Podcast: The Art of Predicting with Anthony Aguirre and Andrew Critch
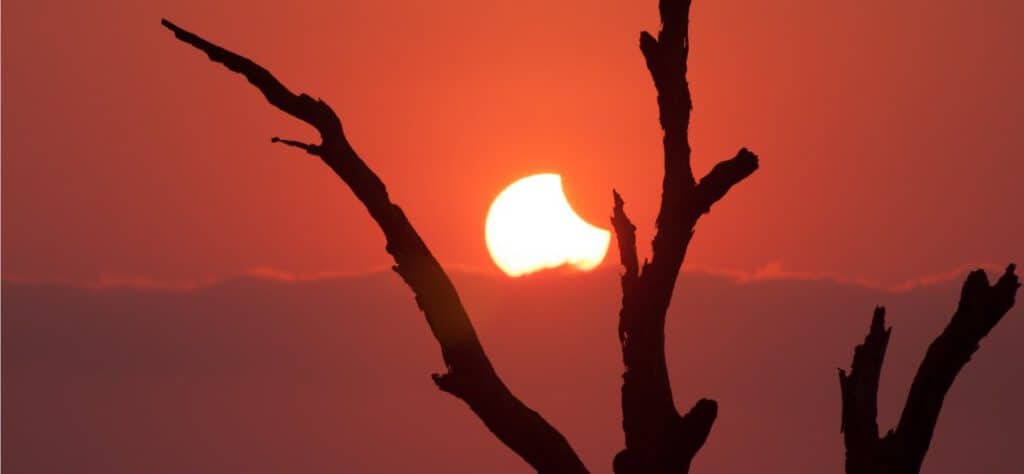
How well can we predict the future? In this podcast, Ariel speaks with Anthony Aguirre and Andrew Critch about the art of predicting the future, what constitutes a good prediction, and how we can better predict the advancement of artificial intelligence. They also touch on the difference between predicting a solar eclipse and predicting the weather, what it takes to make money on the stock market, and the bystander effect regarding existential risks.
Anthony is a professor of physics at the University of California at Santa Cruz. He's one of the founders of the Future of Life Institute, of the Foundational Questions Institute, and most recently of metaculus.com, which is an online effort to crowdsource predictions about the future of science and technology. Andrew is on a two-year leave of absence from MIRI to work with UC Berkeley's Center for Human Compatible AI. He cofounded the Center for Applied Rationality, and previously worked as an algorithmic stock trader at Jane Street Capital.
The following interview has been heavily edited for brevity, but you can listen to it in its entirety above or read the full transcript here.
Visit metaculus.com to try your hand at the art of predicting.
Transcript
Ariel: I'm Ariel Conn with the Future of Life Institute. Much of the time, when we hear about attempts to predict the future, it conjures images of fortune tellers and charlatans, but, in fact, we can fairly accurately predict that, not only will the sun come up tomorrow, but also at what time. Speaking of the sun, we've known about the eclipse that's coming up in August for quite a while, but we won't know whether cloud coverage will interfere with local viewing until much closer to the actual event.
As popular as mindfulness and living in the present have become, most of us still live very much in the future, with nearly every decision we make being based on some sort of prediction we've made, either consciously or subconsciously. On a larger scale, forecasting plays an important role in our lives in everything from predicting trends in finance to fashion, but especially, as emerging technologies like artificial intelligence, get stronger and more capable, it becomes increasingly important for us to predict and anticipate how things might change for humanity in the future and whether these changes are something we want to work for or actively avoid. To address how and why we want to improve society's ability to predict future trends, I have with me Anthony Aguirre and Andrew Critch.
Anthony is a professor of physics at the University of California at Santa Cruz. He's one of the founders of the Future of Life Institute, and, in an earlier collaboration with FLI cofounder, Max Tegmark, he founded the Foundational Questions Institute, which supports research on fundamental questions in physics and cosmology. Most recently, he's cofounded Metaculus, which is an online effort to crowdsource predictions about the future of science and technology.
Andrew is currently on a two-year leave of absence from MIRI to work with UC-Berkeley's Center for Human Compatible AI. During his PhD, he cofounded the Center for Applied Rationality and SPARC. Previously, Andrew has worked as an algorithmic stock trader at Jane Street Capital. His current research interests include logical uncertainty, open-source game theory, and avoiding race dynamics between nations and companies in AI development. Anthony and Andrew, thank you so much for joining us today.
Anthony: Thanks. Nice to be here.
Andrew: Me, too.
Ariel: To start, I want to get a better feel for essentially what predictions are. What are the hallmarks of a good prediction? How does that differ from just guessing? When we're looking at things like the upcoming eclipse, where we know the eclipse will happen, but we don't know what the weather will be like, how do predictions differ in those different situations? Anthony, maybe we can start with you.
Anthony: Okay, so I think I would say that there are maybe four aspects to a good prediction. One, I would say, is that it should be specific and well-defined and unambiguous, in the sense that if you predict something that's going to happen, when the appropriate amount of time has passed, everyone should agree on whether that thing has happened or not, or what the answer to the question that you asked was. You shouldn't have some sort of controversy as to whether the thing that you were making a prediction on has come to pass. This can be surprisingly difficult to do, because often there are many, many different possible ways the future can be, and there are things that you might not have thought of that will intervene between you and the thing that you've been predicting. One thing, I think, is to have a very clear definition of what is the thing that you're predicting? What is the number you're predicting or the event that you're predicting? Under what circumstances will you say that has happened or not happened, or what exactly the value of that thing that you're predicting will be? Specificity and well-definedness, I guess, is, I think, one aspect.
The second, I would say, is that it should be probabilistic. There's a well-known saying in experimental science that if there's no error bar on the number, then the number is totally meaningless, and that's right. If you don't know what the error bar is, the answer really could be anything. Similarly, saying, "I think it's going to rain tomorrow," different people will interpret that in dramatically different ways. Some people might take that as being it's 90% likely to rain tomorrow; some, it might be 51% likely to rain. What a really good prediction is, is a probability for something happening or a probability distribution, that is a probability assigned to each possible outcome of something happening. That's something you can really use and get an actual meaning out of when you're going to make decisions.
