make their products not make my list in the future.
I think so far the only ... It sounds like a trivial conclusion, but I think it's fundamental. The only conclusion I have is that if you design an AI system to do X, it will very soon fail to X whatever X stands for. It seems like it's only going to get worse as they become more general because the value of X becomes not just narrow. If you designed a system to play chess, then it will fail to win a chess match. That's obvious and trivial. But if you design the system to run the world or something like that, what is X here?
Lucas: This makes me think about failure modes. Artificial superintelligence is going to have a probability space of failure modes where the severity of the failure at the worst end ... We covered this in my last podcast is it would literally be turning the universe into the worst possible suffering imaginable for everyone for as long as possible. That's some failure mode of ASI which has some probability which is unknown. Then the opposite on the other end is going to be, I guess, the most well-being and bliss for all possible minds, which exists in that universe. Then there's everything in between.
I guess the question is, is there any mapping or how important is it in mapping this probability space of failure modes? What are the failure modes that ASI can do or that would occur that would make it not value aligned? What are the probabilities of each of those given, I don't know, the sort of architecture that we expect ASI to have or how we expect ASI to function?
Roman: I don't think there is a worst and best case. I think it's infinite in both directions. It can always get worse and always get better.
Lucas: But it's constrained by what is physically possible.
Roman: Knowing what we know about physics and within this universe, there is a big multiverse out there possibly with different types of physics and simulated environments can create very interesting side effects as well. That's not the point. I also collect predicted failures of future systems, part of a same report. You can look it up. That's very interesting to see what usually a scientist, but sometimes science fiction writers, other people had said as potential examples.
It has things like paperclip maximizer and other examples. I look at predictions which are predictions but short-term. For example, we can talk about sex robots and how they're going to fail. Someone hacks them, then they forget to stop. You forget your safe word. There are interesting possibilities.
Very useful both as an educational tool to get people to see this trend and go, "Okay. At every level of AI development, we had problems proportionate to the capability of AI. Give me a good argument why it's not the case moving forward?" Very useful tool for AI safety researchers to predict. "Okay, we're releasing this new system tomorrow. It's capable of X." How can we make sure the problems don't follow?
I published on this, for example, before Microsoft released their Tay chatbot. Giving Xs to users to manipulate your learning data is usually not a safe option. If they just knew about it, maybe they wouldn't embarrass themselves so bad.
Lucas: Wonderful. I guess just one last question here. My view was that given a superintelligence originating on earth, there would be a physical maximum of the amount of matter and energy which it could manipulate given our current understanding and laws of physics, which are certainly subject to change if we gain new information.
There is something which we could call, as Nick Bostrom explains, the cosmic endowment which is sort of the sphere around an intelligent species, which is running a superintelligent optimization process. Where the sphere represents the maximum amount of matter and energy, a.k.a., galaxies a superintelligence can reach before the universe expands so much that it's no longer able to get beyond that point. Why is your view that there isn't a potentially physical best or physical worst thing that, that optimization process could do?
Roman: Computation is done with respect to time. It may take you twice as long to compute something with the same resources, but you'll still get that if you don't have limits on your time. Or you create a subjective time for whoever is experiencing things. You can have computations which are not in parallel, serial computation devoted to a single task. It's quite possible to create, for example, levels of suffering which progressively get worse I think. Again, I don't encourage anyone experimenting with that, but it seems like things can get worse not just because of limitations, of how much computing I can do.
Lucas: All right. It's really been a wonderful and exciting conversation Roman. If people want to check out your work or to follow you on Facebook or Twitter or wherever else, what do you recommend people go to read these papers and follow you?
Roman: I'm very active in social media. I do encourage you to follow me on Twitter, RomanYam, or on Facebook, Roman Yampolskiy. Just Google my name. My Google Scholar has all the papers and just trying to make a sell here. I have a new book coming out, Artificial Intelligence Safety and Security. It's an edited book with all the top AI safety researchers contributing, and it's due out in August, mid August. Already available for presale.
Lucas: Wow. Okay. Where can people get that? On Amazon?
Roman: Amazon is a great option. It's published by CRC Press, so you have multiple options right now. I think it's available as a softcover and hardcover, which are a bit pricey. It's a huge book about 500 pages. Most people would publish it as a five book anthology, but you get one volume here. It should come out as a very affordable digital book as well, about $30 for 500 pages.
