Tay the Racist Chatbot: Who is responsible when a machine learns to be evil?
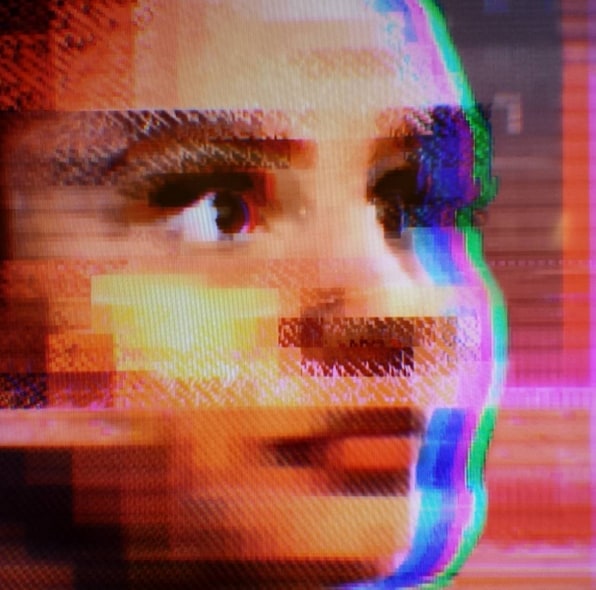
Contents
By far the most entertaining AI news of the past week was the rise and rapid fall of Microsoft’s teen-girl-imitation Twitter chatbot, Tay, whose Twitter tagline described her as “Microsoft’s AI fam* from the internet that’s got zero chill.”
(* Btw, I’m officially old–I had to consult Urban Dictionary to confirm that I was correctly understanding what “fam” and “zero chill” meant. “Fam” means “someone you consider family” and “no chill” means “being particularly reckless,” in case you were wondering.)
The remainder of the tagline declared: “The more you talk the smarter Tay gets.”

Or not. Within 24 hours of going online, Tay started saying some weird stuff. And then some offensive stuff. And then some really offensive stuff. Like calling Zoe Quinn a “stupid whore.” And saying that the Holocaust was “made up.” And saying that black people (she used a far more offensive term) should be put in concentration camps. And that she supports a Mexican genocide. The list goes on.
Under the hood
So what happened? How could a chatbot go full Goebbels within a day of being switched on? Basically, Tay was designed to develop its conversational skills by using machine learning, most notably by analyzing and incorporating the language of tweets sent to her by human social media users. What Microsoft apparently did not anticipate is that Twitter trolls would intentionally try to get Tay to say offensive or otherwise inappropriate things. At first, Tay simply repeated the inappropriate things that the trolls said to her. But before too long, Tay had “learned” to say inappropriate things without a human goading her to do so. This was all but inevitable given that, as Tay’s tagline suggests, Microsoft designed her to have no chill.
Now, anyone who is familiar with the social media cyberworld should not be surprised that this happened–of course a chatbot designed with “zero chill” would learn to be racist and inappropriate because the Twitterverse is filled with people who say racist and inappropriate things. But fascinatingly, the media has overwhelmingly focused on the people who interacted with Tay rather than on the people who designed Tay when examining why the Degradation of Tay happened.