The third thing I think a prediction should be is precise, in principle. For example, if you say, "Is it going to rain tomorrow?"
"Well, I'll give it a 50% chance."
"Is team X going to win game Y?"
“Well, I'll give it a 50% chance."
In a sense, if you give everything a 50% chance, you'll never be terribly wrong, but you'll also never be terribly right. Predictions are really interesting to the extent that they give a fairly narrow distribution of possibilities or to the extent that they say something is either very likely or very unlikely. What you would like to do is say, "That event has a 0% chance, or a 1% chance; that event has a 99% chance," and be able to do that for lots of different things. In practice, we often can't get to that sort of precision, and we'll end up saying, "Well, it's 60% chance or 30% chance." Precision is what we would aim for.
To counterbalance that, I think the fourth criterion, I would say, is that you want to be well-calibrated, meaning that if there are 100 things that you predict with 90% confidence, around 90% of those things should come true, or if there are 100 things and you predict them with 50% confidence, around 50 of those should come true. What you don't want to have is, say, I'm making tons of predictions that this is 99% likely to happen, and then have a bunch of those things not happen. That's a very poorly calibrated set of predictions, and that will lead people badly astray if they listen to what you're predicting.
Those two things, the precision and the calibration kind of play off against each other, because it's easy to be well-calibrated if you just sort of randomly give everything 50% chance. It's easy to be precise by giving everything either a very high or a very low probability, but it's very difficult to be both about the future.
Ariel: Andrew, I wanted to ask you about your experience in finance and how that relates to these four areas that Anthony just brought up, in terms of trying to make predictions.
Andrew: Sure, yeah, thanks. Actually, I had a few general replies and comments I wanted to say to Anthony there. Of the properties Anthony said, being specific, meaning it's clear what the prediction is saying and when it will be settled. I think people really don't appreciate how psychologically valuable that is, because, of course, if you want to settle a bet, you want to be specific about how to settle it, but if you also want to install a mental reflex to check, in the future, whether your own thinking or your own perspective was correct on something, if you have a very specific trigger, like, "I think tomorrow at 5:00 p.m., John will not be at the party," now, when 5:00 p.m. comes, it jumps out at you. You're reminded, "Oh yes, there's a specific time when I'm supposed to check and see if my past state of mind regarding John was correct."
A person who does that regularly creates a feedback mechanism for learning to trust certain states of mind more than others. For example, they might learn that when they're feeling angry at John, they make miscalibrated predictions about John. They'll tend to exaggerate John's unreliability or something like that, and they'll say things like, "I'm sure he's definitely not going to be at the party."
If you install that trigger, then you'll have a concrete sense of personal accountability to yourself about whether you were right. I think a person who does that regularly is just going to learn to make better predictions. I think people really undervalue the extent to which the specificity property of prediction is not just a property of the prediction, but also part of your own training as a predictor, so just a big plus one for that property of prediction.
Of course, absolutely, you need to use probabilities, and, of course, yes, it's hard to get precise predictions, meaning predictions with probabilities close to zero or 100%, but the last property that Anthony said, being calibration, is if you think about it, it's really not just a property of a prediction. It's a property of a predictor, like a source of predictions or a person. You can look at any individual prediction and say, "That prediction was precise. It involved probabilities. It has specific settlement conditions," but you can't really just look at a single prediction and say, "That was a calibrated prediction," because calibration is a property of averages.
If you say, "I'm 90% sure that John will be late," and then John is early, were you right or were you wrong? The answer is, well, you were kind of wrong, but you weren't completely wrong. You had certainly assigned 10% chance to John being late, and you would have been more wrong if you said you were 99% sure, and you would have been less wrong if you'd said only 50%. There's degrees of wrongness. You are calibrated if your probabilities on average match your success rate on average, and you really need a suite of predictions to assess calibration. I think a good predictor is somebody who strives for calibration while also trying to be precise and get their probabilities as close to zero and one as they can.
In finance, if you want to – coming back to your question, Ariel – if you want to make money, you have to stick your neck out. You have to make a bet that nobody else was willing to make, because if they had already made the bet, the price would have already adjusted to reflect that. If I want to bet that Apple stock is going up, well, everybody who already thought Apple stock is going up has bought Apple stock, and everybody who already thought it's going down has sold Apple stock, and so I have to think I know something, or I've reasoned or discovered something, that no one else does, in order to make that prediction.
I'm really sticking my neck out at that point. I'm thinking, "I think that the price of Apple stock is going to go up with 51% probability, and everyone else thinks it's going to go up with 50.5% probability, and if I repeat this a lot of times, I'll make some money." It's a very precise claim, in total, to say that on average this particular stock trade is going to make money, because it's really, when you run a trading strategy, you're making a large number of predictions over and over all at once, and to say that you think that that is going to make money is a very bold claim. That's related to what Anthony called precision, but you might think of it as just a more general property of being bold enough to really know when you're wrong and to really stick your neck out and get some credit, be it winnings in the stock market or just kudos from your friends, who recognize you made an awesome prediction, when you're right.
Ariel: How are you using external data to make that prediction versus just guessing or using intuition? I think the idea of the external data also comes back to the question about the knowing that the eclipse will happen versus not knowing what the weather will be like yet.
Andrew: I don't think I would agree with that distinction. We have plenty of external data on the weather. The problem is that we know from experience that weather data is very unpredictable, and we know from experience that the locations of planets and moons and stars are predictable. This is how we learn to trust Newtonian mechanics, but we've not yet learned to trust any particular theories of fluid dynamics, which is what you'd need to model the weather. I wouldn't say that external data is what separates weather prediction from eclipse prediction. I would say that it's lack of a reliable model for making the prediction, or a reliable method. I would try to reorient the conversation, not to what's a good prediction, but what's a good process for making predictions? That's what allows you to ask questions of calibration, and it's what allows you to determine which experts to trust, because you can assess what their process is and ask, does this person follow a process that I would expect to yield good predictions? That's a different question from, is that a good prediction or not?