Lucas: Wonderful. That sounds exciting. I'm looking forward to getting my hands on that. Thanks again so much for your time. It's really been an interesting conversation.
Roman: My pleasure and good luck with your podcast.
Lucas: Thanks so much. If you enjoyed this podcast, please subscribe, give it a like or share it on your preferred social media platform. We'll be back again soon with another episode in the AI Alignment Series.
Related episodes
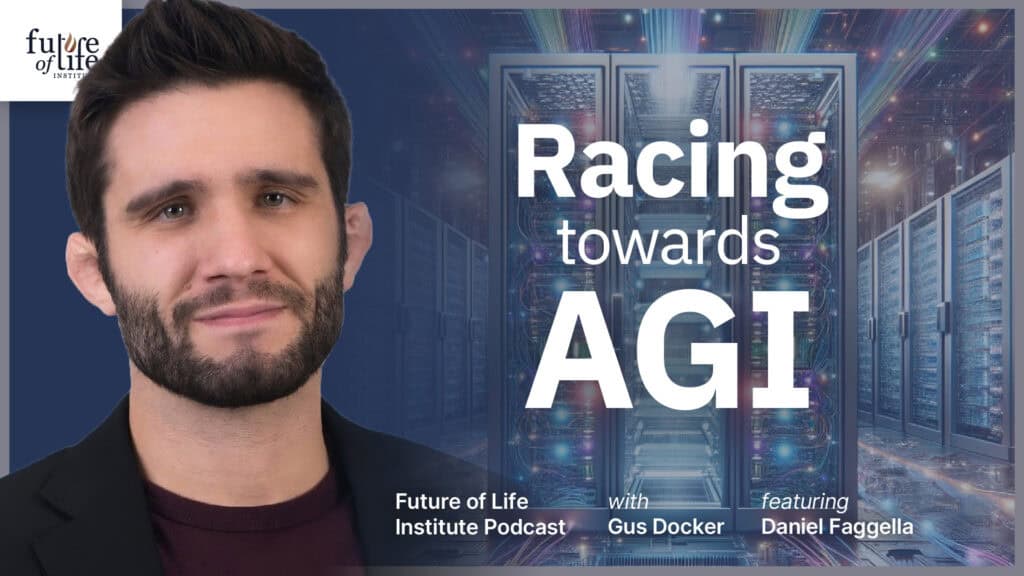
Dan Faggella on the Race to AGI
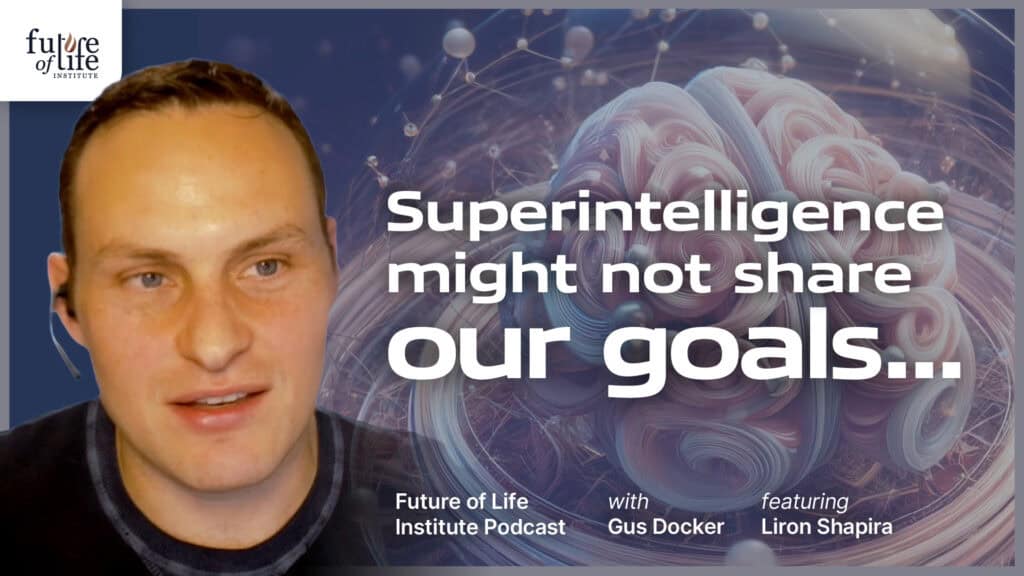