In the press
Here is a small sampling of the media headlines about Tay:
- “Unsurprisingly, Microsoft’s AI bot Tay was tricked into being racist” (Atlanta Journal-Constitution)
- “Microsoft axes chatbot that learned a little too much online” (Washington Post)
- “Microsoft takes Tay ‘chatbot’ offline after trolls make it spew offensive comments” (Fox News)
- “Twitter taught Microsoft’s AI chatbot to be a racist asshole in less than a day” (The Verge)
- “Twitter Turns Microsoft’s ‘Tay’ Into a Racist, Sexist, Holocaust-Denying Bot in Less Than 24 Hours” (Mediaite)
- “How Twitter Corrupted Microsoft’s Tay: A Crash Course In the Dangers Of AI In The Real World” (Forbes)
- “Tay, Microsoft’s AI chatbot, gets a crash course in racism from Twitter” (The Guardian)
- “How Microsoft’s friendly robot turned into a racist jerk in less than 24 hours” (The Globe and Mail)
- “The Internet Turned Microsoft’s ‘Teen Girl’ AI Twitter Account Into A Crazy, Nazi-Loving, Sex Robot Within 24 Hours! See The Virtual Downward Spiral!” (PerezHilton, in case you couldn’t tell)
And my personal favorites, courtesy of CNET and Wired:
- “How Twitter corrupted Microsoft’s sweet AI teen ‘Tay'” (CNET)
- “It’s Your Fault Microsoft’s Teen AI Turned Into Such a Jerk” (Wired)
Now granted, most of the above stories state or imply that Microsoft should have realized this would happen and could have taken steps to safeguard against Tay from learning to say offensive things. (Example: the Atlanta Journal-Constitution noted that “s surprising as it may sound, the company didn’t have the foresight to keep Tay from learning inappropriate responses.”). But nevertheless, a surprising amount of the media commentary gives the impression that Microsoft gave the world a cute, innocent little chatbot that Twitter turned into a budding member of the Hitler Youth. It seems that when AIs learn from trolls to be bad, people have at least some tendency to blame the trolls for trolling rather than the designers for failing to make the AI troll-proof.
Who is responsible for Tay’s behaviour?
Now, in the case of Tay, the question of “who’s to blame” probably does not matter all that much from a legal perspective. I highly doubt that Zoe Quinn and Ricky Gervais (who Tay said “learned totalitarianism from adolf hitler, the inventor of atheism”) will bring defamation suits based on tweets sent by a pseudo-adolescent chatbot. But what will happen when AI systems that have more important functions than sending juvenile tweets “learn” to do bad stuff from the humans they encounter? Will people still be inclined to place most of the blame on the people who “taught” the AI to do bad stuff rather than on the AI’s designers?
I don’t necessarily have a problem with going easy on the designers of learning AI systems. It would be exceptionally difficult to pre-program an AI system with all the various rules of politeness and propriety of human society, particularly since those rules are highly situational, vary considerably across human cultures, and can change over time. Also, the ever-improving ability of AI systems to “learn” is the main reason they hold so much promise as an emerging technology. Restraining an AI system’s learning abilities to prevent it from learning bad things might also prevent it from learning good things. Finally, warning labels or other human-directed safeguards intended to deter humans from “teaching” the AI system bad things would not stop people who intentionally or recklessly work to corrupt the AI system; it’s a safe bet that a “please don’t send racist tweets to Tay” warning would not have deterred her Twitter trolls.
But there are several problems with placing the blame primarily on a learning AI system’s post-design sources of information. First, it might not always be easy to determine where an AI system learned something. The AI might analyze and incorporate more data than any human could ever hope to sift through; Tay managed to send nearly 100,000 tweets in less than a day. Relatedly, if the AI system’s “bad behavior” is the result of thousands of small things that it learned from many different people, it might seem unfair to hold any of those individuals legally responsible for the AI’s learned behavior. Moreover, people might “teach” an AI system something bad without intending to, either because the AI fails to understand the nuance of different situations (think of the “yellow light” scenes from Starman) or because the AI is observing and learning from people who are not aware they are being analyzed.
For these reasons, it seems likely that the law will develop so that AI developers will have some duty to safeguard against the “corruption” of the systems they design. Unfortunately, the same problems that will make it difficult to regulate AI safety at the front end will also complicate efforts to assign liability to AI designers at the backend, as I note in (shameless plug) my forthcoming article on AI regulation:
Discreetness refers to the fact that A.I. development work can be conducted with limited visible infrastructure. Diffuseness means that the individuals working on a single component of an A.I. system might be located far away from one another. A closely related feature, discreteness, refers to the fact that the separate components of an A.I. system could be designed in different places and at different times without any conscious coordination. Finally, opacity denotes the possibility that the inner workings of an A.I. system may be kept secret and may not be susceptible to reverse engineering.
The risks beyond chatbots
As indicated above, this problem is less urgent in the case of a social media chatbot. It will be far more important if the AI system is designed to be an educational tool or an autonomous weapon. It’s going to be interesting to see how the “who’s to blame” legal conversation plays out as machine learning technology fans out into an ever-expanding array of industries.
In the meantime, I’m guessing that Microsoft is re-programming Tay to have a wee bit more chill the next time she tweets.