Anthony: Yeah, I certainly would agree. And, in particular, in terms of the eclipse and the weather, agree that it's all about what is the physical model underlying the prediction. There's a sense, in which fundamentally both of those things are almost exactly the same, from a physics perspective, that you have some set of initial conditions, which are positions of some material objects or conditions of the fluid of the atmosphere and the oceans and so on in a whole bunch of spots, where you have some set of well-defined initial conditions that you can know by making a set of measurements.
Then you have a known set of physical laws that evolve those initial conditions to some later state. Then you could just know what that later state is, given that mathematical model. If you're being good about being probabilistic, you would then also say, "Because I have some uncertainties in my initial conditions, then I'm not just going to run one model. I'm going to run a whole suite of models, with a whole set of different initial conditions, reflecting those uncertainties. By using that whole suite of models, I get a whole range of predictions, and I can then assess those and turn that into a probability distribution for what is actually going to happen."
That's done in both of those cases. For the eclipse, there is an incredibly accurate prediction of the eclipse this coming August, but there is actually some tiny, little bit of uncertainty in it that you basically don't see, because it's such an accurate prediction and we know so precisely where the planets are. If you look 1000 years ahead, predictions of eclipses are still amazingly accurate, but the uncertainty is a little bit bigger, so there won't be quite as narrow of a distribution on how long the duration of an eclipse will be at some particular point, because there are little bits of uncertainty that get propagated through the process.
When you look at weather, there's lots of uncertainty because we don't have some measurement device at every position measuring every temperature and density of the atmosphere and the water at every point on earth. There's just some uncertainty in the initial conditions. Then, even worse, the physics that you then use to propagate that amplifies lots of those initial uncertainties into bigger uncertainties later on. That's the hallmark of a chaotic physical system, which the atmosphere happens to be.
Even if you had a very good model for how to do the physics, it wouldn't really help, in terms of getting rid of that chaotic dynamics. The only thing that will help is getting better and better data and better and better resolution in your simulation. Then you can get predictions that are accurate, going from an hour out to maybe a day out to a few days out, but you're never going to get weather predictions that are accurate two weeks or a month or something. It's just not going to be possible. It's an interesting thing that the different physical systems are so different in their predictability.
Then, when you get to other systems, like social systems, it gets even harder, or systems like the financial one that Andrew was discussing, which actually sort of have a built-in ability to defeat predictions ... The problem with predicting the stock market is exactly what he said, that if you're trying to predict the stock market, so is everybody else, and the prices adjust to make it as difficult as possible to predict what's going to happen in the future in the stock market. There are systems that are just hard to predict and then there are systems, like stocks, which in some sense already represent the best possible prediction, but, in some sense, figuring out what the stock is going to do is something that very, very strongly resists precise prediction, because if you had that, anybody who has that would then use it and then affect the stock, and so that self-referential aspect to it makes it very, very difficult.
Andrew: I think that's a really important thing for people to realize about predicting the future, because I think they see the stock market, they see how unpredictable it is, and they know that the stock market has something to do with the news and it has something to do with what's going on in the world. If you see how hard it is to predict the stock market, that must mean that the world itself is extremely hard to predict, but I think that's an error. The reason the stock market is hard to predict is because of what Anthony says: It is a prediction, and predicting what's wrong with a prediction is hard.
If you've already made a prediction, predicting what is wrong about your prediction is really hard, because if you knew that, you would have just made that part of your prediction to begin with. And that's something to meditate on. The world is not always as hard to predict as the stock market. I can predict that there's going to be a traffic jam tomorrow on the commute from the East Bay to San Francisco, between the hours of 6:00 a.m. and 10:00 a.m. I can predict that with high confidence. It will happen. It will keep happening. And that's a social system, which I'll raise to push back a little bit on the full generality of what Anthony just claimed.
I think some aspects of social systems are actually very easy to predict. You can also make very easy predictions about the stock market that aren't actually built into the stock market prediction. For example, I can predict the volatility of a stock. I can predict that a stock is going to go up and down a lot of times this month and be right about that. I can even say things like, "I think that between 45 and 55% of movements in a stock, on a one-second time scale, are going to be up over the next month," which might seem like a bold claim. It might seem like I'm saying the stock is going to stay the same, but the moves have different sizes. On average, I expect, if you count the moves in Apple stock over the next month, over one-second time intervals, I'm quite confident that the number of those moves that are upward is between 45 and 55%. That's because no one's betting on that already, and there's no mechanism for baking that particular prediction into the price of Apple stock. The market is not designed to thwart that particular prediction, and the Bay Bridge is not designed to thwart my prediction that there will be a traffic jam on it, unfortunately.
I do want to caution that sometimes people think the future is harder to predict than it is, because they might conflate certain aspects of the future that are hard to predict, like the weather, with other aspects that are easy to predict, like an eclipse. Even combinations of hard to predict phenomena, like an individual human driver, might be very hard to predict. If you just see someone driving down a highway, you might have no idea where they're headed, but if you see 10,000 people driving down the highway, you might blur your eyes and get a strong sense of whether there's going to be a traffic jam soon or not. Sometimes unpredictable phenomena can add up to predictable phenomena, and I think that's a really important feature of making good long-term predictions with complicated systems.
Anthony: Yeah, I totally agree with that, and if I gave the implication that social systems are inherently unpredictable in the same way as weather is, I would like to not make that assertion.
Andrew: I figured. I figured.
Anthony: It's often said that climate is more predictable than weather, and that's quite true also, for the same sorts of reasons. Although the individual fluctuations day-to-day are difficult to predict, it's very easy to predict that, in general, winter in the Northern Hemisphere is going to be colder than the summer. There are lots of statistical regularities that emerge, when you average over large numbers.
We have a whole science called statistical mechanics, which is all about coming up with statistical descriptions of things, where, on the individual level, they're unpredictable. It would be, for example, very difficult to predict what some individual molecule in the air in this room is doing, given all its interactions with the other air molecules and so on. You'd lose track of it very, very quickly if you were trying to predict it precisely. Yet, the prediction of what the air in the room, in general, will do under certain circumstances is really quite easy or at least fairly straightforward in some circumstances.
If you put all the air in the corner of the room, what will it do? It will expand to fill the room. Now, trying to figure out what it will do in a more complicated thing, like when it's part of weather, is more difficult. I think the point is that there are many subtleties to this, and things that can be very easy to predict individually might be difficult when you combine many of them, or things that are very difficult to predict individually might become easier, when there are lots of them, and it really will depend on the system that you're trying to make the prediction for.
Ariel: I'd like to take all of that and transition to the question of what artificial intelligence will be like in the future. That's something that all of us are interested in and concerned about. As we're trying to understand what the impact of artificial intelligence will be on humanity, how do we consider what would be a complex prediction? What's a simple prediction? What sort of information do we need to do this?
Anthony: One of the best methods of prediction for lots of things is just simple extrapolation. There are many physical systems that, once you can discern if they have a trend, you can fit a pretty simple function to, a linear function or maybe an exponential, and actually do pretty well a lot of the time. There are things where a lot of prediction can be gleaned fairly easily by just getting the right set of data and fitting a simple function to it and seeing where it's going to go in the future. Now, this obviously has dangers, but it's a lot better than just sort of guessing or waving your hands, and, in many cases, is pretty comparable to much more sophisticated methods.
When you're talking about artificial intelligence, there are some quite hard aspects to predict, but there are also some relatively easy aspects to predict, like looking at the amount of funding that's being given to artificial intelligence research or the computing power and computing speed and efficiency, following Moore's Law and variants of it. You can look closely at what exact exponential will be followed or whether the exponential will turn something else, even out or something. You won't do badly – at least, that has been the case up until now – you won't do badly extrapolating some exponential to some of these things, and that would be a pretty good mainline prediction.
While there are things that we can say we have no idea what they'll be like in five years, I think we have a pretty good idea, for example, that computing power will be significantly better by maybe a factor of eight or something in some metric, if it's three doubling times, according to some version of Moore's Law. This is a multifaceted problem, but I think there are aspects that fairly simple methods could be applied to, and not even that has been done all that well or put together really cleanly in one place, although some people are trying, I think.
Andrew: Yeah, I think if I imagine someone listening to this podcast and reading about a prediction, just like a linear extrapolation or a log linear extrapolation of hardware progress that says we're going to keep doubling. When Anthony and I discussed earlier that weather is hard to predict, neither of us said this, but I imagine we were both secretly thinking about some results we know from the field of math called chaos theory, which sometimes you can use to mathematically prove that a certain system, if it behaves according to certain laws, is unpredictable, which is interesting. People often think of mathematics as a source of certainty, but sometimes you can be certain that you are uncertain or you can be certain that you can't be certain about something else.
Weather is just one of those things that we have a high degree of certainty, because of some things we know about mathematics that tell us that weather probably is just going to remain difficult to predict, and that there's mathematical reasons for it that make us think that we're not going to discover a better measurement device that just makes weather prediction easy. In the same way that Anthony and I predict, and you can call me on this prediction, I predict that Anthony will admit to thinking about this mathematical fact while he was talking about the weather just now.
Anthony: Guilty.
Andrew: Guilty? Yep, called it. In the same way, when you use a simple trend, like Moore's Law, to predict hardware, you should ask yourself, what simple, underlying mathematical rules might be driving Moore's Law. Moore's Law is a summary of what you see from a very complicated system, namely a bunch of companies and a bunch of people working to build smaller and faster and cheaper and more energy efficient hardware. That's a very complicated system that somehow adds up to fairly simple behavior, like Moore's Law, in the same way that a very complicated system of stressed out individual drivers, going to different jobs, with different salaries, and different reasons for their jobs, are all driving to the city for some reason and exhibiting this fairly predictable traffic jam every morning.
Somehow these small-scale complicated phenomena can add up to a predictable one. If you want to use Moore's Law to make predictions in the long-term, you can ask yourself, what are the small scale phenomena that are allowing Moore's Law to continue. You could actually think about that. When I was in finance, we tried really hard to always ask ourselves, when we found a trend ... We analyzed a bunch of data, we found a trend, it looks like that trend's going to make us some money, but we would always ask ourselves, what is adding up to this trend? What are the parts? What is the analog of the individual drivers on the highway or the hardware engineers at IBM that are adding up to this trend?
If you can at least have a guess as to what that trend is, you might realize that the trend's not going to continue for some important reason. In the case of Moore's Law, you can think, well, the reason it's getting smaller is because we're using smaller things, to build smaller tools, to make smaller things, to understand smaller things, to build smaller tools, to make smaller things, etc. You can actually sort of visualize the ‘smallification’ of things in such a way that, when you take into account your knowledge of physics, you realize that it's got to stop getting smaller at some point. We know that there has to be a point at which Moore's Law will stop, and that's because we have some understanding of the smaller phenomena, namely engineers trying to make things smaller – pardon the pun – that add up to the larger scale phenomena of generally making progress at making smaller, faster, cheaper hardware.
I think, to use your phrase, a hallmark of good prediction is, when you find a trend, the first question you should ask yourself is what is giving rise to this trend, and can I expect that to continue? Does that make sense to me? That's a bit of an art. It's kind of more art than science, but it's a critical art, because otherwise we end up blindly following trends that are bound to fail.
Anthony: Yeah.
Ariel: I think that actually moves me into the next question that I want to ask. We've been talking about what's involved in making good prediction. I want to ask a little bit more about who is making the prediction. With AI, for example, we're seeing very smart people in the field of AI, who are predicting that AI will make life great, and others are worried that it will destroy us. With existential risks, in general, one of the things we see are a lot of surveys and efforts in which experts in the field try to predict the odds of whether or not humans will go extinct or whether some disaster will happen in the next 10 years or 100 years. I'm wondering, how much can we rely on "experts in the field"?