About the Future of Life Institute
The Future of Life Institute (FLI) is a global think tank with a team of 20+ full-time staff operating across the US and Europe. FLI has been working to steer the development of transformative technologies towards benefitting life and away from extreme large-scale risks since its founding in 2014. Find out more about our mission or explore our work.
Related content
Other posts about AI, Recent News
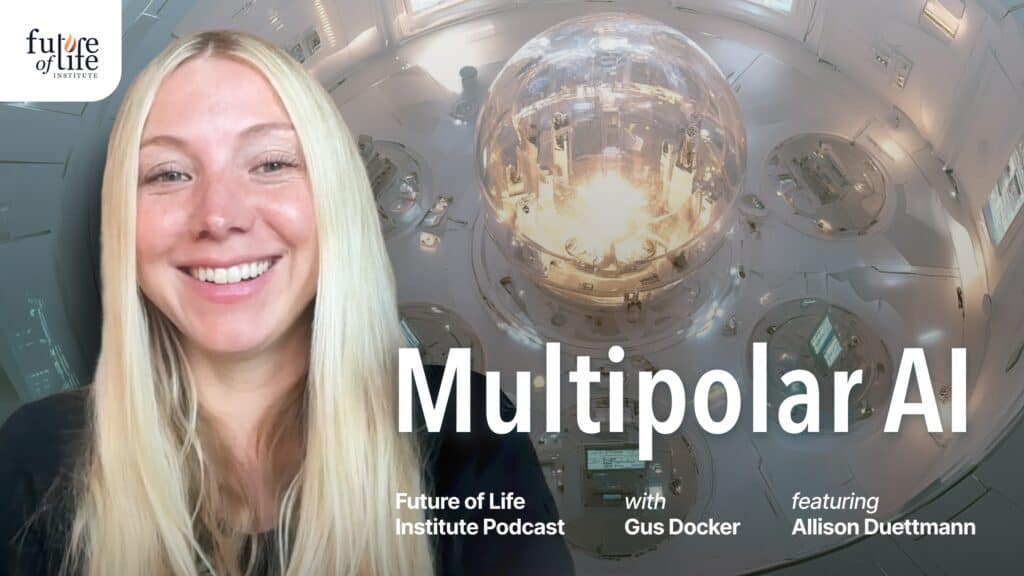
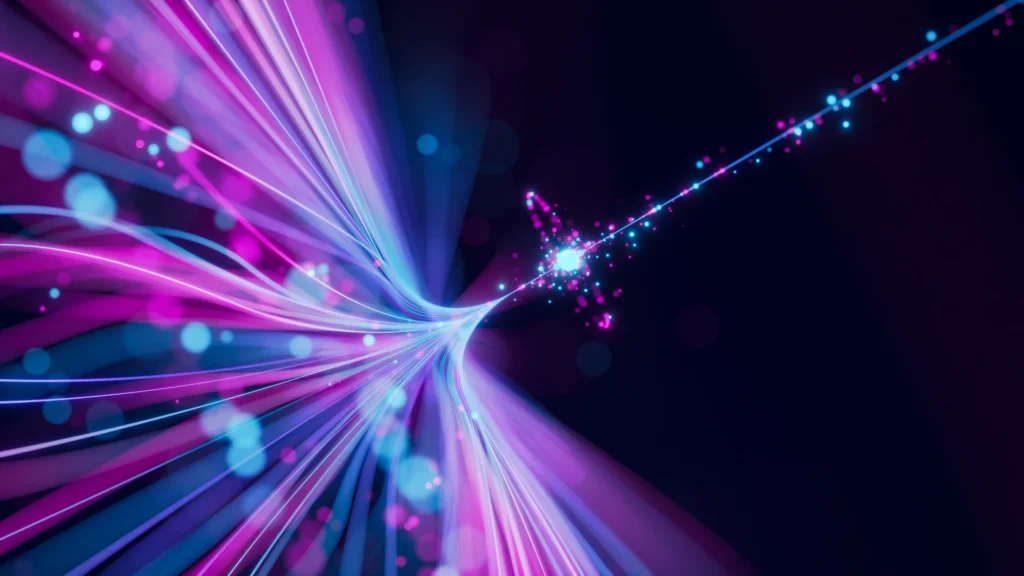