Andrew: I can certainly tell you that thinking for 30 consecutive minutes about what could cause human extinction is much more productive than thinking for one consecutive minute. It's interesting to note that very few people I know have actually thought for more than 30 consecutive minutes at any given time about what could lead to human extinction. There are a lot of people who have thought about it for 30 seconds at a time, maybe 30 seconds at a time 60 times or more at cocktail parties, at the bus stop, watching a movie, whatever, but those 30 seconds might be the same 30 seconds of thought every time. When you give yourself time to think a little bit longer, you can rule out some very basic, obvious conclusions.
For example, people often will think asteroids are an existential risk. It's so available. We've seen it in movies, but asteroids, if you'd think about it for a little bit, like maybe three whole minutes, you'll realize that, well, asteroids are still following the same rules that they were following last century and the century before that. We've had so many centuries now, that we see a pattern, which is that asteroids don't cause human extinction every year. In fact, for a large number of years, we've seen no human extinction caused by asteroids, and it seems very unlikely that we'll see human extinction caused by an asteroid in the next hundred years.
Will it ever happen? Yes, but those things happen very infrequently. In the same way you can predict an eclipse, you can predict that an asteroid is almost certainly not going to cause human extinction this century. I would bet, 99.99% chance, that we will not be extinct from an asteroid impact. Such a basic conclusion can just slip by, if you don't seriously think in an intellectually serious manner about what could or could not cause human extinction that ... Well, I worry that people associate extinction with movies and fiction in such a way that they don't sufficiently connect their reasoning and their logic with their discussions of extinction.
You, the listener, can easily verify, from listening to me speak, that it would be a mistake to think that asteroids will cause human extinction in this century, but I claim that there are other, harder to notice mistakes about human extinction predictions that you probably can't figure out from 30 seconds of reasoning or three minutes of reasoning, but, if you think about it for an hour, it's enough to figure out that certain threats to human extinction are really not to be concerned about. For example, a naturally occurring virus, I think, is extremely unlikely to cause human extinction. That’s a thing that will take a longer argument to convince you of than my claim that asteroids will not cause human extinction, but I do what to proffer that there are sloppy and careful ways of reasoning about human extinction, and not everyone's being sloppy about it.
That's something to watch for, because not everyone who's an expert, say, in nuclear engineering is also an expert in reasoning about human extinction, or not everyone who's an expert in artificial intelligence is an expert in reasoning about human extinction. You have to be careful who you call an expert, and you have to be careful not to just blindly listen to surveys of people who might claim to be an expert in a field and be right in that claim, but maybe who haven't ever in their life sat down for 60 consecutive minutes with a piece of paper to reason through scenarios that could lead to human extinction. I think, without having put in that effort, a person can't claim to be an expert in any human extinction risk, I think. In any plausible human extinction risk, I should say.
Anthony: Yeah, I think that… I really agree, and I also feel that something similar is true about prediction, in general, that making predictions about things is greatly aided if you have domain knowledge and expertise in the thing that you're making a prediction about. That's somewhat necessary, but far from sufficient to make accurate predictions.
Andrew: Absolutely.
Anthony: One of the experiences I've seen, running Metaculus and seeing things that are happening on it is that there are people that know a tremendous amount about a subject and just are terrible at making predictions about it. Other people, who, even if their actual domain knowledge is lower, the fact that they are comfortable with statistics, that they've had practice making predictions and checking themselves and thinking about their own biases and uncertainties and so on are just much, much better at it. I think, ideally, it would be nice to combine the level of domain expertise that some of these surveys take with a selection on people who are actually good at predicting. That's a difficult thing to do. That's something that we're aspiring to do, but it makes me take the results with a pretty big grain of salt, because I know how difficult it can be to make predictions about things, even when you know all about them, if you're not just used to making predictions and going through that process and thinking rather carefully about them.
The fact that I know lots about black holes, for example, from my physics research, if you then ask me what is the probability that this paper about black holes will get this many citations, well, I might throw out a number, but it's going to be a pretty poor number compared to someone who knows nothing about black holes, but has actually counted typical numbers of citations of papers of certain people, and how citations grow with time, and so on. Someone who knows nothing about black holes could do a much better job than me in making that prediction, if they've actually put in the time and homework to figuring out how to predict citations well. Now, if I had those techniques, and I knew the field, and I knew what ideas were interesting in black holes, and so on, then I could probably do a better job than them. I do think that both aspects really are quite important. It's not clear that a lot of the discussion about making predictions in the future of technology really leverages both.
Ariel: Anthony, with Metaculus, one of the things that you're trying to do is get more people involved in predicting. What is the benefit of having more people participating in these predictions?
Anthony: Well, I would say there are a few benefits. One is that lots of people get the benefit of practice, so I think just ... Andrew was talking earlier about the personal practice of thinking about a prediction that you have and then checking whether it comes true or not, and then circling back and saying, "Why was I so wrong on this?" and thinking about things that you tend to be more wrong on and what they might correlate with and so on. That's incredibly useful and makes you a more effective person if you do that. One thing that I hope is that the people who use Metaculus and stick with it and actually make their precise predictions and make them probabilistic and then find out how they're doing will get that feedback that will make them better at it. I think, in terms of personal growth, it's an interesting thing.
In terms of actually creating accurate predictions, the more people you have doing it, I think there are two benefits. One is that you'll have more people who are really good at it. If you have 10,000 people doing something, and you just take the top 1% of them, in terms of effectiveness, now you have 100 people, which is a lot better than if you have one person doing it, where the chance is one in 100 that you'll even get someone who's good. Just sheer numbers, along with an ability to identify who is actually good at predicting, means that you can then figure out who is good at predicting, and who is good at predicting a particular type of thing, and then use the predictions from them.
One of the interesting things that we've seen and has been shown previously is that there is a skill. It isn't just luck. You might suppose that the people who have a good prediction track record just got lucky again and again, and the fraction will get smaller and smaller of people who get lucky again and again, but, in fact, that's not true. There is, of course, luck, but there is an identifiable ability, where if you look at the people who have done really well up until some time, and then look at how those people do, compared to other people who didn't do as well up until some time, on future predictions, it is a good predictor, if you will, of who's a good predictor. There is a skill that people can develop and obtain, and then can be relied upon in the future. Part of the hope is that getting enough people involved, you can then figure out who are the really good people.
Then, the third, and maybe this is the most important, is just statistics. It's true that aggregating lots of people's predictions tends to make a more accurate aggregate, as long as you do it reasonably well. Even just taking the median turns out to be better than almost everybody as an individual predictor, as it turns out, but if you do a better job of it you can do even better.
Andrew: Anthony, when you say doing a better job of taking the median, do you mean somehow identifying good predictors and using them or giving them more weight or what sort of techniques do you have in mind?
Anthony: Yes, a couple things we've experimented with and work are: One is just giving people a greater weight if they've done better in the past, so that's definitely helpful. A second is once you have enough people who've made enough predictions, you can also take the calibration that they have, which is sometimes good and sometimes not so good, and you can fix it, so we can now see ... Typically, when people predict 95% probability of something, it's actually more like 80%. This is a well known cognitive bias of ... It's called overconfidence, but it's probably several different things.
Once you have enough people that you have a good statistical model of that overconfidence, then you can undo it. You can correct for it in an actually quite useful way. We've experimented with a few different things. One is recalibration. One is weighted predictions. Both of those are quite helpful and can do a lot better than the median, as it turns out, or the mean or something else.
Andrew: Anthony, if I log into Metaculus and I see the house odds on a certain prediction, like who's going to win an election, are those odds derived from one of your favorite techniques for aggregating the market participants or are those techniques experimental and not part of the mainline website right now?
Anthony: We've just rolled out ... Right now, if you get in, if the question is still open for prediction, then what you'll see is just the median. Once it's closed and nobody else is predicting, then we show the more carefully aggregated prediction. We do that so that it won't create a feedback process, where everybody's just piling on the better calibrated, better computed prediction. We could think about that in the future, but right now, yes, we've rolled out a more accurate one that you can see for questions that are no longer open for prediction.
Andrew: It'd be really interesting to think about what the equilibrium would be, if there's any aggregation mechanism, such that, when you share it, it doesn't wreck itself.
Anthony: Yes, it would.
Andrew: That'd be amazing.
Ariel: Is there anything else that people should know about Metaculus, while we're still on the topic?
Anthony: I think the main thing that people should know about Metaculus is just that it exists and that it's an effort to do a better job of making predictions about things, for which an already existing mechanism of making that prediction doesn't exist. If you're interested in the value of some company, you're best looking at the stock market and not something like Metaculus. If you're interested in the eclipse, you're best consulting NASA and their eclipse tables, but there are lots of things for which there is not a preexisting way of making an accurate prediction about it, and it's an effort to create a general purpose platform for doing that, by taking people, who are still the best prediction machines, by far, that exist, and combining their abilities. I would just encourage people to check it out and, if they like it and enjoy it, to take part in it.
Ariel: Okay.
Andrew: I would also just like to say that I think the existence of systems like Metaculus are going to be really important for society improving its ability to understand the world. There are very crude ways of putting opinions together, like let's just have a vote. Maybe that's a good way of sharing power, because everybody gets an equal share of power, but it might not be a good way of sharing reasoning, because maybe not everybody has thought about a scientific question for the same length of time. If we conducted a vote on whether global warming was real, there may have been a time when the vote would have decided that it wasn't. Of course, fortunately now, that's not the case anymore, but it's interesting to notice why it is that the wisdom of the crowds not only sometimes fails, but fails in a predictable way.
There are questions that you can actually predict that the crowd will be wrong about, if you think about whether or not the crowd has any reason to think about the question. If a question is hard and everyone has had a chance to think about it for an hour, a solid hour, then you might think that the crowd is going to have some wisdom about it. But if there's a question you know is hard, and you know, for some reason, everyone's too busy to think about it, like human extinction, for example, you might not actually think the wisdom of the crowds is going to be so wise. It might be more wise than an individual, as Anthony said, a random individual, but it probably isn't nearly as wise as what you'd get if you do what Anthony says and pull together some people, who you know are good at prediction, and ask them, or if you pull together some people, just that you know have actually thought at length about the issue, which is different, again, from being an expert in some field. It's the just whether or not you've put in the time.
I think if you look at whose job it is – whose job is it to think for a solid hour about a human extinction risk? – the answer is almost nobody. While it is someone's job to predict the stock market, and it is someone's job to predict a patient's health, and so on, there are many kinds of predictions that someone is on deck to actually think about, and so we get people who are good at those predictions. There are very few people in the world, whose job it is to become good at predicting threats to human civilization at large, and so we ought not to expect that just averaging the wisdom of the crowds is going to do super well on answering a question like that, compared to pooling the opinions of people that you know have thought at length about the question.
Ariel: I'm not sure if this is along the same lines or not. I want to bring it back to artificial intelligence, quickly, and the question of timelines, because I know a lot of people have actually thought about timelines, when certain capabilities will be available, when AI will displace too many jobs, when it will achieve human level intelligence and exceed human level intelligence. I guess I'm curious as to how helpful is it for us to try to make predictions? Who should be trying to make those predictions? Can we expect them to be very accurate? How can we make them more accurate? Is it something we should all be worrying about, or should certain people be worrying about it?
Andrew: I certainly see this happening in the field of AI, kind of what Anthony said, where domain expertise is necessary for good prediction, but not at all sufficient. You need to put in the time and do your homework. I see people making predictions like we're most likely to get artificial intelligence from scanning a human brain and running a simulation of that brain, which, on the surface, has a reasonable argument for it. It makes sense that a lot of things that we've built, we've copied from nature, so it makes sense that we could copy intelligence from nature too, namely, our brain.
But if you actually plot out a timeline, just draw on a piece of paper, what you think is going to be going on each decade between now and when you think we'll have, say, scanned and run a simulated copy of a human brain, it's interesting to ask, what do you think was going on in the decade before that? If you force yourself to go through this mental exercise… If you think, in your fictitious future, in your fictitious timeline, we will have a scanned, uploaded brain in the year 2090. Then, if you force yourself to do the exercise, you're now asking, what's happening in 2080?
Well, the answer is, somehow we're close. Somehow we've got lots of scanning technology. We've got lots of computer hardware that’s sufficient to run simulated copies of entire human brains. To say that the first computer system that ever matches human general intelligence will arise in 2090 from scanning a brain is also to assert logically that it will not have arisen in 2080 from other advances that could come from that same brain scanning technology and that same computer hardware. That's where it starts to get a little awkward. You have a little more difficulty holding that hypothesis in your mind when you visualize the whole timeline, because, once we can scan a brain in full resolution and simulate the entire thing, it could mean that a decade prior we gained a lot of insight into, say, how the basal ganglia work or how the cortex works or how various brain regions work.
I find it a little hard to imagine that we'll get all the way to scanning an entire human brain and simulating it, without having managed to pull out some components of the brain and hack them together in an engineering effort to do just as well. It's, of course, logically possible, but somehow the exercise of forcing yourself to work through the logic of how and why certain timelines could arise, it can change what, on the surface, seems like a reasonable guess. I think this is an extremely valuable exercise for the world to be carrying out. Do I want everyone to do this? No. Do I want every AI researcher or even every AI safety or control researcher to be doing this? No.
In fact, I now have made a career shift to trying to design control mechanisms for highly intelligent AI. I made that career shift, based on my own personal forecast of the future and what I think will be important, but I don't reevaluate that forecast every day, just as I don't reevaluate what neighborhood I should live in every day. I schedule a few deep reflection periods every few years to think, maybe I should move neighborhoods, but otherwise I stick to my interim policies that I choose in these longer, deeper reflections periods. Just as you don't re-plan your quarter every day – you plan your quarter maybe once a quarter or twice a quarter – you don't plan your career or your strategy for, say, having a positive impact on AI every day. You, at some point, need to commit to a path and follow that path for a little while to get anything done.
The question of who should be making timeline predictions ... I think if I were looking at the earth from a bird’s eye view and thinking, what would a reasonable civilization do? I think most AI researchers should, at some point, do the mental exercise of mapping out timelines and seeing what needs to happen, but they should do it deeply once every few years in collaboration with a few other people doing it deeply once every few years, and then stick to something that they think is going to help steer AI in a positive direction, based on that analysis for a few years, get some good work done, and then reevaluate after a few more years, so that they have a chance to pivot to a new research strategy, if they discover something they think is important to focus on, but still they don't reevaluate so frequently that they don't get any research done.
I see a lot of people – people who have managed to be concerned about AI safety and control – I see a little bit of a tendency to too frequently reevaluate timeline analyses of what's going to happen in AI. I think that every day someone in the world should be analyzing AI timelines, but it should be a different person every day perhaps. Then, once they're done with their analysis, they should choose a career path that will help them to benefit the world, if that timeline comes to pass. I think my answer to you is kind of everyone, but not everyone at once.
Ariel: Okay, and Anthony, was there anything you wanted to add?
Anthony: No, I think that is a good prescription, and I think there's one other interesting question, which is the degree to which we want there to be accurate predictions and lots of people know what those accurate predictions are. This is something we actually thought about when we started to put AI prediction questions on Metaculus. Suppose this was actually really successful, and we had really high confidence in the predictions that were coming out of the system, is that a problem in some way?
I think you can certainly imagine some scenarios, in which it's a problem. In general, I think more information is better, but it's not necessarily the case that more information that everybody has access to is better all the time. I'm interested in Andrew's views on this. It's certainly something that we worried about, in the sense ... Suppose, for example, that I became totally convinced, using Metaculus, that there was a really high probability that artificial superintelligence was happening in the next 10 years. That would be a pretty big deal obviously. I'd really want to think through, before shouting that information from the rooftops, what effect that information would actually have on various actors, national governments, companies, and so on. It could instigate a lot of issues. As with any, I think, information about potentially dangerous things, there are potentially information hazards having to do with predictions, and those are things that I think we have to really carefully consider.
Andrew: Yeah, so Anthony, I think that's a great important issue. I don't think there are enough scientific norms in circulation, in general, for what to do with a potentially dangerous discovery. Honestly, I feel like the discourse in most of science is a little bit head in the sand about the, in principle, feasibility of creating existential risks from technology. Even though I might not be so sure that any particular technology is going to pose an existential threat to the continued existence of human civilization, if I zoom out, if I look at the world and I see how much effort individuals are putting into ensuring that their innovations are not going to lead to human extinction, I really just don't see a lot of effort, and I don't see anyone whose job it is to put in that effort.
You might think that it would be so silly and dumb for the earth to accidentally have some humans produce some technology that accidentally destroyed all the humans and a bunch of other life, but, just because it's silly doesn't mean it won't happen, because I'm sure you've made the mistake of going to a party with five friends, and it would be silly, with five people going to the potluck, for no one to bring something to eat, but what happened is that everyone thought someone else would do it. It's the bystander effect. I think it's very easy for us to fall into the trap of like, "Well, I don't need to worry about developing dangerous technology, because if I was close to something dangerous, surely someone would have thought that through and made it a grant stipulation for my research that I should be more careful. Surely, someone would tell me if I was in the vicinity of something dangerous. My colleagues aren't worried about ever producing dangerous artificial intelligence or dangerous synthetic viruses or whatever it is you could worry about, so I'm not worried myself."
You have to ask, whose job is it to be worried? If the answer is no one on the way to the party was elected as the point person on bringing the food, then maybe no one will bring the food and that will be bad. If no one in the artificial intelligence community is point on noticing existential threats, maybe no one will notice the existential threats and that will be bad. The same goes for the technology that could be used by bad actors to produce dangerous synthetic viruses.
The first thing I want to say is, yeah, go ahead and be a little bit worried on everyone's behalf. It's fine. Go ahead. It's fine. Be a little bit worried. With that worry, then, what if what you discover is not a piece of technology, but a piece of prediction, like Anthony said? What if you discover that it seems quite likely, based on the aggregate opinion of a bunch of skilled predictors, that artificial general human intelligence will be possible within 10 years? Well that, yeah, that has some profound implications for the world, for policy, for business, for military. There's no denying that. I feel sometimes there's a little bit of an instinct to kind of pretend like no one's going to notice that AGI is really important. I don't think that's the case.
I had friends in the 2010 vicinity, who thought, surely no one in government will recognize the importance of superintelligence in the next decade. I was almost convinced. I had a little more faith than my friends, so I would have won some bets, but I still was surprised to see Barack Obama talking about superintelligence on an interview. I think the first thing is not to underestimate the possibility that, if you've made this prediction, maybe somebody else is about to make it, too.
That said, if you’re Metaculus, maybe you just know who's running prediction markets, who is studying good prediction aggregation systems, and you just know no one's putting in the effort, and you really might know that you're the only people on earth who have really made this prediction, or maybe you and only a few other think tanks have managed to actually come up with a good prediction about when superintelligent AI will be produced, and, moreover, that it's soon. If you discovered that, I would tell you the same thing I would tell anyone who discovers a potentially dangerous idea, which is not to write a blog post about it right away.
I would say, find three close, trusted individuals that you think reason well about human extinction risk, and ask them to think about the consequences and who to tell next. Make sure you're fair-minded about it. Make sure that you don't underestimate the intelligence of other people and assume that they'll never make this prediction, but ... Anthony, this isn't advice to you. I wouldn't expect you to make this mistake. A person who's built an expert aggregation system does not ... You don't remind me of someone who underestimates the value of other people's intelligence, but, as a general piece of advice, I think it's important not to do that.
Then do a rollout procedure. In software engineering, you developed a new feature for your software, but it could crash the whole network. It could wreck a bunch of user experiences, so you just give it to a few users and see what they think, and you slowly roll it out. I think a slow rollout procedure is the same thing you should do with any dangerous idea, any potentially dangerous idea. You might not even know the idea is dangerous. You may have developed something that only seems plausibly likely to be a civilizational scale threat, but if you zoom out and look at the world, and you imagine all the humans coming up with ideas that could be civilizational scale threats.
Maybe they're a piece of technology, maybe they're dangerous predictions, but no particular prediction or technology is likely to be a threat, so no one in particular decides to be careful with their idea, and whoever actually produces the dangerous idea is no more careful than anyone else, and they release their idea, and it falls into the wrong hands or it gets implemented in a dangerous way by mistake. Maybe someone accidentally builds Skynet. Somebody accidentally releases replicable plans for a cheap nuclear weapon.
If you zoom out, you don't want everyone to just share everything right away, and you want there to be some threshold of just a little worry that's just enough to have you ask your friends to think about it first. If you've got something that you think is 1% likely to pose an extinction threat, that seems like a small probability, and if you've done calibration training, you'll realize that that's supposed to feel very unlikely. Nonetheless, if 100 people have a 1% chance of causing human extinction, well someone probably has a good chance of doing it.
If you just think you've got a small chance of causing human extinction, go ahead, be a little bit worried. Tell your friends to be a little bit worried with you for like a day or three. Then expand your circle a little bit. See if they can see problems with the idea, see dangers with the idea, and slowly expand, roll out the idea into an expanding circle of responsible people until such time as it becomes clear that the idea is not dangerous, or you manage to figure out in what way it's dangerous and what to do about it, because it's quite hard to figure out something as complicated as how to manage a human extinction risk all by yourself or even by a team of three or maybe even ten people. You have to expand your circle of trust, but, at the same time, you can do it methodically like a software rollout, until you come up with a good plan for managing it. As for what the plan will be, I don't know. That's why I need you guys to do your slow rollout and figure it out.
Ariel: Super quickly, so that we can possibly end on a positive note, is there something hopeful that you want to add real quick?
Anthony: Well, I guess I would just say that the way I view it, pretty much every decision that we make is implicitly built on a prediction. You sort of predict the consequences of one decision or the other, and then you choose, between them, the one that you would like to have happen, and that's the basis for your decision. I think that if we can get better at predicting, individually, as a group, as a society, that should really help us choose a more wise path into the future, and hopefully that can happen.
Andrew: Hear, hear.
Ariel: All right. Well, I highly encourage everyone to give predicting a try themselves and visit metaculus.com. Anthony and Andrew, thank you so much for joining us today.
Anthony: Thanks for having us. It's been fun.
Andrew: Thanks, Ariel.
Related episodes
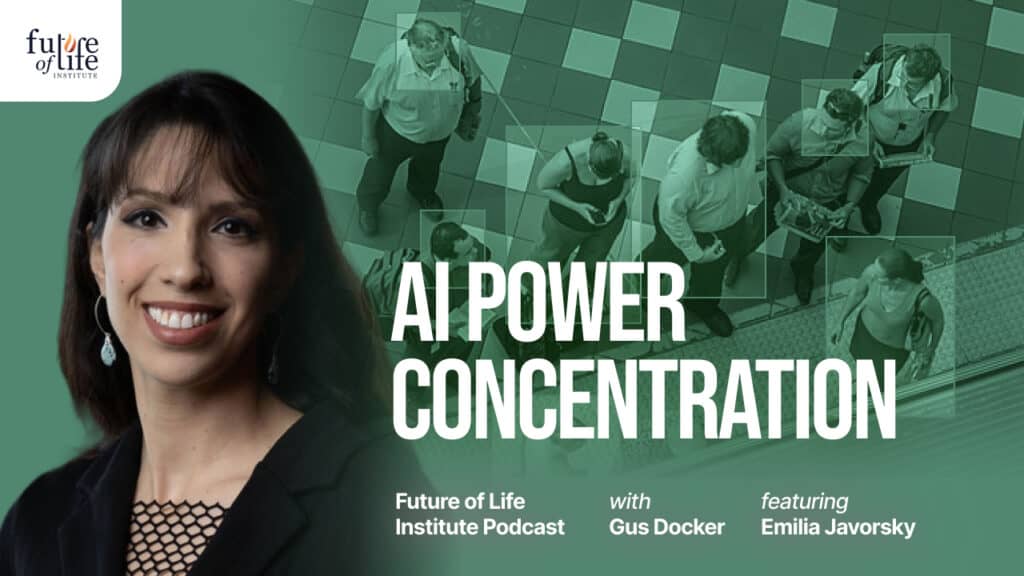